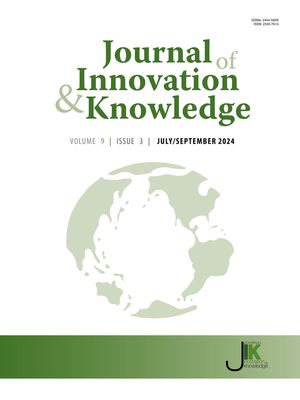
Editado por: Brij B. Gupta, Kwok Tai Chui
Más datosThe purpose of this paper is to explore the role of knowledge-based dynamic capabilities in national innovation systems in the achievement of sustainable development goals, employing an empirical approach in the context of the unprecedented COVID-19 crisis. Based on data from 130 sample countries, we analyzed the impact of knowledge-based dynamic capabilities on the achievement of sustainable development goals using PLS-SEM. In particular, we considered the differences in the impact of knowledge-based dynamic capabilities on the achievement of sustainable development goals at different stages of economic development. The results show that knowledge-based dynamic capabilities have a positive impact on the achievement of sustainable development goals, while their compositional dimensions have a dual impact, both direct and indirect. In addition, knowledge-based dynamic capabilities have different impacts on the achievement of sustainable development goals at different stages of economic development. This indicates that a country's economic development level will affect the relationship between knowledge-based dynamic capabilities and the achievement of sustainable development goals. However, it also means that there is a more complex relationship between these capabilities and the achievement of the goals. This study offers a new perspective for sustainable development research, adds new insights to the theory of linking knowledge-based dynamic capabilities to the achievement of sustainable development goals, and provides a measurement standard for the impact of those capabilities on the goals.
Sustainable development has long been a major challenge that human beings must face. Countries around the world are actively exploring methods to promote the transformation of national sustainable development in order to simultaneously solve economic, social, and environmental challenges (Fernandes, Rodrigues & Ferreira, 2022; Lu, Tsai & Shen, 2020). In 2015, the United Nations proposed 17 Sustainable Development Goals (SDGs), which emphasize the importance of stakeholders and focus on cross-sectoral and transnational cooperation for sustainable development (Chauhan, Kaur, Arrawatia, Ractham & Dhir, 2022; Masuda, Kawakubo, Okitasari & Morita, 2022). Concerted international action by countries around the world is required to address this major challenge (Masuda et al., 2021). Especially given the COVID-19 epidemic and its unprecedented changes, all countries must pursue a green recovery to establish a more effective cooperation mechanism at the global level, thus achieving low-carbon transformation and technical, economic, and industrial cooperation in global climate governance.
Obviously, adding social and environmental goals to economic goals often leads to new trade-offs, inevitably adding more complexity and uncertainty to the innovation process. This requires us to change the innovation paradigm, and innovation for sustainability is seen as one effective strategy for doing so (Fan & Fan, 2020). Research on sustainable innovation at the SME level has shown that SMEs not only have a greater capacity for sustainable innovation but can also create more sustainable production and consumption patterns (Klewitz & Hansen, 2014). Meanwhile, regional-level research focuses on relevant aspects of regional green or high-quality development (Cheng, Wang & Huang, 2021; Wang, Hong & Liu, 2019). Finally, research on sustainable innovation at the national level focuses on the key issues of how to promote and accelerate sustainable innovation (Şiir Kılkış, 2016; Ruii, Mileti & Dobrota, 2021). Although many studies have discussed the link between innovation and sustainability, their complex relationship still needs to be examined in greater depth.
At present, the outbreak of COVID-19 has greatly affected the SDGs of most countries, which represents a serious setback for the achievement of global SDGs (Chopra, Singha & Gupta, 2022). According to the “Sustainable Development Report 2021,” since the adoption of the SDGs in 2015, the score of the global average SDG index in 2020 decreased for the first time compared with 2019. The fundamental reason is that the poverty and unemployment rates increased by a large margin as a result of COVID-19. As a result, the major challenges that now face society require profound changes in social, political, economic, and technological systems (Marti & Puerta, 2022). The national innovation systems (NIS) provide an effective framework for addressing these major challenges (Fernandes et al., 2022). Therefore, it is particularly critical to explore how NIS can promote the achievement of the SDGs, which is also the main goal of NIS (Chaminade, 2020).
Scholars have linked NIS with national innovation performance (Robertson, Caruana & Ferreira, 2021) or environmental sustainability (Bresciani, Puertas, Ferraris & Santoro, 2021; Fernandes et al., 2022) and have proved the important role of NIS in achieving economic or environmental sustainability. However, whether the overall achievement of national SDGs is affected by NIS is rarely considered. Scholars and policymakers generally agree that innovation systems not only contribute to economic growth but are also key drivers of SDG achievement (Wang, Fan & Qian, 2021), and knowledge management (KM) plays an important role in this function. Knowledge management is seen as an important contributor to the pursuit of sustainable development, and it is also gaining increasing attention for its promise as a method of promoting sustainability (Chopra, Saini & Kumar, 2021). Moreover, the diversity and complexity of the SDGs have created new requirements for knowledge management. As a result, NIS require specific and new dynamic capabilities to absorb, create, and reconfigure knowledge in a highly dynamic, uncertain, and complex environment in order to address the environmental dynamics of innovation success (Robertson et al., 2021). Scholars call this knowledge-based dynamic capability (KBDC), and KBDC has become a focus in both society and academia. Most scholars have paid more attention to dynamic capabilities in specific environments (Guo & Zhang, 2021; Helfat & Raubitschek, 2018; Wu et al., 2022).
A few scholars have also begun to focus on insights into the dynamic capabilities required for ecosystem innovation. They have analyzed the role of dynamic capabilities in managing service innovation ecosystems (Lütjen, Schultz, Tietze & Urmetzer, 2019) as well as the impact of KBDC in NIS on national innovation performance (Robertson et al., 2021). Nevertheless, there remains a knowledge gap and a lack of empirical testing on the impact and role of KBDC in SDG achievement (Aldieri, Makkonen & Vinci, 2020). Therefore, new insights are needed to understand KBDC at the macro level in order to reveal how KBDC in NIS affects the achievement of SDGs. At the same time, disparities in economic development and innovation capabilities between economies at different income levels have been identified as a major challenge for countries to achieve SDGs. Moreover, as different NIS have heterogeneous knowledge bases, it is necessary to compare and analyze whether KBDC in NIS affects the achievement of SDGs differently depending on the country's income level.
Research on KBDC in NIS and the achievement of SDGs in the existing literature has the following characteristics: (1) it mainly focuses on the KBDC of enterprises, rarely discussing the compositional dimensions of KBDC in NIS and how to measure them; (2) although scholars have discussed the progress of some SDGs at the firm or regional level, there are few national-level assessments on the achievement of SDGs; (3) despite some research on the relationship between innovation and sustainability, more research is necessary on whether the overall achievement of the national SDGs is affected by KBDC in NIS. Several important research questions have rarely been considered, including how and what factors are affected and whether there are differences in the impact of KBDC in NIS on the achievement of SDGs at different economic development stages. Recognizing these questions’ importance, this paper will further explore the impact of KBDC in NIS on the achievement of SDGs, thereby enriching the existing literature and practical research.
Therefore, this paper aims to focus on the connection between KBDC in NIS and the achievement of SDGs. It attempts to answer the following research questions: (1) How should the compositional dimensions of KBDC in NIS be analyzed, and which dimensions contribute to the achievement of SDGs? (2) Are there differences in the impact of the compositional dimensions of KBDC on the achievement of SDGs at different economic development stages? The innovations of this study are mainly reflected in the following three aspects. First, we considered the relationship between KBDC and the achievement of SDGs based on the research framework of national innovation systems, providing a new perspective for sustainable development research. Second, we sought to elucidate which knowledge capabilities of the KBDC in NIS play a role in SDG achievement through an empirical analysis of the compositional dimensions of KBDC and its impact on SDG achievement, thus providing guidance on how to carry out effective knowledge management to support the achievement of SDGs. Third, it is helpful to understand the heterogeneous effect of the compositional dimensions of KBDC on the achievement of SDGs in economies of different income levels. This comparative analysis provides insights for diverse economies to focus on cultivating and developing knowledge capabilities.
The paper is structured as follows. Section 2 provides theoretical background and hypothesis development. Section 3 explains the methodology. Section 4 explores the results. Finally, Section 5 discusses the key findings, theoretical contributions and management implications, limitations, and future research directions.
Theoretical background and hypothesesNational innovation systemsThe concept of NIS was first proposed by Freeman (1987). Later, Nelson (1993) described NIS with a microeconomic basis of innovation, emphasizing the different roles played by limited rational agents, tacit knowledge, and institutional environment (Acs, Audretsch & Lehmann, 2017). Scholars in China have also offered theoretical explanations from various perspectives, but the core meaning is the same: emphasizing the heterogeneity of the system's internal agents and the complex interaction between multiple agents and processes to integrate science and technology, social economy, and environment and achieve sustainable development through global cooperation, collaborative development, and innovation (Lu, 2002).
In recent years, the discussion of national innovation systems has gradually expanded to the global innovation system. As the rapid development of science and technology has broadened the boundary of innovation, the links between innovation agents have constantly changed, and the boundaries of innovation activities have become more blurred. As a result, innovation has gradually integrated into a more open and globalized system of multilateral cooperation. Indeed, Lee and Lee (2020) believe that innovations are actually generated and continuously developed in the global environment. Since Chesbrough (2003) proposed the open innovation paradigm, scholars have discussed “collaborative innovation,” “cooperative innovation,” “open innovation,” and “networked innovation,” emphasizing the importance and necessity of openness and collaboration in the innovation process (Hutton, Demir & Eldridge, 2021; Li, Wang, Xue & Su, 2022; Ruan, Chi & Zhang, 2022). Moreover, Deng, Liang and Wang (2020) believes that NIS cannot function effectively without the interaction between the agents and cross-border knowledge flows. Subsequently, research on NIS in the world's economies has also been moving from theoretical analysis to practical application, becoming the main means for economies to formulate innovation policies (Liu, Zhang, Zhai & Yang, 2022). Scholars in China have also expanded NIS in many aspects based on China's scientific and technological innovation scene, proposing ideas with Chinese characteristics (Chen, 2018; Zhang & Wen, 2022).
Sustainable development goalsGiven the international nature of environmental issues, each country should establish sustainable development goals to actively address the environmental problems relevant to human development. Given the complexity of environmental problems and the multifaceted nature of their solutions, supranational organizations such as the United Nations must assume more and greater responsibilities to solve global challenges and crises (Montenegro, Ribeiro & Britto, 2020). Accordingly, the UN 2030 agenda provides effective solutions to global challenges such as climate change, social inequality, and environmental degradation. This is related to the concept of policy coherence for sustainable development, which emphasizes the importance of consistent national political solutions (Coscieme, Mortensen & Anderson, 2020). In this context, the UN member states’ agreement on the SDGs is a major policy achievement in assessing global environmental, social, and economic development and guiding future sustainable development (Ripple et al., 2017). In light of the shared commitment of countries around the world, governments will take a variety of effective actions to protect ecosystems, promote equity, and focus on sustainable development while recognizing the interconnectedness of these goals in achieving human well-being (Coscieme et al., 2020).
In recent years, scholars have assessed the progress of some SDGs to varying degrees. Firm-level studies have explored the potential role of corporate activities in supporting SDG achievement (Boiral, Heras-Saizarbitoria & Brotherton, 2019; Cao, 2021; Ike, Donovan, Topple & Masli, 2019), identified the main factors that affect the achievement of SDGs (Li, Peng & Lv, 2022; Vander Waaland Thijssens, 2020), and evaluated firms’ sustainability performance (Khaled, Ali & Mohamed, 2021; Wang, Tan & Yao, 2021). Meanwhile, studies conducted at the city or regional level have focused on the construction of sustainability indicators (Wang, Zhang & Liu, 2020) and the evaluation of sustainable development performance (Zhang, Zhang, Wang & Fan, 2017). Finally, national-level studies mainly focus on the achievement of a few SDGs such as poverty eradication, health, health systems, and the environment (Roy & Pramanick, 2019; Zhao, L. & Chen, 2020). A few researchers have also begun to focus on the overall achievement of the SDGs (Zhu, Sun & He, 2018).
Knowledge and KBDCAs a basic resource in the development of NIS, knowledge is continuously generated and accumulated through the interaction and innovation process among multiple agents inside and outside NIS. This is crucial to a country's sustainable development (Acs et al., 2017). Breznik (2018) argues that an innovative system based on knowledge is not only more innovative but can also determine the appropriate direction for the system's future sustainable development. The concept of knowledge-based dynamic capability (KBDC) originated from the knowledge-based view (KBV), which regards knowledge as a key strategic resource for organizational innovation that contributes to the formation of an organization's competitive advantage. In the context of sustainable development, Lim, Tseng, Tan and Bui (2017) argue that KBDC acquires, creates, and applies knowledge in a sustainable manner primarily by simultaneously considering social, economic, and environmental factors. Similarly, Júnior, Faccin, Martins and Balestrin (2019)) argue that KBDC is the ability to address environmental dynamics by acquiring, generating, and combining internal and external knowledge resources. Abbas and Sagsan (2019) divided KBDC into four dimensions including knowledge creation, knowledge acquisition, knowledge sharing, and knowledge application, while Chien and Tsai (2021) divided the concept into knowledge absorption, knowledge creation, knowledge storage, and knowledge application. Finally, Lee, Lee and Lee (2021) argued that the three most important dimensions that distinguish the types of NIS are those related to innovation, diffusion, and knowledge; accordingly, they focus on the dimensions related to knowledge creation, diffusion, and use.
KBDC is crucial to a country's success in achieving sustainable development goals, as it emphasizes the continuous pursuit of the absorption, integration, and creation of internal and external knowledge in the innovation system. It also promotes the diffusion and influence of knowledge through management in order to effectively cope with the complex and changing international environment and improve the efficiency of NIS. Under the open innovation paradigm, KBDC in NIS combines processes that move from the outside in and from the inside out—that is, knowledge is realized from outside to inside through knowledge absorption and creation and also from inside to outside through knowledge diffusion and knowledge impact, further improving the efficiency of NIS and achieving the SDGs (Laperche, 2016). Therefore, this study defines four related knowledge capabilities—knowledge absorption, knowledge creation, knowledge diffusion, and knowledge impact—as the KBDCs in NIS. This is consistent with the dimensional division of KBDC in the previous literature and the view of Robertson et al. (2021). In summary, these capabilities contribute to innovative activities in NIS to achieve the SDGs.
KBDC and the achievement of SDGsKnowledge absorption and SDGsThe process of absorbing knowledge includes acquiring, interpreting, assimilating, integrating, and finally utilizing knowledge from various sources and of various types (Gold, Malhotra & Segars, 2001). Previous studies have confirmed that there is a positive correlation between knowledge absorption and sustainable development (Pai & Chang, 2013). Moreover, Shahzad et al. (2019) argued that the ability to acquire and absorb knowledge has a significant impact on both economic and environmental performance. Meanwhile, Abbas and Sagsan (2019) analyzed how knowledge management affects the environmental, social, and economic aspects of sustainability, finding that knowledge absorption had a positive impact on sustainable development. Ye and Chen (2022) reported a consistent conclusion: knowledge absorption had a positive impact on organizations’ sustainable development. Given these results, this paper proposes the following hypothesis:
H1. Knowledge absorption in NIS has a positive impact on the achievement of SDGs.
Knowledge creation is a complex and dynamic process that relies on interactions among multi-agent inside and outside NIS, the acquisition of knowledge from outside the system (Júnior et al., 2019), and the formation of new knowledge in an innovative or improved way. As knowledge creation adds new knowledge, technologies, and methods to the knowledge base of NIS, the process of creating or acquiring new knowledge is a necessary condition for the formation of sustainable competitive advantages. Moreover, it can improve national innovation capabilities and promote the achievement of national SDGs (UNGC, 2018). Albort-Morant, Leal-Rodríguez and De Marchi (2018) argue that knowledge-intensive organizations not only encourage knowledge creation and the development of green products but also constantly consider their impact on the environment. In addition, Gupta et al. (2016) have demonstrated that knowledge creation is closely related to competitive advantage and is regarded as an important indicator of innovation performance and environmental performance at the national level. Guzel, Arslan and Acaravci (2021) discussed the positive impact of knowledge management in achieving SDG 3 (good health and well-being) and validated the important role of knowledge creation. Therefore, we propose the following hypothesis:
H2. Knowledge creation in NIS has a positive impact on SDG achievement.
Knowledge diffusion has become a key factor in improving economic and environmental sustainability, as well as an important part of forming a country's sustainable competitive advantage (Tang, Ma, Xiao & Xiao, 2020). Indeed, Manniche and Testa (2018) found that the outcomes of innovation systems were directly affected by the diffusion of the knowledge created. Bossink (2018) analyzed the role and impact of knowledge diffusion on sustainable innovation. The authors found that knowledge diffusion mechanisms stimulate the creation of sustainable innovation, positively influence sustainable innovation performance, and contribute to SDG achievement. Finally, Xue, Jiang, Huang and Liang (2019) find that knowledge diffusion helps to enhance the absorption, development, and application of knowledge in the innovation system. They argue that NIS should have the ability to create or generate new knowledge, disseminate this knowledge through NIS, and quickly transfer the acquired knowledge into new technologies or processes for sustainable development. Given this, this paper proposes the following hypotheses:
H3. Knowledge diffusion in NIS has a positive impact on the achievement of SDGs.
The application of new knowledge—especially competitive knowledge—not only creates new core competencies for the innovation system but also helps to improve its overall performance. Wang (2011) reported that the spillover and degree of application of knowledge had an important impact on the overall technological innovation level and productivity improvement of the region, which is conducive to the region's sustainable development. At the organization level, Albort-Morant et al. (2018) argued that the degree of use of new knowledge affected an organization's environmental and innovation performance. Robertson et al. (2021) claimed that knowledge impact, as an output indicator, had an intuitive relationship with innovation performance. Finally, Aldieri, Makkonen and Vinci (2022) analyzed whether environmental knowledge management can help economies achieve the SDGs. They found that the effective use of environmental knowledge could contribute to achieving SDG12. Given these results, we propose the following hypothesis:
H4. Knowledge impact in NIS has a positive impact on the achievement of SDGs.
Of course, the four dimensions of knowledge absorption, creation, diffusion, and impact have close internal connections. They tend to develop cumulatively and build on each other, forming a knowledge-based dynamic capability in NIS. In other words, knowledge absorption updates and expands the knowledge base of NIS, provides prerequisite knowledge for knowledge creation, and is a prerequisite for knowledge creation. Knowledge creation, in turn, provides new knowledge sources for knowledge diffusion and impact, affecting the degree of diffusion and the quality of impact. As knowledge diffusion broadens external knowledge sources for knowledge absorption, it is a decisive factor affecting absorption. Finally, knowledge impact also has a positive effect on the further diffusion of knowledge. Given this, we assume the following mediation relationships:
H5a. The relationship between knowledge creation and the achievement of SDGs is mediated by knowledge absorption.
H5b. The relationship between knowledge diffusion and the achievement of SDGs is mediated by knowledge creation.
H5c. The relationship between knowledge impact and the achievement of SDGs is mediated by knowledge creation.
H5d. The relationship between knowledge absorption and the achievement of SDGs is mediated by knowledge diffusion.
H5e. The relationship between knowledge diffusion and the achievement of SDGs is mediated by knowledge impact.
Fig. 1 shows a theoretical model of the relationship between knowledge-based dynamic capabilities in NIS and the achievement of national SDGs.
MethodologyData and samplesTo test the above hypotheses, we collected data to measure KBDC and SDGs. The data for KBDC is based on data collected in the publicly released Global Innovation Index (GII) 2021. The GII is a collaborative research project among Cornell University, INSEAD, and the World Intellectual Property Organization. We chose GII 2021 as the main data source for measuring KBDC for the reasons described by Robertson et al. (2021). On the one hand, the datasets contain basic data from countries around the world, the data scale is relatively large, and the data is relatively complete. At the same time, the data is allowed to reflect the improvement of the results during the actual operation. On the other hand, the datasets in the GII are the latest global innovation data sets used in research at that time; as a result, their consistency and reliability have been assessed to ensure the credibility of the research data.
The data for SDG measurement is based on datasets in the publicly released Sustainability Development Report (SDR) 2021. This report was completed by the team of Jeffrey Sachs of Columbia University. The SDR integrates data and analysis provided by international organizations, civil society organizations, and research centers. Most of the data (about two-thirds) is developed by international organizations with extensive and strict data validation processes. Other sources of statistical data (about one-third) include household surveys, data from civil society organizations and networks, and peer-reviewed journals, which also ensure data reliability.
In the sample selection, we selected 130 economies with different incomes as research samples according to the ranking of 132 world economies by income in the GII 2021 and the ranking of 193 economies by income in the SDR 2021. We also considered the requirements of sample uniformity and data completeness. At the same time, this paper divides the 130 sample countries according to different stages of economic development to reveal the differences in the impact of KBDC on the achievement of SDGs in economies at different income levels. The specific classification is presented in Appendix A.
Data analysisWe employed structural equation modeling (SEM) to study the relationship between KBDCs and the achievement of SDGs. SEM is an advanced statistical method that integrates path and factor analysis. It can add explicit variables, implicit variables, and random error terms to the model at the same time, greatly improving the model's applicability and interpretability. At the same time, an important feature of SEM is that the hypothetical causal model must be based on reliable and relevant theories. SEM can be used to verify whether a theoretical model is consistent with actual statistical data; it is confirmatory rather than exploratory (Bai, 2020). Therefore, it is very appropriate to introduce an SEM based on a theoretical model to verify and refine the model that knowledge-based dynamic capabilities affect SDG achievement.
Variables and measurementsIndependent variableThe independent variable in this paper is KBDC. Robertson et al. (2021) and Fernandes et al. (2022) note that the Global Innovation Index (GII) compares the performance of NIS in economies of different income levels, and the framework is well suited for measuring and evaluating elements of NIS. The GII consists of 81 indicators, and it provides a classification index of innovation input and output that includes indicators such as knowledge absorption, knowledge creation, knowledge diffusion, and knowledge impact, which reflect KBDC well. The merits of the GII in measuring NIS have also been recognized by the United Nations. Therefore, this study uses the scores of the four knowledge-related capabilities in the GII—knowledge absorption, knowledge creation, knowledge diffusion, and knowledge impact—to characterize the KBDC in NIS. Table 1 shows the description and measurement of each variable.
Variable Measurements.
The dependent variable of this paper is SDGs. Here, we use the SDG index to measure a country's achievement of the SDGs. The index accurately reflects countries’ performance on the 17 sustainable development goals. It was agreed upon by the international community in 2015, and it gives the same weight to all 17 goals. All countries use the same basket of indicators to generate SDG index scores and rankings. Therefore, the difference between a country's score and 100 points reflects the distance that must be overcome to achieve optimal SDG performance. The SDG index is derived from the Sustainability Development Report (SDR), which presents and aggregates data on countries’ performance in achieving the SDGs. Table 1 shows the variable descriptions and measurements.
ResultsOverall analysisCorrelation and multicollinearity diagnosisTable 2 shows the variables’ descriptive statistics and correlations. We found that, except for the correlation coefficient between knowledge absorption and knowledge diffusion, the correlation coefficients of other variables are all less than 0.7 and were all significant at the 1% confidence level. In addition, we use the VIF to evaluate multicollinearity. Ideally, the VIF value should be close to 3.3 and less than or equal to 5 (Becker, Ringle, Sarstedt & V¨olckner, 2015). Except for knowledge diffusion (VIF = 3.397), all the VIF values are below the threshold of 3.3 and are thus all within the acceptable range.
Descriptive statistics and correlations of the variables(N = 650).
There are many different opinions about the evaluation of model fitness. Appropriate fitting rules change depending on different assumptions about the distribution and attributes of the observed variables, and estimation methods will also be different. The fitting test of the structural model can adopt a variety of indicators. The fitting index of the SEM is generally divided into absolute fit, incremental fit, and parsimonious fit indices. In this paper, we use AMOS25 to analyze the applicability and the overall model fit of the relationship between KBDC and SDGs. As Table 3 shows, the standard chi-square value (χ2/df) of the overall theoretical model is 1.769 (less than the recommended value of 3), the goodness-of-fit index GFI is 0.995, and the comparative fit index AGFI is 0.919. The square root RMSEA is 0.077, which is less than the theoretical standard of 0.08, and the CFI, NFI, NNFI, and IFI are all greater than the standard value of 0.9, indicating that the model fit is adequate.
Path analysis and hypothesis testingTable 4 shows the path coefficients and hypothesis testing results of the influence of KBDC on SDG achievement.
Path coefficients and hypothesis testing results.
Hypothesis | Path | β | t- statistic | p-value | Results |
---|---|---|---|---|---|
H1 | KA→SDGI | −0.007 | −0.1 | 0.92 ns | Reject |
H2 | KC→SDGI | 0.163 | 3.965 | *** | Accept |
H3 | KD→SDGI | 0.047 | 0.804 | 0.421 ns | Reject |
H4 | KI→SDGI | 0.301 | 4.575 | *** | Accept |
H5a | KC→KA | 0.311 | 5.004 | *** | Accept |
H5b | KD→KC | 0.268 | 2.091 | 0.037** | Accept |
H5c | KI→KC | 0.577 | 3.837 | *** | Accept |
H5d | KA→KD | 0.903 | 12.312 | *** | Accept |
H5e | KD→KI | 0.448 | 9.035 | *** | Accept |
We found that knowledge creation (β=0.163; t(650)=3.965; p<0.01) and knowledge impact (β=0.301; t(650)=4.575; p<0.01) have statistically significant positive impacts on the achievement of SDGs, so H2 and H4 are accepted. In contrast, the effects of knowledge absorption (β=−0.007; t(650)=−0.1; p>0.1) and knowledge diffusion (β=0.047; t(650)=0.804; p>0.1) on SDG achievement are not statistically significant, so H1 and H3 are rejected. Nevertheless, knowledge diffusion indirectly affects the achievement of SDGs through knowledge creation (β=0.268; t(650)=2.091; p<0.05) and knowledge impact (β=0.448; t(650)=9.035; p<0.01), and knowledge absorption (β=0.903; t(650)=12.312; p<0.01) also indirectly affects the achievement of SDGs through knowledge diffusion; therefore, H5b, H5d, and H5e are accepted. Furthermore, knowledge absorption (β=0.331; t(650)=5.004; p<0.01) mediates the relationship between knowledge creation and SDG achievement, while knowledge creation (β=0.557; t(650)=3.837; p<0.01) mediates the relationship between knowledge impact and the achievement of SDGs; as a result, H5a and H5c are accepted. According to the analysis results, we can conclude that knowledge creation and knowledge impact have a direct influence on SDG achievement, while knowledge absorption and knowledge diffusion have an indirect influence. Taken together, these results show that KBDC is conducive to the achievement of SDGs. Therefore, each country should take established measures to improve its knowledge-based dynamic capabilities, focusing in particular on knowledge creation and knowledge influence to better promote the realization of SDGs. Fig. 2 shows the path model of KBDC affecting the achievement of SDGs.
Differences at different stages of economic developmentTable 5 summarizes the descriptive statistics for the 130 country classifications considered at different stages of economic development. In general, developed and transition economies are better at achieving the SDGs than developing economies. In addition, the KBDC of developed economies is significantly stronger than those of transition and developing economies. Therefore, we must assess how the impact of the compositional dimensions of KBDC varies with the achievement of the SDGs at different stages of economic development.
Table 6 shows the path coefficients and hypothesis testing results for the different economic stages of development.
Path coefficients and hypothesis testing results.
Economic stage of development | Hypothesis | Path | β | t-statistic | p-value | Results |
---|---|---|---|---|---|---|
Developed economy | H1 | KA→SDGI | 0.102 | 1.82 | 0.069* | Accept |
H2 | KC→SDGI | 0.074 | 2.818 | *** | Accept | |
H3 | KD→SDGI | 0.12 | 2.9 | *** | Accept | |
H4 | KI→SDGI | −0.051 | −0.852 | 0.394 | Reject | |
H5a | KC→KA | 0.26 | 2.644 | *** | Accept | |
H5b | KD→KC | 0.212 | 0.706 | 0.48 | Reject | |
H5c | KI→KC | 0.214 | 2.546 | 0.011** | Accept | |
H5d | KA→KD | 0.843 | 4.606 | *** | Accept | |
H5e | KD→KI | 0.142 | 0.315 | 0.753 | Reject | |
Transition economy | H1 | KA→SDGI | −0.001 | −0.016 | 0.988 | Reject |
H2 | KC→SDGI | −0.013 | −0.204 | 0.838 | Reject | |
H3 | KD→SDGI | 0.225 | 3.392 | *** | Accept | |
H4 | KI→SDGI | 0.011 | 0.15 | 0.881 | Reject | |
H5a | KC→KA | 0.405 | 2.471 | 0.013** | Accept | |
H5b | KD→KC | 0.572 | 1.821 | 0.069* | Accept | |
H5c | KI→KC | −0.329 | −1.03 | 0.303 | Reject | |
H5d | KA→KD | 0.255 | 0.724 | 0.469 | Reject | |
H5e | KD→KI | 0.524 | 2.734 | *** | Accept | |
Developing economy | H1 | KA→SDGI | 0.069 | 0.707 | 0.48 | Reject |
H2 | KC→SDGI | 0.089 | 1.269 | 0.205 | Reject | |
H3 | KD→SDGI | 0.022 | 0.276 | 0.783 | Reject | |
H4 | KI→SDGI | 0.239 | 2.632 | *** | Accept | |
H5a | KC→KA | 0.173 | 1.249 | 0.212 | Reject | |
H5b | KD→KC | 0.283 | 2.309 | 0.021** | Accept | |
H5c | KI→KC | 0.347 | 2.407 | 0.016** | Accept | |
H5d | KA→KD | 0.931 | 8.7 | *** | Accept | |
H5e | KD→KI | 0.395 | 6.013 | *** | Accept |
For developed economies, knowledge absorption (β=0.102; t(180)=1.82; p<0.1), knowledge creation (β=0.074; t(180)=2.818; p<0.01), and knowledge diffusion (β=0.12; t(180)=2.9; p<0.01) have a statistically significant positive impact on the achievement of SDGs; therefore, H1, H2, and H3 are accepted. In contrast, the effect of knowledge impact (β=−0.051; t(180)=−0.852; p>0.1) on the achievement of SDGs is not statistically significant, so H4 is rejected. However, knowledge impact (β=0.214; t(180)=2.546; p<0.05) indirectly affects SDG achievement through knowledge diffusion, so H5c is accepted. In addition, knowledge absorption (β=0.26; t(180)=2.644; p<0.01) mediates the relationship between knowledge creation and the achievement of SDGs, while knowledge diffusion (β=0.843; t(180)=4.606; p<0.01) mediates the relationship between knowledge absorption and SDG achievement; as a result, H5a and H5d are accepted. These results confirm that the compositional dimensions of KBDC play an important role in achieving the SDGs of developed economies, so developed economies should continuously cultivate and develop their dynamic capability. Fig. 3 shows the path model of KBDC affecting the achievement of SDGs in developed economies.
For transition economies, knowledge diffusion (β=0.225; t(80)=3.392; p<0.01) has a statistically significant positive impact on the achievement of SDGs, so H3 is accepted. In contrast, the effects of knowledge absorption (β=−0.001; t(80)=−0.016; p>0.1), knowledge creation (β=−0.013; t(80)=−0.204; p>0.1), and knowledge impact (β=0.011; t(80)=0.071; p>0.1) on SDG achievement are not statistically significant; therefore, H1, H2, and H4 are rejected. Nevertheless, knowledge creation (β=0.405; t(80)=2.471; p<0.05) indirectly affects SDG achievement through knowledge absorption, so H5a is accepted. Furthermore, knowledge creation (β=0.572; t(80)=1.821; p<0.1) and knowledge impact (β=0.524; t(80)=2.734; p<0.01) mediate the relationship between knowledge diffusion and SDG achievement, so H5b and H5e are accepted. We can conclude that knowledge diffusion and knowledge creation play an important role in achieving the SDGs of transition economies. Therefore, these economies should also focus on the development of knowledge absorption and influence capabilities, while further improving knowledge diffusion and creation. Fig. 4 shows the path model of KBDC affecting the achievement of SDGs in transition economies.
For developing economies, knowledge impact (β=0.239; t(390)=2.632; p<0.01) has a statistically significant positive impact on the achievement of the SDGs, so H4 is accepted. Meanwhile, the effects of knowledge absorption (β=0.069; t(390)=0.707; p>0.1), knowledge creation (β=0.089; t(390)=1.269; p>0.1), and knowledge diffusion (β=0.022; t(390)=0.276; p>0.1) on SDG achievement are not statistically significant; therefore, H1, H2, and H3 are rejected. However, knowledge absorption (β=0.931; t(390)=8.7; p<0.01) indirectly affects SDG achievement through knowledge diffusion. Moreover, knowledge diffusion also indirectly affects the achievement of SDGs through knowledge creation (β=0.283; t(390)=2.309; p<0.05) and knowledge impact (β=0.395; t(390)=6.013; p<0.01); accordingly, H5b, H5d, and H5e are accepted. Knowledge creation (β=0.347; t(390)=2.407; p<0.05) also mediates the relationship between knowledge impact and the achievement of SDGs, so H5c is accepted. We can conclude that knowledge impact, knowledge absorption, and knowledge diffusion play an important role in SDG achievement in developing economies. Therefore, such economies should further cultivate and develop these capabilities. Fig. 5 shows the path model of KBDC affecting the achievement of SDGs in developing economies.
The results reported above suggest that the compositional dimensions of KBDC at different stages of economic development have different degrees of impact on SDG achievement. In particular, the level of economic development appears to affect KBDC's role in the achievement of national sustainable development goals. Therefore, economies at different income levels should seek to cultivate and develop different knowledge capabilities in the process of achieving SDGs.
Discussion and conclusionsKey findingsThis paper examined whether and how KBDC affects the achievement of the SDGs and explored the differences in the impact of KBDC on SDGs achievement at different stages of economic development.
First, our overall analysis of 130 countries shows that knowledge creation and knowledge impact have a direct positive impact on SDG achievement. Although the findings show that knowledge absorption and knowledge diffusion are not statistically significant for SDG achievement, knowledge absorption indirectly affects the achievement of the SDGs through knowledge creation. Moreover, knowledge diffusion also indirectly affects SDG achievement through knowledge impact and knowledge creation, which has a completely intermediary relationship. This suggests that KBDC in NIS has an important impact on the achievement of the SDGs. It also means that the impact is twofold, both direct and indirect. Therefore, all countries should pay attention to these intermediary factors’ important role in the effect of knowledge absorption and knowledge diffusion on SDG achievement while also improving their knowledge creation and knowledge impact capabilities.
Second, the compositional dimensions of KBDC under different stages of economic development have different impacts on the achievement of the SDGs. In developed economies, knowledge absorption, knowledge creation, and knowledge diffusion have a significant impact on the achievement of SDGs. In addition, knowledge impact indirectly affects SDG achievement in developed economies through knowledge creation. For transition economies, knowledge diffusion is also an important factor affecting SDG achievement, while knowledge creation indirectly affects the achievement of SDGs through knowledge absorption. Although the effect of knowledge impact on the achievement of SDGs in developed and transition economies is not significant, knowledge impact is an important driver of SDG achievement in developing economies. At the same time, knowledge absorption indirectly affects the achievement of SDGs through knowledge diffusion, and knowledge diffusion also indirectly affects SDG achievement through knowledge creation and knowledge impact. This suggests that a country's level of economic development affects the relationship between KBDC and SDG achievement, indicating that there is a more complex relationship between these factors.
Theoretical contributions and management implicationsThe present study makes several theoretical contributions. On the one hand, the existing research rarely discusses the compositional dimensions of KBDC in NIS and how to measure them. On the other hand, issues related to the influence of KBDC in NIS on the achievement of the national SDGs—as well as the differences in the impact of KBDC in NIS on the achievement of SDGs at different economic development stages—are rarely considered. Therefore, this study explores the relationship between KBDC in NIS and the achievement of SDGs by combining research in the field of knowledge-related resources with literature on dynamic capabilities, thereby expanding the understanding of this topic. At the same time, empirical testing of the relationship between the compositional dimensions of KBDC and the achievement of the SDGs enhances the understanding of SDG's relationship with knowledge capabilities, supporting future quantitative analyses of this topic. Furthermore, this study offers an assessment of the impact of KBDC on the achievement of national SDGs at different stages of economic development.
From a management perspective, this study emphasizes the role and impact of KBDC in NIS on the achievement of SDGs, implying that knowledge management contributes to SDG achievement. The results suggest that governments should accelerate the construction of knowledge-based innovation ecosystems. In particular, governments should develop a knowledge ecosystem for sustainable development, encourage the participation of multiple forces, and incentivize all stakeholders to commit to the change process required for sustainable development. Considering the dynamic, complex, and unpredictable characteristics of innovation and sustainable development, the relevant departments should fully rely on digital technology and methods. This approach will allow them to identify and absorb knowledge from different sources, promote the rational transfer and flow of knowledge, and build digitalization into an important tool of national knowledge productivity. At the same time, governments should continuously cultivate and develop the necessary dynamic capacity through the formulation of strong and mission-oriented systematic policies and measures. Moreover, they should effectively integrate and coordinate individuals, enterprises, and countries to participate in knowledge management while considering the high degree of synergy among the compositional dimensions of KBDC. Such an approach will greatly contribute to the achievement of SDGs.
This study further provides a practical understanding of the knowledge-related capabilities that influence the achievement of SDGs at different stages of economic development. In reality, the classification can also be used to assess knowledge capabilities and the achievement of the SDGs from the perspective of NIS. It can thus determine the ideal knowledge-related capabilities of different income economies, which can be used as a basis to promote the construction and development of such capabilities for economies at different income levels. In particular, developed economies should be more concerned about the impact of knowledge absorption, knowledge creation, and knowledge diffusion on the achievement of SDGs; transition economies should focus on the impact of knowledge diffusion; and developing economies should be more concerned about the role of knowledge impact.
In addition, given the differences in the knowledge capability of economies of different income levels in the process of SDG achievement, it is necessary to strengthen the open cooperation of knowledge among such economies. This includes strengthening exchanges and cooperation in intellectual property laws, regulations, policies, and strategies; encouraging countries to promote the development of intellectual property with the concept of mutual benefit and win–win results; strengthening the sharing and utilization of intellectual property information; and actively promoting the sharing and transfer of knowledge. It also involves promoting exchanges and cooperation among countries to raise public awareness of intellectual property rights, as well as strengthening cooperation in intellectual property personnel training. Especially in the current complex situation, including COVID-19 and hostilities between major countries, there is an urgent need for international cooperation to promote the joint achievement of the SDGs.
Limitations and future research directionsAlthough the findings of this paper provide some implications for theory and practice, it has certain limitations. First, most of the data used in the paper come from second-hand data collected in GII and SDR. In the future, more data sources should be considered to examine the relationship between KBDC in NIS and SDG achievement. Second, further research is necessary to determine whether it is reasonable to rely only on knowledge absorption, knowledge creation, knowledge diffusion, and knowledge impact in the GII framework as the compositional dimensions characterizing KBDC while using SDG indexes to characterize SDG achievement. Future research could include case studies and real-world investigations to further demonstrate the rationality of these variables. Finally, as some of the variables in this study have marginal effects on the achievement of SDGs, future research can further explore these areas in depth and expand knowledge on this topic.
Author's contributionXing Li: conceptualization and writing; Ting Wu: methodology and data curation; Hong-Juan Zhang: supervision; De-Yan Yang: software.
This work was supported by the National Natural Science Foundation of China [No72064003], MOE (Ministry of Education in China) Project of Humanities and Social Science (No.18 YJCZH234), Research Project of Philosophy and Social Sciences of Department of Education of Hubei Province (No.20D017), and High Level Project Plan of Wuhan University of Science and Technology (No.W201804).