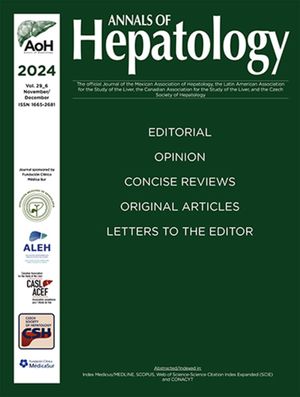
Abstracts of the 2023 Annual Meeting of the ALEH
Más datosNo
Introduction and ObjectivesDifferents ultrasound (USD) signs have been described for the diagnosis of cirrhosis. Among them, the irregularity of the liver shape and the liver echostructure are the most specific and sensitive findings. The echostructure of the liver parenchyma can be classified by the operator as smooth or coarse, the latter being suggestive of chronic liver disease. This classification is not free of subjectivity. The objective of our study was to diagnose cirrhosis by analyzing the liver echostructure through artificial inteligence (AI). We here propose LivGuard, a deep learning binary classifier for cirrhosis detection from a single ultrasound image from general and point-of-care pocket-handheld USD (POCUS).
Patients / Materials and MethodsThe dataset was composed of 1625 two-dimensional, ultrasound liver images annotated as cirrhotic (N=677) or not (N=948) captured from 165 individuals at Sanatorio Sagrado Corazon and Sanatorio de los Arcos, Buenos Aires, Argentina. We stochastically split the master set into training (N=1297; 79.8%), validation (N=159; 9.7%), and test (N=169; 10.2%) sets that were completely disjointed. The output of the efficientNetv2 convolutional neural network (CNN) was a score between 0 and 1 to exhibit the probability of cirrhosis.
Results and DiscussionThe Artificial Intelligence (AI) System achieved accuracy in the test set of 88.7%. Sensitivity, specificity, positive (P) and negative (N) predictive values (PV) were 88.8%, 88.5%, 85.5% and 92.2%, respectively. The system was additionally evaluated in a test set of images (N=180; positive for cirrhosis=64) obtained through Butterfly POCUS. The AI system achieved an overall detection rate of 88.8%. Sensitivity, specificity, positive (P) and negative (N) predictive values (PV) were 100%, 82.7%, 76.1% and 100%, respectively.
ConclusionsLivGuard is proven to be a high performer as cirrhosis classifier in ultrasound images. Further work is required to validate this algorithmic framework in prospective cohorts of patients in additional clinical trials and/or real-world datasets.