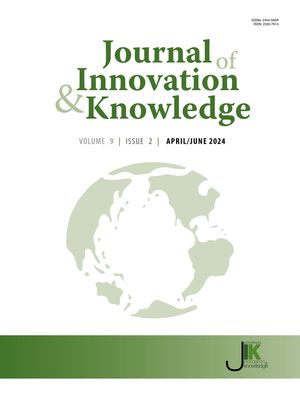
Edited by: Abbas Mardari
More infoUnder the new normal of global governance driven by innovation, competitiveness in science and technology has become a key indicator of a country's or a region's comprehensive strength. Science and technology innovation (STI) policy has become a significant instrument for governments to guide and advance science and technology competitiveness. STI policies do not exist independently, and interactions exist among policies; however, studies till date have not sufficiently investigated such interaction. Hence, this study analyzed the factorial effect of STI policies using multifactor analysis of variance. We discovered that there are significant interactions among STI policies and that a policy mix can produce some new properties not possessed by a single STI policy. We statistically identified these interactions and sorted the magnitude of policy effects. This study enriches and improves the existing research, thereby offering scholars and policymakers with a more comprehensive and in-depth understanding of the effects of the policy mix. Moreover, this study contributes to the scientific implementation of policies for improved STI and economic development.
Science and technology innovation (STI) can promote production factor quality and labor productivity. Moreover, STI is an important driver for maintaining sustainable and healthy economic development (Walsh et al., 2020). STI ability is a vital sign of comprehensive regional competitiveness and a decisive factor for obtaining international competitive advantage. Countries have increasingly focused on the role of STIs in national development, and the prominence of STI policy in governance is rising (Surana et al., 2020). STI policy is an essential tool for promoting STI; it is a crucial part of the national public policy, a necessary means to shape the innovation environment and stimulate innovation vitality, a fundamental guarantee for innovation-driven development, and a key element of national innovation systems. STI policy influences national innovation ability and national competitiveness (Peng et al., 2008).
STI research has received increasing attention (Soete, 2019; Naqshbandi & Jasimuddin, 2022), particularly regarding STI policy and its effect. STI policy has evolved from focusing on solving market and innovation system failures to a combination of transformation and reform and foresight and synergy (Schot & Steinmueller, 2018). Policy synergy refers to a combination of policy tools and cooperation between policies to improve policy effects (Flanagan et al., 2011); its core is the interaction among policies (Meissner & Kergroach, 2021). As an essential tool for shaping the innovation environment and stimulating innovation vitality, STI policy can express its role in environmental construction and innovation incentive primarily through a perfect and optimal policy system. Optimizing the STI policy system increasingly emphasizes the systematization of STI policy, and the multifactor analysis of variance (ANOVA) can effectively identify the interaction between policies.
This study explored the factorial effect in the STI policy mix wherein the main effect and interaction are collectively referred to as the factorial effect. Using the multifactor ANOVA to investigate the factorial effect, this study determines what interaction exists among STI policies, if all main effects and interactions are significant, and if all main effects are greater than interactions.
Theoretical frameworkMarket failure theory states that the rationality of government intervention in technological innovation lies in “market failure” (Che et al., 2022), which refers to the inequality between the marginal value of technological innovation activities for the entire society and the private sector, resulting in the nonoptimal allocation of resources in the free market. Governments should intervene in the field of market failure and close the gap between social and private benefits through investment, subsidies, and intellectual property protection to rectify market failure (Dodgson et al., 2011; Wang et al., 2020a). Schumpeter's innovation theory notes that technological innovation is crucial, playing a core role in economic growth. It is regarded as a complex process comprising the interaction of science, technology, and the market (Lipieta & Lipieta, 2022). This theory also proposes that all links in the technological innovation process require policy support, emphasizing that policies aimed at promoting technological innovation are essential. The endogenous growth theory proposes three major forces driving economic growth: human capital, technological progress, and knowledge (Akcigit & Ates, 2021; Cauwels & Sornette, 2022). Romer (1990) believes that technological progress is decisive; thus, the policies and measures for promoting technological progress are vital. These policies and measures include investing in education and improving the human capital stock of research and development (R&D).
Study gap and contributionAs mentioned above, STI policy has transitioned from focusing on solving market and innovation system failures to a combination of transformation and reform and foresight and synergy (Schot & Steinmueller, 2018). Policy synergy here refers to a combination of policy tools and cooperation among policies to achieve better policy effects (Flanagan et al., 2011); the interaction among policies is at the core (Meissner & Kergroach, 2021). Most studies have focused on analyzing the implementation effect of a single policy; however, further analysis is required on the effect of the STI policy mix, especially the interaction among policies. This interaction needs to be immediately addressed in the current regional policy research (Meissner & Kergroach, 2021). Our study focuses on this interaction using the multifactor ANOVA to investigate the factorial effects of STI policies.
STI is the foundation of sustainable economic and social development (Xu et al., 2022). The realization of innovation-driven development is inseparable from supporting scientific and technological innovation policies (Zhu et al., 2022). First, this study aims to enrich and improve the existing research. Second, it offers scholars and policymakers with a more comprehensive and in-depth understanding of the effects of the policy mix. Moreover, it can contribute to the scientific implementation of policies while promoting STI and economic development. This study's primary significance, innovation, and contribution are as follows. First, the study addresses urgent problems to be solved in the current regional policy research by employing the multifactor ANOVA to examine the factorial effects of STI policies, thereby supplementing existing research. Second, the quantitative analysis method is used to examine these factorial effects, which, in turn, helps equip scholars and policymakers with a better understanding of STI policies for improved scientific implementation. Finally, STI policy can guide the development of STI practices in a country or a region, and the corresponding research can provide guidance for the cause of STIs.
This paper is structured as follows. Section 2 provides a review of relevant literature. Section 3 describes the methods employed in this study, especially the three-way ANOVA method in multifactor ANOVA. Section 4 presents the analysis of the factorial effect of the STI policy mix, detailing the process and results of the analysis. Section 5 discusses the results and policy implications, and Section 6 summarizes the conclusion and highlights the scope for future research.
Literature reviewThe concept of STI policy originates from the gradual development and expansion of economic policy, environmental policy, and other fields (OECD, 2010; Flanagan et al., 2011). In the field of STI policy research, existing literature primarily focuses on analyzing the implementation effect of a single policy (Dou et al., 2019). For instance, Alvarez-Ayuso et al. (2018), Cappelen et al. (2012), and Mukherjee et al. (2017) studied the implementation effect of tax policy on STIs. Li et al. (2019) studied the impact of tax policy on STI and noted that the impact was positive; Pan & Lou (2021) deduced that tax preference can actively promote STI; Xu (2021) studied the intermediary effect between R&D subsidy policy and innovation performance. Ortigueira-Sánchez et al. (2022) deduced that government subsidies could promote innovation, whereas Lu et al. (2014) reported that government subsidies could help alleviate enterprise financing constraints, disperse enterprise technological innovation investment risks, reduce marginal enterprise costs, and boost enterprise R&D investment. Xu (2015) found that R&D subsidies could effectively promote enterprise R&D investment, whereas Guerzoni & Raiteri (2015) and Aschhoff & Sofka (2009), Song & Zhang (2014), and Hu et al. (2013) examined the impact of government procurement on STIs. Through an empirical study, Deng et al. (2018) discovered that government procurement promoted enterprise technological innovation by alleviating financing constraints. Finally, Lyu et al. (2022) empirically analyzed the impact of social capital on innovation.
Although the above studies focused on analyzing a single STI policy's implementation effect, it sets a foundation for STI policy mix research, which is gaining popularity in the STI policy community (Meissner & Kergroach, 2021; Kern et al., 2019). In this domain, Kalcheva et al. (2018) noted that the interaction between supply and demand policies could promote innovation. Empirically, Wu et al. (2021) analyzed the impact of the R&D subsidy policy mix on innovation, whereas Wang et al. (2020) analyzed the impact of the STI policy mix on innovation efficiency. Reichardt & Rogge (2016) investigated the effect of policy mix on innovation and deduced that policy mix was crucial for technological innovation. Guo et al. (2019) noted that the STI policy mix could play a better role on innovation, and Dou et al. (2019) noted that it promoted enterprise technological innovation.
Although the above studies carefully analyzed the STI policy mix, further studies are required to analyze its effect (Schmidt & Sewerin, 2019). In particular, STI policies do not exist independently, and interactions exist among policies. For example, results tend to be biased when assessing the role of a single policy on enterprise technological innovation without considering the role of other policies (Dumont, 2017). Furthermore, considering diversified policy objectives, decentralized governance structure, multilevel management, multiple market failures, and system failures, it is necessary to strengthen the combination of different policies to improve STI policies’ coordination (Kivimaa & Kern, 2016; Scordato et al., 2018), wherein the interaction among policies is the key factor (Meissner et al., 2021).
While extant literature agrees with the interaction among policies, there is no consensus on the definition of the STI policy mix (Rogge & Reichardt, 2016; Flanagan et al., 2011). A few representative definitions of the STI policy mix are as follows. According to the OECD (2010), the STI policy mix refers to the balance and interaction among policies. Flanagan et al. (2011) noted that the STI policy mix included the combination and interaction processes of policy tools. Rogge & Reichardt (2016) noted that the STI policy mix was a tool and means for achieving the interaction of goals in a dynamic environment. Additionally, Meissner & Kergroach (2021) referred to the STI policy mix as the balance and interaction among policies, with interaction at the core. These definitions implicitly or explicitly define the policy mix as a combination of policy tools in similar or different fields, emphasizing that the interaction among policies is the core of the policy mix.
Overall, in the field of STI policy research, the existing literature mainly focuses on analyzing the implementation effect of a single policy. Conversely, the effect of the STI policy mix needs to be analyzed more thoroughly, noting that the interaction among policies is the core feature of the policy mix.
Research methodMultifactor ANOVAANOVA is used to separate the impact of factor changes in the presence of random interference and then infer whether the changes significantly impact the research object. ANOVA is divided into one-way and multifactor ANOVA; the fundamental idea of multifactor ANOVA is consistent with that of one-way ANOVA. The difference is that the former analyzes roles and different levels of collocation of various factors on test indicators (DeGroot & Schervish, 2012; Bartoszy¿ski & Niewiadomska-Bugaj, 2007). When two or more factors affect the dependent variable, multifactor ANOVA uses variance comparison and hypothesis tests to determine whether each factor significantly impacts the dependent variable. In multifactor ANOVA, the impact of a factor on the dependent variable alone is termed the “main effect,” the combined impact produced by different factors is termed the “interactive effect,” and the specific state or quantity level of factors is termed “level.”
In multifactor ANOVA, the dependent variable is affected in three aspects. The first aspect is the independent effect of factors, i.e., the independent effect of a single factor on the dependent variable. The second aspect is the effect of factor interaction, i.e., the effect of multiple factors on dependent variables. The third is the effect of random factors, i.e., the effect introduced by sampling error. The following identity relationship exists in the multifactor ANOVA.
Sum of the squares of total errors = sum of the squares of errors caused only by the factors themselves + sum of the squares of errors caused by the interaction of factors + sum of the squares of random errors
This study used the three-way ANOVA as it aimed to analyze the impact of three factors on dependent variables. Thus, this paper primarily describes the three-way ANOVA method in multifactor ANOVA.
Assume that there are three factors, A, B, and C, and the number of their levels are A, B, and C, respectively. The sum of the squares of total errors is recorded as SST. The sum of the squares of errors caused by the independent action of factors A, B, and C are recorded as SSA, SSB, and SSC, respectively. The sum of the squares of errors caused by the interaction of factors A, B, and C are recorded as SSAB, SSAC, SSBC, and SSABC, respectively. The sum of the squares of random errors is recorded as SSE. Therefore, the identity relationship of the three-way ANOVA is as follows. SST = (SSA + SSB + SSC) + (SSAB + SSAC + SSBC + SSABC) + SSE
Assume that there are three factors, A, B, and C, with corresponding quantities of levels a, b, and c, respectively. Additionally, assume that r experiments are repeated for the combination of each level of factors A, B, and C, and n = a × b × c × r. Thus, the three-way ANOVA table can be obtained as Table 1.
Three-way ANOVA table.
This study aimed to explore the factorial effect of the STI policy mix and determine the interaction between STI policies and ascertain whether all main effects and interactions were significant. Therefore, the following hypotheses were tested.
Hypothesis 1: STI supply, demand, and environmental policies significantly impact annual gross domestic product (GDP) and annual patent application acceptance (APAA).
Hypothesis 2: There are significant interactions among STI supply, demand, and environmental policies.
Hypothesis 1 analyzes whether each policy has a significant effect, i.e., whether each policy can significantly affect GDP and APAA. Meanwhile, Hypothesis 2 analyzes whether there are significant interactions between supply and demand policies, supply and environmental policies, and demand and environmental policies. Thus, Hypothesis 2 analyzes the two-dimensional effect. Moreover, it is necessary to analyze the interaction effect among all three policies, i.e., the three-dimensional effect.
ResultsDescriptive statisticsAfter descriptive statistical analysis, certain basic information is obtained as shown in Table 2.
Descriptive statistical analysis of dependent variables.
Note: X1 represents supply policy, X2 represents demand policy, and X3 represents environmental policy. The three levels −1, 0, and 1 of X1 correspond to the three levels of supply policy. Likewise, the three levels −1, 0, and 1 of X2 correspond to the three levels of demand policy, and the three levels −1, 0, and 1 of X3 correspond to the three levels of environmental policy.
When using the multifactor ANOVA for research and analysis, it is necessary to test its basic assumptions (mainly the test of normality and homogeneity of variance) and then perform an effect analysis of factors (including the primary effect and interaction effect analyses).
Normality and homogeneityThe normality test results show that populations are normally distributed. Furthermore, when the significance level is 0.05, the significance (p-value) of the Shapiro–Wilke test is greater than 0.05, and the Kolmogorov–Smirnov test shows that most of the populations meet the normality requirements. Table 3 presents the details.
Normality test.
X1 | X2 | X3 | Kolmogorov–Smirnov | Shapiro–Wilk | |||||
---|---|---|---|---|---|---|---|---|---|
Statistic | df | Sig. | Statistic | df | Sig. | ||||
PCGDP | 0 | 0 | 0 | .048 | 50 | .200* | .989 | 50 | .918 |
1 | 0 | 0 | .051 | 50 | .200* | .981 | 50 | .602 | |
−1 | 0 | 0 | .049 | 50 | .200* | .993 | 50 | .988 | |
0 | 1 | 0 | .053 | 50 | .200* | .988 | 50 | .890 | |
1 | 1 | 0 | .053 | 50 | .200* | .980 | 50 | .568 | |
−1 | 1 | 0 | .048 | 50 | .200* | .992 | 50 | .985 | |
0 | −1 | 0 | .046 | 50 | .200* | .990 | 50 | .939 | |
1 | −1 | 0 | .050 | 50 | .200* | .982 | 50 | .629 | |
−1 | −1 | 0 | .052 | 50 | .200* | .993 | 50 | .990 | |
0 | 0 | 1 | .055 | 50 | .200* | .988 | 50 | .900 | |
1 | 0 | 1 | .051 | 50 | .200* | .981 | 50 | .579 | |
−1 | 0 | 1 | .052 | 50 | .200* | .992 | 50 | .986 | |
0 | 1 | 1 | .060 | 50 | .200* | .987 | 50 | .862 | |
1 | 1 | 1 | .057 | 50 | .200* | .980 | 50 | .542 | |
−1 | 1 | 1 | .057 | 50 | .200* | .992 | 50 | .983 | |
0 | −1 | 1 | .049 | 50 | .200* | .989 | 50 | .930 | |
1 | −1 | 1 | .050 | 50 | .200* | .981 | 50 | .615 | |
−1 | −1 | 1 | .054 | 50 | .200* | .993 | 50 | .988 | |
0 | 0 | −1 | .045 | 50 | .200* | .990 | 50 | .937 | |
1 | 0 | −1 | .054 | 50 | .200* | .981 | 50 | .608 | |
−1 | 0 | −1 | .055 | 50 | .200* | .993 | 50 | .988 | |
0 | 1 | −1 | .049 | 50 | .200* | .989 | 50 | .919 | |
1 | 1 | −1 | .056 | 50 | .200* | .980 | 50 | .573 | |
−1 | 1 | −1 | .050 | 50 | .200* | .993 | 50 | .988 | |
0 | −1 | −1 | .046 | 50 | .200* | .990 | 50 | .949 | |
1 | −1 | −1 | .052 | 50 | .200* | .982 | 50 | .644 | |
−1 | −1 | −1 | .051 | 50 | .200* | .993 | 50 | .990 | |
APAA | 0 | 0 | 0 | .080 | 50 | .200* | .983 | 50 | .699 |
1 | 0 | 0 | .057 | 50 | .200* | .986 | 50 | .822 | |
−1 | 0 | 0 | .128 | 50 | .039 | .973 | 50 | .308 | |
0 | 1 | 0 | .079 | 50 | .200* | .984 | 50 | .743 | |
1 | 1 | 0 | .063 | 50 | .200* | .987 | 50 | .835 | |
−1 | 1 | 0 | .123 | 50 | .056 | .974 | 50 | .338 | |
0 | −1 | 0 | .080 | 50 | .200* | .982 | 50 | .641 | |
1 | −1 | 0 | .057 | 50 | .200* | .986 | 50 | .804 | |
−1 | −1 | 0 | .123 | 50 | .057 | .972 | 50 | .283 | |
0 | 0 | 1 | .079 | 50 | .200* | .985 | 50 | .766 | |
1 | 0 | 1 | .059 | 50 | .200* | .987 | 50 | .851 | |
−1 | 0 | 1 | .110 | 50 | .177 | .975 | 50 | .349 | |
0 | 1 | 1 | .080 | 50 | .200* | .986 | 50 | .823 | |
1 | 1 | 1 | .063 | 50 | .200* | .987 | 50 | .853 | |
−1 | 1 | 1 | .105 | 50 | .200* | .976 | 50 | .399 | |
0 | −1 | 1 | .084 | 50 | .200* | .984 | 50 | .712 | |
1 | −1 | 1 | .060 | 50 | .200* | .987 | 50 | .847 | |
−1 | −1 | 1 | .116 | 50 | .092 | .973 | 50 | .303 | |
0 | 0 | −1 | .083 | 50 | .200* | .981 | 50 | .586 | |
1 | 0 | −1 | .062 | 50 | .200* | .985 | 50 | .783 | |
−1 | 0 | −1 | .121 | 50 | .064 | .973 | 50 | .293 | |
0 | 1 | −1 | .080 | 50 | .200* | .982 | 50 | .651 | |
1 | 1 | −1 | .057 | 50 | .200* | .986 | 50 | .808 | |
−1 | 1 | −1 | .122 | 50 | .059 | .973 | 50 | .309 | |
0 | −1 | −1 | .090 | 50 | .200* | .979 | 50 | .511 | |
1 | −1 | −1 | .060 | 50 | .200* | .985 | 50 | .750 | |
−1 | −1 | −1 | .129 | 50 | .036 | .972 | 50 | .277 |
When the significance level is 0.05, the significance (p-value) of the homogeneity test of variance is less than 0.05. Tables 4 and 5 present the details, indicating that the variance is nonhomogeneous. The multifactor ANOVA has a certain tolerance to heterogeneity. When data are unbiased and lack an extreme value, it does not have much impact. Moreover, the multifactor ANOVA function in the SPSS software is based on the framework of the least square method, which is relatively robust and insensitive to the problem of homogeneity of variance. Therefore, we used the multifactor ANOVA method in the SPSS software to examine the factorial effect of the STI policy mix.
Considering GDP as the dependent variable and three types of STI policies as three factors, Table 6 presents the results of multifactor ANOVA. When the significance level is 0.05, the main effects of the three types of STI policies are extremely significant. Among pairwise interactions of the three STI policies, the interaction effect of supply and demand policies is extremely significant and that of supply and environmental policies and demand and environmental policies is insignificant. The interaction effect of the three STI policies, namely, the three-dimensional interaction effect, is insignificant. The last column in Table 6 refers to partial Eta squared, and its value represents the size of the main effect or interaction effect. Table 6 shows that among all significant main effects and interaction effects, the impact on GDP in descending order of magnitude is supply policy > demand policy > supply policy × demand policy > environmental policy.
Multifactor ANOVA with GDP as the dependent variable.
Source | Type III Sum of Squares | df | Mean Square | F | Sig. | Partial Eta Squared |
---|---|---|---|---|---|---|
Corrected Model | 2.2E+012a | 26 | 8.6E+010 | 392.732 | .000 | .885 |
Intercept | 6.7E+013 | 1 | 6.7E+013 | 305634.388 | .000 | .996 |
X1 | 2.0E+012 | 2 | 1.0E+012 | 4654.654 | .000 | .876 |
X2 | 1.9E+011 | 2 | 9.3E+010 | 426.015 | .000 | .392 |
X3 | 3.8E+009 | 2 | 1.9E+009 | 8.593 | .000 | .013 |
X1 × X2 | 5.3E+009 | 4 | 1.3E+009 | 6.059 | .000 | .018 |
X1 × X3 | 1.5E+009 | 4 | 3.9E+008 | 1.759 | .135 | .005 |
X2 × X3 | 2.2E+008 | 4 | 5.6E+007 | .256 | .906 | .001 |
X1 × X2 × X3 | 4.7E+007 | 8 | 5.9E+006 | .027 | 1.000 | .000 |
Error | 2.9E+011 | 1323 | 2.2E+008 | |||
Total | 6.9E+013 | 1350 | ||||
Corrected Total | 2.5E+012 | 1349 |
Null hypothesis: Supply, demand, and environmental policies with varied strengths have no significant impact on GDP. There is no significant interaction between supply and demand policies on GDP. There is no significant interaction between supply and environmental policies on GDP. There is no significant interaction between demand and environmental policies on GDP. There is no significant interaction between supply, demand, and environmental policies on GDP.
Table 7 shows that the results of multifactor ANOVA can be obtained when APAA is regarded as the dependent variable, and the three types of STI policies are regarded as three factors. When the significance level is 0.05, the main effects of supply, demand, and environmental policies are extremely significant. When these STI policies interact, the interaction effect between supply and demand policies is extremely significant, as is the interaction effect between supply and environmental policies; however, the interaction effect between demand and environmental policies is insignificant. Additionally, the three-dimensional interaction effect of the three STI policies is insignificant. The last column of Table 7 indicates that among all the significant main effects and interaction effects, the impact on APAA in descending order of magnitude is supply policy > demand policy > supply policy × demand policy > supply policy × environmental policy > environmental policy.
Multifactor ANOVA with APAA as the dependent variable.
Source | Type III Sum of Squares | df | Mean Square | F | Sig. | Partial Eta Squared |
---|---|---|---|---|---|---|
Corrected Model | 5.9E+015a | 26 | 2.3E+014 | 499.640 | .000 | .908 |
Intercept | 4.6E+016 | 1 | 4.6E+016 | 101398.552 | .000 | .987 |
X1 | 5.5E+015 | 2 | 2.7E+015 | 6082.494 | .000 | .902 |
X2 | 3.1E+014 | 2 | 1.6E+014 | 348.581 | .000 | .345 |
X3 | 7.8E+012 | 2 | 3.9E+012 | 8.683 | .000 | .013 |
X1 × X2 | 3.2E+013 | 4 | 8.0E+012 | 17.668 | .000 | .051 |
X1 × X3 | 1.6E+013 | 4 | 4.1E+012 | 9.040 | .000 | .027 |
X2 × X3 | 1.4E+012 | 4 | 3.6E+011 | .800 | .525 | .002 |
X1 × X2 × X3 | 4.9E+011 | 8 | 6.1E+010 | .136 | .998 | .001 |
Error | 6.0E+014 | 1323 | 4.5E+011 | |||
Total | 5.2E+016 | 1350 | ||||
Corrected Total | 6.5E+015 | 1349 |
Null hypothesis: Supply, demand, and environmental policies with varied strengths have no significant impact on APAA. There is no significant interaction between supply and demand policies on APAA. There is no significant interaction between supply and environmental policies on APAA. There is no significant interaction between demand and environmental policies on APAA. There is no significant interaction between supply, demand, and environmental policies on APAA.
This study analyzed the factorial effect of the STI policy mix in detail, primarily the interaction between STI policies. For achieving this objective, we employed the multifactor ANOVA method to analyze the factorial effect of STI policies.
The first question we sought to address is the interaction between STI policies. We found significant interactions between STI's supply, demand, and environmental policies, which significantly impact GDP or APAA (Meissner & Kergroach, 2021). These significant interactions also demonstrate that the STI policy mix can produce specific new properties (in system science, this is termed the emergence phenomenon), which is not possessed by a single STI policy (Kalantari et al., 2020; Puga-Gonzalez & Sueur, 2017).
The second question is whether all the main effects and interactions are significant. The results of the multifactor ANOVA demonstrate that the main effects of the three types of STI policies are extremely significant regarding GDP as the dependent variable or APAA as the dependent variable; however, regarding GDP as the dependent variable, only the interaction between supply and demand policies is significant among the three STI policies. Other interactions are insignificant. Meanwhile, regarding APAA as the dependent variable, the interaction between supply and demand policies is significant among the three STI policies; the interaction between supply and environmental policies is also significant, whereas other interactions are insignificant.
The third question is whether all the main effects are greater than the interactions. Results show that by regarding GDP as the dependent variable, the interaction between supply and demand policies is significant; the impact of this interaction on GDP is greater than that of the main effect of environmental policy on GDP. When APAA is the dependent variable, the interaction between supply and demand policies and between supply and environmental policies is significant, and the impact of these two interactions on APAA is more significant than that of the main effect of environmental policy.
Policy implicationsThe following policy suggestions are proposed based on our research conclusions. First, policymakers should approach STI policies from a systematic perspective. Policymakers can rationalize policy decision-making by completely mastering the primary information and comprehensively analyzing the policy impact mechanism. Second, policymakers should realize that STI policies do not exist independently but interact with one another. As human society advances, the types and number of policies have considerably increased. Various policies are currently mixed. As such, the governance of one policy can be affected by other policies, and the effects are often positive or negative. Hence, policymakers should have a holistic consideration when formulating a particular STI or several STI policies.
This study provides the following policy implications. First, a single STI policy treatment is unsuitable for the scientific implementation of policies because STI policies do not exist independently; there are interactions between policies that cannot be disregarded. Results tend to be biased when interactions between STI policies are ignored while examining the impact of a single policy. Scientific and effective policymaking requires decision-makers to approach STI policies systematically. Second, all types of STI policies play different roles and should be treated equally. Considering the constraint of limited human and material resources, we should grasp the key points when formulating STI policies to maximize their role. Additionally, we should sort the policies according to their impact on policy objectives and decide on the STI policies to be implemented according to the policy with the most significant impact on the policy objectives.
ConclusionSTI policies do not exist independently, and there are interactions between policies; however, further research is required on the interaction among STI policies. Moreover, the core of the STI policy mix is the interaction among STI policies. Therefore, we used multifactor ANOVA to investigate the factorial effects on the STI policy mix. Results revealed the main effects and interactions of STI policies. Our empirical research showed that the main effects of STI policies were significant. We also found significant interactions among STI policies; however, not all interactions were significant, and not all main effects were greater than the interactions. Therefore, the scientific decision-making in relation to STI policy could occur only after a systematic analysis.
Research limitations and future prospectsWe examined the factorial effect of the STI policy mix and analyzed the main effect and the interaction of STI policies. The analysis of their main effect, which was based on multifactor ANOVA, effectively supplements the existing research and serves as a reference for the government to formulate scientific policies. Although this study obtained some meaningful results, only the factorial effect of the STI policy mix was studied; differences in the effects of the STI policy mix were ignored. These differences should be considered in future research to make the present findings more applicable in practice.
Data Availability StatementThe data used to support the findings of this study are included within the article.
This work was supported by the China Postdoctoral Science Foundation under Grant 2021M693775.