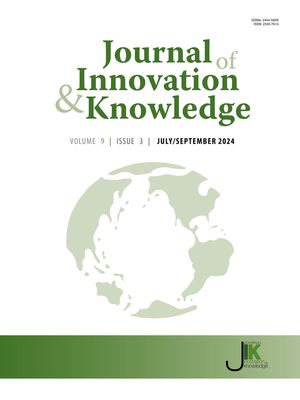
Edited by: Abbas Mardari
More infoIn the context of developing FinTech innovation, a commercial bank’s use of FinTech innovation can improve its risk management capability, thereby reducing its risk-taking. This paper explores the impact and mechanism of a bank’s FinTech innovation on its risk-taking using panel data of 65 commercial banks between 2008 and 2020. We innovatively construct a bank-level index based on web crawler technology and obtain the annual numbers of news items about a bank’s FinTech innovation from each bank in Baidu News. The empirical results show that improvement in the bank’s FinTech innovation significantly reduces its risk-taking. To overcome endogenous problems, including measurement errors and omitted variables, we use the instrumental variables (IV) and difference-in-differences (DID) methods to test the hypothesis and obtain consistent estimated results. The mechanism analysis shows that banks rely on FinTech innovation to reduce their risk-taking by improving their operating income and capital adequacy ratio, optimizing their operating performance, and improving their risk control capabilities. Further, a heterogeneity analysis shows that the effect of a bank’s FinTech innovation in reducing its risk-taking is more pronounced in larger, state-owned, joint-stock, and highly-competitive commercial banks. Our research results still hold after a series of robustness tests, including changing the construction methods of the bank’s FinTech innovation index, replacing the bank’s risk-taking indicators, tail-shrinking treatment, and changing samples. Our findings provide micro evidence for the application of FinTech innovation in commercial banks to reduce their risk-taking.
FinTech innovation is impacting commercial banks and has been changing their business practices. In order to better adapt to trends in FinTech innovation development, the commercial banking industry is accelerating digital transformation and improving the level of a bank’s FinTech innovation. However, there remains a lack of micro evidence as to whether a bank’s FinTech innovation currently impacts commercial banks’ risk-taking level. Existing studies find that various factors can affect the level of a bank’s risk-taking, including bank size (Khan et al., 2017), ownership structure (Berger and Bouwman, 2013), bank concentration (Efthyvoulou and Yildirim, 2014), the degree of competition in the banking market (Wagner, 2010), capital adequacy ratio (Chen et al., 2019a), GDP growth rate (Lozano-Vivas et al., 2001, 2002), and inflation (Pasiouras, 2008).
The increasing impacts of emerging FinTech companies have resulted in banks facing tremendous operating pressures, with effects on their risk-taking levels. Several studies have explored the impact of FinTech innovation on a bank’s risk from the perspective of non-bank FinTech innovation. Online loans illustrate the impacts of FinTech innovation on the traditional banking industry, as the rise of online loans can directly affect a bank's loan business with the emergence of competition with traditional banks (Buchak et al., 2018; Boot et al., 2021), and the end result is to squeeze the profitability of traditional banks. Simultaneously, the development of FinTech innovation has also increased the share of shadow banking in the United States (Buchak et al., 2018), raising the cost of bank debt as well as asset risk (Qiu et al., 2018). In the context of the external squeeze from FinTech and internal risk, meanwhile, and following the impact of the COVID-19 epidemic, banks are accelerating their pace of FinTech innovation, using FinTech innovation to build data platforms and gradually create a comprehensive risk-control system in order to better adapt to market changes and hedge against the impact from external FinTech companies so as to achieve rapid development. Thus, we focus on micro-level evidence and study the impact of banks applying FinTech innovation on their risk-taking.
The findings of existing studies show that commercial banks can enjoy the benefits of the technology spillover effect by using FinTech innovation, such as optimizing operating performance and improving risk control capabilities. In terms of operating performance, commercial banks can be empowered by FinTech innovation to augment service options, meet the diverse needs of customers, and boost their growth space (Gomber et al., 2017), thus improving profitability. In terms of risk control, FinTech innovation can use advanced technologies, including biometrics and voice recognition, to reduce labor, capital, and time costs in order to improve data accuracy, which in turn can reduce the internal risk of fraud as well as systemic risk (Fuster et al., 2019). In addition, FinTech innovation can also combine with banks’ loan services to reduce information asymmetry between banks and borrowers, thereby making banks more secure and flexible (Gomber et al., 2017) while reducing the probability of borrowers’ defaulting. Thus, FinTech innovation may reduce commercial banks’ risk-taking. However, existing studies do not provide a clear answer to the question of the impacts of a bank’s FinTech innovation on its risk-taking. Most relevant studies are carried out from the perspective of macro FinTech innovation (Ernst and Young, 2019; Guo et al., 2020; Lee et al., 2021; Zhao et al., 2022) or merely demonstrate the impact of a bank’s FinTech innovation on a single indicator, such as liquidity and asset quality (Cheng and Qu, 2020).
Therefore, panel data of 65 commercial banks from 2008 to 2020 are used to examine the impact of a bank’s FinTech innovation on its risk-taking through empirical analysis, and the following findings are obtained. First, the ordinary least squares (OLS) estimation method is used in the research, and the corresponding finding is that a bank’s FinTech innovation can reduce its risk-taking. For every 1% increase in the development of a bank's FinTech innovation, its risk-taking level can be decreased by 9.4% accordingly. Second, due to some endogeneity problems, such as autocorrelation, in the OLS estimation method, the Peking University Digital Financial Inclusion Index (PKU-DFII) is used in our study as an instrumental variable (Guo et al., 2020) for the second estimation, and consistent results are obtained. Moreover, another value for regression is used in the paper. That is, the value with a lagged one period of bank FinTech innovation is an alternative to the current value, and the results remain consistent. Another finding is that after promulgating a report titled “Guiding Opinion on Promoting the Healthy Development of Internet Finance” in 2015, the bank’s FinTech innovation has increasingly flourished. Therefore, we use the difference-in-differences (DID) method to further verify the relationship between a bank’s FinTech innovation and its risk-taking, and the results remain unchanged. Third, through mechanism analysis, one finds that a bank’s FinTech innovation reduces its risk-taking by improving operating income and capital adequacy ratio. Fourth, according to our heterogeneity analysis findings, the effect of a bank’s FinTech innovation in reducing its risk-taking is more pronounced among larger, state-owned, joint-stock, and highly-competitive commercial banks. Fifth, the research results are still valid following a series of robustness tests, including changing the construction method of a bank’s FinTech innovation index, replacing a bank’s risk-taking indicators, tail-shrinking treatment, and changing samples.
The three contributions of the paper to the existing literature are as follows. First, the study of FinTech innovation effects has been enriched by examining the impact of a bank’s FinTech innovation on its risk-taking through empirical analysis from the bank’s perspective. Most of the existing studies focus on the composition and characteristics of FinTech (Chen, 2016; Gomber et al., 2017), the impact of FinTech start-ups (Giaretta et al., 2021), and the impact of FinTech development on the economy (Chen et al., 2019c; Zhu, 2019; Chiu and Koeppl, 2019), or describe the pros and cons of FinTech on the development of banks (Anagnostopoulos, 2018; Drasch et al., 2018). Furthermore, most of the researchers conduct only qualitative analysis. Of course, other studies have explored the impact of FinTech innovation on a bank’s risk-taking using empirical analysis from the perspective of macro FinTech innovation (Ernst and Young, 2019; Guo et al., 2020; Lee et al., 2021; Zhao et al., 2022). Moreover, a few articles, based on a bank’s FinTech innovation level, have created a bank’s FinTech innovation index using the relevant vocabulary and have used empirical analysis to determine the impact of a bank’s FinTech innovation on its performance (Cheng and Qu, 2020). However, articles based on a bank’s FinTech innovation using empirical analysis to verify the relationship between a bank’s FinTech innovation and its risk-taking are relatively scarce. For this reason, our research has enriched the existing body of literature, offering new ideas and perspectives related to this research direction.
Second, based on the Baidu big data search engine, we innovatively construct a metric to measure the development of FinTech innovation at the bank level, compensating for the shortcomings of the existing literature on the application level of a bank’s FinTech innovation. At present, the FinTech innovation index is established from the broad perspective of demand or supply sides in most articles. For example, Ernst and Young (2019) have used the proportion of FinTech users in the financial market to construct the FinTech innovation index from the demand side; Guo et al. (2020) have used the PKU-DFII to measure the degree of FinTech innovation development; International Monetary Fund (IMF) (2017), Klynveld Peat Marwick Goerdeler (KPMG) (2017), and other research institutions believe that FinTech companies’ scale and venture capital can be used as indicators to measure FinTech innovation development, and the diversification of financial services provided by FinTech companies can also reflect the development of the industry (EBA, 2017). Zhao et al. (2022) applied Principal Component Analysis (PCA) from the supply side, based on the data of Chinese FinTech start-ups as well as the total registered capital and financing rounds from 2003 to 2018, to construct an annual FinTech innovation index, while Lee et al. (2021) used a similar approach to construct a FinTech innovation index from the perspective of technology providers. In addition, other scholars have constructed a FinTech innovation index at the bank level. Cheng and Qu (2020), using the word frequency statistics method in the text mining method, have constructed FinTech innovation indexes for each sub-region of banks. From the perspective of the degree of regional FinTech innovation development, although the above methods can measure the overall level of FinTech innovation, they cannot precisely describe the development of a bank’s FinTech innovation. Cheng and Qu (2020) have constructed a bank’s FinTech innovation index at the bank level based on the five dimensions of artificial intelligence, blockchain, cloud computing, big data, and internet technology. Their research comprises a mere 20 terms1, which are limited to essential keywords related to a bank’s FinTech innovation, such as digital currency, online banking, etc. This limitation may cause a deviation in a bank’s measurement and make it difficult to accurately measure a bank’s overall FinTech innovation development level.
In the above articles, the word frequency statistics method is used to measure the developing level of a bank’s FinTech innovation using the number of search results of a bank’s FinTech innovation keywords in Baidu News to assess the effect of a bank’s FinTech innovation on its risk-taking. Here are three innovations of our research. The first innovation is to enrich the bank’s FinTech innovation lexicon. The bank’s FinTech innovation keywords adopted in this paper have almost covered the standard terms displayed in the existing literature. After data cleaning, some of the keywords that contained any negative words, for example, “no” or “none,” have been deleted, and 35 terms are identified after combining similar terms2. Newly-added terms have enriched the lexicon of Cheng and Qu's research (2020) and provided references for subsequent research. The second innovation is the change in crawler technology. A script crawler framework is used in the paper to add “in-title” when sending Uniform Resource Locator (URL) requests to the Baidu website to achieve accurate matching at the title level and reduce interferences from irrelevant information. The third innovation is the improvement in the bank’s FinTech innovation dimensions. According to the structural discrepancy, the bank-level FinTech innovation index3 has been constructed in the paper from two dimensions: technology foundation and technology application. The newly-constructed index is able to extend the research content of this paper and provide additional research ideas for similar topics.
Third, we have innovated in influencing the factors affecting banks’ risk-taking, significantly expanding the impact of FinTech innovation on commercial banks’ risk-taking. The existing literature mainly emphasizes bank size (Khan et al., 2017), ownership structure (Berger and Bouwman, 2013), bank concentration (Efthyvoulou and Yildirim, 2014), degree of bank market competition (Wagner, 2010), and capital adequacy ratio (Chen et al., 2019a) as research variables to study the impact of these variables on banks’ risk-taking. Based on the limitations of the present research, there are three innovations in terms of influencing factors of a bank’s risk-taking in our study. First, a mediating effect method is adopted in the paper to analyze the transmission channels through which a bank’s FinTech innovation affects its risk-taking. Although Lee et al. (2021), Zhao et al. (2022), and other scholars have discussed the impact of FinTech innovation on commercial banks’ risk-taking, they do not discuss the specific impact mechanism. We have thus made up for the deficiencies of previous research in the paper. Second, we innovatively propose to use operating income and capital adequacy ratio as mediating variables to enrich the paper’s mechanism analysis. Since operating income can be a measurement of bank operating status, operating income is used as a substitute variable of bank operating performance in the paper. Third, we have synthesized the existing literature (Filip et al., 2017; Liberti, 2018; Banna et al., 2021; Sheng, 2021) to investigate the heterogeneous impact of bank size, ownership structure, and competitiveness on a bank’s FinTech innovation and its risk-taking.
The paper is structured as follows: the background of the study is provided in Section 2; in Section 3, the impact of the bank’s FinTech innovation on its risk-taking from a theoretical perspective is analyzed; Section 4 presents the data, variables, and analytical model; Section 5 shows the main findings, including mechanism, heterogeneity, and robustness analysis; and Section 6 is the conclusion.
Overview of FinTech development in Chinese commercial banksThe development history of FinTech in Chinese commercial banksAt present, commercial banks are in an information technology online construction period, and the development of FinTech has gone through roughly three stages. The first stage is the FinTech 1.0 era, when finance improved the electronic and automated level of office and business through the application of traditional Information Technology (IT), thus improving business efficiency. The change at this stage is that commercial banks began to adopt Automated teller machines (ATMs) to replace counters and tellers and credit cards to partially replace the role of cash. The second stage is the FinTech 2.0 era, as seen in the Internet finance stage. Banks expanded customer channels through online business platforms on the Internet or mobile terminals, enabling the interconnection of any combination of the asset side, transaction side, and capital side of the business, which is essentially a change in the traditional financial channels and the integration of business. The changes in this stage are reflected in the emergence of third-party payments, including Alipay and Yu'e Bao, which began to squeeze banks’ profits and directly compete with banks. Currently, we are facing the FinTech 3.0 era. Banks are enhancing the efficiency of traditional finance and bringing new financial services capabilities via IT technologies, such as artificial intelligence, big data, cloud computing, and blockchain. At this stage, commercial banks are beginning to optimize their own business by using cloud computing, big data, and other technologies, fully deploying digital transformation (Chen et al., 2022), and building middle office and digital systems.
The development status of banks’ FinTech innovationThe relevant data are counted in Table 1, and the trend in commercial banks’ FinTech innovation development is portrayed in Fig. 1. As shown in Fig. 1(1), the overall development of commercial banks’ FinTech innovation Index (FTII) shows an increasing trend. However, there was an inflection point in 2013; that is, from 2013 to around 2015, the level of commercial banks’ FinTech innovation declined. One possible reason for this phenomenon is a large number of collapses of online Peer-to-Peer lending platforms occurring during this period. The regulators were increasingly stricter in their supervision of FinTech innovation, which reduced the development level of banks’ FinTech innovation.
FinTech innovation index statistics of technology foundation and application of different types of commercial banks.
Development trends of FinTech innovation in commercial banks66 In the figure, State-owned, Joint-stock, City, and Rural represent state-owned commercial banks, joint-stock commercial banks, city commercial banks, rural and village commercial banks, respectively; FTII is the banks’ FinTech innovation index. In general, TF-FTII is the technology foundation index of FinTech innovation, and TA-FTII is the technology application index of FinTech innovation.
Fig. 1(2) shows the overall trend in FinTech innovation in different types of commercial banks going upward. However, there are significant differences in the level of FinTech innovation development between State-owned and Joint-stock commercial banks and other banks. From the overall trend in banks’ FinTech innovation, there is little difference in the development level of FinTech innovation among State-owned and Joint-stock commercial banks, which are at the forefront of all kinds of commercial banks developing FinTech innovation followed by city commercial banks. Rural commercial banks began to focus on FinTech innovation only around 2015 due to their significant differences in resources and capabilities from other kinds of banks; hence their FinTech innovation development level is the lowest among all kinds of commercial banks. Fig. 1(3) and (4) show that the development trend of different types of commercial banks in terms of FinTech innovation’s technology application (TA-FTII) and technology foundation (TF-FTII) index is also generally upward. Moreover, the development of State-owned and Joint-stock commercial banks is still in the leading position.
Theories and hypothesesGenerally speaking, FinTech innovation affects the development of banks from two aspects: external FinTech innovation and a bank’s FinTech innovation. External FinTech innovation refers to FinTech other than banks, including FinTech companies, which can influence the development of commercial banks through competitive effects and technology spillovers (Cheng and Qu, 2020). The impact on the banking industry is examined in most of the existing studies from the perspective of FinTech innovation external to banks, whereas we have examined the impact of a bank’s FinTech innovation on its risk-taking from a micro-level perspective.
FinTech innovation enhances banks’ risk control capabilities by improving capital adequacy ratiosFrom a risk control perspective, commercial banks can obtain technology spillovers by using emerging technologies (Cheng and Qu, 2020), improve risk control capabilities and management efficiency (Newman et al., 2015), and improve internal governance and controls, thus increasing the diversification of banks to reduce their credit risk (Demirgüç-Kunt and Huizinga, 2010).
Specifically, during the pre-loan period, for individual borrowers, banks can use “big data” to find potential borrowers, predict their behaviors (Pérez-Martíet et al., 2018), and reduce credit risk (Sheng, 2021). For corporate borrowers, FinTech innovation can help banks improve the availability and accuracy of the information, increase the number of channels and sources of information, and reduce information frictions between banks and SMEs (Sánchez, 2018). In the mid-loan process of credit review, on the one hand, banks build long-term stable relationships by increasing investments to improve digital inclusion, shorten the physical distance to customers, and help uninformed customers collect quality information that helps establish an accurate customer risk profile (Hauswald and Marquez, 2006). On the other hand, based on automated credit scoring and credit decision systems, banks can prevent risks by scoring key indicators and matching them with their risk appetite to arrive at a credit limit following a comprehensive evaluation. In the post-loan management process, on the one hand, FinTech innovation can promote information sharing among lenders, restrain the behavior of borrowers, and improve the ability of lenders to handle risk information (Livshits et al., 2016), thus reducing the risks associated with loans (Sutherland, 2018). On the other hand, big data can help banks identify fund usage irregularities or other potential default risks in a timely manner, and relevant machine learning is used to dispose of risks and arrive at specific solutions for different risk categories. Moreover, in terms of internal control, a bank’s FinTech innovation, such as advanced technologies, including biometrics, voice recognition, and intelligent robots, can be used to reduce human, financial, and time costs and improve the accuracy of data, thus reducing a bank’s risk of fraud as well as systemic risk (Fuster et al., 2019).
In conclusion, banks’ credit systems developed based on big data and other technologies can reduce transaction risk (Dynan et al., 2006) and weaken commercial banks’ risk-taking incentives. Moreover, the system can also improve banks’ pre-loan, mid-loan, and post-loan review capabilities, thus reducing their risk-taking levels (Sutherland, 2018). As a result, Hypothesis 1 is proposed for the paper, namely, that a bank’s FinTech innovation can improve its risk control capabilities and thus reduce its risk-taking levels.
FinTech innovation reduces banks’ willingness to take risks by improving operational performanceFrom a business operation perspective, commercial banks can take advantage of using big data, artificial intelligence, and other kinds of technologies. On the one hand, banks can process small and medium-sized enterprises and individual transactions, solve the original problem of the high cost of acquiring long-tail customer information and improve customer experience and convenience by meeting diversified customer needs (Stulz, 2019), thus improving their profitability. On the other hand, commercial banks can enhance the development space (Gomber et al., 2017) by absorbing advanced technology concepts to improve their traditional operational efficiency and offer more services.
Specifically, in terms of cost reduction, many studies have shown that the application of emerging technologies can reduce transaction costs and fundamentally change financial services. On the one hand, in the traditional credit model, commercial banks must expend much time and effort on the pre-loan assessment, and the cost of post-loan risk and bad debt disposal due to information asymmetry is also very high. However, nowadays, commercial banks can use big data and cloud computing technologies to quickly query customer information, dramatically dropping the cost of the entire process of credit review by banks. FinTech innovation can also help banks reduce the information asymmetry problem arising from distance barriers, improve information sharing within the financial sector (Law et al., 2018), and reduce transaction costs (Liberti, 2018; Grennan and Michaely, 2021). On the other hand, in the past, if traditional commercial banks wanted to provide more financial services to their customers, they had to open additional branches and increase the cost of leasing, personnel, and equipment. However, with the empowering effect of FinTech innovation, commercial banks can provide services to customers 24 hours a day regardless of time and space constraints, which dramatically reduces branch layout and operation costs (Chiu and Koeppl, 2019). In terms of improving performance, FinTech innovation helps banks to further expand their business (Campanella et al., 2017) and improve their performance. The Financial Stability Board (FSB) points out that FinTech is low-cost and high-efficiency, which not only promotes the availability of financial resources, improves the symmetry of transaction information, and enhances disintermediation in resource allocation (FSB, 2016) but also enhances the efficiency of the financial sector and broadens the business boundaries of traditional finance. Compared with banks’ traditional business model, FinTech innovation can provide financial services to different consumers more conveniently and efficiently to meet their diversified needs (Lee et al., 2021). In addition, banks can use digital financial technologies to gain “digital” benefits in terms of deposit and loan diversification, thus reducing intermediary costs and integrating technology more efficiently (Heredia et al., 2022), and increasing stable deposits (Danisman and Tarazi, 2020). FinTech innovation also complements traditional industries, as banks have the advantage of accumulating a large amount of customer information and transaction data (Dorfleitner et al., 2017), and these data advantages can help banks achieve better business performance (Begenau et al., 2018).
In conclusion, FinTech innovation based on cloud computing can not only improve the efficiency of banks’ internal management, make information communication among departments more efficient, and help banks expand their organizational model (IMF, 2017), achieve technological progress, and increase productivity (Berger, 2003), but also make up for a bank's shortcomings in profitability and risk management, increase business performance, and reduce a bank’s willingness to engage in risky business, thus reducing risk-taking. Hypothesis 2 is therefore proposed: a bank’s FinTech innovation can improve its business performance and thus reduce risk-taking levels.
FinTech innovation has heterogeneous impacts on different types of banksIn addition, we find heterogeneous effects of banks’ FinTech innovation on their risk-taking. First, Khan et al. (2017) found that bank size and capital buffers usually limit banks from taking more risk by examining the relationship between financing liquidity and bank risk-taking. Large banks are usually risk-averse because they do not suffer from liquidity shortages and can use large amounts of capital for FinTech innovation and reduce financing costs (Bertay et al., 2013), whereas small banks may have a stronger urge to take risks (Banna et al., 2021). Second, scholars in general suggest that banks behave differently under different ownership structures (Huang et al., 2017). For example, State-Owned Commercial Banks (SOCBs) differ relatively from other banks in that they have a specific social responsibility, the obligation to finance the government, and state-owned enterprises (Lee and Huang, 2016, 2017, 2019), which can influence their risk-taking behavior. Further, Boyd and De Nicolo (2005) suggest that banks may enhance their risk-taking behavior in less competitive markets (FSB, 2017; Anagnostopoulos, 2018). However, the effect of market competition on banks is reversed by adjusting their loan portfolios (Wagner, 2010). Martinez-Miera and Repullo (2010) argue that there is a U-shaped relationship between competition and risk but that the relationship exists only in the loan market (Jiménez et al., 2013). Specifically, large banks, which are more experienced in transacting loans than small banks, can use FinTech innovation to improve their lending technology (Sheng, 2021), eliminate biases in human data collection or real-time decision making, simplify information transfer, and reduce costs, thus facilitating continuous improvement in lending transactions (Cenni et al., 2015), whereas smaller banks have been slower to embrace and adopt FinTech innovation (Filip et al., 2017).
Therefore, differences in the characteristics of various banks in the development of FinTech innovation can lead to the formation of heterogeneity in the risk-taking of FinTech innovation for banks with different asset sizes, bank types, and competitiveness. Thus, we propose Hypothesis 3, namely, the development of a bank’s FinTech innovation has a heterogeneous impact on the level of risk-taking of commercial banks with differences in size, bank type, and competitiveness.
Data and methodsDataThe data of commercial banks’ reports used in this paper are obtained from the CSMAR and WIND database. The bank FinTech innovation index is an indicator measuring the number of FinTech innovation news-related keywords in Baidu news obtained by the authors through the web crawler, and the macroeconomic data are collated from the official websites of the National Bureau of Statistics and the People’s Bank of China. The sample data are processed to make them more representative using the following steps. First, we eliminate the samples with main variable data for less than five consecutive years; second, we fill in a small amount of missing data by linear interpolation and average interpolation. Following the above processing, the final sample is determined as the annual balanced panel data of 65 commercial banks from 2008 to 2020, including 6 state-owned commercial banks, 10 joint-stock commercial banks, 29 city commercial banks, 17 rural commercial banks, and 3 village banks4.
VariablesCurrently, the measurement index of commercial banks’ risk-taking can be considered from two aspects. First, based on corporate governance theory, the perspective of bank insolvency is considered. The indicators considered in the existing literature are Z-value (Danisman and Tarazi, 2020; Zhao et al., 2022), non-performing loan ratio (Papadopoulos, 2019; Cheng and Qu, 2020), and asset return variance. Second, based on the Basel Accord, the perspective of regulatory authority is considered. The indicators used in existing studies to measure risk-taking are asset-to-capital ratio (Zhao et al., 2022), deposit-to-loan ratio (Zhao et al., 2022), risk-weighted asset ratio (Qiu et al., 2018), and risky asset ratio (Laeven and Levine, 2009). However, from the perspective of corporate governance, such indicators measure a bank’s ex-post risk and cannot fully reflect the bank’s own risk-taking. From a Basel perspective, as there are too many missing values in the risk-weighted asset ratio, we refer to Zhao et al. (2022), select the asset-capital ratio to measure the risk of commercial banks, and use the Z-score5 and the deposit-lending ratio (DRISK) for robustness testing.
In this paper, we refer to the methods used to construct the FinTech innovation index adopted by IMF (2017), KPMG (2017), EBA (2017), Ernst and Young (2019), Guo et al. (2020), Cheng and Qu (2020), Lee et al. (2021), and Zhao et al. (2022). To construct the FinTech innovation index, we obtain the number of news items related to the FinTech innovation of each bank through Baidu News Advanced Search, for example, “Commercial Bank of China + mobile payment,” and after summation and normalization of the number of the bank’s FinTech innovation words, we obtain the bank’s FinTech innovation index (FTII). The larger the FTII, the higher the degree of commercial banks’ FinTech innovation. In addition, according to the structured difference, we further divide the bank’s FinTech innovation index into technology foundation (TF-FTII) and technology application (TA-FTII) to improve the content of this study.
Following Alqahtani et al. (2016) and Lee and Lee (2019), the following control variables are selected for this paper: SIZE, OVER, INV, ROA, INF, and SGDP. The calculation of the above variables can be found in Table 2.
Definition of main variables6.
The essential statistical characteristics of the main variables are reported in Table 3. The mean value of commercial banks’ risk-taking is 15.197, the minimum value is 4.251, and the maximum value is 60.612, which indicates that there are significant differences in the risk-taking levels of different commercial banks. The mean value of the commercial bank’s FinTech innovation index is 0.018, the minimum value is 0, and the maximum value is 1, which indicates that there are significant differences in the degree of FinTech innovation development of different commercial banks and that some commercial banks have not even begun to apply FinTech innovation. Individual differences are relatively noticeable in other control variables, which indicates that the sample has good differentiation.
Descriptive statistics.
Note: The definitions and descriptive statistics (number of observations, mean, standard deviation, minimum and maximum values) are provided in the table for the variables used in this study.
The risk-taking level of commercial banks is influenced by bank FinTech, individual characteristics at the bank level, and macroeconomic variables. For this reason, based on the above data and variables, with reference to Zhao et al. (2022) and Lee et al. (2021), the following balanced panel regression model is developed in this paper:
Where RISK is the asset-to-capital ratio, which measures the risk-taking level of commercial banks and FTII is the core explanatory variable, which measures the FinTech innovation index of commercial banks. Control is a set of control variables considered in this paper; the subscript i denotes the sample banks, t represents the year, α1 and γ are regression coefficients, δi is bank fixed effects, and εit is the random disturbance term.
After controlling the heteroskedasticity-corrected standard errors, an ordinary least squares (OLS) approach is adopted to verify the impact of bank FinTech innovation on its risk-taking. To reduce the potential endogeneity problems associated with reverse causality, referring to Ahamed and Mallick (2019) and Kim et al. (2020), a two-stage least squares-instrumental variables approach (2SLS-IV) is used, as well as a systematic GMM approach for testing. In addition, the research sample has been categorized three individual times: first, regarding size, the sample banks have been divided into large-scale and small-scale banks; second, using bank type as the division criterion, the sample is divided into state-owned banks, joint-stock banks, city commercial banks, rural and village commercial banks; third, in terms of bank competitiveness, sample banks have been divided into more competitive and less competitive commercial banks. Lastly, the heterogeneity is tested separately in each category.
ResultsBaseline regressionThe regression results are shown in Table 4 below. Columns (1) and (2) are the baseline regression results, which are merely regressed for a bank’s FinTech innovation index and a commercial bank’s risk-taking, and the coefficients of these two variables are both significantly negative at the 1% level, indicating that a commercial bank’s development of FinTech innovation, in general, reduces its risk-taking level. Column (3) adds bank-level control variables to column (2), and column (4) adds macro-level control variables to column (3). Both results indicate that a commercial bank’s FinTech does reduce its risk-taking level.
Impact of a bank's FinTech innovation on its risk-taking.
Note: *** and ** indicate significance at the statistical levels of 1% and 5%.
In terms of control variables, the larger the bank, the more pronounced its reduction effect of developing FinTech innovation on risk-taking. On the one hand, a large-sized bank would have a dramatic impact once risks arise; hence such banks are more cautious in their operations. On the other hand, large-sized banks can take advantage of their scale and make better use of FinTech innovation, thus reducing their risk-taking level. The improvement in banks’ profitability and the increase in income diversity will also have the effect of reducing risk-taking. Because banks can obtain high returns through their current operating status, there is no need to take more significant risks, which will correspondingly reduce their risk-taking motivation. Lastly, at the macro level, the larger scale of social financing indicates the higher demand for capital from enterprises or individuals in society, and banks are likely to increase loans to meet the demand for social financing, which in turn will lead to a corresponding increase in risk.
Endogeneity issuesSystem GMM methodTo reduce potential endogeneity problems, a system GMM approach (Blundell and Bond, 1998) is used in the paper to test the baseline results. On the one hand, the system GMM approach can eliminate the strict exogenous assumptions of the regressions, unobserved bank-specific effects, and path-dependence in the sequence of control-dependent variables. On the other hand, since bank risk may persist over time, the system GMM allows for the dynamic modeling of bank risk. Considering that bank risk has dynamic continuation effects, the current risk-taking level may be influenced by the risk level from the previous period. Therefore, a one-period lagged explanatory variable, L.RISK, is introduced in the paper to construct a dynamic panel model, and the GMM estimation method is used to test the baseline results. As shown in column (1) of Table 5, the regression coefficient of the core explanatory variable FTII is significantly negative, which is consistent with the previous regression results; thus, the results of this paper are not affected by potential endogeneity bias and show good robustness.
Endogeneity test results.
Note: *** and ** indicate significance at the statistical levels of 1% and 5%.
FinTech innovation can influence the risk-taking level of commercial banks, and likewise, commercial banks will take the initiative to seek technological changes and develop FinTech innovation for self-development. Therefore, there may be a causal relationship between FinTech innovation and commercial banks’ risk-taking. Potential endogeneity can be reduced using the following two instrumental variable approaches (Roberts and Whited, 2013):
- (i)
A commercial bank’s FinTech innovation will be influenced by the external digital environment. If the external digital environment is favorable, it will promote the bank’s interaction with the external environment, strengthen strategic cooperation with other FinTech companies or enterprises, and promote the bank’s FinTech innovation. To demonstrate whether the difference in digital development levels in the region where the bank is located affects the impact of its FinTech innovation on risk-taking, referring to Guo et al. (2020), the provincial-level digital financial inclusion index compiled by the Digital Finance Research Center of Peking University is used as an instrumental variable (PKU-DFII) to measure the level of regional digital development, after which two-stage least squares (2sls) is applied for estimation. Before estimation, a weak instrumental variables test with an F-value greater than 10 is conducted, indicating that there is no problem with weak instrumental variables and that the instrumental variables are valid. The estimation results of the instrumental variables method are shown in column (2) of Table 5, where the coefficients of the core explanatory variables remain negative, indicating that the development of the bank’s FinTech innovation can significantly reduce its risk-taking, which is entirely consistent with the previous results.
- (ii)
Given that a commercial bank’s risk-taking in the current period does not affect the FinTech innovation development level in the previous period, with reference to Sheng’s study (2021), a one-period lagged bank’s FinTech innovation index is used to replace the current period value for re-estimation. The results are shown in column (3) of Table 5, and the coefficient of FTII is significantly negative at the level of 1%, so the research results remain robust.
The causal relationship between a bank’s FinTech innovation and its risk-taking is analyzed using policy shocks regarding the development of the bank’s FinTech innovation in the paper. In July 2015, the People’s Bank of China, with relevant ministries and commissions, took the lead in drafting, formulating, and issuing the “Guidance on Promoting the Healthy Development of Internet Finance”. In accordance with the general requirements, “encouraging innovation, preventing risks, drawing on advantages and avoiding disadvantages, and developing healthily”, a series of policy measures were proposed in the document to encourage innovation and support the stable development of the Internet. As this policy plays a significant role in promoting the development of banks’ FinTech innovation, we take this guideline as a policy shock for banks’ FinTech innovation (Cheng and Qu, 2020) and use the difference-in-differences method (DID) to reduce the potential intrinsic bias in the regression model. Specifically, according to the statistical eigenvalues of bank-level variables, the original samples are divided into treatment groups and control groups in the paper (Chen et al., 2019b; Deng et al., 2020). First, the mean value of a bank’s FinTech innovation development level is used as the criterion to divide the original sample into banks with a high level of FinTech innovation (treatment group) and banks with a low level of FinTech innovation (control group). The level of a bank’s FinTech innovation in the treatment group is higher than the mean value, and the level of a bank’s FinTech innovation in the control group is lower than the mean value. Next, we constructed the following DID model:
Where the coefficients of Postit*Treatit reflect the treatment effect of this policy on a bank’s risk-taking. The subscript i denotes the individual bank, subscript t denotes the time, and RISKit is an indicator of a bank’s risk-taking. Treatit is a dummy variable that is 1 for banks with a high level of FinTech innovation and 0 for banks with a low level of FinTech innovation. If the bank sample is after the policy is enacted, Postit is 1, but if it is before the policy is enacted, the value is 0. Controlit are additional control variables, including management capacity (OVER), Inflation (INF), and social financing scale (SGDP). Banki is a fixed effect of the bank; ε is the error term. Before running the difference-in-differences models (DID), we perform a parallel trend test to test whether the data are feasible. The parallel trend test is shown in Fig. 2 below, and the results float around 0 for all six periods before the policy time point, indicating that the parallel trend hypothesis is supported and can be analyzed using the DID method.
Next, the regression is conducted using DID method in this paper, and the results are shown in Table 6. Column (1) shows the empirical results without adding control variables, and column (2) shows the empirical results after adding control variables. The coefficients of Post*Treat are significantly negative in both columns, indicating that the development of a bank’s FinTech innovation reduces its risk-taking. It can be seen that the results of this paper are not the result of potential endogeneity bias.
Impact of a bank's FinTech innovation on its risk-taking (DID method).
Note: ***, **, and * indicate significance at the statistical levels of 1%, 5%, and 10%, respectively.
The previous findings show that a bank’s FinTech innovation reduces its risk-taking, as evidenced by increased operating income and capital adequacy ratio. To further explore the mechanism of a bank’s FinTech innovation on its risk-taking, the analysis is carried out from two perspectives: increasing operating income and capital adequacy ratio. Drawing on the stepwise method of mediating effects proposed by Preacher and Hayes (2008), we construct a multiple mediating effects model consisting of four progressive equations, with the bank’s operating income and capital adequacy ratio as mediating variables.
According to the test steps of the multiple mediating effects model, the first step is to regress the model (3). If the regression results satisfy α1, which are significantly negative, the next step can be taken; otherwise, the test must be stopped. The second step is to regress model (4) and model (5) simultaneously. If β1 is significantly positive, the value means that a bank’s FinTech innovation will increase its operating income, thus enhancing the operating capacity of commercial banks; if γ1 is significantly positive, the value indicates that a bank’s FinTech innovation can significantly improve its risk control ability. The third step is to regress model (6). If the coefficients δ2 and δ3 are significant but δ1 is not significant, the results mean that business performance and financial control play the role of full mediator in the influence of a bank’s FinTech innovation on its risk-taking; if the coefficientsδ1, δ2 and δ3 are all significant, and the value of δ1 is decreased compared to α1, the results indicate that the two mediating variables have partial mediating effects. In the following empirical results, the contribution of the two mediating variables is further analyzed in terms of the reduction in risk-taking by a commercial bank’s FinTech innovation. Table 7 below shows the test results of the effect of a bank’s FinTech innovation on the level of risk-taking via two paths: business performance and risk control.
Mechanism test.
Note: ***, **, and * indicate significant at the statistical levels of 1%, 5%, and 10% respectively.
Column (1) of Table 7 shows the results of the baseline regression. Columns (2) and (3) show that the estimated coefficients of a bank’s FinTech innovation index (FTII) on capital adequacy ratio (CAR) and operating income (INC) are significantly positive, which indicates that a bank’s FinTech innovation significantly improves its business performance and risk-control ability. According to the regression results in column (4), after adding both the core explanatory variables and the mediating variables, the estimated coefficient of a bank's FinTech innovation index (FTII) is significantly negative, and the estimated coefficients of the mediating variables, capital adequacy (CAR), and operating income (INC) are also significantly negative. These results show that business performance and risk control play a partially mediating role in the effect of a bank’s FinTech innovation on its risk-taking.
In terms of the total contribution of the two types of mediating effects, the mediating effect of a bank’s FinTech innovation in reducing its risk-taking by risk control is -1.433 (-0.174 × 8.236), accounting for 15.3% of the total effect. The mediating effect of reducing its risk-taking by business performance is -0.635 (-0.181 × 3.507), accounting for 6.8% of the total effect. These results show the contributions of improving risk-control ability and business performance to explain the increase in a bank’s FinTech innovation, as the reduction in risk-taking has reached 15.3% and 6.8%, respectively. The empirical results effectively identify that a bank’s FinTech innovation affects risk-taking through the two paths, risk control capability and business performance, thus expanding the study of a bank’s FinTech innovation. Therefore, Hypotheses 1 and 2 are valid.
Heterogeneity analysisLevel of bank's FinTech innovation, bank size, and bank risk-takingStudies have shown that bank size and capital buffers usually limit banks from taking more risks (Khan et al., 2017). To verify this view, in line with research by Zhao et al. (2022), using bank size data from the Wind database, the SCALE of large and medium-sized banks is defined as large-scale banks, with a value of “1”; the SCALE of small and micro banks is defined as small-scale banks, with a value of “0”.
As shown in Table 8, column (1) is the result of the regression after adding the interaction term between the bank’s FinTech innovation index and the bank’s size (FTII*SCALE); that is, the larger the commercial bank, the lower its risk-taking. One possible reason for the above result is that commercial banks can take full advantage of the scale. On the one hand, they can diversify the allocation of resources to achieve the effect of risk diversification, improve their ability to manage and prevent risks comprehensively, and reduce risk-taking (López et al., 2011). On the other hand, they can save marginal costs, increase operating income, and reduce the incentive of risk-taking. Columns (2) and (3) are grouped regressions based on differences in bank size: column (2) shows the regression results of small-scale (micro and small) commercial banks, while column (3) shows the regression results of large-scale (medium and large) commercial banks. Both coefficients of columns (2) and (3) are significantly negative, and the coefficient of column (2) is smaller than that of column (3), indicating that the larger the bank is, the stronger the effect of its FinTech innovation in reducing risk-taking appears to be, which is consistent with the results in column (1). Thus, it is clear that Hypothesis 3 holds.
Heterogeneity test based on bank size.
Note: ***, **, and * indicate significance at the statistical levels of 1%, 5%, and 10%, respectively.
Existing studies generally maintain that banks differ under different ownership structures (Huang et al., 2017). For this reason, the division method of bank types in the Wind database is used, and bank types (TYPE) are divided into a group of state-owned and joint-stock commercial banks with a value of “1”, and a group of city, rural, and village commercial banks with a value of “0”.
As shown in Table 9, column (1) shows the regression results after adding the interaction term between the bank’s FinTech innovation index and the type of bank (FTII*TYPE). Compared with other kinds of banks, state-owned and joint-stock commercial banks have a more significant effect on the reduction of risk-taking in FinTech innovation development. One possible reason is that state-owned and joint-stock commercial banks not only pursue profits but also shoulder the social responsibility of maintaining financial stability. On the one hand, the regulatory systems and governance mechanisms of state-owned and joint-stock commercial banks are more mature and have better risk control capabilities due to facing additional risk management, information disclosure, and corporate governance. On the other hand, as state-owned and joint-stock commercial banks must consider more complex factors in the operation process, they are more cautious than other banks. Columns (2) and (3) are grouped regressions based on differences in bank types: column (2) shows the regression results of state-owned and joint-stock commercial banks, and column (3) are the regression results of city, rural, and village commercial banks. After grouping, the sample size is too small, so the conclusions obtained in this paper are not significant. The coefficient of column (2) is larger than that of column (3), which can indicate that the role of state-owned and joint-stock commercial banks in developing FinTech innovation to reduce risk-taking may be more vital. Thus, the conclusion is that Hypothesis 3 holds.
Heterogeneity test based on bank type.
Note: ***, **, and * indicate significance at the statistical levels of 1%, 5%, and 10%, respectively.
In addition, Boyd and De Nicolo (2005) indicate that banks may enhance their risk-taking behavior in less competitive markets (FSB, 2017; Anagnostopoulos, 2018). Therefore, referring to research by Banna et al. (2021), we analyze whether there is heterogeneity from the banking competition level to the relationship between a bank’s FinTech innovation index and its risk-taking. In the paper, the net interest margin (NII), which means the share of interest income in the interest-bearing assets, is used as a proxy variable for bank competition level. The higher the indicator, the less competitive the banking system. Since there are significant differences in competition levels among banks, it is difficult to distinguish the differences in banks’ competitiveness if the average value is directly used to judge the level of competition. Therefore, in this paper, the first quartile (25%) of net interest margin (NII) is used as the standard to divide the overall sample of banks. The samples of banks with NII above 25% belong to one group with a value of “1”, and the rest belong to another group with a value of “0”; they are then regressed separately.
The results are shown in column (1) of Table 10, where the interaction term (FTII*NII) between the level of a bank’s FinTech innovation and net interest margin is significantly positive; in contrast, the baseline regression results of this research are significantly negative. The results indicate that the higher a bank’s net interest margin is, the weaker the competitiveness of the banking system will be, and that the bank’s net interest margin inhibits a reduction in risk-taking caused by the bank’s FinTech innovation and vice versa. For banks with strong banking system competitiveness, the bank’s FinTech innovation exhibits more obvious effects on risk-taking. Columns (2) and (3) are the results of group regressions according to bank size. The coefficients of both regression results are significantly negative, and the coefficient of column (2) is larger than that of column (3). The results indicate that the higher the level of competition, the more significant the effect of FinTech innovation on the reduction in risk-taking, which is consistent with the results of column (1). Thus, the conclusion is that Hypothesis 3 holds.
Heterogeneity test based on bank competitiveness.
Note: ***, **, and * indicate significance at the statistical levels of 1%, 5%, and 10%, respectively.
To further illustrate the reliability and validity of the findings, we conduct robustness tests on the baseline regression findings from four perspectives: reconstructing explanatory variables, replacing explained variables, tail-shrinking treatment, and removing the samples prior to 2010.
As can be seen from Table 11, columns (1) and (2) are the regression results after replacing the measure of the FinTech innovation index (FTII). The explanatory variable (FTII) in the baseline regression measures the degree of FinTech innovation based on the summed word frequency of all words related to FinTech innovation in commercial banks. However, in column (1), we take only the vocabulary frequencies of words related to the technology foundation (TF-FTII) in the development of FinTech innovation in commercial banks. In column (2), we take only the vocabulary frequencies of words related to the technology application of FinTech innovation (TA-FTII) in commercial banks. The regression results are still significant, indicating that the conclusions of this paper are robust. Columns (3) and (4) are the regression results after replacing the risk-taking variables of commercial banks. For the baseline results, the capital-to-asset ratio (RISK) is used to measure the risk-taking level of commercial banks. To ensure the robustness of the empirical results, we refer to research by Danisman and Tarazi (2020), Houston et al.(2010), and Laeven and Levine (2009) to perform robustness tests using Z-score, and the results remain consistent with previous results. In addition, referring to research by Zhao et al. (2022), the deposit-to-loan ratio (DRISK) is used as a proxy variable for a commercial bank's risk-taking and the baseline results of the research regress once again. The re-regression results show that a bank’s FinTech innovation will increase its liquidity level by increasing its deposit-to-loan ratio so as to reduce risk-taking.
Robustness tests.
Note: ***, **, and * indicate significant at the statistical levels of 1%, 5%, and 10% respectively.
In Table 11, column (5) shows the regression results after the tailoring process. To eliminate the influence of some extreme values on the regression results, we apply 1% tailoring to all continuous variables in the sample, and the results are still consistent with the previous ones. Column (6) shows the regression results after deleting data prior to 2010. Firstly, during the period from 2008 to 2010, the level of bank FinTech innovation development was very shallow; secondly, the 2008 global financial crisis may still have had an impact on banking risk. Thus, to avoid the impact on the baseline results, we delete the sample data between 2008 and 2010 before regression and the results remain significant.
ConclusionUnlike most existing studies, this paper is not based on macro or regional FinTech innovation. Instead, our research is based on the perspective of bank FinTech innovation at the micro level to explore the impact on banks’ risk-taking. Using the panel data of 65 Chinese commercial banks from 2008 to 2020, we have innovatively constructed a bank-level FinTech innovation index using web crawler technology to count news items related to commercial bank FinTech innovation terms from Baidu News advanced retrieval. The results obtained are the following. First, the improvement of a commercial bank’s FinTech innovation can reduce its risk-taking levels in general; the higher the degree of FinTech innovation a commercial bank has, the lower the risk-taking levels it will bear. Second, the result of the mechanism analysis shows that a bank’s FinTech innovation reduces its risk-taking through two channels, namely, increasing operating income and capital adequacy ratio. Third, heterogeneity analysis of bank size, bank type, and competitiveness shows that larger, state-owned, joint-stock, and highly-competitive commercial banks have a more pronounced effect on risk-taking reduction in developing FinTech innovation.
In view of the above conclusions, we have some suggestions for commercial banks. First and foremost, all commercial banks should follow the era’s development pattern and embrace FinTech solutions to expedite their digital transformation based on their unique qualities. Second, as commercial banks’ usage of FinTech will entail certain potential dangers, all banks must increase risk management. Third, the government must enact applicable regulatory measures, such as standards for information disclosure and risk management indicators.
Simultaneously, there are some limitations in the research. First, due to data availability, only a sample of listed commercial banks is used in the research, one that does not cover unlisted commercial banks. Thus, further discussion is needed on the impact of banks’ FinTech innovation on the risk-taking of small and medium-sized banks. Second, the bank’s FinTech innovation index used in this paper was obtained through textual analysis. Further analysis is needed if more direct indicators of a bank’s FinTech innovation are to be obtained in the future. Third, only the impact of a bank’s FinTech innovation on its risk-taking for the time being was examined, which does not cover the impact on other bank aspects, which could be explored in future studies. Fourth, the definition of bank risk-taking in the paper is relatively narrow, and the specific impact of a bank’s FinTech innovation on its various types of risks was not discussed separately. Given these research limitations, in subsequent studies, we could further extend sample size and scale to cover as many bank samples as possible, adopt a multidimensional bank FinTech innovation index to upgrade the conclusions of similar research, and subdivide the bank risk-taking variables to further characterize the types of bank risks in order to provide banks with more valuable suggestions.
Funding StatementThe authors did not receive specific funding.
Data Availability StatementThe data used to support the findings of this study are included within the article.
Author ContributionsConceptualization, Chengming Li and Si He; Methodology, Lu Ning; Software, Chengming Li and Si He; Validation, Shiqi Sun, Yuan Tian; Formal Analysis, Chengming Li and Lu Ning; Investigation, Yuan Tian and Si He; Resources, Shiqi Sun; Data Curation, Chengming Li and Si He; Writing – Original Draft Preparation, Chengming Li and Si He; Writing – Review & Editing, Yuan Tian, Chengming Li and Lu Ning; Visualization, Yuan Tian; Supervision, Chengming Li and Lu Ning; Project Administration, Chengming Li and Lu Ning; Funding Acquisition, Chengming Li and Lu Ning.
CRediT authorship contribution statementChengming Li: Conceptualization, Software, Formal analysis, Data curation, Writing – original draft, Writing – review & editing, Supervision, Project administration, Funding acquisition. Si He: Conceptualization, Software, Investigation, Data curation, Writing – original draft. Yuan Tian: Validation, Investigation, Writing – review & editing, Visualization. Shiqi Sun: Validation, Resources. Lu Ning: Methodology, Formal analysis, Writing – review & editing, Supervision, Project administration, Funding acquisition.
In the figure, State-owned, Joint-stock, City, and Rural represent state-owned commercial banks, joint-stock commercial banks, city commercial banks, rural and village commercial banks, respectively; FTII is the banks’ FinTech innovation index. In general, TF-FTII is the technology foundation index of FinTech innovation, and TA-FTII is the technology application index of FinTech innovation.
Artificial intelligence includes intelligence, face recognition, real-time detection, fingerprint recognition; Blockchain-related includes blockchain, alliance chain, test chain, interconnection chain; Cloud technology includes loud computing, cloud architecture, cloud services, cloud finance; Data technology includes big data, data layer, data set, data flow; and Internet technology includes mobile, interconnection, network, online.
The common terms related to the development of a bank’s FinTech innovation can be divided into two dimensions: technology application and technology foundation. Technology applications includes digital currency, online banking, smart investment advisor, online payment, e-banking, smart risk control, cross-border payment, smart banking, regulatory sandbox, mobile payment, mobile banking, regulatory technology, third-party payment, smart customer service, compliance technology, mobile wallet, smart finance, and digital signature. The technology foundation includes online lending, big data, crowdfunding, cloud computing, online finance, artificial intelligence, credit scoring, blockchain, online financing, biometrics, online investment, Internet of Things, virtual reality, quantum computing, 5G, distributed architecture, and financial cloud.
Data has become the most important economic “new energy” in the new era, but the development of FinTech innovation in banks to promote digital transformation is not simply the digitization of data; they must use cutting-edge digital technology and hardware systems to promote the digitization of their data and production processes to achieve the goal of reducing costs, increasing efficiency, and improving quality. When banks develop FinTech innovation, they must rely on “digital science-technology driven” to transform and improve the digitalization of the original technology system and production system, and this change depends on the layout and development of key core technologies. Among them, A (artificial intelligence), B (blockchain), C (cloud computing), and D (big data) have comprised the core underlying technology architecture of banks’ FinTech innovation, and this level of FinTech innovation focuses more on the embedding of digital technologies to improve the technical support of banks’ internal production economy and management model. Secondly, the purpose of developing FinTech innovation in banks is to apply and integrate FinTech innovation into their business development and risk management; thus FinTech innovation at this level focuses more on the integration and innovation of digital science and technology, and complex business ecological scenarios, from the first level of technological innovation to the front-end market scenario application. Therefore, according to the structural differences, the development of a bank’s FinTech innovation can be divided into two levels, “technology foundation” and “technology application,” to begin this research.
The 65 commercial banks used in this paper are all listed banks, among which 26 are listed in A-shares, such as Ping An Bank, Huaxia Bank, and Bank of Ningbo. Bohai Bank, Guizhou Bank, and another 17 banks are listed in Hong Kong Stocks. There are 15 companies listed in both A-shares and Hong Kong shares, including Bank of China and Industrial and Commercial Bank of China. Kunshan Lucheng Village Bank, Meizhou Hakka Village Bank, and another 7 banks are listed in New Third Board. In addition, the above 65 commercial banks are divided into four categories: state-owned, joint-stock, city, rural and village commercial banks. Among them are six state-owned commercial banks, including Bank of China and Industrial and Commercial Bank of China. Joint-stock commercial banks include Ping An Bank, Huaxia Bank, and another 10 banks. There are 29 city commercial banks, such as Bank of Ningbo and Bank of Zhengzhou. There are 20 rural and village commercial banks, including Jiangsu Jiangyin Rural Commercial Bank and Jiangsu Zhangjiagang Rural Commercial Bank, among others.
In order to keep the sign of the regression results consistent with the baseline results, the paper treats the Z value as negative, i.e., the smaller the Z value, then the smaller the bank’s risk-taking.