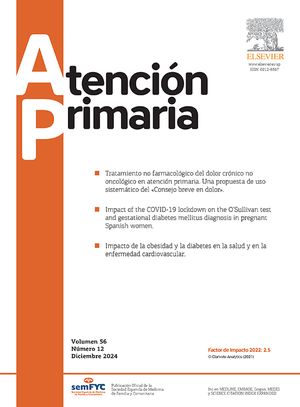
Editado por: Dr. Josep Vidal Alaball General Generalitat de Catalunya Spain
Más datosArtificial intelligence (AI) can be a valuable tool for primary care (PC), as, among other things, it can help healthcare professionals improve diagnostic accuracy, chronic disease management and the overall efficiency of the care they provide. It is important to emphasise that AI should not be seen as a replacement tool, but as an aid to PC professionals. Although AI is capable of processing large amounts of data and generating accurate predictions, it cannot replace the skill and expertise of professionals in clinical decision making. AI still requires the interpretation and clinical judgement of a trained healthcare professional and cannot provide the empathy and emotional support often required in healthcare.
La inteligencia artificial (IA) puede ser una herramienta de gran valor para la atención primaria (AP), ya que, entre otras cosas, puede ayudar a los profesionales de la salud a mejorar la precisión en los diagnósticos, la gestión de enfermedades crónicas y la eficiencia general del cuidado que proporcionan. Es importante subrayar que la IA no debe ser vista como una herramienta de sustitución, sino como una ayuda para los profesionales de la AP. Aunque la IA es capaz de procesar grandes volúmenes de datos y generar predicciones precisas, no puede reemplazar la destreza y la experiencia de los profesionales en la toma de decisiones clínicas. La IA todavía requiere la interpretación y el juicio clínico de un profesional de la salud capacitado, y no puede ofrecer la empatía y el apoyo emocional que a menudo se requiere en el ámbito sanitario.
In the context of a conference on AI in Family and Community Medicine (FCM), a survey was conducted among the participants to learn about their perception of AI in the healthcare field. The words most often mentioned when describing AI in healthcare by attendees were ‘help’, ‘innovation’, ‘tool’, ‘future’ and ‘facilitation’. Sixty-one percent of the 57 attendees who responded to the audience interaction questions expressed that they believe AI in healthcare is already a reality.
When ethical principles related to AI in healthcare were explored, participants highlighted ‘responsibility’ as the most important principle, with 33% of responses. It was followed in importance by ‘protection of privacy and intimacy’ (17%), ‘caution’ (13%), ‘equity’ (13%), ‘respect for autonomy’ (11%), ‘well-being’ (7%), ‘sustainable development’ (4%) and ‘solidarity’ (2%).
Regarding the applicability of AI in FCM, participants considered that AI could be most useful in the ‘debureaucratization of consultations’ (with an average rating of 4.46 out of 5), followed by ‘aspects of epidemiological surveillance’ (4.43/5), ‘analysis of medical data’ (4.43/5), ‘tools for research’ (4.38/5) and ‘diagnostic support’ (4.34/5), as shown in Table 1.
Applicability of AI in different healthcare settings.
Options | Average score (1–5) |
---|---|
Diagnostic support tool | 4.34 |
Therapeutic support tool | 4.04 |
Follow-up issues | 3.84 |
Epidemiological surveillance | 4.43 |
A tool for optimising the management of healthcare resources | 4.07 |
Tools for debureaucratization | 4.46 |
Teaching aids | 4.16 |
Research avenues | 4.38 |
Triage tools | 3.96 |
Screenings | 4.09 |
Disease risk prediction | 3.96 |
Patient empowerment | 3.50 |
Analysis of medical data | 4.43 |
It is important to note that the vast majority of respondents (96%) had not received previous training in AI, but 96% of them expressed interest in receiving training or expanding their knowledge in this area.
The purpose of this article is to serve as a basis for bringing AI closer to PC professionals, addressing from its most technical concepts to the ethical aspects surrounding it and the possible ways of applying it in consultations.
What do we mean by artificial intelligence?There is currently no single globally accepted definition for the term AI. This year, the European Parliament has started to establish the legislative framework that will regulate AI-based systems, and this framework provides a description that is as extensive as it is generic: “software that is developed using one or more of the techniques and approaches […] and that can, for a given set of human-defined objectives, generate results such as content, predictions, recommendations, or decisions that influence the environments with which they interact”.1
Therefore, by way of introduction, it is proposed to address AI as opposed to human intelligence. To put it very simply, the intelligence of human beings functions on the basis of the search for meaningful patterns and characteristics from which, depending on the context in which these patterns and characteristics are detected, meaning is inferred.2 This meaning will depend on the education and experience of the human being in question. Human beings are limited in the amount of information we can process per unit of time. In addition, the information we receive is limited to our sensory capacity: sight, hearing, touch, smell and taste. Moreover, we cannot encompass all the knowledge and all the experiences that exist in the world and that have occurred throughout history to try to find that context that explains what characteristics are significant and what their meaning is. AI, however, is based on the ability of computer systems to process information very quickly and massively, extracting useful information, allowing to classify this information, and even generating new information.3 These systems run algorithms that search for patterns and features on databases. It is obvious that the algorithm will limit its analysis capability to patterns and characteristics that we (humans) have told it to find or for which we have sufficiently representative databases for the algorithm alone to discover the existence of these patterns. Computers have a greater capacity for real-time processing, and they are able to encompass a lot of information and of many types, but they do not know how to identify those characteristics that, depending on the context, make the information meaningful, or allow to infer a context that makes them understandable.4
AI has been with us since the 1960s; the first computer programmes helped us to make calculations, store digital information, and edit music, among many other tasks. Over the last 15 years, we have started to use these programmes for other types of tasks that we also need: reasoning, massive data analysis and knowledge development. Therefore, although it is a relatively new term, it has been with us for a long time. The first artificial neural network was proposed before 1960, acting as a precursor to the union of the knowledge branches of science, statistics and computer science. The most relevant milestone in the application of AI occurred in 2010 when the use of parallel data processing architectures (Graphical Processing Units (GPUs)) began to accelerate the processing of information by AI algorithms.5 Since 2010 the world has experienced an explosion in the number of use cases and applications involving AI.
AI basically works on the basis of a set of data to which a series of labels are assigned. These labels reflect a judgement or categorisation proposed by an expert. From the combination of data and labels, different algorithms and mathematical approaches extract a behavioural pattern from which a model can be implemented. In this way, by having a model and new data that have no labels, we can discover that label that an expert would assign.
There are different methodologies and different approaches for implementing AI-based models. These are basically classified into three major branches: the first is supervised learning, which is based on data that have a label and, therefore, we learn through these labels to classify in order to understand the rules that separate the data that would then allow us to classify. The second is unsupervised learning, using data that have no labels to find groupings and differences in these data that allow us to explain their distributions and find common characteristics. The third branch, and the one that has gained the most importance in recent years, is based on artificial neural networks (whose origin dates back to 1960), in which an attempt is made to emulate the behaviour of a model as in a neuron. The neuron receives multiple inputs that may have a different level of importance, which make the combination of the input signals, depending on the level of importance they have, activate or not a neuron that passes information to another neuron. Neural networks have been widely used in many applications and, nowadays, they are greatly prestigious and have a high level of precision for multiple tasks involving audio processing, image processing, and text generation, among many others. A further step in the application of neural networks is what we know as deep learning. This deep learning does not require us to indicate relevant features of the data in order for the model to be able to differentiate the different labels that are present. In these models we let the model itself find the features that may be relevant to discover patterns. That is, the model automatically finds the features and then learns to differentiate which features are associated with a phenotype. This requires a large, representative and robust dataset that allows us to train and validate the model.
The use of AI in medicine is growing at an exponential rate in recent years and is expected to have a major impact on all aspects of not only medicine but also healthcare.6 Despite this, there is a very significant gap between the development of models in a laboratory setting, i.e., in a scientific environment, and the implementation of these models in real conditions.7
Ethics and legislation in artificial intelligence in healthcareIn the field of AI ethics, traditional bioethics is based on four fundamental pillars: beneficence, autonomy, justice and non-maleficence. However, the advance of technological science, particularly in AI research, demands an evolution of bioethics towards new premises and principles. This transformation is essential to avoid repeating past mistakes and to proactively address new ethical and moral challenges, considering key aspects such as transparency, fairness, accountability and privacy.8
One of the main challenges of AI is the accessibility of health services, as there is evidence that new technologies in developed countries generate inequalities. In addition, amplification of inequities has been detected due to algorithms that overestimate certain racial groups to the detriment of others.8
The push towards a new ethic in AI implementation was initiated by non-governmental institutions such as the Future of Life Institute (https://futureoflife.org/). These entities have played a pivotal role in establishing the starting point for the discussion and development of ethical principles related to AI. Their work has been a catalyst for governmental institutions such as the World Health Organization (WHO), the United Nations Educational, Scientific and Cultural Organization (UNESCO) and the European Union to create specific ethical and legal frameworks for the regulation of AI.
In 2017 the Future of Life Institute organised a conference in Asilomar, California, at which AI experts established a set of ethical principles and guidelines with the goal of ensuring that AI is developed in a way that is safe and beneficial to humanity. These principles became known as the “23 commandments to prevent AI from dominating us”.9 Earlier this year, AI and robotics experts brought together by the University of Montreal published the “Montreal Declaration for Responsible Development of Artificial Intelligence” with 10 well-defined principles.10 In 2018, the European Union proposed to the “European Group on Ethics in Science and New Technologies” the formulation of unified ethical and legal principles to regulate the development and implementation of AI. These principles are reflected in the “Declaration on Artificial Intelligence, Robotics and Autonomous Systems” and are based on the treaties and human rights already established by the EU.11
In 2021, the WHO published a guideline on AI applied to health, recognising the great potential of AI in the field of public health and medicine. Although many of the ethical concerns existed before the advent of AI, this technology raises a number of novel concerns. The WHO recommended that the design of AI in health care should be a careful process, reflecting the diversity of socioeconomic contexts and health care systems. It also stressed the importance of training in digital skills, community participation and awareness of this technology. The WHO stressed the need to prevent and mitigate harmful biases in AI that may hinder the equitable provision of medical care. This is because AI systems based on data from high-income countries may not be effectively applicable in low- and middle-income contexts. The WHO hopes that these principles will serve as a sound basis for governments, developers, companies, civil society and intergovernmental organizations to adopt ethical and appropriate approaches in the implementation of AI in the healthcare field.12
A comparison between the AI ethics principles proposed by Asilomar, the Montreal Declaration, the EU Declaration and the WHO guideline is summarised in Table 2.
Comparison between the principles of ethics in AI.
Asilomar | Montreal declaration | EU declaration | Guideline on Al for health (WHO) |
---|---|---|---|
1. Common good | 1. Well-being | 1. Safety, protection and physical and mental integrity | 1. Promoting human well-being, safety and the public interest |
2. Liberty and privacy | 2. Respect for autonomy | 2. Autonomy | 2. Protecting human autonomy and data privacy |
3. Personal privacy | 3. Protection of privacy and intimacy | 3. Data protection and privacy | |
4. Shared benefit | 4. Solidarity | 4. Fairness, equity and Solidarity | 3. Solidarity |
5. Shared prosperity solidarity | |||
6. Non-subversion | 5. Equity | 4. Ensuring inclusion and equity | |
7. Research goal | 6. Democratic participation | 5. Democracy | |
8. Research funding | |||
9. Science-policy link | |||
10. Research culture | |||
11. Value alignment | 7. Inclusion and diversity | 6. Human dignity | 5. Human dignity |
12. Human values | |||
13. Safety | 8. Caution | 7. Rule of law and accountability | 6. Promoting responsibility and accountability |
14. Failure transparency | |||
15. Judicial transparency | |||
16. Responsibility | 9. Responsibility | 8. Responsibility | |
17. Capability caution | |||
18. Race avoidance | |||
19. Risks | 7. Responsive and sustainable | ||
20. Human control | |||
21. Recursive self-improvement | 10. Sustainable development | 9. Sustainability | |
22. Importance | |||
23. Arms race |
Regarding AI legislation in Europe, on 14 June 2023, the proposed European Regulation on AI was published. This regulation aims to establish an AI risk pyramid (Fig. 1). Firstly, it identifies unacceptable risks, such as cognitive manipulation, social scoring, and real-time and remote biometric identification. Secondly, they include high-risk products, which will be subjected to evaluations prior to their commercialisation and during their life cycle. This covers AI systems integrated into products already subject to EU safety legislation, such as toys, aviation, automobiles, medical devices and lifts. This regulation is expected to be approved by the end of 2023 and enter into force by the end of 2025.1
Despite these advances and positive intentions in the field of AI ethics, it is essential that civil society remains vigilant. Muller (2021) warns that there is a tendency for some companies, the military sector and some public administrations to engage in “ethical laundering” to preserve a good public image and continue operating in the same way.13 It is therefore necessary to maintain a critical and constant scrutiny with the active participation of the whole society to ensure an ethical and responsible development of AI.
The development of a new ethic for AI is imperative. These ethics must address crucial aspects including personal data protection, harm minimisation, accountability, ensuring cybersecurity, transparency, data traceability, explainability (an area that requires improvements in AI algorithms), promotion of autonomy, fairness, equity, non-discrimination, responsibility and, not least, sustainability. These ethical principles are fundamental to guide the ethical and responsible use of AI in healthcare, ensuring that this technology contributes to the benefit of humanity as a whole.
Artificial intelligence applications in primary careAI is beginning to have and will undoubtedly have a wide variety of applications in PC (Fig. 2). One of the most important of these applications will be as a diagnostic support tool. For example, it can be used in the recognition of medical images, such as fundus images, X-rays, or dermatological images. In addition, AI can also assist in the interpretation of electrocardiograms, and in the transcription of clinical information using speech recognition tools.14,15
Another relevant area of application is as therapeutic support tools. AI makes it possible to personalise medical treatments and predict patient adherence to these treatments, resulting in more efficient and effective medical care. It can also anticipate adverse drug reactions, improving treatment safety.14,15
In addition, AI can be very useful in risk prediction. In this way, it can be used as a medical triage tool helping professionals to prioritise patients according to their needs. Personalised screening and follow-up for specific diseases based on individual risk estimation is another possible use of AI.14,15
AI can also aid clinical research by enabling the extraction and analysis of large volumes of data from electronic health records. 14.15
Speech recognition and text generation algorithms can contribute to the simplification and debureaucratization of PC consultations, for example, by assisting in the writing of clinical courses, electronic recording in medical records synchronously during the consultation and the writing of medical reports.16
Generative AI, through chatbots, provides patients with a consultation tool for common symptoms and health advice, contributing to health promotion through personalised advice. In addition, wearable devices, such as smartwatches and sensors, allow the recording of health constants and states, facilitating personal health monitoring by patients.16
Finally, in clinical and educational settings, tools based on natural language recognition can be used to improve the communication skills of professionals.14,15
In the context of chronic diseases that are highly prevalent in PC, AI has been studied mainly in the management of cardiovascular diseases, such as arterial hypertension.17 In this sense, applications have been explored that range from diagnostic support, which allows the establishment of optimal blood pressure values for each patient, to improving therapeutic adherence and predicting the evolution of the disease.17 Likewise, research has been conducted for the early diagnosis of conditions such as heart failure by analysing electrocardiogram recordings, or to identify atrial fibrillation from normal ECGs.18
In the field of dermatology, numerous algorithms have been developed for image analysis. Initially these focused on skin cancer screening, but in recent years they have broadened their focus to address all types of skin pathologies, from infectious to inflammatory illnesses, including benign tumours, demonstrating diagnostic accuracy comparable to that of dermatologists. In studies specifically focused on PC, inferior results have been seen in clinical practice, which demonstrates the need for training of these algorithms with illnesses prevalent in PC. However, it is important to highlight that most of these algorithms provide more than one diagnosis, which is especially useful in the differential diagnosis process, being helpful in 92% of the cases assessed in this study by PC professionals.19
In the management of diabetes, the use of algorithms for retinography reading support for screening diabetic retinopathy is well documented with sensitivities around 87–97% and specificities around 96–97%,20,21 as well as for the detection of other ocular pathologies such as glaucoma and age-related macular degeneration.20
In respiratory pathology there are several models of support for reading chest radiographs,22 as well as for the better classification and diagnostic accuracy of pulmonary diseases such as COPD, asthma, interstitial pathology, neuromuscular diseases, among others, combining pulmonary function tests with the clinical data of patients.23
So far, these are some of the main described uses of AI in the PC setting. However, it is very likely that in the coming years we will see a significant increase in the literature and potential applications of this technology in PC.
ConclusionsWhile it is true that we are at an early stage of maturity in the implementation of AI in the healthcare field, particularly in PC, more and more studies indicate that we could benefit from using it in multiple tasks in our daily lives to improve the diagnosis, treatment, and follow-up of numerous diseases.
Collaboration between AI developers and healthcare professionals is essential to ensure effectiveness and safety in the use of technological solutions for the benefit of patients. Interaction between AI and healthcare professionals, supported by appropriate training of professionals and external validation of algorithms in the PC context, emerges as a promising approach to improve healthcare in PC and optimise outcomes for both patients and healthcare professionals.
The ability of AI to analyse and process large amounts of data can provide valuable information for clinical decision making, but it is the experience and clinical judgement of healthcare professionals that provides the necessary context for interpreting these findings.
FundingThere has been no financial support for this work.
Conflict of interestThere are no known conflicts of interest associated with this publication.