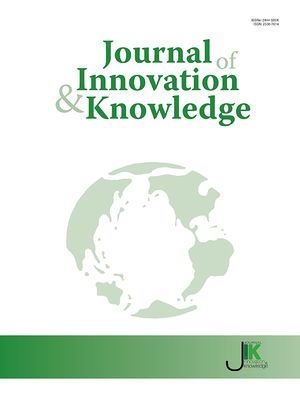
Edited by: Abbas Mardari
More infoThis study develops a conceptual framework based on the unified theory of acceptance and use of technology, the network theory perspective, and metaverse characteristics. Data were gathered through questionnaires from 209 Chinese manufacturers, and covariance-based structural equation modelling was the main approach used. The results indicate that performance expectancy, facilitating conditions, and establishing initial trust amongst supply chain partners can drive behavioural intention with respect to metaverse adoption for knowledge sharing to improve supply chain resilience. In addition, sensory feedback is an important characteristic of the metaverse and has a critical influence on adoption behaviour. Moreover, it has a complementary partial mediation effect on the relationship between establishing initial trust amongst supply chain partners and behavioural intention as well as a full mediating effect on the relationship between the initial trust of supply chain partners and behavioural expectations. Finally, sensory feedback moderates the relationship between behavioural intention and expectancy. Our findings contribute to the literature on metaverse adoption in the context of supply chain resilience.
The COVID-19 pandemic has had a huge impact on the global economy and human health (Modgil et al., 2021). The pandemic has also resulted in supply chain interruptions in different industries and across various countries (Yang et al., 2021). The lack of resilience in preventing, managing, and recovering from emergencies is a critical issue. Supply chain resilience is important for managing unexpected disruptions and emergency events and can even improve supply chain processes, enhance communication between supply chain partners, and help decision-makers make more precise decisions to mitigate unexpected risks (Martínez et al., 2020). However, McKinsey reported that only 21 % of supply chain organisations believed that their networks had a high level of resilience during the COVID-19 pandemic (Taghizadeh & Taghizadeh, 2021). Many companies face significant challenges in preserving resilience because of a lack of knowledge sharing (Lusiantoro & Pradiptyo, 2022).
According to Chen (2023), effective knowledge sharing between supply chain firms is crucial for achieving supply chain resilience, because high knowledge sharing can help firms develop similar innovation strategies to face emergencies (Sabahi & Parast, 2020). To improve knowledge sharing, greater communication with partners through information and communication technologies (ICTs) has become an important issue (Ozkanlisoy, 2021). However, individual enterprises are likely to share false knowledge throughout the communication process (Ada et al., 2021). Therefore, building a resilient supply chain is challenging.
The main reason that individual enterprises may share false knowledge is a lack of trust during the communication and sharing processes through ICTs. Knowledge-sharing through ICT is a form of computer-mediated communication (CMC). Although CMC overcomes the problem of communication across distances, poor sensory feedback (SF) remains a weakness (Scholl et al., 2020). SF is an important factor throughout the communication and knowledge-sharing process, and people feel trust when they receive greater SF while communicating (Schmidt et al., 2020). According to Freitas-Magalhães (2020), SF in communication usually occurs through facial expressions, body language, or changes in emotions. Therefore, when communicating and sharing knowledge through ICT, partners might not receive any SF, which would affect their level of trust (Nilsson & Mattes, 2015); this is why partners in the supply chain usually share false knowledge during the communication process (Kumar et al., 2021).
It is easier to develop trust and receive SF through face-to-face communication (FtFC) than through CMC (Handy, 1995), and FtFC increases interactions (Kröger et al., 2010). However, FtFC is difficult to implement in a global supply chain environment, especially during emergencies. Thus, as an ICT platform, the metaverse may facilitate FtFC. The metaverse provides an immersive three-dimensional (3D) virtual world in which people can perform social and economic activities through digital avatars based on the convergence of various state-of-the-art technologies (Lee et al., 2021). By creating a virtual world, the metaverse shortens the distance between supply chain partners and strengthens users’ sense of reality (Akhavan & Namvar, 2021). Partners can communicate with each other as if they were meeting in a real room (Huang et al., 2013), whereby it is arguably easier to receive SF from others (Chylinski et al., 2020). Therefore, participants in the metaverse increase their trust in each other because of their rich SF (Wallace, 2018). If applied to a supply chain's operational environment, knowledge sharing throughout the supply chain becomes more real, efficient, and transparent, further enhancing resilience (Akhavan & Namvar, 2021).
However, applying the metaverse to supply chains is still relatively new, especially for creating more resilient supply chains. Therefore, although the metaverse has a positive effect on knowledge sharing and resilience, not all partners have the intention to accept it for adoption. Thus, the supply chain leader should understand the factors that drive or affect their partners’ adoption intentions and acceptance use. Few empirical studies have been conducted on the metaverse and supply chain resilience. Furthermore, behaviour with respect to the metaverse and the motivations for metaverse technology adoption are mostly unexamined. To bridge this gap, this study focuses on answering the following questions. (1) What factors drive or affect metaverse adoption behaviour to improve knowledge sharing and enhance supply chain resilience? (2) Why do these factors drive or affect adoption behaviour?
Based on these research questions, we use the unified theory of acceptance and use of technology (UTAUT) as the main theoretical base to develop the conceptual framework. The UTAUT has been widely used to explain the adoption of different technologies; it employs an empirical investigation method to evaluate the introduction of new technology and predict user adoption and acceptance of the technology by incorporating external factors to better predict and explain individuals’ behavioural intent to use the new technology (Venkatesh et al., 2003; Khechine et al., 2016; Aslam et al., 2021; Skare & Soriano, 2021). The use of the UTAUT is in its infancy and is restricted to the initial implementation stages of a new information technology (Ali et al., 2016; Hadi et al., 2022). As the application of the metaverse to supply chains is still in its initial implementation stage, most organisations have yet to move beyond analysis to the adoption phase. The adoption of a new technology implies change, which invokes uncertainty that can hinder individual adoption. For this reason, as the literature on the UTAUT (Chao, 2019; Queiroz & Wamba, 2019; Venkatesh, 2022) notes, various scholars have dedicated significant efforts to understanding how individuals behave with regard to accepting a new technology, a necessary step in the process of companies acquiring the benefits of technology. Therefore, the UTAUT is a suitable method for understanding metaverse adoption in supply chain resilience. Related research can flexibly modify the UTAUT framework by adding specific constructs from other theories of ICT adoption (Queiroz & Wamba, 2019; Huang et al., 2021). Therefore, based on the characteristics of the metaverse and supply chain network relationships, we develop related constructs based on network theory and combine them with the constructs of the UTAUT to develop a conceptual framework.
Since the metaverse is a trending topic, few studies have explored its benefits in supply chain resilience (Trivedi & Negi, 2023; Queiroz et al., 2023). However, certain driving factors may affect supply chain partners’ adoption and use intention, and influence the degree to which companies can effectively benefit from related technology. Therefore, this study provides new knowledge for scholars and practitioners eager to gain an in-depth understanding of metaverse adoption behaviour to successfully implement the metaverse to enhance supply chain resilience.
The remainder of this paper proceeds as follows. Section 2 describes the metaverse model and its application in a supply chain context. Section 3 describes the theoretical background of the UTAUT and network theory. In Section 4, we develop the conceptual framework and hypotheses. In Section 5, we describe the methodology. Section 6 presents the results and Section 7 discusses them. Finally, Section 8 concludes with theoretical and managerial implications, limitations, and suggestions for future research.
The metaverse and its application in the supply chain contextThe metaverse foundation and technology frameworkThe metaverse first appeared in a speculative fiction novel called Snow Crash (1992). The term ‘metaverse’ is a combination of ‘meta’ and the stem ‘verse’, taken from ‘universe’, and describes a situation in which participants use digital avatars to represent their behaviour and interact with each other in a 3D virtual space (Jun, 2020). Initially, the metaverse depended on four technologies: (1) augmented reality (AR), (2) lifelogging, (3) mirror worlds, and (4) virtual reality (VR) (Bolger, 2021). AR allows virtual information to be connected to the real world when a corresponding AR device provides an enhanced view of the physical world (Bolger, 2021). Lifelogging can capture what is happening in people's lives via wearable devices and represents a situation in which everything that a person does is recorded and expanded. VR refers to a VR system that enables users to have an immersive experience (Jang et al., 2021). The face-to-face world is mapped onto a mirror world to create a digital twin of Earth. A mirror world can accurately model the real world (Bolger, 2021). The 3D mirror world can be continuously updated by object-aware sensors placed in public spaces and visible through VR headsets (Smart et al., 2007).
The development of multimedia technologies alone cannot overcome certain challenges related to the development of the metaverse. For example, these technologies cannot provide a transparent, stable, and sustainable digital economy because of the centralised and sophisticated digital environment (Duan et al., 2021). However, the rapid development of artificial intelligence (AI) and blockchain technologies has made it possible to solve these problems (Lee et al., 2021). AI is a prominent technology that enables machines to complete tasks in a manner similar to that of human beings, and allows communication between devices (Bolger, 2021). AI can manage and store data effectively, which helps eliminate difficult tasks. In the third era of the internet, the development of AI has involved an understanding of the physical world (Kelly, 2019). AI technology can create a digital twin of the Earth using data collected from user-created photos of all geophysical spaces in three dimensions (Bolger, 2021). A seamless connection between the physical and digital realms can be realised using AI (Lee et al., 2021). The metaverse integrates the physical and digital worlds (Smart et al., 2007, p. 1). Therefore, AI can realise these functions. Simultaneously, blockchain can be used to build a fair, decentralised, and sustainable interactive multimedia community within the metaverse (Duan et al., 2021). The core characteristic of blockchain is its decentralisation, such as the emergence of a distributed database in the cryptocurrency market (Min, 2019). Blockchain transactions are validated and stored by a distributed database in a peer-to-peer network (Chen, 2018), which is difficult to alter. The efficiency of the operation is enhanced by the knowledge shared over the blockchain Queiroz and Wamba (2019). Based on the above discussion, the metaverse technological framework has the following six characteristics: (1) AR, (2) life-logging, (3) mirror worlds, (4) VR, (5) AI, and (6) blockchain.
Applying the metaverse in the supply chainsSupply chains involve the physical movement of products from suppliers to customers and form an integrated and collaborative network of organisations (Jabbarzadeh et al., 2017). Knowledge sharing is particularly important for building an effective supply chain. Effective knowledge sharing leads to a high level of supply chain efficiency by allowing participants to better grasp the situation of all tiers in the supply chain Akhavan and Namvar (2021). Many ICTs have been applied in supply chain operational environments to improve knowledge sharing.
However, knowledge sharing through ICTs is a CMC with limited SF (Scholl et al., 2020). SF is an important factor in the knowledge-sharing and communication process. When partners try share related knowledge during the communication process with other partners, a lack of SF dampens trust (Nilsson & Mattes, 2015), and partners may share false knowledge to protect their own benefits (Ada et al., 2021). This undermines supply chain efficiency.
Providing a powerful immersion experience through SF is an important characteristic of the metaverse (Lee et al., 2021). SF, including rich non-verbal cues, which are key in FtFC, plays an important role in building trust in communication and knowledge sharing in virtual environments (Akhavan & Namvar, 2021). Consequently, in FtFC, supply chain partners trust others more, because lying behaviours are easily revealed through unconscious body language and facial expressions (Choi et al., 2022a). According to Gustafson-Pearce and Grant (2017) and Aseeri and Interrante (2021), rich SF provides realistic and immersive communication between partners in a supply chain network. Immersive communication promotes trust and knowledge sharing. Knowledge sharing amongst supply chain partners is becoming increasingly efficient, authentic, and transparent. This is especially the case for negotiations between purchasing managers and suppliers (Akhavan & Namvar, 2021), which are related to the sharing of private knowledge, such as supplier inventory levels and purchase prices. Prabhakaran et al. (2022) indicated that immersive technology can promote logistics planning and training in the construction supply chain to achieve better communication and collaboration. Therefore, the metaverse enables supply chain partners to make better decisions and act based on effective information and knowledge sharing, and collaboration amongst partners in a supply chain network (Sharma et al., 2022). Furthermore, the metaverse, which is a fusion of various ICTs, can impact supply chain trust and further promote knowledge sharing.
Many companies collaborate with technology service providers to implement metaverse strategies at different stages of the supply chain. For example, BMW has partnered with NVIDIA Omniverse for virtual electric vehicle production in plants worldwide. In the omniverse, BMW members can run a variety of real-time digital twin simulations, virtual optimised layouts, and logistics systems in a 3D virtual environment. New solutions can be tested and validated using digital twins across thousands of scenarios and edge cases, eliminating the need for physical prototypes, which minimises critical downtime and increases operational efficiency. At the same time, the omniverse allows BMW's production staff and suppliers to access the metaverse through VR, AR, and other smart devices and uses real-time data to plan and optimise processes to strengthen knowledge sharing and collaboration amongst partners.
Efficiency of the metaverse in supply chain resilienceSupply chain resilience is an important issue in supply chain research (Novak et al., 2021). Modgil et al. (2021) and Novak et al. (2021) defined supply chain resilience as a supply chain's ability to resist interruptions and recover quickly after an interruption. This ability allows the supply chain to return to its original state or move to a new and more desirable state after a disturbance. Specifically, supply chain resilience is an important ability that helps partners in the supply chain deal with emergency events, disasters, and unexpected disruptions, such as the COVID-19 pandemic. However, Ivanov (2021) indicated that high knowledge sharing is necessary to enhance supply chain resilience, because it is a crucial capability for coping with massive disruptions. Resilience can help supply chain partners enhance existing redundancy and flexibility resilience capabilities, such as flexibility inventory, supplier selection, logistics strategy, and capacity planning; recover from damage from disruptions; and maintain the supply chain's operational efficiency.
Gu et al. (2021) emphasised the application of ICTs to promote knowledge sharing and build supply chain resilience. Online communication and knowledge-sharing environments can be built amongst supply chain partners to promote knowledge sharing. However, traditional ICTs cannot provide SF owing to CMC. As mentioned previously, poor SF regarding CMCs has been reported (Scholl et al., 2020). Specifically, any ICT creates an ‘online environment’, and partners usually use computers to communicate or share knowledge with others. However, the online environment tends to erase spatial proximity (Pu et al., 2021), and partners may find it difficult to fully trust other partners because of obstacles to observing and receiving SF via CMCs, such as emotional, facial, and even body language expressions. Specifically, people must still depend on FtFC to observe other people's emotional, facial, and body language expressions to obtain real SF; otherwise, it is difficult to build trust (Damen et al., 2020). Although related innovative ICTs can use videoconferencing platforms to simulate a situation closer to that of FtFC (Basch et al., 2021), providing SF remains a challenge. Low SF is an obstacle to partners fully obtaining their partners’ trust. This situation affects not only trust between partners but also knowledge-sharing and communication intentions. Consequently, this situation constrains knowledge-sharing, leading to poor resilience.
A few studies, such as those by Ivanov (2021) and Choi et al. (2022b), have also suggested that metaverse may be better than other ICTs at enhancing supply chain resilience. According to Gupta et al. (2021), Wang et al. (2022), and Yu et al. (2022), the metaverse can create a virtual world using AR/VR/AI, which enhances SF. Lee et al. (2021) and Bian et al. (2021) indicated that when SF was enhanced, participants in the metaverse environment increased their trust and became willing to share related knowledge with other partners. Based on these factors, the metaverse can overcome challenges with existing ICTs and is better suited for enhancing supply chain resilience.
As a metaverse technology platform, NVIDIA Omniverse creates 3D virtual collaboration environments that allow the construction of physically accurate digital twins to strengthen supply chain visibility. In addition, through AI, omniverse users can quickly create vivid 3D avatars with facial features and body movements and access the metaverse through smart devices, such as VR headsets and AR glasses. The metaverse technology platform provided by NVIDIA has been used by BMW and Siemens. With real-time supply chain data provided by the digital twin and immersive SF with a realistic digital avatar for communication, supply chain members around the globe can collaborate in real time across geographies and time zones, communicating as if they were face-to-face rather than sharing knowledge through less immersive mediums. Additionally, engineering, manufacturing, and logistics teams can be immersed in the metaverse platform. This collaborative approach helps companies facilitate supply chain visibility, respond, and recover quickly when supply chain problems occur with higher supply chain resilience.
Theoretical backgroundUnified theory of acceptance and use of technologyTo answer the research questions, this study adopts the UTAUT as a basis for developing a conceptual framework. The UTAUT model extends the technology acceptance model (TAM) (Venkatesh et al., 2003). Rozmi et al. (2019) indicated that the UTAUT explains the factors influencing ICT acceptance intention. The UTAUT framework is a product of integrating elements across eight models obtained from the literature. The eight models include the theory of reasoned action, social cognitive theory, TAM, theory of planned behaviour (TPB), model of PC utilisation, innovation diffusion theory, motivational model, and a combination of TAM and TPB.
In the UTAUT model, performance expectancy (PE), effort expectancy (EE), social influence (SI), and facilitating conditions (FCs) are modelled as exogenous constructs to understand user adoption intentions for new ICTs. In addition, behavioural intention (BI) was used as a measurement construct to measure adoption intention. Verifying the relationships amongst PE, EE, SI, FCs, and BI can explain which factors drive or affect adoption intention (Venkatesh et al., 2003). In addition, use behaviour is the final construct that measures whether usage behaviour occurs when the user has an adoption intention. Venkatesh et al. (2008) and Maruping et al. (2017) proposed that the integration of behavioural expectations (BE) into the UTAUT theory enables the theory to be robust in terms of explaining the internal and external determinants of technology use. They considered that BE is a better predictor of new ICT use. Therefore, BE is included in the UTAUT model. Moreover, the UTAUT model attempts to explain how individual differences influence technology use through certain mediators and moderators, such as age, sex, and experience. The original UTAUT has been applied in different studies and allows for some modifications by adding specific constructs or moderators for the adoption of ICTs (Queiroz & Wamba, 2019), such as trust, risk, and security (Dwivedi et al., 2017). Mediators and moderators also allow for modifications (Queiroz & Wamba, 2019). We consider the UTAUT model to be an appropriate model for our study's purpose owing to its superior fit.
Existing studies have widely explored new information technology adoption behaviours using the UTAUT model. When considering the different usage environments and users of new ICTs, research can reveal different adoption behaviours and provide different suggestions. For example, blockchain technology has become the most recent technology. Queiroz and Wamba (2019) explored blockchain adoption behaviour in India and the US. finding that different adoption intentions exist. For example, SI affects adoption intention in India but not in the US. Therefore, different strategies should be designed to implement blockchain. Thus, the analysis based on the UTAUT model does not provide absolute results. The UTAUT has been used to study the adoption and use of AI, which is a key technical component of the metaverse. Venkatesh (2022) proposed a research agenda for the adoption and use of AI tools from an individual perspective based on the UTAUT model, including the determinants of PE, EE, SI, and FC. Andrews et al. (2021) and Lin et al. (2022) empirically analysed the intention of different cultural backgrounds in North America and China to adopt AI and related technologies. PE is an important factor influencing the adoption and use of new technologies in most studies, whereas other determinants have different impacts on the adoption of AI in other contexts.
Network theory perspectiveBy using the UTAUT model to develop the conceptual framework, we can better understand the adoption behaviour of supply chain partners regarding the metaverse for supply chain resilience. Network theory can also contribute to a better understanding of which related networks and how the related network's relationship factors affect adoption behaviour (Queiroz & Wamba, 2019). This study explores the adoption behaviour of the metaverse in the context of supply chain resilience. The supply chain is a network operation (Ye, 2019); therefore, some factors may exist and affect adoption behaviour that can be explained through network theory. These factors should be combined with the UTAUT model to develop a conceptual framework.
Network theory, specifically social network theory, is defined as a specific set of linkages amongst a defined set of persons that allows the social behaviour of the persons involved to be interpreted (Mitchell, 1969). Social network theory focuses on the interpretation of social behaviour. Existing studies, such as Rahmana et al. (2015) and Yin and Ran (2021), indicate that the interaction behaviours of supply chain partners in the supply chain network environment may produce factors that affect new ICT adoption intentions. In this study, initial trust amongst supply chain partners (TR) was deemed an important driving factor. According to the network theory, to make or practice any decision in the supply chain operational environment, it is necessary to establish a consensus between supply chain partners (Treiblmaier, 2018). Therefore, before adopting any new ICT, the supply chain partners should reach a consensus. However, establishing a consensus on the adoption of new ICT relies on initial trust (Kiwala et al., 2022). Initial trust is defined as ‘a need of a given individual to meet each other's requirements with no prior experience or accurate and relevant information and knowledge’ (Almajali, 2021). Therefore, when supply chain partners decide whether to adopt new ICTs, the perceived risk of cooperation due to a lack of prior cooperation experience with new ICTs is possible (Zhang et al., 2019). Lu et al. (2005) defined perceived risk as ‘the degree to which a user feels uncertainty and adverse consequences’. Perceived risk decreases adoption intention and the degree of usage acceptance (Yuen et al., 2022). However, if ICT is important and efficiency is needed, the supply chain partner must bear the perceived risk. Therefore, supply chain partners must establish initial trust. Van der Sijde et al. (2015) explained that establishing initial trust is necessary when implementing new ICTs.
However, today's ICTs, such as blockchain and AI, create an environment without trust (Xu et al., 2019). Without trust, a supply chain partner does not have to trust specific participants in a network-collaboration environment (Koh et al., 2020). This system could help participants reduce their perceived risks and protect their benefits. This eliminated the need for initial trust and consensus. For example, Balaji et al. (2018) found that initial trust had no substantial effect on driving or the adoption behaviour of IoT, the Internet of Things. Blockchain and AI are the two main technologies in the metaverse; they provide related functions to build an environment without trust and protect the benefits of participants. Therefore, initial trust, regardless of whether it is necessary, driving, or affecting the adoption behaviour of the metaverse, becomes an important construct and is combined with the UTAUT model to develop the conceptual framework.
A few studies, such as Chiu et al. (2017), claimed that if new ICT certainly brought greater efficiency to enhance network relationships and reduced perceived risk, it might raise trust and enhance adoption behaviour. A few studies, such as Oliveira et al. (2014), have suggested that the characteristics of new ICTs can be used as mediators and moderators to verify the relationship change between initial trust and adoption behaviour. Specifically, the analysis results prove whether the characteristics of new ICT have a significant influence on promoting adoption behaviour.
Theoretical framework and hypothesesDrawing on the theoretical background, we develop the conceptual framework shown in Fig. 1. The framework first adopts related constructs from the UTAUT, including PE, SI, FCs, BI, and BE. BI measures the adoption intention of the metaverse. In addition, we adopt BE instead of usage behaviour. Although our survey respondents had some experience, the integration of several advanced and new technologies in the metaverse for the supply chain is still at its initial implementation stage and has not yet gained a significant degree of awareness; therefore, we exclude the original construct ‘usage behaviour’ of the UTAUT. As an important determinant of IT adoption and use, BE is formed by BI and is considered the most suitable predictor of IT use intention (Venkatesh et al., 2008; Maruping et al., 2017). Hence, BE is more suitable than usage behaviour for our research questions. In addition, PE, SI, and FCs are the driving factors that affect adoption behaviour. However, EE is not included in our framework. Recently, scholars have shown that EE is not statistically significant in explaining the BI of the technical components of the metaverse, such as blockchain Wong et al. (2020), AI (Andrews et al., 2021), and AR (Pinto et al., 2022). In the supply chain field, researchers EE to be a poor predictor of blockchain adoption (Queiroz & Wamba, 2019; Wong et al., 2020); therefore, EE is dropped in this study. Second, according to the related literature on network theory, TR becomes a new construct for measuring whether it can drive and affect the adoption behaviour of the metaverse. Considering that the SF of the metaverse is a critical characteristic that can promote trust in communication and knowledge sharing, further enhancing resilience, its efficacy may change the relationships amongst TR, BI, and BE. Based on this, SF becomes both a mediator and a moderator in the conceptual framework. In addition, by verifying the mediating and moderating effects, we can determine whether SF has a critical influence on driving adoption behaviour. Thus, we propose the hypotheses discussed in the following subsections.
Performance expectancyPE is defined as ‘the degree to which an individual believes that using the system will help him or her attain gains in job performance’ (Venkatesh et al., 2003). Individuals’ perceived advantages can motivate them to accept and use new technology in their work routines (Venkatesh et al., 2003). PE has been justified as a predictor of individuals’ intentions to adopt a technology (Venkatesh et al., 2012; Alalwan et al., 2017; Hensel et al., 2021). In our study context, PE is conceptualised as the degree to which supply chain professionals believe that adopting the metaverse can improve their communication efficiency with respect to resilience. Some studies consider that the metaverse can help supply chain-related companies improve their communication productivity and enhance supply chain resilience (Min, 2019; Modgil et al., 2021). Therefore, it is possible that this belief influences adoption behaviour. Therefore, we propose the following hypothesis.
H1 Performance expectancy positively affects behavioural intention to adopt the metaverse.
SI is defined as ‘the degree to which an individual perceives that it is important other believe he or she should use the new system’ (Venkatesh et al., 2003). Because the supply chain is a network environment, prior studies have confirmed the validity of SI in each supply chain partner's intention to use new technologies (Venkatesh & Brown, 2001; Brown et al., 2010). In this study, SI indicates that when different supply chain partners understand the value of the metaverse, other partners are also affected, thereby increasing their adoption intention. Some studies, such as Lee and Kim (2022), considered that when some users understand the value of the metaverse, others may also follow suit. Based on the existing studies, we propose the following hypothesis.
H2 Social influence positively affects behavioural intention to adopt the metaverse.
FCs are defined as ‘the degree to which an individual believes that an organisational and technical infrastructure exists to support the use of the system’ (Venkatesh et al., 2003). In this study, FCs are related to how organisational and technical infrastructures can be constructed to support the use of the metaverse. FCs, such as internet speed and IT resources, can influence organisational behaviour towards technology adoption and use (Queiroz & Wamba, 2019). As the infrastructure that constitutes digital technologies (VR, AR devices, blockchain, and the internet) continues to improve and mature in companies (Lee et al., 2021), more technology resources should become available to support the use of the metaverse (Lee & Kim, 2022). Therefore, FCs can drive or affect adoption behaviour. Thus, we propose the following hypothesis.
H3 Facilitating conditions positively affect behavioural intention to adopt the metaverse.
Initial trust can be defined as ‘willingness to rely on a third party after a first interaction with that party and may be the factor that determines the extent to which future interactions occur’ (Hampton-Sosa & Koufaris, 2005; Hsu & Tang, 2020). In our study, TR means that they have no experience cooperating in jointly adopting and using new ICTs. However, ICTs offer greater efficiency in promoting supply chain resilience. Therefore, supply chain partners must bear the perceived risks of adoption. Establishing initial trust is necessary to reach a consensus on adopting new technologies; otherwise, metaverse adoption will be adversely affected. When TR is established, it is possible to adopt the metaverse. Initial trust must usually be greater than perceived risk, because not every supply chain partner can expect effective cooperation when deciding to jointly adopt new ICTs, which can hinder metaverse adoption. However, if partners are willing to bear the perceived risk and establish initial trust, then their adoption intention will increase. Therefore, existing studies, such as Van der Sijde et al. (2015), indicate that a relationship exists between TR and BI. Based on the above discussion, the following hypothesis is proposed.
H4 Initial trust amongst supply chain partners positively affects behavioural intention to adopt the metaverse.
BI is defined as ‘the degree to which a person has formulated conscious plans regarding whether to perform a specified future behaviour’ (Maruping et al., 2017). In our study, BI refers to partners’ intention to engage in a behaviour leading toward metaverse adoption. Many studies report that BI directly influences technology adoption (Venkatesh et al., 2003; Chao, 2019). Behavioural expectation is the subjective probability of performing a behaviour based on an individual's cognitive appraisal of volitional or non-volitional determinants. (Maruping et al., 2017). In this study, BE refers to supply chain professionals’ evaluation of the probability of engaging in a particular behaviour associated with the use of metaverse technology. Previous studies have shown that high intention promotes use behaviour (Maruping et al., 2017; Queiroz & Wamba, 2019). According to Venkatesh (2008, p. 486), individuals first form volitional determinations to perform behaviour-BI, and then individual perceptions gradually incorporate non-volitional factors that may hinder or facilitate the successful execution of this behaviour, which is the formation of BE. Their relationship reflects the timeline of events that lead to behaviour (Maruping et al., 2017). Based on the above discussion, we develop the following hypothesis.
H5 Behavioural intention positively affects behavioural expectation with respect to metaverse adoption.
SF is defined as a subjective experience in which an individual experiences the sensory information of communication partners in a virtual environment using VR, AR, and other devices (Rice, 1992; Sharan et al., 2021). Supply chain members can enter the 3D virtual world of a metaverse person through VR headsets, AR glasses, or other smart devices (Queiroz et al., 2023). These devices help members receive and experience SF through 3D virtual representations. This enables supply chain members to receive and experience the facial expressions, voices, body language, and emotional changes of others. This experience can create a great sense of presence, such as staying with others in the physical world (Buhalis et al., 2022). As mentioned in the related literature, new ICT that provides greater efficiency in enhancing network relationships and reducing perceived risk may raise initial trust intention and enhance adoption behaviour (Oliveira et al., 2014; Chiu et al., 2017). SF is an important characteristic of the metaverse that has a positive effect on raising partners’ trust during communication and knowledge-sharing processes and enhancing the resilience of the supply chain. Specifically, initial trust is the basis of members’ subsequent virtual collaboration. The metaverse provides new conditions for members to understand behaviours and attitudes to raise trust through SF (Greenberg et al., 2007; Laukkanen et al., 2022). According to Alnoor et al. (2022), virtual interactive media effectively reduces perceived risk and strengthens participants’ sense of presence, thereby positively affecting their IT use intention. Thus, the presence of SF reduces the perceived risk of TR in virtual environments. In our study context, supply chain partners’ understanding of the efficiency of the SF of the metaverse in improving trust through communication and knowledge sharing has a positive effect on resilience. Therefore, it is possible to reduce perceived risk, improve the intention to strengthen TR, facilitate the formation of BI, and consciously estimate the probability of using a metaverse, referred to as BE. Thus, SF may have a mediating effect on the relationship between TR, BI, and BE. Therefore, we propose the following hypotheses.
H6a Sensory feedback has a mediating effect on the relationship between initial trust in supply chain partners and behavioural intention with respect to metaverse adoption.
H6b Sensory feedback has a mediating effect on the relationship between initial trust in supply chain partners and behavioural expectations with respect to metaverse adoption.
This study maintains that accurate SF from metaverse experiences versus face-to-face interactions can improve resilience amongst supply chain partners. These conditions lead supply chain partners to engage in behaviours that increase the adoption intention of this technology. As mentioned above, supply chain members can enter the metaverse through different smart devices, such as VR headsets, AR glasses, smartphones, and computers. These devices provide users with different experiences based on their level of SF when accessing the metaverse. A high level of SF improves the quality of collaborative interactions between members (Nam et al., 2008; Chung et al., 2015), and users can be immersed in a high-quality visual and audible world similar to FtFC in the real world. This can lead to efficient knowledge and information sharing and further improve resilience (Scholten & Schilder, 2015). Thus, with high-quality and accurate SF, it is possible to increase the frequency of the targeted behaviour with respect to meta-verse usage. Conversely, if high-quality SF cannot be achieved and maintained in the metaverse, the actual usage behaviour can decrease despite a high adoption intention (Gao et al., 2021). This is referred to as BE. Related studies, such as Sim et al. (2021), remind us that BE decreases when the efficiency of new ICTs cannot be continuously maintained. Therefore, SF may moderate the relationship between BI and BE. Thus, we propose the following hypothesis.
H7 Sensory feedback has a moderating effect on the relationship between behavioural intention and behavioural expectations regarding metaverse adoption.
In this section, we first describe how we designed the questionnaire to collect related empirical data for empirical analysis. In addition, we describe the sample characteristics and explain how we distributed the questionnaire to collect data. Next, we explain how we measure these data to introduce the related measurement approaches used in this study. Third, we introduce the analytical method and explain why we chose it. Finally, we analyse the robustness of the results.
Questionnaire constructs, sample design, data collection, and sample profilesSeven constructs were included in the questionnaire according to the conceptual framework and relevant studies were cited as design variables for each construct. The first construct is PE, for which we referred to the research questionnaire by Wong et al. (2020) to revise and design four variables for the construct. The second and third constructs are SI and FCs, each of which involves four variables designed according to Lee and Kim's (2022) research questionnaire. TR is the fourth construct, which has four variables designed with reference to questionnaires from Queiroz and Wamba (2019). BI and BE are the fifth and sixth constructs, respectively, each of which has three variables, following Maruping et al. (2017), Queiroz and Wamba (2019), and Lee and Kim (2022). The seventh construct is SF, which has four variables, based on Kreijns et al. (2021), Sharan et al. (2021), and Lee and Kim (2022). These variables are included in the questionnaire items. All items from each construct were measured on a 7-point Likert scale. When completing the questionnaire development, a survey pre-test was conducted by 31 scholars with an information technology background to ensure that the questionnaire was valid. We examined inner consistency using Cronbach's alpha, which should exceed 0.83 for inner consistency (Chang et al., 2011). According to the pre-test results, the Cronbach's alpha values of the seven constructs are higher than 0.83, satisfying the requirement of the pre-test. The questionnaire is presented in the Appendix.
With the Ministry of Industry and Information Technology of China announcing its digital development policy on the metaverse, many provinces in China, including Beijing, Jiangsu, Zhejiang, Hainan, and the Guangdong-Hong Kong-Macao Greater Bay Area (GBA), actively supported related manufacturers to improve metaverse technology application in the manufacturing supply chain operational environment. This study attempted to connect with related government units and obtained a list of 544 manufacturers that had improved or planned a metaverse implementation to strengthen resilience and encourage others to participate. Our questionnaire was distributed to 544 Chinese manufacturers via the largest survey platform (https://wjx.cn) from 15 December 2021 to 15 January 2022. During this period, manufacturers decided to improve or plan to implement new information technology. During this period, some manufacturers designed pilot production environments to evaluate the efficiency of the new technology. This is a regular company process that avoids high-cost production and reduces the waste of resources. When these manufacturers improved or planned a metaverse implementation, they usually gained experience in pilot test production environments and obtained an understanding of the use efficiency of the metaverse in supply chain operations. Subsequently, 209 manufacturers responded, with a response rate of 38.42 %. The profiles of the 209 manufacturers are listed in Table 1.
Sample profiles.
China has become the world's largest manufacturing base (Ma et al., 2013). Since the COVID-19 pandemic began in 2019, the Chinese government adopted a serious lockdown policy since the COVID-19 pandemic began in 2019. Until now, the lockdown policy has remained in effect, including in Shanghai, beginning March 2022. This situation has led to serious supply chain disruptions over the past three years, and the damage in China is far greater than that experienced by manufacturers in other countries. Chinese manufacturers have continued to improve and strengthen their supply chain resilience over the past decade; however, they still find it difficult to address supply chain disruptions and quickly recover from challenging situations. Therefore, Chinese manufacturers have recognised the need to overcome their weaknesses in terms of resilience. As demonstrated by the efficiency of metaverse in supply chain resilience, an increasing number of manufacturers have begun to cooperate with ICT firms to develop metaverse technology and implement it in the supply chain to enhance resilience. For example, the official policy on the metaverse in the GBA in China has promoted industrialisation and large-scale application of the metaverse in the manufacturing industry and has created new business and supply chain models. This increase in GBA manufacturers has improved the implementation of the metaverse in their supply chains. Therefore, although we collected data only from Chinese manufacturers, the analysis results provide valuable suggestions for global manufacturers.
After data collection, common method bias (CMB) and non-response bias analyses should be tested. CMB may arise when sample data are collected from a single source or common measurement environment. Therefore, we calculated and tested the CMB to ensure that the measurement model was not affected. According to Podsakoff and Organ (1986), the relationship between exogenous and endogenous variables can be inflated by CMB. A full collinearity assessment approach has been suggested for the CMB analysis (Kock, 2015). The inner variance inflation factor (VIF) values were generated from the analysis results. According to Kock (2015), whole values should be lower than 3.3 if the model is unaffected by CMB. Regarding the non-response bias analysis, our response rate to the questionnaire was 38.42 %; therefore, we should consider non-response bias, which could affect the findings of the research. To analyse non-response bias, the time-trend extrapolation method was used according to suggestions from Armstrong and Overton (1977) to compare the top 25 % and bottom 25 % respondents, and an independent sample t-test was conducted. The work experience and job position of the respondents were adopted as the two variables to test for non-response bias. SPSS (version 23.0) was used for the analysis.
Table 2 shows the analysis results of the CMB; the VIF values are lower than 3.3 and satisfy the requirements noted by Kock (2015). The analysis results of the non-response bias show that the F value of work experience is 3.317 (t-value is 3.484, p-value > 0.05), and the F value of the job position of the respondents is 0.357 (t-value is −3.843, p-value > 0.05). Therefore, there is no difference between the two groups, and there is insufficient evidence to suggest that non-response bias exists.
Analysis results regarding CMB.
Subsequently, the correlation matrix, construct reliability, validity, and discriminant validity were tested. The purpose of a correlation matrix is to develop initial insights into the relationships that may exist amongst the variables (Afzal et al., 2013). According to Udovičić et al. (2007), calculating the correlation matrix involves Pearson's coefficient and the importance of the coefficient is expressed by the p-value. Four approaches–Cronbach's alpha, factor loading, composite reliability (CR), and average variance extracted (AVE)–were used to assess the construct reliability and validity. Hair et al. (2021) indicated that Cronbach's alpha should be higher than 0.7, and the factor loading should exceed 0.5. With respect to CR and AVE, Hair et al. (2021) also suggested that these values should exceed 0.7 and 0.5, respectively. Additionally, it is necessary to ensure the quality of the measurement results of the model and the discriminant validity analysis. The Fornell–Larcker criterion and cross-loadings were adopted to measure discriminant validity.
Methods for structural modelling and control variablesThis study adopted covariance-based structural equation modelling (CB-SEM) as an analytical method for testing and verifying the conceptual framework and hypotheses. According to Sadikaj et al. (2021), CB-SEM is a better method for empirically estimating models with latent variables and verifying mediation and moderation effects. Partial least squares structural equation modelling is also a popular method; however, we did not adopt it. According to Hair et al. (2011), CB-SEM should be adopted when a study adopts theory as a basis to develop a model. Because this study adopts UTAUT and network theory as bases to develop a conceptual framework, we adopted CB-SEM as the main method. For CB-SEM analysis, we used AMOS 23.0 as our analysis tool. However, during the analysis process, control variables should be added to avoid potential confounding impacts. Firm size and process positioning in the supply chain are the two main control variables. Firm size was assessed using the natural log of the number of employees. Firm size was used to assess a firm's process positioning.
Robustness analysisIn this study, we assumed that four factors – PE, SI, FCs, and TR – drive and affect BI to BE. In addition, we assumed that SF, as a driver, may mediate the relationship between TR, BI, and BE. Through CB-SEM analysis, we can understand the variables that drive the intention to adopt the metaverse. However, to ensure that the analysis results are stable and continue to provide strong evidence for the research aim, we need to verify the robustness of the results regarding the relationships amongst PE, SI, FCs, TR, SF, BI, and BE, including the mediating effect of SF on the relationship between TR, BI, and BE. According to Hocine et al. (2016) and Hao et al. (2019), culling variables and random screening of the sample are two methods for robustness analysis. In culling the variables, we separately removed PE, SI, FCs, TR, and SF, and proposed research hypotheses for different models corresponding to the path coefficients. Following Hao et al. (2019), we randomly screened 80 % of the sample and verified the research hypotheses. The results confirmed the stability and robustness of the CB-SEM analysis results.
Analysis resultsAnalysis results of the measurementTable 3 shows the analysis results of the correlation matrix. Substantial correlations exist between the variables. Table 4 presents the measurement results for construct reliability and validity. All Cronbach's alpha values are higher than 0.7, the CR and AVE values of each construct are higher than 0.7 and 0.5, respectively, and the factor loading of each construct is higher than 0.5. Thus, construct reliability and validity satisfy the requirements of Hair et al. (2021). Regarding the discriminant validity results, Table 5 shows that the AVE square root for the construct is greater than the correlations between that construct and all other constructs. According to Fornell and Lacker (1981), these results confirm discriminant validity. In addition, Table 6 shows the results of the cross-loadings and confirms discriminant validity.
Correlation matrixes.
Mean | S.D. | PE | SI | FCs | TR | BI | BE | |
---|---|---|---|---|---|---|---|---|
PE | 3.876 | 0.776 | ||||||
SI | 3.749 | 0.794 | 0.759⁎⁎ | |||||
FCs | 3.689 | 0.890 | 0.612⁎⁎ | 0.690⁎⁎ | ||||
TR | 3.855 | 0.777 | 0.637⁎⁎ | 0.651⁎⁎ | 0.674⁎⁎ | |||
BI | 3.880 | 0.801 | 0.622⁎⁎ | 0.580⁎⁎ | 0.677⁎⁎ | 0.692⁎⁎ | ||
BE | 3.976 | 0.749 | 0.655⁎⁎ | 0.612⁎⁎ | 0.632⁎⁎ | 0.639⁎⁎ | 0.736⁎⁎ | |
SF | 3.872 | 0.727 | 0.617⁎⁎ | 0.605⁎⁎ | 0.584⁎⁎ | 0.675⁎⁎ | 0.629⁎⁎ | 0.684⁎⁎ |
* p-value ≦ 0.05.
Measurement of reliability and validity.
Analysis results regarding cross-loadings.
Table 7 shows the full regression analysis results of the structural model. The analysis results can further verify the effect of the control variables and the results of the research hypotheses. We present all results for the hypotheses regarding β, t, and p values. Model 1 verifies the effect of the control variables on BI and BE. Concerning the relationship between firm size, the process positioning of the firm and BI, β = 0.131 and 0.039, t = −1.675 and −0.500, and the p values are all higher than 0.05; thus, these two control variables cannot affect BI. Concerning the relationship between firm size, process positioning of the firm, and BE, β = 0.065 and 0.058, t = −0.815 and −0.737, and p value are all higher than 0.05; thus, these two control variables cannot affect BE.
Full regression analysis results of the structural model.
Variables | BI | BE | |||||
---|---|---|---|---|---|---|---|
Model 1 | Model 2 | Model 3 | Model 1 | Model 2 | Model 3 | Model 4 | |
Control Variables | |||||||
Firm size | 0.131 (t = −1.675) | −0.016 (t = −0.237) | −0.021 (t = −0.304) | 0.065 (t = −0.815) | −0.072 (t = −1.000) | −0.091 (t = −1.288) | 0.003 (t = 0.323) |
Process positioning of firm | 0.039 (t = −0.500) | 0.049 (t = 0.742) | 0.050 (t = 0.734) | 0.058 (t = −0.737) | 0.023 (t = 0.315) | 0.026 (t = 0.379) | 0.009 (t = 0.806) |
Independent Variables | |||||||
PE | 0.484⁎⁎ (t = 4.132) | 0.431⁎⁎ (t = 3.438) | |||||
SI | −0.045 (t = −0.408) | −0.099 (t = −0.828) | |||||
FCs | 0.510⁎⁎ (t = 4.709) | 0.526⁎⁎ (t = 4.490) | |||||
TR | 0.482⁎⁎ (t = 4.487) | 0.376⁎⁎ (t = 3.209) | |||||
BI | 0.956⁎⁎ (t = 9.149) | 0.786⁎⁎ (t = 6.739) | |||||
Mediator | |||||||
SF | 0.309⁎⁎ (t = 2.688) | 0.382⁎⁎ (t = 2.789) | |||||
Moderator | |||||||
SF | −0.437⁎⁎ (t = −7.518) | ||||||
BI x SF | 0.827⁎⁎ (t = 7.861) | ||||||
Point estimate | 0.105⁎⁎ | ||||||
Condifence Intervals | (0.070, 0.147)⁎⁎ |
*p-value ≦ 0.05.
Regarding Model 2, in addition to considering the effects of the control variables, we add independent variables based on the research hypotheses and verify H1, H2, H3, H4, and H5. For H1, β = 0.484, t = 4.132, and p < 0.01; therefore, PE has a positive effect on BI, and H1 is supported. For H2, β = −0.045, t = −0.408, and p > 0.05; thus, SI has no considerable effect on BI. For H3, concerning the relationship between FCs and BI, β = 0.510, t = 4.709, and p < 0.01; thus, H3 is supported. For H4, β = 0.482, t = 4.487, and p < 0.01; thus, TR has a positive effect on BI. For H5, β = 0.956, t = 9.149, and p < 0.01; thus, BI is proven to have a positive effect on BE. In Model 2, the relationships between the two controls and BI, β = −0.016 and 0.049, t = −0.237 and 0.742, and the p values are all higher than 0.05; in addition, the relationships between the two controls and BE, β = −0.072 and 0.023, t = −1.000 and 0.315, and the p values are all higher than 0.05. Thus, the analysis results cannot be affected by control variables.
Regarding Model 3, we added the mediating effect of SF based on Model 2. H6a verifies the relationships between TR, SF, and BI. The relationship between SF and BI is β = 0.309, t = 2.688, and p < 0.01. Combined with the bootstrapped mediation results of Table 8, we find β = 0.288, t = 2.821, and p < 0.01 for the direct effect and β = 0.119, t = 2.082, and p < 0.05 for the indirect effect. It shows complementary partial mediation; thus, H6a is supported. H6b is to verify the relationships between TR, SF, and BE. The relationship between SF and BE is β = 0.382, t = 2.789, and p < 0.01. According to Table 8, β = 0.095, t = 1.076, and p > 0.05 of the direct effect; β = 0.223, t = 3.804, and p < 0.01 of the indirect effect. This shows full mediation; thus, H6b is supported. When checking other hypotheses, for H1, β = 0.431, t = 3.438, and p < 0.01; for H2, β = −0.099, t = −0.828, and p > 0.05; for H3, β = 0.526, t = 4.490, and p < 0.01; for H4, β = 0.376, t = 3.209, and p < 0.01; and for H5, β = 0.786, t = 6.739, and p < 0.01. Thus, the above hypotheses are still supported. Regarding the control variables, the relationships between the two controls and BI are β = −0.021 and 0.050, t = −0.304 and 0.734, and the p values are all higher than 0.05; in addition, the relationships between the two controls and BE are β = −0.091 and 0.026, t = −1.288 and 0.379, and the p values are all higher than 0.05. Thus, the analysis results cannot be affected by control variables.
Bootstrapped mediation results.
Total effects | Direct effects | Indirect effects | Indirect effect - Condifence Intervals | |||||||
---|---|---|---|---|---|---|---|---|---|---|
Model | β | t-value | β | t-value | β | t-value | Point estimate | Lower | Upper | Results |
TR→SF→BI | 0.407⁎⁎ | 3.580 | 0.288⁎⁎ | 2.821 | 0.119⁎⁎ | 2.082 | 0.119 | 0.007 | 0.231 | Complementary partial mediation |
TR→SF→BE | 0.507⁎⁎ | 5.501 | 0.095⁎⁎ | 1.076 | 0.223⁎⁎ | 3.804 | 0.223 | 0.122 | 0.351 | Full mediatoin |
*p-value ≦ 0.05.
Model 4 is used to verify the moderating effect. H7 attempts to test the moderating effect of SF. Concerning the relationship between SF and BE, β = −0.437, t = −7.518, and p < 0.01; regarding the relationship between BI x SF, β = 0.827, t = 7.861, and p < 0.01. Based on the above, the moderating effect of SF is the relationship between BI and BE. Regarding the moderating effect, according to Fig. 2, when the SF created by the metaverse is substantial, the BI of supply chain partners in the metaverse increases considerably and further enhances BE. Based on the above results, H7 is supported.
Based on the analysis results, we conclude with the conceptual framework and hypotheses, as presented in Table 9.
Analysis results for hypotheses.
We adopt two methods, culling variables and random screening of the sample, for the robustness analysis. A total of 167 samples are randomly screened. The culling variable test results are listed in Table 10, and the random screening analysis results are listed in Table 11. When compared with the analysis results in Table 7, we find that the analysis results in Tables 10 and 11 correspond to those in Table 7. Based on the above discussion, we find that the robustness and stability of the analysis results meet the requirements. Thus, the robustness analysis helps us demonstrate the importance of these four drivers.
Robustness analysis results in culling variables.
Model 1 (Removing PE) | Model 2 (Removing SI) | Model 3 (Removing FCs) | Model 4 (Removing TR) | Model 5 (Removing SF) | |
---|---|---|---|---|---|
PE → BI | 0.391 (3.528⁎⁎) | 0.454 (3.583⁎⁎) | 0.452 (3.795⁎⁎) | 0.478 (4.064⁎⁎) | |
SI → BI | 0.120 (1.122) | 0.074 (0.629) | −0.031 (−0.273) | −0.038 (−0.341) | |
FCs → BI | 0.547 (4.520⁎⁎) | 0.512 (4.402⁎⁎) | 0.591 (5.170⁎⁎) | 0.504 (4.648⁎⁎) | |
TR → BI | 0.436 (3.573⁎⁎) | 0.380 (3.146⁎⁎) | 0.533 (4.280⁎⁎) | 0.485 (4.471⁎⁎) | |
SF → BI | 0.375 (3.206⁎⁎) | 0.317 (2.658⁎⁎) | 0.371 (3.093⁎⁎) | 0.416 (3.772⁎⁎) | |
SF → BE | 0.409 (2.986⁎⁎) | 0.387 (2.724⁎⁎) | 0.355 (2.536⁎⁎) | 0.328 (2.426⁎⁎) | |
BI → BE | 0.737 (6.467⁎⁎) | 0.779 (6.717⁎⁎) | 0.789 (6.437⁎⁎) | 0.806 (6.537⁎⁎) | 0.954 (9.167⁎⁎) |
*p-value ≦ 0.05.
According to the results of the analysis, three constructs–PE, FCs, and TR–have positive effects on BI; SF has mediating effects on the relationship between TR, BI, and BE. Additionally, BI has a positive effect on BE, and SF has a moderating effect on the relationship between BI and BE. We discuss the results as follows.
First, PE can drive and affect the intention to adopt the metaverse. Specifically, if the metaverse cannot show significant efficiency in enhancing supply chain resilience, then individuals or supply chain members will lose faith, reducing their adoption intention. Therefore, to implement a metaverse strategy, managers should consider how to demonstrate the efficiency of the metaverse in enhancing supply chain resilience and raising members’ expectations of performance. For example, Abbate et al. (2022) and Choi et al. (2022b) indicated that if digital technology can offer high recognition efficiency for a supply chain in terms of resilience and sustainable development, it will raise participants’ interest, and the new technology will be widely implemented in a supply chain operational environment. Thus, our findings match those of previous studies.
Second, FCs can drive and affect metaverse adoption intention. Specifically, the use of the metaverse depends on the combination of six information technologies: blockchain, lifelogging, mirror worlds, AR/VR, and AI. Supporting the resources required by these technologies plays an important role in the metaverse. Despite their focus on technical resources, FCs are also involved in organisational support. Emhmed et al. (2021) indicated that organisational FCs, such as management or human resource support, have a significant influence on the adoption of new technology. Therefore, it is an important resource that drives adoption intention. If technical and organisational resources are lacking, then the practice of the metaverse will be affected. Finally, enhancing resilience using meta-verse methods is difficult. Based on the above discussion, FCs may lay the foundation that drives and affects the metaverse's adoption intentions. However, the analysis results of Lee and Kim (2022) indicated that FCs do not affect the user adoption intention of the metaverse. However, their research focused only on Ifland, a business metaverse platform. Users of a metaverse business platform interact only with other individuals through the platform and never rely on it for important business activities. Therefore, technical support conditions were not important in their study. However, if their study had focused on the organisational support of FCs, then we believe that the analysis results would have matched our results. Enhancing resilience is an important operation in a supply chain that must be continuously improved. If metaverse can enhance resilience, its usage should remain stable. Therefore, the FCs play an important role in this process. Therefore, our findings differ from those of the previous studies.
Our findings indicate that TR considerably affects BI. Specifically, new ICTs must be implemented through cooperation amongst supply chain members. However, each member or partner is independent. Because partners lack cooperation experience in new ICTs, they usually perceive risk and worry about cooperation risk, which limits their benefits. Based on the above discussion, it is difficult to arrive at a consensus on new ICT adoption to protect one's own benefits and avoid risks. However, if participants lack trust, their intention to implement new ICTs is affected. Based on the above discussion, when initial trust is established, then partners will establish a network relationship, and they are willing to bear the perceived risk and adopt new ICTs. They will formulate a consensus on adopting the new ICTs. The results of Van der Sijde et al. (2015) and Yuen et al. (2022) support the influence of initial trust on adoption intentions. Therefore, our findings are consistent with those of previous studies.
In this study, we propose that SI indicates that when different supply chain partners understand the value of the metaverse, other partners are affected, raising their adoption intention. However, our results indicated that SI has no positive effect on BI. Our results highlight the importance of ‘seeing is believing’ in the supply chain operational environment. Thus, if the metaverse cannot demonstrate its efficiency in enhancing supply chain resilience for every partner, it will be difficult to attract other partners, further affecting adoption intention. Therefore, although some partners may believe in the metaverse's efficiency, if one partner cannot understand its efficiency in enhancing supply chain resilience, then it would be impossible to raise the overall adoption intention.
Finally, this study explores the mediating and moderating effects of SF. First, SF is a complementary partial mediator in the relationship between TR and BI. This indicates that SF can strengthen the relationship between TR and BI. As mentioned earlier, when initial trust is established, although it increases the adoption intention of the metaverse, it means that partners need to bear the perceived risk. However, if SF has a complementary partial mediating effect, then the establishment of the degree of initial trust substantially increases metaverse adoption intention. We believe that the main reason for this is SF efficiency. Di Pietro and Cresci (2021) claimed that the metaverse can provide users with unprecedented SF in a virtual communication environment. According to Heller and Bar-Zeev (2021), greater levels of SF can promote partners’ trust in a virtual communication environment. When trust is promoted through SF, it features efficiency in knowledge-sharing and enhances resilience. These efficiencies can reduce the perceived risk and strengthen partners’ confidence, thereby raising their initial trust and increasing their adoption intention. Second, our findings show that SF is a full mediator between TR and BE. When SF features resilience efficiency, increasing the initial trust degree drives the intention to use the technology. Based on the above discussion, we find that SF has a greater influence on changing the initial degree of trust. Third, our findings reveal that SF has a moderating effect on the relationship between BI and BE. According to the verification of mediating effects, we know that SF has a critical influence. However, the level of SF experienced by supply chain members using smart devices to access the metaverse varies. High-quality SF enables members to communicate and collaborate more quickly and effectively than low-quality SF. Generally, FtFC is regarded as having a higher level of SF. Hence, whether a smart device can match the effectiveness of FtFC in accessing the metaverse will enhance the effectiveness of knowledge sharing between supply chain partners, thus building resilience. Therefore, highlighting the efficiency of SF can drive and affect the adoption behaviour of metaverse supply chain partners. However, when SF efficiency decreases, the efficiency of the metaverse in supply chain resilience is affected. Although the supply chain partners have adoption intentions, they may reduce their actual usage intentions.
ImplicationsBased on these results, this study has the following academic and managerial implications.
Academic implicationsIn terms of academic implications, this study develops a model to explore adoption behaviour with respect to metaverse technology in the context of supply chain resilience. The metaverse was first introduced in 1992. However, over the past two decades, its application has been limited to marketing, in which companies create a virtual environment to enrich their customer shopping experience. Owing to the COVID-19 pandemic, researchers and practitioners have recognised that existing levels of resilience are poor, but the metaverse can be adopted in the context of supply chain knowledge sharing to strengthen resilience. Few studies have explored the application of the metaverse in supply chain resilience. However, certain factors, such as drivers, may affect the adoption intention of supply chain partners regarding the metaverse. A few existing studies, such as those of Di Pietro and Cresci (2021), Heller and Bar-Zeev (2021), Abbate et al. (2022), and Choi et al. (2022b), have recently explored this issue; nevertheless, they have focused only on a single affecting factor. Lee and Kim (2022) focused on Korea's metaverse business platform and expanded its scope to explore the related factors that affect use intention. Their analysis provided valuable suggestions but did not fully focus on the supply chain's operational environment. Our study focuses on supply chain resilience and explores the factors affecting the adoption behaviours of supply chain partners. Moreover, it expands the research scope to explore and identify related factors affecting adoption behaviour.
Managerial implicationsAlthough the adoption of the metaverse can address the challenges of establishing and maintaining trust and communication with partners and can improve knowledge sharing and supply chain resilience, it is difficult to require every partner to adopt it. Our results indicate several directions for developing related strategies or approaches, reorganising cooperation models, and integrating resources to increase adoption intentions. Thus, this study provides important managerial implications for engineering managers trying to explore how to successfully implement a metaverse strategy to enhance supply chain resilience.
PE, FCs, and TR are the three critical drivers affecting the intention to adopt the metaverse. Although the metaverse has a positive effect in promoting supply chain resilience, if partners are difficult to understand in this environment or experience inefficiencies, they will lack PE and reduce their adoption behaviour. Thus, engineering managers, especially those from leading firms in the supply chain, should guide their partners in understanding or creating an experimental environment to demonstrate the efficiency of the metaverse and increase their partners’ PE. In addition, the metaverse is an innovative ICT that depends on complicated information technology support. Therefore, if there is a lack of technology-facilitating conditions to support the metaverse, partners may be sceptical, reducing their adoption intention and affecting the implementation of the metaverse. To implement the metaverse, engineering managers, especially project and industrial engineering managers, should ensure that technologically FCs are adequate. Finally, establishing TR is necessary to drive adoption intention. However, if partners never cooperate in new ICTs, they are likely to be concerned about perceived risk. Our findings indicate that SF improves the intention to establish initial trust and drives participants to adopt, and even use, metaverse trust. Thus, when engineering managers, especially from leading firms in the supply chain, hope to implement the metaverse to enhance supply chain resilience, they should focus on demonstrating that the efficiency of SF can address the trust problem in communication and the knowledge-sharing process. When partners experience the efficiency of SF, they change their intention to establish initial trust and even bear the perceived risk, further raising their metaverse adoption behaviour.
ConclusionThis study explores metaverse adoption behaviour in the context of supply chain resilience. We develop a conceptual framework in accordance with the UTAUT, network theory, and the characteristics of the metaverse, and use the CB-SEM approach to estimate it. Based on these results, this study contributes new knowledge. First, raising PE can drive supply chain partners to adopt the metaverse to enhance supply chain resilience. Second, FCs have good preparation and support, which affect the adoption intention of supply chain partners. Third, if supply chain partners are willing to bear perceived risk and establish TR with each other, then a network relationship will be established, a consensus will be reached, and adoption intention will increase. Fourth, although some partners understand the value of the metaverse in enhancing supply chain resilience, this does not mean that other partners will be affected or will increase their adoption intention. This means that the SI cannot drive or affect the adoption intention of supply chain partners. Additionally, SF is a critical characteristic of the metaverse, and its efficiency can improve trust, further enhancing knowledge sharing and supply chain resilience. When SF features resilience efficiency, it will raise the partners’ confidence, increase the intention to establish initial trust, and continue to strengthen the adoption intention of the metaverse. This drives the partners to believe that the efficiency of SF can help them reduce their perceived risk. Increasing SF efficiency can improve the degree of TR between partners, which can even drive partners’ technology-usage intentions. Finally, if the efficiency of the SF cannot remain stable, although partners still have adoption intentions, they may reduce their technology usage intentions. Thus, maintaining SF efficiency is a critical factor in driving and maintaining adoption and usage intentions.
This study has the following limitations with respect to the sampling approach and verification of the analysis results. First, regarding the sampling approach, we employ a government-provided list of 544 manufacturers who had improved or planned a metaverse implementation to strengthen supply chain resilience. We then employ data from 209 respondents to analyse the research question. Although sample sourcing is from a government source and we also analysed CMB and non-response bias, sample bias is still a possible concern. To address this problem, a strict sampling approach should be adopted to strengthen analytical results. Future research could use the same conceptual framework for a deeper analysis. Second, our analysis results are derived from data and statistical approaches. However, the data are from manufacturers that improved and planned the metaverse application of supply chain resilience. However, this was only preliminary and might have affected the credibility of the results. Additional observational results from real cases should be combined to enhance the credibility of the results. Furthermore, the development of metaverse supply chain resilience remains in its infancy. Therefore, it is difficult to find more real cases to verify our results, which is a limitation of this study. Thus, as the application of the metaverse in supply chain knowledge sharing and resilience gradually matures, future research could analyse and observe more real cases to verify our analysis results. In addition, our study is conducted in the context of Chinese manufacturers; management and behavioural approaches in other regions may differ. Hence, expanding the scope of this study beyond Chinese manufacturers is necessary to enhance its generalizability in future research.
Disclosure statementThis paper has not any competing financial, professional, or personal interests from other parties.
Data availability statementThe data used to support the findings of this study are included within the article.
This work was supported by Zhejiang Provincial Philosophy and Social Sciences Planning Project [Grant Numbers: No. 23NDJC306YB], National Natural Science Foundation of China [Grant Numbers: No. 71872131], the Starting Research Fund of Great Bay University [Grant Numbers: No. YJKY220020].
Questionnaire content, variables for each construct and sources
Construct | Variables | Source |
---|---|---|
Performance expectancy (PE) | PE1. I would find metaverse technologies to be useful in improving supply chain knowledge sharing and resilience | Wong et al. (2020); Scaff. (2022) |
PE2. Using metaverse technologies enables our company to improve supply chain knowledge sharing and resilience more quickly | ||
PE3. Using metaverse technologies increases our supply chain knowledge sharing and resilience | ||
PE4. If I used metaverse technologies, I would increase supply chain knowledge sharing and resilience and thus increase my probability of attaining a raise in pay | ||
Social influence (SI) | SI1. People who influence the improvement of the supply chain knowledge sharing and resilience of our company should use metaverse technologies | Lee and Kim (2022) |
SI2. People who are important to me think that I should use the metaverse to improve supply chain knowledge sharing and resilience | ||
SI3. The senior management of this business has been helpful with respect to using the metaverse to improve supply chain knowledge sharing and resilience | ||
SI4. In general, the organisation has supported the use of metaverse technology to improve supply chain knowledge sharing and resilience | ||
Facilitating conditions (FC) | FC1. Our company has the necessary resources to use metaverse technology to improve supply chain knowledge sharing and resilience | Lee and Kim (2022) |
FC2. Our company has the knowledge necessary to use metaverse technology to improve supply chain knowledge sharing and resilience | ||
FC3. Metaverse technologies are compatible with other systems we use to improve supply chain knowledge sharing and resilience | ||
FC4. A specific person and group are available to assist in case of metaverse-related difficulties | ||
Initial trust amongst supply chain partners (TR) | TR1. I think that I can trust supply chain partners when implementing the metaverse to improve supply chain knowledge sharing and resilience | Queiroz and Wamba. (2019); Kathiala (2022) |
TR2. We can trust the supply chain partners to carry out metaverse communication faithfully when adopting the metaverse to improve supply chain knowledge sharing and resilience | ||
TR3. In my opinion, supply chain partners are trustworthy when implementing the metaverse to improve supply chain knowledge sharing and resilience | ||
TR4. I trust supply chain partners to keep my best interests in mind after implementing the metaverse to improve supply chain knowledge sharing and resilience | ||
Behavioural intention (BI) | BI1. I intend to use metaverse in the following weeks to improve supply chain knowledge sharing and resilience | Queiroz and Wamba. (2019); Lee and Kim (2022) |
BI2. I predict that I will use metaverse in the following weeks to improve supply chain knowledge sharing and resilience | ||
BI3. I plan to use metaverse in the following weeks to improve supply chain knowledge sharing and resilience | ||
Behavioural expectation (BE) | ||
BE1. I expect to use metaverse in the following weeks to improve supply chain knowledge sharing and resilience | Maruping et al. (2017); Queiroz and Wamba. (2019) | |
BE2. I will use metaverse in the following weeks to improve supply chain knowledge sharing and resilience | ||
BE3. I am likely to use metaverse in the following weeks to improve supply chain knowledge sharing and resilience | ||
Sensory feedback (SF) | SF1. I can experience the sensory feedback of engaging completely with others in a metaverse communication environment | Sharan et al. (2021); Kreijns et al. (2021); Lee and Kim (2022) |
SF2. I believe that the sensory feedback provided by the communication environment of the metaverse is consistent with face-to-face communication | ||
SF3. I can experience the sensory feedback provided by the communication environment of the metaverse (such as facial expressions or body language) | ||
SF4. I can examine the sensory feedback provided by the communication environment of the metaverse |