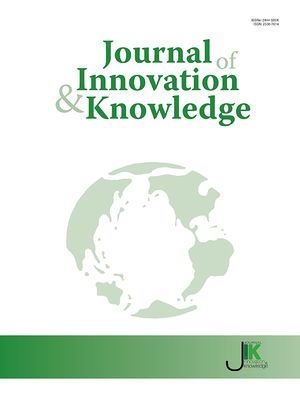
Edited by: Abbas Mardari
More infoFood businesses, especially those facing supply chain challenges, operate within a global environment characterized by uncertainty. The effects of the COVID-19 pandemic have underscored substantial losses in this sector. Our study aims to develop a mixed-formative-reflexive construct model that evaluates the impact of relational practices and resilience on the performance of enterprises within the Romanian food chain during crises. To select the sample, we employed a proportional stratified sampling method. The survey design included establishing the demographic profile of respondents, constructing items, and identifying latent variables specific to the proposed construct model. Data collection was conducted through an online questionnaire, resulting in 298 valid responses and a response rate of 35.06 %. Hypothesis testing and validation of the proposed construct model were performed using the Partial Least Squares Structural Equation Modeling (PLS-SEM) method. The research findings indicate that 56.9 % of the variance in food supply chain resilience can be attributed to the combined effects of proactive, reactive, and network design capabilities. The practical implications of the research suggest a need to reconsider planning strategies during crises. This involves reassessing risks, designing or redesigning products, and improving communication and collaboration with customers, suppliers, and other stakeholders. Therefore, enhancing relational practices and resilience can significantly and directly improve the performance of the food supply chain.
Many studies have shown that, in recent years, the "coronavirus crisis" has caused numerous problems in supply chains, including those in the food sector (Hobbs, 2020). Supply chain disruptions have been the most common issue faced by enterprises worldwide, irrespective of country or continent (Khan et al., 2022). As a result, during the emergency and quarantine stages, affected enterprises have turned to e-commerce in an attempt to improve their production and distribution operations, providing substantial support for buyers (Galhotra & Dewan, 2020).
Another issue concerns products and prices, as delays in the delivery of final products, changes in stock levels, shifting suppliers, price increases, and profit decreases have also impacted e-commerce. Lockdowns, social distancing measures, and quarantine have disrupted supply chain operations, causing labour shortages and transportation blockages. Reduced labour availability in processing plants and distribution centres has led to a slowdown in production and product shortages. Additionally, the pandemic has caused significant food waste due to the closure of restaurants, schools, and other food service establishments, resulting in many food products remaining unsold or uncollected at various points in the supply chain Aldaco et al. (2020). For most enterprises, inventory management has been a significant challenge. Many businesses struggled with managing inventory as consumer demand patterns rapidly changed during and after the pandemic. Common issues included stock depletion and shortages, leading to financial losses. Many businesses faced product shortages, high inventory costs, and decreased performance in supply chains because they did not anticipate the crisis risks and the widespread disruption of production stocks (Ivanov, 2024).
Four essential trends have influenced consumer behaviour during the pandemic: economic impact, erosion of trust, digital growth, and home becoming the new centre of activity (Gu et al., 2021). Consumer panic, reflected in buying and hoarding, disrupted the supply chain as retailers struggled to cope with sudden increases in demand. Most businesses in the supply chain faced issues such as the lack of digital infrastructure needed to quickly adapt to changing circumstances and efficiently track and manage products, as well as changes in regulations regarding food safety and customer health.
These challenges necessitated significant investments in digitization and technology adaptation (Vern et al., 2022), along with the development of new regulations regarding food safety and the protection of employees and customers (Maragoni-Santos et al., 2022). This occurred against a backdrop of vulnerability arising from dependence on a single supply source or a specific region for production (Zhu et al., 2020).
E-commerce and the food supply chain are interconnected in various ways (Al Mashalah et al., 2022). One key aspect is efficiency and visibility. Online platforms offer consumers an effective means to access a diverse range of food products from the convenience of their homes. A recent study showed that the most frequently cited reasons for online food purchases include the quality-price ratio, concerns about safety and quality, the convenience of the purchase, and the reviews of others (Wang et al., 2020).
The second aspect is related to resilience. E-commerce can enhance the resilience of the food supply chain only if all external determinants of resilience and vulnerability are taken into account, such as natural disasters, wars, pandemics, and transportation issues (Zhang et al., 2023). The pandemic has accelerated the rapid adoption of innovations, the spread of e-commerce, and the use of delivery intermediaries, allowing businesses in the food sector to redesign their supply chains to enhance resilience and contribute to food security (Reardon et al., 2021).
The third aspect involves relational practices. Online platforms engage various stakeholders - producers, distributors, retailers, and consumers - who can develop direct relationships. For example, consumers can purchase directly from local farmers or producers, bypassing intermediaries. E-commerce platforms can generate vast amounts of data on consumer behaviour, including preferences, buying patterns, and feedback through reviews, ratings, and product comments. This data allows food producers to adapt their offerings and improve relational practices. As consumer preferences for e-commerce and the quality of retail services increase, service costs decrease, and the rate of recovery of used products rises, contributing to improved supply chain performance (Mu et al., 2020).
The last aspect is related to maintaining performance. E-commerce can significantly influence the performance of the food supply chain through inventory management, demand forecasting, increasing customer satisfaction, and reducing costs. Online platforms often employ advanced inventory management systems, which can reduce food waste and enhance the overall efficiency of the supply chain Rodrigues et al. (2021). The data generated by e-commerce can improve demand forecasting, allowing suppliers to optimize their production and distribution. By streamlining operations, reducing the need for physical retail spaces, and improving supply chain efficiency, e-commerce can lower costs for both businesses and consumers. In short, e-commerce is deeply integrated into the food supply chain, offering numerous benefits.
Currently, there is a continuous need to explore the link between e-commerce, resilience, and the performance of the food supply chain, especially in times of crisis. While extensive research exists on resilience as a mechanism for anticipating, adapting to, and overcoming potential disruptions in supply chains, this study makes several vital contributions to the existing literature and practice. It introduces a formative structural model, highlighting the importance and combined effect of exogenous variables - proactive capacity, reactive capacity, and network design - on the resilience of the food supply chain under the pressures of the pandemic crisis.
Only two empirical studies uniquely emphasize the moderating role of relational practices on supply chain performance (Chowdhury et al., 2019, 2023). Consequently, this study contributes to the existing but limited literature on relational practices and food supply chain performance during crises by introducing a reflexive construction model. This model allows for the exploration of the resilience effect on the performance of the food supply chain and highlights the role and effects of relational practices on enterprise resilience strategies and performance improvement in crisis conditions.
The lack of studies providing solutions to issues such as operational interruptions or blockages, food waste, inventory management, adaptation to changes in consumer behaviour, improvement of digital infrastructure, and alignment with new food regulations underscores the need to find ways to enhance the resilience and performance of the entire food supply chain in crisis conditions.
This study aims to develop and validate a multidimensional mixed formative-reflexive model measuring the combined impact of relational practices and resilience on the performance of Romanian enterprises in the food supply chain during crisis conditions.
The main beneficiaries of this study are food supply chains and managers of all involved enterprises. They will discover the importance of using proactive capacity, reactive capacity, and network design to reduce or mitigate disruptions, minimize damages, and improve recovery within the food supply chain. Additionally, the study identifies the unique roles of resilience and relational practices in enhancing the performance of the food supply chain in crisis conditions, contributing to a better understanding of how solutions to emerging issues can be found and how these emergency resources can be efficiently managed.
The paper is structured as follows: Section 2 delves into the most significant scientific works on the resilience of the food supply chain and its key relational practices; Section 3 outlines the research methodology; Section 4 unveils the results derived from applying the PLS-SEM algorithm; finally, Section 5 accentuates the theoretical and practical implications, limitations in marketing research, and points toward future directions.
Literature reviewThe supply chain is a complex system involving organizations, human, technological, and financial resources, activities, and information, all working towards the goal of moving products and services from suppliers to customers (Lam, 2018). Managing the food supply chain relies on coordinating the efforts of participating enterprises, which involves the exchange of information and collaborative planning to enhance efficiency and competitiveness. Its success largely depends on the integration of several key aspects: the development of effective and efficient logistical capabilities, knowledge of costs per customer, improvement of the organizational structure, provision of high-quality, personalized services, intensified cooperation between suppliers, development of sustainable relations, and increased international cooperation (Bălan, 2021).
The food supply chain presents unique challenges and characteristics compared to other sectors due to the movement of perishable products that directly impact public health. The perishable nature of food products necessitates strict inventory control, rapid turnover, and efficient transportation. Regulatory requirements related to food safety, labelling, traceability, and quality standards are stringent and numerous. Contamination or mishandling of food products can lead to public health crises and widespread illness (Gurrala & Hariga, 2022). Continuous control of quality, hygiene, and traceability is essential throughout the entire supply chain Han et al. (2019). Food production in many countries, including Romania, is seasonal and subject to geographical variations, leading to supply and distribution chain challenges. The chain involves various stakeholders, from farmers and processors to distributors, retailers, and restaurants, each playing a significant role in ensuring the transfer of food products from suppliers to consumers (Bhattacharya & Fayezi, 2021).
Consumer preferences and food trends significantly influence the food sector (Köhler & Pizzol, 2020). Therefore, all parties involved in the food supply chain must be agile and responsive to shifts in demand and frequently adjust their supply, production, and distribution strategies. Sustainable practices must align with consumer requirements, expectations, and regulations. The increasing consumer interest in knowing the origin of food, how it was produced, and its environmental impact poses a significant challenge in ensuring transparency and traceability in the supply chain Scholten et al. (2016). Menon and Jain (2024) have demonstrated that the implementation of blockchain in agri-food supply chains improves distribution, provides transparency regarding the origin and sources of agri-food products, and ensures safety and quality assurance for food products. Therefore, integrating blockchain brings traceability, immutability, auditability, and provenance, promoting transparency in supply chains (Köhler & Pizzol, 2020). Given these unique characteristics and challenges, the food supply chain requires a high degree of resilience, adaptability, and rigorous quality control measures. To remain effective, this sector must continuously innovate and embrace technology to address these challenges, prioritizing consumer safety and well-being.
The heightened market dynamics and turbulence caused by the COVID-19 pandemic have disrupted the food supply chain, affecting its efficiency and effectiveness. Declining economic growth, declining food prices, declining incomes, disruptions in the food supply chain, intensified consumer panic buying behaviour regarding basic foods, abrupt changes in consumption patterns with an increase in prepared meals and those consumed at home, and the lack of local labour associated with the pandemic have all contributed to increasing food insecurity in most countries. Due to the short duration of the shock and the inelasticity of demand for most agricultural commodities, global food consumption has been minimally affected (Hobbs, 2020). From an environmental perspective, the impact of COVID-19 has contributed to a reduction of approximately 1 % in direct greenhouse gases from agriculture, equivalent to 50 million tons of carbon dioxide during the period 2020–2021 (Elleby et al., 2020).
The concept of resilience, with its multidisciplinary approach, emphasizes a system's capacity to protect its assets, adapt to changes, and recover from damages promptly (Firesmith, 2019).
Supply chain proactive capability (SCPC)Melnyk et al. (2014) argue that food supply chain businesses need to develop proactive capacity to recover business and operational costs after a series of disruptions. Siagian et al. (2021) emphasize the role of management in adapting and integrating business strategies, as well as the skills and functional competencies necessary to anticipate and adapt to environmental disturbances. Proactive capacity also involves the ability of management to adapt, integrate, and reconfigure all available resources of the enterprises to quickly overcome environmental changes and vulnerabilities in the food supply chain Ambulkar et al. (2015). Flexibility in the food supply chain refers to the ability to respond swiftly to external changes and return to normalcy, enabling businesses to maintain their resources and grow steadily (Yu et al., 2018). Being flexible means developing contingency plans for all processes involved in the network, from product manufacturing to delivery to the end-user (Tarigan et al., 2021). Redundancy in the food supply chain should support the vulnerable points of the network, prevent slowdowns or overcome blockages, and provide safety stocks in case of delivery errors or losses. This aims at intentional duplication of components to increase the system's reliability (Chowdhury et al., 2019). Swafford et al. (2008) indicated that IT integration allows enterprises to exploit supply chain flexibility, leading to increased agility and, implicitly, business performance. Tarigan et al. (2021) view supply chain efficiency in terms of the rapid development of food processes and timely delivery, while enhancing food quality in compliance with customer expectations.
The existing literature indicates limited empirical research in the field of food supply chain proactive capability, especially related to its integration with other concepts such as sustainability, resilience, and digitization (Gurrala & Hariga, 2022). There is a need for more empirical studies to understand how to adapt proactive capability to the specific requirements of the perishable supply chain and to identify new variables and their influence on chain resilience and performance. Mishra et al. (2022) identified poor communication between businesses and inadequate flexibility in the agricultural supply chain during crises. They noted that the situation worsened due to post-COVID-19 lockdown measures, lack of managerial commitment and support, poor communication and collaboration between businesses, limited use of ICT, and reduced inputs at ground level, leading to low resilience. Furthermore, implementing redundancy resulted in increased supply chain costs due to additional sources, safety inventory, and alternative transportation methods, reducing profitability. Pavlov et al. (2019) highlighted a gap in new methodological approaches for modelling network redundancy optimization, calling for new models for analysis, calculation, and optimization of supply chain redundancy as well as proactive contingency plans based on supply types and structural disruption risks.
The main limitations hindering the implementation and management of proactive capacity at the supply chain level are related to the low financial and technological resources of small and medium-sized enterprises in the food sector, the complexity of the chains, strict government regulations related to labelling, storage, and transport, as well as risks specific to the food industry such as contamination, seasonal changes, volatile raw material prices, and stringent food safety requirements. Tomlin and Wang (2011) showed that the lack of viable operational strategies at the enterprise level hinders the implementation of proactive capability and complicates the management of supply chain disruption risks. They explain that stockpiling, supply diversification, reserve sourcing, demand management, and supply chain consolidation are key operational strategies that managers should consider when developing and implementing an overall disruption mitigation strategy.
Proactive capacity was reflected and measured by the following elements: flexibility, redundancy, integration, and efficiency. Considering the above, the authors proposed the following hypothesis:
Hypothesis 1 (H1): Supply Chain Proactive Capability (SCPC) has a positive, significant impact on Food Supply Chain Resilience (FSCR).
Supply chain reactive capabilities are often depicted through the ability of enterprise management to respond swiftly to environmental changes and recover from disruptions (Jüttner & Maklan, 2011; Winter & Knemeyer, 2013). Wieland and Wallenburg (2013) propose a series of reactive strategies in the post-disruption phase to strengthen food supply chain resilience (FSCR) and support enterprise performance. These strategies encompass response, recovery, and growth phases following a disruption. Elements of a reactive strategy include agility, collaboration, flexibility, and human resource management, all aimed at recovery and growth in the post-disruption phase. Visibility is another crucial element that can help maximize supply chain performance. Full visibility of the entire food supply chain can be achieved by implementing platforms (for procurement, warehousing, etc.) that allow clarity, control, and execution across all operational levels (Ahimbisibwe et al., 2016). Moreover, sustainable business performance among enterprises may result from the application of strategies in supply chain management and human resource skills, such as research and development, technology commercialization, production capacity, and marketing capacity (Wieland, 2021).
Patel and Sambasivan (2022) highlighted the existence of gaps and future research directions for a more comprehensive understanding of supply chain agility. By understanding the role of enabler modelling, assessment, implementation, agility, and agility maximization, supply chain managers will find it easier to remain competitive in today's increasingly dynamic and turbulent business environment. Caridi et al. (2014) noted gaps regarding the evaluation of benefits offered by supply chain visibility. Zhang and Su (2020) assert that few studies address issues related to reducing quality risks, ensuring safety, food security, and sustainability of performance, which depend primarily on improving quality visibility throughout the food supply chain. McAfee et al. (2002) identified a research gap in human resources management and proposed four different logistics strategies for enterprises to implement in the selection of supply chain partners, considering the impact of organizational culture, human resource management policies, and the orientation towards transactions/employee relations. Although recent literature extensively covers supply chain resilience, there is still a lack of research on theorizing resilience capabilities, particularly the measurement of reactive capability. Faruquee et al. (2023) found that reactive resilience capabilities, more than proactive ones, significantly impact performance indicators and are essential for ultimate supply chain resilience strategies. Nha Trang et al. (2022) investigated the interest gap in supply chain collaboration, demonstrating its importance in enhancing the performance of cold supply chains in agriculture and food. Applying appropriate strategies can generate a greater impact of collaborative partnerships on supply chain performance. Constraints on the implementation of supply chain management in the food processing industry include significant issues with human resources, technologies, facilities, supplier relationship management, customer relationship management, regulatory factors, and logistics and transportation, which support vulnerability and threaten viability in any country. Nguegan Nguegan and Mafini (2017) emphasized the need to understand the sources of these problems, utilize this information, and implement supply chain management practices to improve business performance in the food processing industry.
For this study, the elements defining the reactive capacity of the supply chain were agility, visibility, collaboration, and human resources management. Consequently, the authors proposed the following hypothesis:
Hypothesis 2 (H2): Supply Chain Reactive Capability (SCRC) has a positive, significant impact on Food Supply Chain Resilience (FSCR).
Supply Chain Network Design (SCND), also referred to as supply chain strategic planning, is a comprehensive process involved in shaping and configuring a supply chain. This process focuses on determining optimal locations and sizes for all units within the network to ensure efficient management of resources and locations (Jouzdani & Govindan, 2021). When designing the supply chain network, businesses must consider various elements, such as production facilities, warehouses, distribution centres, available transportation networks, customer locations, and labour costs. These elements must be optimized to maximize the efficiency of the entire system. Schmidt and Wilhelm (2000) demonstrated that modelling a competitive global supply chain requires addressing three interacting decision levels: operational, tactical, and strategic. Shen (2007) identified three types of SCND: location-routing, inventory-routing, and location-inventory, although routing decisions are primarily concerned with vehicle management (Lemmens et al., 2016). In 2010, Klibi et al. (2010) suggested incorporating uncertainty and discontinuities into SCND modelling. An SCND is vulnerable to risks of discontinuities, which can arise from high-risk but low-impact events, or from low-probability but high-impact events. Researchers argue that SCND may include environmental objectives (e.g., global warming, eco-indicator 99) related to environmental responsibility (Dreyer et al., 2003; Garcia & You, 2015), economic responsibility objectives such as tax obligations or shareholder returns, profitability through cost control, and social responsibility aspects like job creation (Liu et al., 2017; You et al., 2012) or social welfare (Mota et al., 2014; Mota et al., 2015; Simões et al., 2014).
SCND in the food industry presents challenges due to network complexity, specific storage and transportation requirements, and demand variability (Jain & Benyoucef, 2008). The complexity of supply chains, which include multiple stages of procurement, production, distribution, and transportation, is particularly pronounced for perishable products. Food storage and transport require specific controls for temperature, hygiene, and safety, adding layers of complexity to network design. Seasonal and variable demand for food products further complicates the design process. To better align supply and demand and manage market equilibrium prices and quantities, many supply chain businesses are increasingly adopting automated machinery and equipment to replace manual labour (Rakhra et al., 2022). Despite the wealth of valuable studies available, further research is needed in less explored industries, such as aviation, heavy machinery, and service sectors, to develop a sufficiently detailed and complete model for SCND (Corominas et al., 2015). Limitations in food supply chain design include the high costs associated with constructing and managing warehouses and production facilities, along with the limited financial and human resources of many enterprises, particularly small and medium-sized ones.
Teodorescu and Korchagina (2021) demonstrated that integrating innovative technologies can enhance the efficiency, transparency, and verifiability of supply chains. However, implementing such technologies remains technically challenging and requires significant financial investment. Differentiation and adaptation of large food stocks can mitigate spoilage and loss risks, particularly for perishable products, thereby positively impacting financial and logistical aspects. The food sector has faced stringent regulations and standards related to food safety, labelling, quality, and sustainability. Therefore, supply chain design must address each of these requirements. Rao et al. (2021) noted that current EU food safety legislation still lacks sufficient regulations for food processing by-products. Bridging this regulatory gap could involve adopting private food safety standards that address sustainability issues in food supply chains and designing feasible decision trees for food product utilization, ensuring both safety and nutritional relevance. Enhancing responsiveness to climate change, supply chain disruptions, and other external factors can significantly influence network design by enabling swift and efficient management of new risks and challenges.
Based on these considerations, the authors have chosen to examine SCND through elements such as differentiation, adaptability, sustainability, and responsiveness. Consequently, the third hypothesis is proposed:
Hypothesis 3 (H3): Supply Chain Network Design (SCND) has a positive, significant impact on Food Supply Chain Resilience (FSCR).
Wieland and Durach (2021) define supply chain resilience as the capacity to endure, adapt, or transform in response to current changes. Other scholars describe resilience as the ability to survive in a challenging environment (Jamali et al., 2017) or as the capability to effectively respond to negative environmental disruptions and threats, or to recover swiftly from losses caused by such disruptions (Carvalho et al., 2012). Pettit et al. (2013) characterize supply chain resilience as “the ability of the supply chain to anticipate and overcome potential disruptions.” Disturbances in the supply chain create uncertainty (Tang, 2006) and can originate from natural or anthropogenic sources, potentially having significant impacts despite their lower likelihood of occurrence (Alikhani et al., 2021). To handle potential disruptions, supply chains must enhance their resilience.
Research indicates that resilience in the context of food supply chains involves three dimensions: anticipation, adaptation, and recovery from disruptions (Martins de Sá et al., 2019; Chowdhury & Quaddus, 2016). Anticipating market changes during crises involves mechanisms such as risk assessment and management, scenario planning, diversification, flexibility, and redundancy (Christopher & Peck, 2004; Fiksel, 2003; Jüttner & Maklan, 2011; Pettit et al., 2011; Ponomarov & Holcomb, 2009). To ensure robust resilience, food supply chain managers must conduct ongoing comprehensive risk assessments to identify potential disruptions related to natural disasters, pandemics, blockades, or regulatory changes, and develop mitigation strategies. Resilient food supply chains continuously seek to diversify their sources of supply, modes of transportation, reserve storage, and establish redundancy in critical areas (Ivanov & Sokolov, 2012; Ponomarov & Holcomb, 2009; Pettit et al., 2013).
Adaptation, a crucial aspect of supply chain resilience, includes agility, flexibility, digitalization, technology integration, and enhanced communication and collaboration with suppliers. Resilience is closely tied to the supply chain's ability to be agile and flexible (Fiksel, 2003; Ponomarov & Holcomb, 2009; Pettit et al., 2011). Supply chain managers must be innovative and capable of rapidly adjusting their strategies, turning crisis challenges into opportunities for learning and development (Messabia et al., 2022). They must make decisions under pressure, such as changing supply locations or production methods in response to disruptions. Digitalization and the adoption of new technologies are key to adaptation, including the use of real-time data for improved decision-making, advanced analytics for demand forecasting, and Internet of Things (IoT) devices. IoT technology enables data collection across various stages within supply chains, facilitating the establishment of data-driven, transparent systems for the food supply chain Astill et al. (2019). Expanding communication and collaboration with suppliers allows for quicker adaptation to disruptions through alternative supply options or expedited deliveries during crises (Ali et al., 2017; Scholten & Schilder, 2015).
The recovery dimension involves effective crisis management, increased supply chain visibility, and continuous improvement. Resilient food supply chains typically include crisis management plans that outline roles, responsibilities, communication strategies, and specific actions during disruptions. The COVID-19 pandemic highlighted the importance of investing in technology and real-time visibility into operations (James, 2019; Nilsson et al., 2019). This visibility enables quick recovery of losses, inventory localization, transportation redirection, and impact assessment of disruptions. Continuous improvement, based on post-disruption data analysis, helps managers learn from past experiences, manage disruptions better, and refine strategies for future disruptions (Béné, 2020). In the food industry, resilience involves not only recovering from disruptions but also preparing for, adapting to, and mitigating the impacts of disruptions to protect public health, ensure food safety, and maintain a consistent supply of essential products. A holistic approach, encompassing risk management, technology adoption, collaboration, and continuous improvement, is essential for building robust and adaptable supply chains (Gurrala & Hariga, 2022; Joshi & Sharma, 2021).
Contemporary markets are experiencing significant turbulence and increasing volatility, which heightens the vulnerability of food supply chains to interruptions or blockages. To measure the impact of resilience on food supply chain performance, the authors propose a multidisciplinary and hierarchical approach, addressing its particularities, attributes, dimensions of resilience, and relational practices.
In organizational theory, the resilience of a business following crises characterized by turbulence and disruptive events can be assessed through the Dynamic Capabilities View (DCV) theory (Teece et al., 2016). This theory extends the Resource-Based View (RBV) theory, emphasizing the need to identify and utilize resources that contribute to a sustainable competitive advantage (Barney, 1991; Wernerfelt, 1984). DCV theory builds on RBV by incorporating strategies for effective resource planning and managing rapid changes in uncertain markets. DCV involves the ability of an enterprise to integrate, build, and reconfigure internal and external competencies, develop new resource planning systems, and substantiate value creation strategies (Eisenhardt & Martin, 2000; Teece et al., 2016). Therefore, supply chain enterprises must develop dynamic capabilities to mitigate risks associated with vulnerability in an uncertain macroeconomic environment, which requires resilience to adapt quickly to adverse situations and seize opportunities created by changes to ensure business continuity.
DCV provides an optimal framework for understanding both proactive and reactive resilience in supply chains (Teece et al., 2016). To effectively reconfigure resources and capabilities, businesses must be proactive by examining environmental changes, updating budgets, streamlining processes, and modifying IT infrastructure to prevent performance issues. In our study, these aspects are proportional to the proactive capacity of the supply chain. Enterprises that can effectively manage dynamic changes in the business environment are more likely to survive and thrive in the future (Siagian et al., 2021).
Additionally, supply chains must develop reactive capabilities by measuring, reporting, and reviewing current business performance, and proposing and implementing corrective measures to ensure optimal functioning and recovery from interruptions or closures. Expanding and adapting DCV within supply chain enterprises to support profitability can enhance long-term resilience (Ponomarov & Holcomb, 2009). In our study, we propose measuring food supply chain resilience using the two known dynamic capabilities: proactive and reactive. Based on Wieland and Wallenburg (2013), the resilience capability of a supply chain is enhanced by relational practices among supply chain partners, ultimately improving Supply Chain Performance (SCP) (Chowdhury et al., 2023) because a resilient supply chain ensures a competitive advantage for the firm (Blackhurst et al., 2011). Literature on supply chain relational practices (SCRP) indicates that trust, cooperation, and communication strengthen relational ties (Wieland & Wallenburg, 2013), while “relational embeddedness of social capital” is a critical factor in SCP (Bernardes, 2010; Chowdhury et al., 2023). Additionally, research suggests that SCP is positively influenced by Supply Chain Resilience (Chowdhury & Quaddus, 2017; Wieland & Wallenburg, 2013). If Supply Chain Resilience and SCP are positively influenced by SCRP, and Supply Chain Resilience positively affects SCP, it can be assumed that Supply Chain Resilience mediates the relationship among SCRP, Supply Chain Resilience, and SCP (Chowdhury et al., 2023).
Therefore, based on the above arguments, we hypothesize that:
Hypothesis 4 (H4): Food Supply Chain Resilience (FSCR) has a positive, significant impact on Supply Chain Relational Practices (SCRP).
Hypothesis 5 (H5): Food Supply Chain Resilience (FSCR) has a positive, significant impact on Supply Chain Performance (SCP).
Supply Chain Relational Practice (SCRP) refers to the establishment and maintenance of effective collaborative and cooperative relationships among suppliers, manufacturers, distributors, retailers, and other stakeholders involved in the production and distribution of goods and services within a supply chain. It encompasses the enhancement of Supply Chain Performance (SCP) through these relationships (Wei et al., 2012). Effective SCRP involves building trust, fostering communication, engaging in joint problem-solving, upholding commitments, and ensuring cooperation among these diverse parties (Fynes et al., 2004; Srinivasan et al., 2011). The primary objective is to improve efficiency, reduce costs, and enhance overall firm and supply chain performance (Cannon & Homburg, 2001; Shin et al., 2000).
SCRP contributes to a deeper commitment among supply chain partners towards mutual understanding and support, resulting in better management of logistics activities (Dwyer et al., 1987; Wong et al., 2012) and a reduction in operational risk (Tazelaar & Snijders, 2013). This involves sharing information, coordinating activities, and collaboratively addressing challenges within the supply chain. By fostering strong relationships and effective communication, SCRP enhances the resilience of the supply chain, enabling it to withstand disruptions and adapt to changing circumstances. Furthermore, SCRP can improve product quality and safety, reduce waste, and increase customer satisfaction. It involves developing long-term partnerships and trust, which leads to improved communication, better coordination, and increased efficiency within the supply chain.
However, the application of relational practices in food supply chains can present several challenges, including dependence on business partners, relationship complexity, and cultural differences. Relational practices may lead to excessive reliance on business partners and the transmission of their issues or unforeseen changes to the enterprise. Managing and sustaining relationships within food supply chains can be particularly challenging when dealing with many producers, suppliers, transporters, retailers, and consumers. Additionally, the diverse cultures, values, and goals of these participants can complicate relationship management. Cadden et al. (2013) found that complementarity, rather than congruence, between supply chain partners, and the adoption of a shared culture based on trust and openness, can reduce conflict, decrease uncertainty, and enhance performance throughout the supply chain.
Limitations associated with relational practices in food supply chains include scalability issues, data privacy concerns, vulnerability, and resistance to change. Challenges related to scalability can arise from improper labelling, handling errors, production faults, and the difficulties of managing numerous relationships as organizations expand (Malik et al., 2018). Vulnerabilities often emerge from changes in ownership or management within partner companies. Additionally, implementing relational practices that involve different cultures or behaviours can create resistance to change among employees and organizations (Lines, 2004). Such resistance, coupled with differing behaviours and processes, can hinder successful supply chain alliances (Fawcett et al., 2015). Sharing sensitive information and data with partners may also raise privacy and security concerns.
Nevertheless, SCRP is crucial for developing a resilient supply chain capable of rapidly adapting to changes while maintaining customer value and high firm performance (Agarwal & Selen, 2009; Wieland & Wallenburg, 2013).
Thus, it is evident that SCRPs enhance SCP. Consequently, we propose the following hypothesis:
Hypothesis 6 (H6): Supply Chain Relational Practice (SCRP) has a positive, significant impact on Supply Chain Performance (SCP).
Aslam et al. (2021) recommend that managers and decision-makers continuously evaluate the link between supply chain practices and Blockchain features, seeking viable solutions to enhance supply chain practices and improve operational performance.
Supply chain performance (SCP)Supply Chain Performance (SCP) is significantly enhanced by Supply Chain Resilience. According to Chowdhury and Quaddus (2016, 2017), Brusset and Teller (2017), Ponomarov and Holcomb (2009), and Roberta Pereira et al. (2014), resilience is widely defined as "the capability of a supply chain to develop readiness, response, and recovery capabilities to manage disruptions, and to bounce back to the pre-disruption stage or a better stage after experiencing a disruption" (Chowdhury et al., 2023).
Resilience, by preventing disruptive events, contributes to SCP (Jüttner & Maklan, 2011; Pettit et al., 2013; Ponomarov & Holcomb, 2009). Numerous studies have developed both tangible and intangible resilience capabilities to measure Supply Chain Resilience. Intangible resources include Supply Chain Relational Practices (SCRP), supply chain flexibility, redundancy, integration, efficiency, and agility. Tangible capabilities encompass logistical capacities associated with Supply Chain Resilience (Chowdhury et al., 2019; Pettit et al., 2011; Ponomarov & Holcomb, 2009; Roberta Pereira et al., 2014; Sheffi & Rice, 2005; Wieland & Wallenburg, 2013; ). Finaly, a resilient supply chain maintains "the ability to ensure high performance and customer value through high visibility and responsiveness" (Wieland & Wallenburg, 2013). In a highly competitive environment, the focus shifts from individual enterprise performance to the performance of the entire supply chain. SCP involves all activities aimed at synchronizing demand with supply, meeting end-customer requirements, creating net value, developing a competitive logistics infrastructure, and achieving continuous performance improvement (Hausman, 2004).
To gain a more comprehensive view of performance, supply chain enterprises can utilize the Balanced Scorecard, a model that integrates both strategic and financial measures. Applied to supply chains, the Balanced Scorecard evaluates performance from several perspectives: financial (analysing production and storage costs), customer (tracking on-time delivery and order completion rates), internal business processes (comparing actual production with planned production), and innovation and learning (measuring the cycle time for new product development) (Frederico et al., 2021).
A critical issue for modern enterprises is the difficulty in measuring actual performance relative to required performance, leading to uncertain and fragile supply chains. Given these challenges and the need to enhance food supply chain performance, our study will apply the Balanced Scorecard model variables. Consequently, the analysis of the latent variable, Supply Chain Performance (SCP), will be based on the four components specific to the model: Learning and Growth, Internal Processes, Customer, and Financial.
The Learning and Growth perspective is crucial for supporting any sustainable strategy, focusing on intangible assets such as internal skills and capabilities necessary for supporting value-creating processes. Research on Brazilian agribusinesses indicates that increasing collaboration and knowledge sharing, employing the latest technologies, providing staff training and continuing education, and implementing effective management control practices contribute to improved performance and play a significant role in supply chain coordination (Callado & Jack, 2021; Fernandez et al., 2021). Maull et al. (2012) examined how customer service supply chains are managed from a customer perspective, investigating the management, coordination, evaluation, and integration of business-provided services to achieve optimal pricing and product quality. For food supply chain businesses, the financial perspective includes strategies and operations aimed at improving financial health and performance. Pohjola and Stenholm (2012) developed and validated a structural model of supply chain resilience that demonstrates how dynamic regenerative and renewable capabilities influence organizational performance.
Therefore, we propose using four items to measure food supply chain performance: Learning and Growth, Internal Business Processes, Customer Experience, and Cost Reduction.
Data and methodTo assess the impact of resilience and relational practices on food supply chain performance and to validate the proposed multidimensional model, a survey was conducted from September to November 2021. The authors employed a stratified sampling method, specifically the proportional variant, which was constructed randomly. This method involves dividing the target population into strata and then randomly selecting enterprises from each stratum until the predetermined sample sizes are achieved. This approach was deemed suitable due to its ability to represent a complex set of easily identifiable criteria.
The implementation of the stratified sampling method comprised three stages. In the first stage, the relevant population was identified using the database of the National Institute of Statistics in Romania. The sampling base included Romanian enterprises engaged in the production, sale, transportation, and storage of food, all integral to various supply chains. The criteria for structuring the sampling base into strata were sector, number of employees, turnover, and market seniority. In the second stage, the strata were defined, forming the basis for sample selection. In the third stage, a predetermined number of enterprises were randomly chosen from each stratum until the sample size was reached. This ensured that the number of enterprises selected from each stratum was proportional to the stratum's representation in the overall population. The choice of this sampling method was justified by two main reasons: it generates a sample that is both highly representative and economical, and it provides information about proportions associated with each sampling criterion.
The authors distributed over 850 questionnaires to managers in the Bucharest-Ilfov region. This region was chosen due to its significant representation in Romania's supply chain, owing to its central location, economic importance, and well-developed infrastructure. Bucharest-Ilfov is strategically located in southern Romania and serves as a crucial hub for various industries and supply chain activities. It hosts numerous logistics and distribution centres as well as manufacturing enterprises across diverse sectors, including automotive, electronics, textiles, and food processing. Additionally, the region's status as a major market ensures a qualified and educated workforce and plays a vital role in supporting supply chain activities such as financing, legal compliance, and consulting services.
Out of the distributed questionnaires, 309 were returned by participants who agreed to partake in the survey, with 298 being valid after excluding 31 incomplete responses.
The response rate was 35.06 %. Given a confidence level of 0.05 and a margin of error of ± 9 %, the final sample comprised 267 respondents (; Nichifor et al., 2021; Tecău et al., 2020). Statistical formulas to determine sample size are commonly referenced in research literature (Nichifor et al., 2021; Tecău et al., 2020; ). Due to the small sample size, results should be interpreted with caution. To mitigate potential selection biases, the survey was conducted among representative Romanian enterprises, with detailed analysis of the data including socio-demographic characteristics.
Table 1 illustrates that 61.49 % of the enterprises in the sample are from the food trade sector, while 23.51 % are from the production sector. Food transport accounts for 10.44 % of the enterprises, and food storage constitutes 4.56 %. In terms of employee count, 78.26 % of the enterprises are medium or large. Over half of the enterprises report a turnover exceeding 6 million euros (53.88 %) and have been in the Romanian food market for over five years (55.17 %).
Demographic profile of respondents.
Where: N= sampling base; n = sample of respondents.
Given the current context, data collection was conducted via an online questionnaire sent by email to supply chain managers who had expressed willingness to participate. The use of email was deemed appropriate due to the target population's active online presence and daily use of email for communication. All data collection was performed with the respondents' consent, ensuring fairness, transparency, and confidentiality of the data and email addresses.
The questionnaire was structured in two parts: the first part gathered demographic information about the respondents and their enterprises, while the second part included questions related to the multidimensional structural model proposed by the authors. The questionnaire was pretested several times using samples of 12 supply chain managers (Ishak et al., 2023). The initial pretest led to the inclusion of the Supply Chain Relational Practice (SCRP) variable. Subsequent pretests improved question wording, order, and construct reliability. Feedback from pretests focused on refining language, simplifying and prioritizing questions, and ensuring clarity about the SCRP variable. Adjustments included revising language, correcting errors, and incorporating valuable suggestions to enhance the questionnaire's robustness and effectiveness.
After making the necessary corrections, the questionnaire underwent validation, also known as interactive data validation. Results demonstrated that the variables analysed met the criteria for convergent and divergent validity. The indicators for the latent variables Supply Chain Proactive Capability (SCPC), Supply Chain Reactive Capability (SCRC), and Supply Chain Network Design (SCND) exceeded the threshold of 0.5, indicating the reliability of the formative model (Hair et al., 2019). Specifically, Food Supply Chain Resilience (FSCR) (CA = 0.630, CR = 0.646, AVE = 0.728, VIF = 1.243), Supply Chain Relational Practice (SCRP) (CA = 0.823, CR = 0.825, AVE = 0.655, VIF = 1.102), and Supply Chain Performance (SCP) (CA = 0.758, CR = 0.755, AVE = 0.587) all met the conditions for Cronbach's Alpha (CA) greater than 0.6, Composite Reliability (CR) greater than or equal to 0.6 for exploratory studies, Average Variance Extracted (AVE) greater than 0.5, and Variance Inflation Factor (VIF) less than 4.0 (Henseler et al., 2014). Ultimately, 298 valid responses were obtained from supply chain managers in the Bucharest-Ilfov region.
Data measurement for marketing research utilized an ordinal Likert scale from 1 to 7 (where 1 = strongly disagree and 7 = strongly agree). The Likert scale provides a structured, quantitative means of measuring respondents' opinions, facilitating data analysis and comparison, ease of administration, flexibility, interpretability, and construct validity.
Data processing was performed using Excel and Smart PLS 3 software. The Partial Least Squares - Structural Equation Modelling (PLS-SEM) method was employed to validate the multidimensional model. Smart PLS 3 was chosen for its suitability for PLS-SEM, its user-friendly interface, and its effectiveness in predictive modelling, particularly with smaller sample sizes (Aslam et al., 2021; Chowdhury et al., 2023).
Multidimensional modelFig. 1 illustrates the proposed multidimensional model for the research. The formative model depicts the interplay between three exogenous variables: Supply Chain Proactive Capability (SCPC), Supply Chain Reactive Capability (SCRC), and Supply Chain Network Design (SCND), and the endogenous variable, Food Supply Chain Resilience (FSCR). According to the proposed model, enhancing food supply chain resilience requires the integration of proactive and reactive capabilities, alongside the strategic design of the network. The reflective model further suggests that supply chain performance (SCP) can be improved both directly through increased resilience (FSCR) and indirectly by fostering better relational practices among supply chain partners (SCRP).
In the formative model, the degree of supply chain resilience is measured by the combined effect of the latent variables SCPC, SCRC, and SCND (Jarvis et al., 2003; Pettit et al., 2013). Prior research indicates that these variables are crucial determinants of Food Supply Chain Resilience (FSCR) (Ambulkar et al., 2015; Ahimbisibwe et al., 2016; Jouzdani & Govindan, 2021). FSCR, as a formative construct, integrates indicators from different dimensions. It creates variations in the model and establishes causal relationships between the exogenous variables SCPC, SCRC, and SCND, and the FSCR factor. SCPC is assessed through items such as flexibility, redundancy, integration, and efficiency (Pettit et al., 2013). SCRC is measured using items including agility, visibility, collaboration, and capacity management. SCND is evaluated based on differentiation, adaptability, sustainability, and responsiveness. Each set of four indicators represents a dimension of the respective variable's significance. These composite variables reflect independent indicators, which are essential for making informed decisions regarding resource adaptation, chain vulnerability management, strategic planning, and loss recovery in the food supply chain.
The second part of the model features a reflective pattern that evaluates the effects of Supply Chain Relational Practice (SCRP) and Food Supply Chain Resilience (FSCR) on Supply Chain Performance (SCP). Previous studies suggest that these variables contribute positively to supply chain performance (Chowdhury et al., 2023; Wieland & Wallenburg, 2013). For the SCRP exogenous variable, the items included are trust, communication, commitment, and cooperation, which are essential for strengthening relational ties among supply chain enterprises to enhance SCP. These four indicators effectively reflect the latent SCRP variable. The values for SCRP and FSCR, measured through the one-dimensional basic construct, provide a solid foundation for improving SCP, particularly in the food industry, where high standards of quality and safety are critical and supply-related risks are considerable.
The decision to construct a structural model incorporating both formative and reflective constructs, analysed using Partial Least Squares Structural Equation Modelling (PLS-SEM), is supported by a robust rationale. This rationale is based on the nature of the variables, indicators, and research objectives. Previous studies have addressed mixed statistical modelling, incorporating both reflective and formative models (Chowdhury & Quaddus, 2017; Jarvis et al., 2003; MacKenzie et al., 2011). Additionally, the PLS-SEM approach is suitable for both types of models (Henseler et al., 2014; Ringle et al., 2009). Building on these studies, a new multidimensional, hierarchical, mixed reflective-formative model was developed focusing on the correlation between FSCR and SCP (see Fig. 1).
ResultsIn the proposed multidimensional model, the Partial Least Squares Structural Equation Modelling (PLS-SEM) method was employed to measure the effects of exogenous latent variables on endogenous ones, following the sequence established for the research hypotheses (Hair et al., 2019).
Formative modelThe validation of the multidimensional model includes both a formative and a reflective path model. Given the nature of the indicators, the PLS-SEM statistical modelling procedure was used, as it is well-suited for these types of models (Dijkstra, 2014). The formative path model can be seen in Figs. 1 and 2. Each formative factor (SCPC, SCRC, and SCND) in this model represents a composite variable reflecting various indicators, with each indicator representing a distinct dimension of the factor. In Fig. 2, the formative model displays measurement loadings for SCPC items ranging from 0.013 to 0.569. The standardized path weights for items SCRC_1 (Agility), SCRC_2 (Visibility), SCRC_3 (Collaboration), and SCRC_4 (Capacity Management) range from 0.215 to 0.541. For SCND, the weights of paths from items SCND_1 (Type of Produce), SCND_2 (Mode Formulation), SCND_3 (Sustainability), and SCND_4 (Solution Applied) vary between 0.035 and 0.609. Additionally, the measurement loadings for FSCR items (FSCR_1 and FSCR_2) are high, at 0.884 and 0.821, respectively. Although some indicator path loadings are below 0.5, their exclusion was not considered due to their relevance for content validity (Hair et al., 2014). Reliable loadings indicate whether the selected indicators are effective measures for each latent variable. These results suggest that the latent variables encompass trust indicators, which are relevant for the formative construct.
Since all 12 indicator variables fall within the range of 0 to 1, with some exceeding 0.5, the formative measurement model is deemed reliable (Hair et al., 2019). Path coefficients (β) for the inner model represent the connections between latent variables. The path from SCPC to FSCR shows the lowest coefficient (β = 0.154), indicating a positive effect of SCRC on FSCR. Conversely, the highest coefficient is found for the path from SCND to FSCR, with a positive effect (β = 0.415). These path coefficients approach 1, reflecting moderate to strong relationships (Henseler et al., 2014).
The fit of the formative model was also assessed using outer model loadings. The reliability coefficients for formative model items, shown in Table 2, are all above 0.60 (Henseler et al., 2014; Hair et al., 2019). To establish discriminant validity in the formative model, the Cross-loadings table was used. According to this table, each indicator variable should have a higher correlation with its own latent variable compared to other latent variables (Dijkstra, 2014). Table 2 reveals that for the SCND latent variable, the variance shared with its own indicators (0.804, 0.754, 0.828, 0.921) is greater than the variance shared with any other latent variables (ranging from 0.350 to 0.675). The loadings of SCPC indicators (SCPC_1 to SCPC_4) on the SCPC variable are high (0.824, 0.658, 0.894, 0.673), with low cross-loadings with other factors (ranging from 0.328 to 0.559). For the SCRC latent variable, the variance shared with its indicators (0.879, 0.766, 0.694, 0.636) is higher than with other latent variables (ranging from 0.327 to 0.632). A similar pattern is observed for FSCR, where its block's cross-loadings (0.880, 0.826) are significantly higher than with indicators of other variables (ranging from 0.317 to 0.649). The square root of the AVE for the FSCR latent variable is 0.854, meeting the condition for discriminant validity.
Results of the Cross loadings - formative model.
Where: Food Supply Chain Resilience (FSCR).
Supply Chain Network Design (SCND).
Supply Chain Proactive Capability (SCPC).
Supply Chain Reactive Capability (SCRC).
Source: Survey data processing by the authors.
Formative models often face potential multicollinearity at the structural level, where latent variables modelled as causes of an endogenous latent variable may exhibit multicollinearity. To address this, VIF coefficients were examined (Fig. 3). All structural VIF coefficients in the formative model range from 1.223 to 1.962, below the maximum allowable limit of 4.0 (Hair et al., 2019; Henseler et al., 2021). For FSCR, the VIF values for items FSCR_1 and FSCR_2 are both 1.268, indicating no multicollinearity. For SCPC, item SCPC_3 has the highest VIF (1.608) and SCPC_1 has the lowest (1.433). Since VIF values for SCPC items range from 1.433 to 1.608, multicollinearity is considered low. The SCRC variable also shows VIF values ranging from 1.223 (SCRC_3) to 1.512 (SCRC_1). The SCND variable exhibits moderate VIF values (ranging from 1.765 to 2.088), but these values are not high enough to indicate serious multicollinearity issues.
The standardized root means square residual (SRMR) obtained was 0.05, indicating a good fit for the formative model, as SRMR is below the thresholds of 0.080 (Hu & Bentler, 1998) and 0.100 (Henseler et al., 2014).
Reflective modelOn the right side of Fig. 2, the reflective model is illustrated with causal arrows. In this model, indicators represent a set of elements that together reflect the latent variable they measure (Henseler et al., 2021). The standardized path weights connecting the latent variable Supply Chain Relational Practice (SCRP) to its indicators - SCRP_1 (Trust), SCRP_2 (Communication), SCRP_3 (Commitment), and SCRP_4 (Cooperation) - range from 0.731 to 0.810. For the Supply Chain Performance (SCP) variable, the indicators -SCP_1 (Learning and Growth Perspective), SCP_2 (Internal Business Process), SCP_3 (Customer Perspective), SCP_4 (Financial Perspective) - show values between 0.602 and 0.855. The reflective measurement model is deemed reliable, as most indicator loadings exceed the minimum acceptable threshold of 0.50. Reliable loadings and weights in PLS-SEM are crucial, as they provide valuable information about the relationships between variables. The significant weights for the indicators of each latent variable suggest that each indicator substantially contributes to the measurement of the latent construct (Hair et al., 2014). The high weights (close to 1) indicate that the indicators are appropriate and strongly reflect the latent variables.
The pathway coefficient from Food Supply Chain Resilience (FSCR) to SCRP (β = 0.645) has a stronger positive effect compared to that from SCRP to SCP (β = 0.620). Conversely, the path coefficient from FSCR to SCP (β = 0.220) reflects a positive effect that is closer in magnitude. Unlike the formative model, the reflective model allows for testing convergent validity using two indicators: Cronbach's Alpha (CA) and Composite Reliability (CR).
In Table 3, Cronbach's Alpha values for the three analysed variables range from 0.630 to 0.823. Since these values exceed the 0.60 threshold typically accepted in exploratory marketing research (Chin, 2010), the latent variables demonstrate convergent validity and, consequently, reliability. However, some researchers argue that Cronbach's Alpha may underestimate reliability (Daskalakis & Mantas, 2008; Hair et al., 2014). To address this concern, Composite Reliability (CR) indicators were calculated as an alternative to Cronbach's Alpha. The CR values range from a minimum of 0.646 to a maximum of 0.825, surpassing the 0.60 threshold allowed for similar research and confirming the reliability of the reflective model (Chin, 2010; Höck et al., 2010).
Results of the construct Reliability and Validity – reflective model.
Variables | Composite Reliability (CR) | Cronbach's Alpha (CA) | Average Variance Extracted (AVE) |
---|---|---|---|
FSCR | 0.646 | 0.630 | 0.728 |
SCRP | 0.825 | 0.823 | 0.655 |
SCP | 0.755 | 0.758 | 0.587 |
Where: Food Supply Chain Resilience (FSCR).
Supply Chain Relational Practice (SCRP).
Supply Chain Performance (SCP).
Source: Survey data processing by the authors.
Table 3 shows that the Average Variance Extracted (AVE) for each of the three latent variables ranges from 0.587 to 0.728 (> 0.50), demonstrating both convergent and divergent validity of the reflective model (Fornell & Larcker, 1981). Table 4 indicates that the square root of the AVE for FSCR is higher than the variance shared with SCP and SCRP (0.853 > 0.620; 0.853 > 0.645). A similar pattern is observed for the other variables (SCP and SCRP), confirming that the reflective model meets the condition for discriminant validity (Haverila et al., 2021; Ringle & Sarstedt, 2016).
The multicollinearity of the reflective model is assessed by the Variance Inflation Factor (VIF) indicators, which range from 1.101 to 2.569 (see Fig. 4). These values fall within the acceptable range of 0.25 to 4.0 (Hair et al., 2019; Henseler et al., 2021). For SCRP, half of the items have VIF values exceeding 2 (SCRP_2 = 2.116; SCRP_3 = 2.022), while the other half have lower values (SCRP_1 = 1.405; SCRP_4 = 1.836). Although some items show higher VIF values, the overall multicollinearity remains low, and no issues are indicated. The VIF values for SCP items range from a minimum of 1.101 (SCP_1) to a maximum of 2.569, suggesting low multicollinearity and no significant problems.
With an SRMR of 0.076 (0.076 < 0.080), the reflective model demonstrates a good fit (Hu & Bentler, 1998). This good fit is further supported by the Goodness of Fit (GoF) index, which is 0.590, indicating that the geometric mean of the average communality for the outer model and the average R-squared for the inner model approaches 1 (Hair et al., 2019).
Structural modelIn the context of the proposed multidimensional model, a higher R-squared (R²) value indicates a more substantial overall effect (Chin, 2010; Höck et al., 2010). The R-squared value reveals that 56.9 % of the variation in the endogenous variable Food Supply Chain Resilience (FSCR) is explained by the exogenous latent variables Supply Chain Proactive Capability (SCPC), Supply Chain Reactive Capability (SCRC), and Supply Chain Network Design (SCND) (formative model). Additionally, the factors Supply Chain Relational Practice (SCRP) and FSCR account for 60.8 % of the variation in the endogenous variable Supply Chain Performance (SCP). Both values exceed 0, indicating that the proposed multidimensional model has substantial relevance for predicting endogenous factors.
In SEM-PLS modelling, the application of t-tests for significance is crucial for assessing the overall fit of the structural model and determining whether the proposed hypotheses are supported by the data. A relationship between latent variables is considered significant if the t-value is greater than 1.96, suggesting that the construct model effectively explains the variance in the data (Hair et al., 2014). The p-value is a fundamental statistical measure used to evaluate the significance of a statistical test, such as the t-test in structural equation modelling (SEM). P-values less than 0.05 (Becker et al., 2015; Höck et al., 2010) or even 0.001 (Rigdon, 2010; Schloderer et al., 2014) indicate a significant influence between the analysed latent variables. A small p-value (<0.000 or <0.50) provides evidence supporting the existence and direction of relationships between latent variables.
In the formative model, the pathways are significant for the correlations between SCPC → FSCR (t-Value = 2.619 > 1.96), SCRC → FSCR (t-Value = 4.987 > 1.96), and SCND → FSCR (t-Value = 7.179 > 1.96) (see Table 5). The correlation between SCPC and FSCR (p-value = 0.009) indicates a probability level below the 0.05 threshold. Meanwhile, the correlations between SCRC → FSCR (p-value = 0.000) and SCND → FSCR (p-value = 0.000) register probability levels lower than 0.001 (Rigdon, 2010; Schloderer et al., 2014). These results suggest a direct and positive relationship: as SCPC, SCRC, and SCND improve, FSCR tends to increase. The significant and positive correlations among SCPC → FSCR, SCRC → FSCR, and SCND → FSCR support the statistical hypotheses of the study (H1, H2, and H3).
Correlation results.
Where: Food Supply Chain Resilience (FSCR).
Supply Chain Network Design (SCND).
Supply Chain Proactive Capability (SCPC).
Supply Chain Reactive Capability (SCRC).
Supply Chain Relational Practice (SCRP).
Supply Chain Performance (SCP).
Source: Survey data processing by the authors.
In the reflective model, significant paths are observed between the correlations of FSCR → SCRP (t-Value = 18.603 > 1.96), FSCR → SCP (t-Value = 4.102 > 1.96), and SCRP → SCP (t-Value = 12.400 > 1.96). P-values lower than 0.001 were recorded for the paths FSCR → SCP (p-value = 0.000), FSCR → SCRP (p-value = 0.000), and SCRP → SCP (p-value = 0.000), confirming their positive and significant nature (Rigdon, 2010; Schloderer et al., 2014). Consequently, enhancing relational practices and increasing resilience directly contribute to improving food supply chain performance. These results indicate significant and positive correlations between FSCR → SCRP, FSCR → SCP, and SCRP → SCP, which support the null hypotheses H4, H5, and H6 and align with the research objectives, as with the findings from the formative model.
DiscussionBased on Dynamic Capabilities View (DCV) theory, this study investigates the impact of relational practices and resilience on the performance of Romanian enterprises in the food supply chain during crisis conditions. The empirical results show the following.
SCPC had a moderate, positive, and significant influence on FSCR (β = 0.154), consistent with the findings of Melnyk et al. (2014) and Chowdhury et al. (2019). This reflects a firm's ability to anticipate and prepare for potential disruptions before they occur. Unlike previous studies (Gurrala & Hariga, 2022; Tarigan et al., 2021), our research employed a diverse set of formative indicators to measure SCPC, aiming to better capture the dimensions of proactive strategies. Flexibility indicates the firm's capability to adapt quickly to new changes, while redundancy involves having additional capacities or resources to address unexpected issues. Integration emphasizes the coordination of various supply chain functions, and efficiency pertains to process optimization. The combination of these indicators provides a comprehensive measure of SCPC, highlighting its role in enhancing resilience and overall performance during crises.
SCRC had a strong, positive, and significant impact on FSCR (β = 0.287), aligning with the research of Winter & Knemeyer (2013). With SCRC, an enterprise is better equipped to respond to and manage disruptions effectively. Agility enables firms to react swiftly to changes, visibility provides real-time information about the supply chain, collaboration enhances coordination with partners, and capacity management ensures efficient resource allocation during crises. Similar results regarding visibility and collaboration were demonstrated by the studies of Ahimbisibwe et al. (2016) and Nha Trang et al. (2022). The combined contribution of these indicators is vital for understanding how a company's reactive capabilities support operational continuity and achieve performance in challenging situations.
The effect of SCND on FSCR was the strongest and most significant (β = 0.415), compared to the first formative variables of the proposed construct model. Differentiation involves tailoring the supply chain to meet diverse needs, adaptability ensures that the network can adjust to changes, sustainability addresses long-term viability, and responsiveness refers to the network's ability to react to demands. Our study demonstrated that the combined action of these four diverse formative items - differentiation, adaptability, sustainability, responsiveness - directly contributes to ensuring the optimal strategic configuration of the food supply chain network, supporting the enterprise's resilience and performance. Other studies offer similar results, although the items were analyzed separately at the level of food supply chains (Jouzdani and Govindan 2021; Rakhra et al. 2022; Rao et al. 2021).
FSCR is an endogenous variable with critical outcomes that reflect the overall capacity of the food supply chain to withstand and recover from disruptions. Our study demonstrated that 56.9 % of the variation in food supply chain resilience was explained by the strong influence of the formative variables SCND and SCRC, correlated with the moderate influence of the SCPC factor. As a reflective variable, FSCR captures the cumulative impact of various resilience indicators. Key dimensions such as the ability to withstand disruptions, recovery speed, adaptability, and redundancy indicate how well the food supply chain can maintain operations and performance under crisis conditions. The analysis of these items has been the subject of studies by Wieland and Durach (2021) and Alikhani et al. (2021). The research findings reveal that FSCR has a strong, positive, and significant impact on SCRP (β = 0.645), whereas its effect on SCP is three times smaller (β = 0.220). Unlike previous studies (Chowdhury et al., 2019; Chowdhury et al., 2023), this study demonstrates the significant effect of FSCR on SCRP.
SCRP had a strong, positive, and significant influence on SCP (β = 0.620), aligning with previous research (Chowdhury et al., 2023; Srinivasan et al., 2011). By improving SCRP, as the relational dynamics among supply chain partners, efficient collaboration and enterprise performance are facilitated. Trust, communication, commitment, and cooperation are essential for building strong relationships that enhance coordination and problem-solving capabilities. These reflective indicators provide insight into how relational practices influence the performance of the food supply chain. Additionally, approximately 41.6 % of the variation in the endogenous variable SCRP was explained by the specific action of the FSCR variable.
The combined action of FSCR and SCRP explains 60.8 % of the variation in the latent variable SCP, which measures the effectiveness and efficiency of the food supply chain from multiple perspectives. Previous studies have shown that FSCR significantly improves SCP (Brusset & Teller, 2017; Chowdhury et al., 2023). Additionally, other studies indicate that SCP can be further enhanced by the intangible resources included in SCRP. Our study shows that the learning and growth perspective evaluates capacity development, the internal business process perspective focuses on operational efficiency, the customer perspective assesses satisfaction and service quality, and the financial perspective examines economic performance. By analysing these diverse items, the study provides a more comprehensive view of how to improve supply chain performance.
Theoretical and practical implicationsTheoretical implicationsThis study makes several contributions to the specialized literature concerning the impact of resilience and relational practice on food supply chain performance.
To fill the research gap regarding the lack of a comprehensive structural model for measuring SCP based on strong theoretical foundations and the influence of the three variables mentioned above, we developed and validated a formative-reflective multidimensional mixed model. Given that resilience is a dynamic capability, our study first proposes the integration of a formative construct in the initial part of the multidimensional model, adopting a distinctly different approach compared to other studies (Siagian et al., 2021; Chowdhury et al., 2023), for measuring FSCR. Second, it extends enterprise resilience along the food supply chain and related processes by including distinct latent variables that contribute to sustaining enterprise performance under disruptions or turbulence in crisis-affected markets. Measuring SCPC and SCRC variables addresses the managers' lack of knowledge about resource specificity, dynamic capability measurement, and the building process to better manage supply chain information and relationships, addressing disruption challenges and negative environmental threats more easily, and recovering from post-crisis losses. Rising unemployment, declining incomes, changing consumption patterns in the food sector, and intensifying consumption panic behaviours are among the factors that significantly influence both business activity and the entire food supply chain.
Therefore, our study extends the existing literature (Chowdhury et al., 2023; Jüttner & Maklan, 2011; Siagian et al., 2021; Tarigan et al., 2021; Wieland, 2021) and proposes that enterprises along the entire supply chain develop various proactive and reactive forms of dynamic capabilities to remain efficient and effective in target food markets, coping with the many disruptions and turbulences generated by crises.
Moreover, food supply chains need to develop proactive capacity to recover business and operational costs, becoming flexible by developing contingency plans that prevent slowdowns and overcome blockages, integrating information and material flows, and becoming efficient by increasing productivity. On the other hand, by designing a reactive supply chain capacity, enterprises need to improve their agility, visibility, enhance collaboration, and improve human resources management.
Third, our study extends the resilience of enterprises along the food supply chain by incorporating the latent variable SCND into the formative model of FSCR and, implicitly, extending the integrated theory of dynamic capabilities (DCV). Thus, our study complements Jouzdani and Govindan (2021) by incorporating a new latent variable, thereby revealing the significant contribution of this variable to food supply chain resilience.
By proposing the inclusion of strategic planning (materialized by identifying optimal locations and proper sizing of units in the network, as the third variable of the formative model) it extends the scope of the theory of dynamic capability from the enterprise's frontier to the entire food supply chain. The validity and robustness of the formative model are demonstrated by the action of the three exogenous (independent) variables SCPC, SCRC, and SCND which explain 62.8 % of the variation of the endogenous (dependent) FSCR factor. The PLS-SEM results indicate that the hypotheses H1, H2, and H3 are valid. The strongest impact was demonstrated by the exogenous variable SCND (SCND → FSCR; β = 0.415; t-Value = 7.179 > 1.96; p-Value = 0.000), followed by the SCRC factor (SCRC → FSCR; β = 0.287; t-Value = 4.987 > 1.96; p-Value = 0.000). A significant, slightly more moderate impact was proved by the first latent variable SCPC (SCPC → FSCR; β = 0.154; t-Value = 2.619 > 1.96; p-Value = 0.009 < 0.05). Therefore, the size of the correlation pathways of the exogenous variables (SCPC, SCRC, and SCND) demonstrates their positive, significant, and strong impact on FSCR.
Fourth, by integrating the reflective construct into the second part of the multidimensional model, our study assessed the measure of the endogenous variable SCP based on the joint influence of two latent variables FSCR and SCRP. Thus, processing all variables, we developed a comprehensive mixed formative-reflective multidimensional model for measuring SCP, which is novel in the existing SCP literature. Some studies have analysed the SCP measure through some processes and developed distinct models (such as SCOR, ASLOG, Balanced Scorecard, etc.) to help businesses throughout the supply chain improve their performance (Akif et al., 2005; Akkucuk, 2020; Hausman, 2004).
Our study extends the work of Wieland and Wallenburg (2013) and Chowdhury et al. (2023) and contributes to the evaluation of the SCP model by highlighting the importance of increasing relational ties between companies operating within the supply chain, especially in an uncertain environment, also amplified by the effects caused by the COVID-19 pandemic.
Finally, this study investigated the effect of SCRP on FSCR and SCP. Previous works (Aslam et al., 2021; Chowdhury et al., 2023; Srinivasan et al., 2011; ) highlighted the contribution of SCRP to improving supply chain resilience and operational performance, but the authors did not provide any empirical evidence. We addressed this gap in the literature by identifying new items and evaluating the impact of the SCRP latent variable on resilience and on the performance of the food supply chain in the context of the crisis. Our findings show that in addition to resilience, the performance of supply chains should be enhanced by improving trust along the supply chain, by better communicating information with partners and using sharing tools, by committing to developing sustainable relationships with chain members, and by achieving cooperation to solve problems and eliminate unfair advantages.
The variation of the endogenous SCP variable of 60.8 % is explained by the action of the SCRP and FSCR factors, demonstrating the validity of the reflective model. The last four hypotheses of the study are confirmed by the data obtained after the PLS-SEM analysis (H4-H6). Analysing the size of the SCRP correlation path, we observe that this variable has a significant and strong effect on SCP (H6; SCRP → SCP; β = 0.620; t-Value = 12.400 > 1.96; p-Value = 0.000). At the level of the researched population, FSCR exerted a strong and significant impact on the SCP variable, therefore the null hypothesis H5 was accepted for a β of 0.220 and a t-value of 4.102 (> 1.96). The reflective model indicates the highest positive correlation for the FSCR → SCRP pathway (H4; β = 0.645; t-Value = 18.603 > 1.96; p-value = 0.000). Both the SRMR measures (0.050 for the formative model, and 0.076 for the reflective model) and the value of the Goodness of Fit indicator (GoF = 0.590) justified that the multidimensional SCP model proves a good match with the set of selected variables. Using the DCV theory as the foundation for FSCR and the Balanced Scorecard model as the basis for measuring SCP, this study makes a significant contribution to the FSCR and SCP specialized literature in general, and, in particular, to the food supply chain management specialized literature.
Practical implicationsThe research findings are valuable for Romanian food supply chain enterprises and can serve as a useful reference for entities in other countries. To recover activities and operational costs after successive interruptions caused by environmental factors and crises, managers adopted proactive approaches, indicating high flexibility among them. The study results indicate a high flexibility (SCPC_1) among managers. They developed contingency plans to support production, used platforms to increase orders, and sought solutions to introduce healthy products. By creating redundancies (SCPC_2), the enterprises found new alternatives that allowed them to continue their activity, overcoming uncertain situations and blockages by creating buffer/reserve stocks in case of delivery errors and/or losses, expanding spare capacities and utilities, and extending collaboration with new food suppliers. Sharing databases and information with supply chain partners, ensuring information flows between different departments, and developing joint collaboration plans for integrating healthy products stimulated the integration (SCPC_3) of enterprises in the network. At the same time, the efficiency (SCPC_4) of enterprises increased due to rigorous quality control processes, increasing customer satisfaction by delivering healthy/quality products, improving employee performance in terms of quality, working speed, and productivity, creating opportunities for training and skill development, and obtaining new job satisfactions.
Analysing proactive capabilities provides supply chain businesses with the opportunity to improve risk assessment and mitigation, contingency planning, and increase product integration and efficiency. For example, managers could identify potential food safety and supply chain vulnerability risks and develop appropriate strategies to mitigate them, including supplier diversification, strategic stockpiling, and contingency planning. Contingency plans will support production by including predefined actions, communication strategies, and clear roles and responsibilities. By investing in technology and data analysis, information managers can monitor and anticipate supply chain disruptions, detect potential problems in real time, integrate healthy products, and increase customer satisfaction. Overall, by applying proactive capability, managers can make the best decisions to enhance food supply chain performance.
In addition to proactive approaches, food supply chain managers have implemented reactive measures. By developing agile management (SCRC_1), enterprises have strengthened and streamlined the communication system with customers, suppliers, and third parties, identified new customer segments, and collaborated with suppliers offering significant discounts on food purchases. Many enterprises improved visibility (SCRC_2) through inventory management platforms, ensuring quick visualization and fulfilment of strict customer requests. Managers have intensified collaboration (SCRC_3) between enterprises to increase process integration, ensuring information exchange, relationship management, and technology transfer; rapidly implementing technologies such as IoT and cloud computing for more efficient operations management, or extending supplier certification to enhance food safety and strengthen total quality management systems. At the same time, specific strategies for capacity management (SCRC_4) applied by managers aimed at replanning activities and adapting business infrastructure to environmental changes, checking and adjusting planning parameters, and recommending resource reallocation and loss recovery.
Analysing reactive capacity can improve communication, collaboration, and crisis management. By implementing capture and communication tools such as IoT devices, cloud computing, real-time tracking systems, and digital communication platforms, managers can communicate and collaborate in real time with all microenvironment factors. Investments in supply chain visibility technologies allow managers to adjust their offerings and efficiently manage inventory based on demand fluctuations. By fostering collaborative relationships with suppliers, food supply chain managers can identify alternative supply options, expedite deliveries, or adjust production schedules. Crisis management implementation obliges enterprises to establish a crisis management team and develop a response plan, including clear communication strategies, alternative supply options, resource reallocation options, production replanning, and loss recovery measures.
To reduce vulnerabilities, managers have enhanced their knowledge and technology to effectively design food supply chain networks. Enterprises that have chosen differentiation (SCND_1) as a competitive advantage have applied sustainable strategies, addressed inventory routing issues related to perishable food, maintained food quality and safety, and reduced food costs and/or losses. The adaptability (SCNC_2) of enterprises has increased, with managers redesigning networks based on changing food transport volumes and modifying transport routes due to COVID-19 pandemic traffic restrictions. Sustainability actions (SCNC_3) undertaken by managers aimed at optimizing chain node locations and efficiently using available resources without harming the environment or compromising the ability to meet future needs. The study results highlight that managers have dedicated significant time to digitalization and developed greater responsiveness (SCND_4) regarding the use of sophisticated software, which helps them make optimal decisions for network unit management and respond promptly to customer requests or market changes.
Results from chain design analysis will prompt companies to focus more on differentiation, redundancy, nearshoring, and adopting new digital technologies. In designing supply chain networks, managers can consider supply options, position distribution centres in strategic locations, adopt redundant transport routes, and ensure food quality and safety. These measures enhance operational continuity during disruptions. For perishable food products, managers can place production units closer to final markets, reducing transport risks, optimizing resource use, and ensuring faster deliveries. Optimizing food supply chain node locations, analysing product flows and data, conducting market research, selecting transport modes and locations strategically, and implementing efficient stock management systems contribute to improving enterprise efficiency, responsiveness, and reducing environmental impact. This involves adjusting operations based on performance parameters like production times, costs, and logistics efficiency.
Managers have taken a proactive approach to food supply chain resilience, adapting plans and strategies to changes, and reactive measures by reevaluating programs to recover losses and network design, supported by strategic planning and efficient resource management in available units. To improve the food supply chain, managers agree they must apply smart solutions to ensure traceability, reputation, and trust throughout the supply chain (SCRP_1), ensure optimal communication through accurate information exchange with chain businesses and quick information sharing tools (SCRP_2), commit to responsible practices and sustainable relationships with chain members (SCRP_3), and cooperate to solve problems and eliminate unfair advantages (SCRP_4).
To successfully apply these directions in food sector supply chain management, managers should formulate new strategies and actions related to relational practices. Some strategies could target implementing smart technologies (IoT or RFID tagging solutions) to better track food products throughout the supply chain, ensuring product authenticity and safety. Integrating blockchain technology could increase product transparency and security, allowing transaction monitoring and product status changes. To build trust, chain managers could ensure product compliance with all relevant food safety and quality certifications and standards and communicate this to customers. Businesses could implement information management systems to centralize, organize, and share supply chain data with partners. Effective communication with all supply chain stakeholders could be achieved through platforms such as supplier portals, interactive communication systems, or mobile applications. Managers may adopt resource management and social responsibility practices to demonstrate commitment to corporate sustainability. Collaborating with industry organizations, food associations, and local or regional governments, businesses can eliminate unfair advantages, respect fair competition rules, and promote fair trade.
The study's valid hypotheses certify that FSCR and SCRP are factors with significant and strong influences on SCP, contributing to the success of all enterprises. Part of the investments in enterprise performance enhancement will focus on intensifying learning and growth actions (SCP_1), such as increasing staff expertise, organizing and classifying skills, staff qualification and professional development, embracing telecommuting, attracting talent, improving successful leadership thinking, maintaining active social media presence, sharing knowledge on platforms like Quora, and creating and maintaining a food blog. Other investments involve developing internal business processes (SCP_2) by reducing marketing and operations costs, entering new markets, channels, or products, merging or concluding new transactions with long-term positive impacts, and using recent innovative technologies to facilitate interdepartmental knowledge exchange. Managers agree that enterprise performance can be ensured by improving the personalized customer experience (SCP_3), implementing loyalty programs, increasing industry leader awareness, improving brand awareness, using e-books specific to the food industry for promotion, and cantering brand image around social and environmental improvements. Financial measures (SCP_4) aimed at reducing operational costs and increasing profits or revenues in target markets also contribute.
Therefore, the proposed model offers strategic alternatives for businesses throughout the food supply chain to overcome market vulnerabilities and integrate sustainable practices across the system.
Limitations and future researchOur study presents several limitations that merit discussion. Firstly, the research investigates the direct effects of Supply Chain Proactive Capabilities (SCPC), Supply Chain Reactive Capabilities (SCRC), and Supply Chain Network Design (SCND) on Food Supply Chain Resilience (FSCR), while the second phase explores the influence of Supply Chain Relational Practices (SCRP) and FSCR factors on Supply Chain Performance (SCP). Future studies should develop structural models incorporating new latent variables, such as ethical, responsible, or legislative supply chains, to reduce the vulnerability of the food supply chain in the face of environmental changes and crises and to enhance its performance.
Moreover, the marketing research was conducted within the food industry, and although a representative sample of enterprises was selected, the potential influence of Romanian cultural bias cannot be overlooked, as reflected in the continuity and unity over time and space (Mihut & Lungescu, 2006). Extending the applicability of the multidimensional model beyond the Romanian food industry and into different sectors of other countries' economies would strengthen its credibility.
Building on our research results, future studies could also focus on assessing the digital or sustainable impact of the supply chain on performance during interruptions, particularly in the context of recent global changes. An important limitation considered by the authors is the omission of the consequences/implications of two major global events: the war in Ukraine and the conflict in Israel. The authors believe that future research is necessary to investigate the economic effects resulting from both global events.
ConclusionThe study found that the proactive capabilities of enterprises, such as developing contingency plans, creating redundancy, and improving integration and efficiency, significantly enhance their ability to adapt and respond to disruptions, leading to improved overall supply chain performance. Reactive measures, including agile management, enhanced visibility, and intensified collaboration, enable enterprises to effectively manage crises and disruptions by strengthening communication with customers, suppliers, and partners, thereby maintaining operational continuity. The incorporation of Supply Chain Network Design (SCND) significantly contributes to food supply chain resilience by ensuring adaptability, sustainability, and responsiveness, which are essential for maintaining operational efficiency and meeting diverse needs during crises.
The study highlights the importance of relational practices (SCRP), such as trust-building, effective communication, and collaboration, in enhancing supply chain resilience and performance, particularly in an uncertain environment amplified by crises like the COVID-19 pandemic. By integrating the Dynamic Capabilities View (DCV) theory with the Balanced Scorecard model, the study presents a comprehensive multidimensional model that effectively measures supply chain performance, highlighting the interplay between resilience, relational practices, and performance outcomes. The study underscores the need for future research to incorporate additional latent variables, such as ethical, responsible, and legislative supply chains, to further enhance supply chain performance and resilience.
CRediT authorship contribution statementMirela Cătălina Türkeș: Writing – review & editing, Writing – original draft, Visualization, Validation, Supervision, Software, Resources, Project administration, Methodology, Investigation, Funding acquisition, Formal analysis, Data curation, Conceptualization. Aurelia Felicia Stăncioiu: Writing – review & editing, Writing – original draft, Visualization, Validation, Supervision, Software, Resources, Project administration, Methodology, Investigation, Funding acquisition, Formal analysis, Data curation, Conceptualization. Roxana-Cristina Marinescu: Writing – original draft.