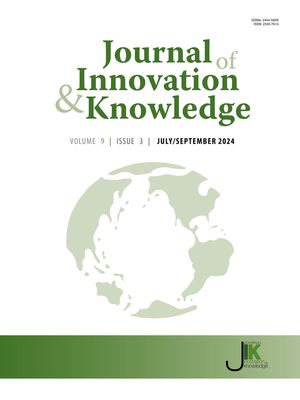
Editado por: Marcin W. Staniewski
Más datosThe development of the sharing economy offers opportunities to reduce urban carbon emissions. Unfortunately, few studies have examined the impact of the sharing economy on urban carbon emissions from a holistic view based on the integration of local and neighborhood hierarchies. This paper examines the impact of the sharing economy on urban carbon emissions from 2011 to 2017 using a sample of 275 prefecture-level cities in China. The results show that the development of personal credit information services and digital finance both contribute to curbing urban carbon emissions. Technological innovation is not only beneficial to urban carbon emission reduction, but also has a negative moderating effect on the relationship between economic development and carbon emissions. Spatial spillover effects of the sharing economy help curb carbon emissions in neighboring cities. Finally, policy recommendations are presented to promote the collaborative economy and reduce urban carbon emissions.
Carbon emission reduction is a long-term strategic task with a high priority for China and the world (Song et al., 2021). It is imperative to significantly reduce carbon emissions or even achieve zero emissions (Xia et al., 2020). A growing number of countries have made commitments to “carbon neutrality”. China's carbon emissions increased from 7.7 billion tons in 2009 to 9.9 billion tons in 2020 (BP, 2021). In 2020, China's share of total global carbon emissions rose to 31%, which is much higher than the second largest share, 13.8%, of the United States (BP, 2021).China has proposed that it will “strive to achieve carbon peak by 2030, and strive to achieve carbon neutrality by 2060”. As China and the global economy enter a new era, the rate of economic growth is slowing down, and there is increasing pressure to maintain a continuous decline in carbon emissions intensity. In this context, it is particularly critical to deeply explore the influencing factors and mechanisms of low-carbon development.
The rapid development of Internet information and mobile application technologies has spawned a new type of economy, namely the sharing economy (Strømmen-Bakhtiar & Vinogradov, 2020). The sharing economy refers to the sum of economic activities that integrate decentralized idle resources and meet the diverse needs of users based on the separation of use and ownership rights by relying on Internet information and mobile application technologies (Martin et al., 2015; Zhou & Wan, 2021). With the rapid development of sharing platforms, there is a growing interest in restraining carbon emissions through the sharing economy. However, existing studies have mainly focused on analyzing the role of the sharing economy in reconciling the contradictions between economic development and energy conservation as well as emission reduction within the framework of shared socioeconomic pathways (Fujimori et al., 2013; Peng et al., 2022; Yang et al., 2018). There is a lack of in-depth research on how components of the sharing economy affect energy conservation and carbon emission reduction, and there is little evidence to test the impact of the sharing platform itself.
The sharing economy can be divided into different categories (Verboven & Vanherck, 2016), which are inseparable from Internet platforms, mobile applications and digital finance (Munkoe, 2017; Zhou & Wan, 2021), and the key to its rapid development lies in the social trust mechanism (Moriuchi, 2020; Stemler, 2017). For example, in China, Alibaba Group's Sesame Credit and Alipay have provided the basic support for the development of the sharing economy. As an Internet-based social credit mechanism, Sesame Credit objectively presents the credit status of individuals and companies through cloud computing, machine learning, and other technologies (McDonald & Dan, 2021). From financial leasing, to shared travel, shared space, shared food, shared medical care, and shared knowledge and education, Sesame Credit has provided users and merchants with convenient credit services in hundreds of sharing economy scenarios (Zhang & Rieckmann, 2018). Sesame Credit realizes paperless inquiries of personal credit information, which directly reduces the felling of trees driven by paper consumption and consumes less energy to accomplish the same work. As a result, it greatly contributes to the process of energy saving and carbon emission reduction in the sharing economy (Chong, 2019).Although components of the sharing economy such as personal credit mechanisms and digital finance play a key role in energy conservation, their striking effect in curbing carbon emissions has been systematically ignored.
In recent years, several studies have affirmed the positive role of the sharing economy in curbing carbon emissions (Zhu et al., 2018). The sharing economy can reduce carbon emissions via green delivery-pickup (Asghari & Al-E-Hashem, 2020). In Brazil, e-carsharing reduces carbon emissions by 29% compared to a business-as-usual scenario (Luna et al., 2020). In Beijing, China, a dockless bike-sharing system has mitigated carbon emissions (Ding et al., 2020). In 2016, bike sharing in Shanghai decreased carbon emissions by 25,240 tons (Zhang & Mi, 2018). Even in household chores, the sharing of laundry cleaning helps reduce carbon emissions in households (Lackner et al., 2020). However, there is also evidence that the development of digital technologies related to the sharing economy involves large-scale data generation, transmission and processing, which increases the energy consumption during the operation of the sharing economy and makes the level of carbon emissions of the digital industry even comparable to that of the aviation industry (Nicola, 2018). Therefore, research on the sharing economy inevitably involves energy efficiency issues. The few existing studies on the energy efficiency of the sharing economy are concentrated in developed countries (Dabbous & Tarhini, 2020; Pucheanu et al., 2020). At present, there is no research in this aspect in developing countries, which is obviously not commensurate with the global carbon emission pattern, where developing countries dominate carbon emissions. Theoretical research clearly lags behind social practice and cannot meet the needs of developing countries to achieve carbon neutrality.
Exploring the energy efficiency of the sharing economy requires technological innovation. The sharing economy is an economic form realized through a sharing platform based on cloud computing, big data, and Internet technology, which is itself a product of technological innovation (Abhari et al., 2019). Technological innovation is an important way to connect the sharing economy and energy efficiency (Hou et al., 2021). Technological innovation in the sharing economy is an effective way to curb carbon emissions (Liu et al., 2021). However, there is still a lack of research examining the impact of sharing economy-related technologies on reducing carbon emissions from the perspective of urban innovation. The moderating role of technological innovation has been confirmed in vehicle carbon emissions (Zhao et al., 2021). However, more important from an energy efficiency perspective is the moderating role of technological innovation in the relationship between economic growth and carbon emissions. Empirical evidence in this regard is currently very sparse.
The existing literature has paid attention to the spatial spillover effects of carbon emissions (Li et al., 2018; Ren et al., 2019). In sharing economy research, although a few recent publications have begun to note the spatial spillover phenomenon of the sharing economy (Gao et al., 2022; Önder et al., 2018), most studies still assume regional or city independence in the development of the sharing economy. In fact, not only does the development of the local sharing economy have an impact on local carbon emissions, but also the development of the sharing economy in neighboring regions or cities. In theory, through the mechanism of spatial spillovers, neighboring regions or cities can form an organic whole to develop the sharing economy and control carbon emissions. However, in reality, is there a spatial spillover effect of the development of sharing economy on carbon emissions of neighboring countries or regions? Little is known so far.
To compensate for the above deficiencies, this study aims to explore the impact of the sharing economy and technological innovation on urban carbon emissions from a holistic perspective based on an integrated local and neighborhood hierarch. This paper contributes to the literature in the following three aspects: first, most of the existing literature focuses on a particular industry or sector of the sharing economy, while this study examines the impact of two core components of the sharing economy (social trust and digital finance) on urban carbon emissions, contributing to a systematic understanding of the role of the sharing economy on carbon emission reduction at a holistic level. Second, unlike the traditional energy efficiency perspective of the sharing economy, this study adopts a new perspective, which is to explore the negative moderating effect of technological innovation on the relationship between economic growth and carbon emissions. This can effectively make up for the insufficiency of traditional perspectives and expand the research related to the sharing economy. Third, this paper finds that there is a negative spatial spillover effect of the sharing economy on carbon emissions between neighboring cities, which provides a new perspective on how the government implements regional carbon emission reduction policies in the context of the sharing economy.
Here, panel data for 275 prefecture-level cities in China from 2011 to 2017 are used. Chinese cities are suitable for this study mainly for the following reasons: first, the sharing economy in Chinese cities is relatively developed. According to the “China Sharing Economy Development Report (2021)” officially released by the State Information Center of China, the market transaction value of the sharing economy was about 3.38 billion yuan in 2020, accounting for more than 3% of China's GDP. The main body of the sharing economy lies in the city. Second, cities are the main battleground for controlling carbon emissions. In China, urban carbon emissions account for nearly 80% of the national carbon emissions (Cai et al., 2019). Cities are home to more than half of the world's population and account for about 70% of the world's energy-related greenhouse gases (Baeumler et al., 2012; Morrison & Maxim, 2021). Therefore, this study has important reference value for both developing and developed countries and is of great significance in promoting global carbon emission reduction.
The following contents of this paper are structured as follows. Section 2 presents the literature review and hypothesis development. Section 3 provides the data, variables, and methods. The empirical results are provided in Section 4. Section 5 contains the discussion. The final section concludes the paper.
Literature review, hypothesis development and analytical frameworkThe sharing economy is a process in which buyers and sellers share any underutilized assets through trust-based peer-to-peer transactions (Huurne et al., 2017). Trust is the key to making the sharing economy possible (Moriuchi, 2020). In peer-to-peer (P2P) accommodation markets, building social trust is the key to success (Zhang et al., 2018). Airbnb has realized that “trust is what makes Airbnb work” and has established a series of trust mechanisms over the years (Ert & Fleischer, 2019). Trust can significantly influence the likelihood of re-using Airbnb (Möhlmann, 2015). Conversely, distrust towards hosts and the Airbnb platform has deterred some American and Finnish travelers from using P2P accommodation (Tussyadiah & Pesonen, 2018). In the sharing economy, problems such as malicious damage to shared bicycles and damage to short-term rental housing facilities have emerged from time to time (Yin et al., 2016). Trust is the premise of sharing behavior among individuals, which in turn affects the development of sharing activities and platforms (Ye et al., 2018). In the P2P lending market, trust is a prerequisite for participating in the sharing economy (Septiani et al., 2020). Digital identity, which is used to solve the credit problem, is increasingly widely used in the sharing economy (Zloteanu et al., 2018).
In China, the official personal credit system is the personal credit information service platform of the People's Bank of China (Jie, 2006). However, this credit reporting system does not involve commercial or personal credit among customers. As the sharing economy flourishes and people's consumption behavior becomes more and more diversified, third-party credit reporting agencies, such as Sesame Credit, have emerged with required time. The third-party credit reporting agency is rapidly connecting to the sharing economy platform and becoming an essential choice for sharing services. According to the “National Urban Credit Deposit-Free Service Report”, in 2017, 381 cities in China have opened deposit-free services, and the deposit-free model has penetrated 8 major industries covering nearly 20 million people (Alibaba, 2017). Users can use these shared facilities without paying a deposit as long as they have a good personal credit record. Personal credit information services have become a new engine for the development of the urban sharing economy.
The personal credit information service in this study refers specifically to the services of Internet-based third-party credit reporting agency such as Sesame Credit. Such personal credit information services are directly connected to various sharing platforms such as shared spaces, shared vacations, shared games, shared work platforms, shared funds, shared meals, etc., and have become an integral part of the sharing economy (McDonald & Dan, 2021; Zhang & Rieckmann, 2018). This kind of personal credit information business can improve energy efficiency and reduce carbon emissions in the following aspects: first, it can effectively reduce the harm of bad credit issues to the interests of sharing economic entities, promote the reuse of shared idle resources, and make full use of idle resources and waste reduction (Li et al., 2021; Tussyadiah & Pesonen, 2018). Second, it uses big data technology to evaluate personal credit and accurately predict personal behavior, which can significantly reduce search costs in the sharing economy, as well as search-related energy consumption and carbon emissions (Peng & Zhu, 2021). Third, it can effectively and timely prevent the risk of personal loan default and breach of trust, thus avoiding additional energy consumption and carbon emissions caused in the process of pursuing personal loan default or breach of trust (Wang et al., 2022). Finally, it provides a paperless personal credit service, which can reduce the use of on-site meeting rooms, reduce water and electricity consumption, and help reduce carbon emissions (Chong, 2019). Therefore, the following hypothesis is obtained:
Hypothesis 1(H1) : The development of Internet-based third-party personal credit service is negatively correlated with carbon emissions.
Digital finance such as online credit, mobile payment, digital crowdfunding, e-commerce supply chain finance, etc. has a multidimensional and compound impact on economic performance (Jiang et al., 2021). The development of the sharing economy has promoted the digital transformation of the P2P transaction system (Strømmen-Bakhtiar & Vinogradov, 2020), enabling the rapid rise of digital finance (Zhou & Wan, 2021). Cashless digital payments and mobile wallets have become a standard configuration for P2P transactions in the sharing economy (Gupta et al., 2020). Mobile payment shortens the distance between users and services in the sharing economy (Li et al., 2021). At the same time, mobile payment not only ensures the safety of users' property, but also makes micropayments easier (Pfeiffer, 2019).
Digital finance has a significant inhibitory effect on carbon emissions (Zhao et al., 2021). The development of digital finance avoids the practice of continuously building physical outlets resulting in a large amount of carbon emissions (Polzin, 2017). For individual consumers, digital finance can reduce carbon emissions generated by offline participation in these services through behaviors such as online life payment, online traffic ticket payment, online registration and ticket purchase (Zhao et al., 2021). The development of digital finance is conducive to broadening the channels of public participation in environmental protection, and to deepening public participation in environmental protection through digital finance platforms, thus significantly improving the public's energy-saving and low-carbon lifestyles (Li et al., 2020). A typical example is the “Ant Forest” project launched by Alipay through the establishment of personal “Carbon Accounts”, which concretizes carbon emission reduction behavior into a certain amount of energy to encourage daily low-carbon behavior (Guo & Lo, 2019). In 2017, over two hundred million consumers opened the “Ant Forest” on the Alipay platform, thus saving 2500 tons of carbon emissions per day (Zhang et al., 2018). For small and micro enterprises, digital finance can improve the efficiency of financial services, reduce borrowing costs, and also reduce carbon emissions generated by small and micro enterprises entering and leaving financial institutions (Cao & Zhang, 2021).
In addition, digital finance also reduces carbon emissions by improving energy efficiency and reducing energy intensity. First, financial institutions can select enterprises through digital financial platforms, eliminate high energy-consuming and high-polluting enterprises, and guide the flow of financial resources to energy-saving and low-carbon enterprises, thus effectively improving energy efficiency and reducing carbon emissions (Wang et al., 2022). Second, with the development of digital technologies and accurate customer identification technologies, digital finance can help financial institutions prioritize loans to enterprises that use green energy-saving technologies, which not only realizes the upgrading and optimization of energy consumption systems, but also optimizes the allocation of energy resources, thereby reducing carbon emissions while improving energy efficiency (Cao et al., 2021). The digital financial platform can conduct big data mining of enterprise information and provide a decision-making basis for precise government regulation, which can effectively reduce the government's information search cost and promote carbon emission reduction (Gomber et al., 2017; Zhao & He, 2022).
All these studies show that the development of digital finance contributes to energy efficiency and has an inhibitory effect on carbon emissions. Therefore, the following hypothesis is obtained:
Hypothesis 2(H2) : The development of digital financial services is negatively correlated with carbon emissions.
In the sharing economy, the rapid development of Internet technology of openness, equality, collaboration, and sharing provides new opportunities for leapfrogging technological innovation (Shaikh et al., 2021). The development of big data, cloud computing and mobile intelligence makes knowledge sharing and dissemination increasingly fast, which undoubtedly accelerates technological innovation (Min et al., 2019). In the era of sharing economy, the elemental system of technological innovation mode includes innovation subjects, shared platforms, innovation resources and elemental integration mechanisms (Abhari et al., 2019). The new model of technological innovation in the sharing economy has bred a lot of grassroots social innovation (Martin et al., 2015).
In the sharing economy, the importance of technological innovation in reducing carbon emissions has become increasingly evident. Technological innovation has a positive role in reducing carbon emissions (Yang et al., 2014). Empirical evidence from Malaysia suggests that technological innovation can help reduce carbon emissions in the long run (Ali et al., 2016). Technological innovation has effectively contributed to energy saving and carbon emissions reduction in China (Zhang et al., 2020). In the sharing economy, commerce and industry leaders such as Wal-Mart and Huawei are also committed to technological innovation to save energy and reduce emissions in order to contribute to building a low-carbon society (Mao et al., 2016; Zu et al., 2017). Technological innovation in P2P energy exchange can effectively reduce carbon emissions (Liu et al., 2021). The sharing economy has spawned the technological innovation of distributed on-site PV generation, which makes traditional consumers active participants in a decentralized energy system that can effectively save electricity and reduce carbon emissions (Liu et al., 2021).
In addition, technological innovation can reduce carbon emissions by improving energy efficiency. First, knowledge sharing in the sharing economy contributes to technological innovation of enterprises, while innovation is an important way to improve energy efficiency and reduce carbon emissions (Cheng et al., 2021; Khalid et al., 2018; Zheng, 2021). Secondly, the development of the sharing economy has given rise to the innovation of energy internet technologies characterized by the in-depth integration of new energy technologies and information technology, which is considered to be the key to solving the problems of fossil energy shortage and environmental pollution (Weng et al., 2018). Finally, the development of the sharing economy facilitates the construction of energy digitalization, making it easier to trade energy across time and space, thus improving the optimal allocation and use efficiency of energy (Loseva et al., 2020).
This evidence shows that technological innovation in the sharing economy remains an important way to curb carbon emissions. Therefore, the following hypothesis is obtained:
Hypothesis 3(H3) : Technological innovation is negatively correlated with carbon emissions.
The relationship between economic growth and carbon emissions has been increasingly debated since Grossman and Krueger (1993) proposed the Environmental Kuznets Curve (EKC) hypothesis (Roberts & Grimes, 1997). Under the premise of constant energy efficiency, economic growth is positively related to carbon emissions. However, technological innovation can improve energy efficiency (Sun et al., 2021). Therefore, even if the economy continues to grow, it is likely that carbon emissions will decline (Sun et al., 2018). There are also many studies that show the existence of a more complex relation between GDP and carbon emissions compared to the EKC curve (Lin et al., 2017; Wang & Zhao, 2017). In the relationship between GDP and carbon emissions, technological innovation often plays an important role that cannot be ignored. Technological innovation plays a moderating role in the relationship between capital allocation efficiency and vehicle carbon emissions (Zhao et al., 2021). Technological innovations related to the sharing economy, such as cloud computing, big data, artificial intelligence, digitization, and other technologies, can significantly improve energy efficiency, thus promoting economic development while reducing carbon emissions (Zhang & Li, 2020).
In the context of relatively low technological capacity, economic development relies mainly on high energy-consuming and high-emission industries (Bai & Yang, 2012). Therefore, economic development can aggravate carbon emissions (Guo et al., 2016). However, technological innovation can play a moderating role between economic development and carbon emissions. On the supply side, technological innovation can accelerate the exit of backward, energy-intensive, and carbon-emission production capacity (Hasanov et al., 2021). Technological innovations represented by big data, artificial intelligence, and blockchain, which are directly related to the sharing platform, guide more high-quality production factors to flow to more environmentally friendly industries, while restraining the flow of production factors to low-end, energy-intensive, and high-carbon emission industries, thus improving energy efficiency and controlling carbon emissions (Cao et al., 2021; Plewnia, 2019). On the demand side, with the in-depth implementation of the concept of sharing economy and low-carbon development, the consumer market's demand for high-energy-consuming and high-carbon-emission industrial production continues to decline, forcing companies to adopt initiatives for expanding their energy-saving and low-carbon production through technological innovation (Lombardi & Schwabe, 2017; Qi et al., 2017). Therefore, technological innovation can simultaneously achieve economic quality improvement and energy efficiency improvement, thereby controlling carbon emissions. As a result, economic development aggravates carbon emissions when the capacity for technological innovation is relatively weak and suppresses carbon emissions when the capacity for technological innovation is relatively strong. Therefore, the following hypotheses are obtained:
Hypothesis 4a(H4a) : Economic growth is positively correlated with carbon emissions.
Hypothesis 4b(H4b) : Technological innovation will negatively moderate the impact of economic growth on carbon emissions.
Carbon emissions in one region usually have an impact on carbon emissions in neighboring regions (Cui et al., 2019). Factors that drive spillover of carbon emissions to neighboring regions include economic growth (Zhou et al., 2019), industrial development (Li et al., 2018), technology transfer (Qi et al., 2019), and urbanization (Shahbnzi et al., 2015). It can be seen that there is a strategic interaction between neighboring regions in terms of carbon emissions (Lu et al., 2021). As a result of strategic interaction, carbon emissions in neighboring regions tend to be the same or similar, resulting in spatial convergence of carbon emissions (Huang et al., 2019). Regions adjacent to regions with higher carbon emissions will also have higher carbon emissions, and vice versa.
In fact, the sharing economy also generates spatial spillover effects. For example, peer-to-peer accommodation and dockless bike sharing have significant spatial spillover effects (Gao et al., 2022; Gutierrez et al., 2017). Therefore, it can also be speculated that Internet-based third-party personal credit services and digital finance, which are directly related to the sharing economy, will also generate spatial spillover effects. For example, in the field of digital finance, the positive spatial spillover effect is obvious (Jiang et al., 2022; Lin & Zhang, 2022). Since the development of Internet-based third-party personal credit services is very similar to that of digital finance, it is reasonable to infer that the development of Internet-based third-party personal credit services will also generate a positive spatial spillover effect. When a city's internet-based third-party personal credit services and digital finance develop, they will soon spill over to neighboring cities, thus promoting the development of internet-based third-party personal credit services and digital finance in neighboring cities. This will promote the development of sharing economy in neighboring cities, thereby curbing carbon emissions in neighboring cities. Therefore, it can be inferred that the development of Internet-based third-party personal credit services and digital finance will have a negative spatial spillover effect on the carbon emissions of neighboring cities.
Therefore, the following hypotheses are obtained:
Hypothesis 5a(H5a) : There is a positive spillover effect of carbon emissions.
Hypothesis 5b(H5b) : The development of Internet-based third-party personal credit services will have negative spatial spillover effects on carbon emissions in neighboring cities.
Hypothesis 5c(H5c) : The development of digital finance will have negative spatial spillover effects on carbon emissions in neighboring cities.
The analytical framework for understanding the determining mechanism of carbon emissions in the sharing economy is illustrated in Figure 1.
As shown in Figure 1, this study will examine the impact of the sharing economy on carbon emissions at both the local level and the neighborhood level. Therefore, this study is based on an integrated local and neighborhood hierarchy from a holistic perspective and is an emerging field that has so far received relatively little attention from scholars.
Data, variables and empirical strategiesDataThe carbon emissions of prefecture-level cities have always been the focus of academic attention (Chen et al., 2021; Wan et al., 2022). The research objects of this study are prefecture-level cities in China, with a total number of 275 prefecture-level cities in the sample. The time span is from 2011 to 2017. Therefore, the total number of observations is 1925. The data were obtained from the statistical yearbooks of Chinese cities over the years.
Variables and measurementDependent variablesRegarding the measurement of carbon emissions, the National Geophysical Data Center (NGDC) used two sets of nighttime light data (DMSP/OLS and NPP/VIIRS data) to measure CO2 emissions from Chinese prefecture-level cities prior to 2018 (Chen et al., 2021). This data is the most comprehensive and authoritative dataset of urban carbon emissions that has been repeatedly peer-reviewed and cross-validated. Therefore, this study also uses this indicator as the dependent variable (Carbon), and is expressed in millions of tons.
Independent variablesIn the measurement of the sharing economy, it is generally done from the perspective of the connection between the sharing platform and the Internet, since the sharing economy is generally realized through the Internet (Rivares et al., 2019). For example, Dabbous and Tarhini (2020) built a proxy measure for the development of shared platforms based on their popularity as keywords in the Google Trends search engine. However, this indirect measurement is problematic because people who search for sharing platforms on Google are not necessarily those who participate in the sharing economy. The mistakes of the Google Trends approach have been exposed in the field of public health. Big data hubris and algorithmic dynamics contributed to the ultimate failure of Google Flu Trends (Lazer et al., 2014). This study directly measures the development of the sharing economy in terms of two indispensable components of the sharing platform: Internet-based third-party personal credit services and digital financial services. Both are standard configurations on today's shared platforms. In the sharing economy, services such as deposit-free and enjoy first and pay later were all based on Internet-based third-party personal credit services (Li et al., 2021; McDonald & Dan, 2021; Wang et al., 2022; Zhang & Rieckmann, 2018). Transaction payments in the sharing economy were basically realized through digital finance (Gomber et al., 2017; Jiang et al., 2022; McDonald & Dan, 2021). Therefore, it is more direct and accurate to measure the development of the sharing economy from these two dimensions than from Google Trends.
Internet-based third-party personal credit services were measured by the cities personal credit service index (Credit) which was constructed based on two indicators: the number of personal credit information calls per capita and the number of people using personal credit services (including finance, accommodation, travel, social networking, etc.) per 10,000 Alipay users. The digital financial index (Digit) was constructed based on four indicators: the proportion of mobile payment transactions, the proportion of mobile payment amount, the average loan interest rate for small and micro business operators, and the average loan interest rate for individuals. Both indices were derived from the digital financial inclusion index developed by Peking University, and widely used in economics research (Li et al., 2020). In constructing the above two indices, the indices with different properties and measurement units were processed in a dimensionless manner. The above two indices were compiled with a range of data efficiency scores from 0 to 100 points for each indicator, based on the cities in 2011. The higher the score, the higher the development level of the corresponding indicator. For data from years after 2011, the efficacy score of the indicator may be less than 0 or greater than 100, which reflects the decrease or increase of the indicator value relative to 2011 in each region. Technological innovation is measured by the number of patents granted by the city (Patent), and the unit is in thousands. GDP (GDP) is a measure of a city's overall economic strength, expressed in 100 billion yuan.
In addition, several other independent variables were included in this study. Sulfur dioxide and nitrogen oxides from the combustion of industrial fossil fuels constitute one of the major sources of air pollution (Zhang et al., 2015). Emissions of sulfur dioxide and nitrogen oxides are inversely related to energy efficiency (Hu et al., 2019). Therefore, the two variables of industrial sulfur dioxide emissions (Sulfur) and industrial nitrogen oxide emissions (Nitrogen) are introduced here, and their units are 10,000 tons. Population growth is an important factor in increasing carbon emissions (Dong et al., 2018; Ypersele & Bartiaux, 1995). The total population at the end of the year (Population) is also introduced into the model as an explanatory variable, with units of 10,000 persons. Carbon emissions were dominated by the secondary industry (Wang & Yang, 2015). As a result, the percentage of employees in the secondary industry (Industry) was also introduced in the model. The sample sizes and descriptive statistics of these variables are shown in Table 1. Definitions of these variables are summarized in Appendix 1. The correlation coefficients between these variables can be found in Appendix 2.
Sample size and descriptive statistics of variables
Table 2 summarizes the mean values of these variables in different years. As seen in Table 2, carbon emissions showed an overall upward trend from 2011 to 2017. This shows that the rapid growth of carbon emissions of Chinese cities has been effectively controlled, but carbon reduction still needs to be stepped up to achieve the carbon emission peak as soon as possible. During the same period, the personal credit business and digital finance grew rapidly. This is due to the rapid development of China's sharing economy and digital economy. The number of patent grants has been growing steadily and slightly, and GDP has shown a clear and continuous growth trend. The patent policy has achieved a transformation from pursuing quantity to improving quality, and innovation has provided strong development momentum for economic growth. Emissions of sulfur dioxide are on a continuous downward trend, while emissions of nitrogen oxides are still on the rise. The population is increasing, but the percentage of employees in the secondary industry is showing signs of decline.
Means of variables in different years
Considering the spatial spillover effect of carbon emissions, the spatial lag models are first used here for empirical testing. The spatial lag models have been shown to be a good tool for examining spatial spillovers (Gu, 2021; Lambert et al., 2010). The models are as follows:
The empirical regression results may vary due to different spatial regression model settings. Therefore, it is necessary to judge the robustness of the empirical results by changing the settings of the spatial regression model. To ensure the robustness of the empirical model, a regression was also performed on two sets of spatial error models. The specific spatial error models are defined as follows:
W is the constructed spatial weight matrix. Here, the spatial weight is defined by the proximity relationship between cities. If two cities are adjacent, their spatial weight is 1; if two cities are not adjacent, their spatial weight is 0.ResultsThe regression results are shown in Table 3. Models 1, 2, 3, and 4 in Table 3 correspond to Formulas (1), (2), (3), and (4), respectively. In these regression analyses, a fixed-effects model and a random-effects model were performed for each model, and the corresponding Hausman test is also reported in Table 3. The Hausman test was insignificant, indicating that the random-effects model outperforms the fixed-effects model. Therefore, only the regression results for the random effects model are reported in Table 3. The year and city effects are fixed in all four models. The empirical regression results are reported and summarized in Table 3.
Results of empirical estimation
Note: *, **, and *** are statistically significant at 0.1, 0.05, and 0.001, respectively.
According to Table 3, the regression coefficients of the Credit variable are negative and significant in all four models. This shows that the development of personal credit business in cities associated with the sharing economy helps to curb carbon emissions. Thus, Hypothesis 1 is confirmed here. In cities, credit-based life services such as shared finance, shared accommodation, shared travel, shared social networking, and shared goods are important components of the sharing economy. In this sharing process, an increasing reliance is placed on digital identities based on personal credit information services (Zloteanu et al., 2018). This can effectively reduce the city's carbon emissions. In Table 3, the regression coefficients of the Digit variable are negative and significant in all four models. It can be seen that the development of digital finance is also an effective way to curb urban carbon emissions. Thus, Hypothesis 2 is confirmed here. The development of digital finance can reduce the carbon emissions generated by reducing offline participation in these services (Zhao et al., 2021).
In Table 3, the regression coefficients of the Patent variable are negative and significant in all four models. It is clear that technological innovation contributes to the reduction of carbon emissions. Thus, Hypothesis 3 is confirmed here. In the long run, technological innovation definitely contributes to the reduction of carbon emissions (Ali et al., 2016). Moreover, the role of technological innovation in reducing carbon emissions does not stop there. In Models 1 and 3, the regression coefficients for GDP are both positive and significant. However, after adding the interaction variable of Patent and GDP, the regression coefficients of GDP become both negative and significant, as shown in Models 2 and 4. According to Models 2 and 4, the impact of economic development on carbon emissions is significantly positive after separating the moderating effect of technological innovation. Thus, Hypothesis 4a is confirmed here. Meanwhile, the regression coefficient of the interaction term between the Patent variable and the GDP variable is significantly negative. According to the empirical test method of the moderating mechanism (Ehigiamusoe et al., 2020), this illustrates the moderating role of technological innovation in the carbon emissions-economic development nexus of the sharing economy. Thus, Hypothesis 4b is confirmed here. A previous study (Zhao et al., 2021) also found this moderating mechanism of technological innovation. The present study goes a step further and finds a negative moderating effect within the framework of the sharing economy.
The regression coefficients of the spatial lag variable ρ are positive and significant in both Models 1 and 2. Similarly, the regression coefficients of the spatial error variable γ are positive and significant in both Models 3 and 4. According to the empirical test method of spatial spillover (Anselin et al., 2004; Sage & Pace, 2009), this shows that there is a positive spatial spillover effect of carbon emissions. Thus, Hypothesis 5a is confirmed here. Carbon emissions will spread to neighboring cities (Cui et al., 2019). In addition, Table 3 shows that the proportion of population and employees in the secondary industry increases urban carbon emissions, which is similar to industrial sulfur dioxide emissions or industrial nitrogen oxide emissions.
In the robustness test, the results of the spatial error models in Table 3 are consistent with the results of the corresponding spatial lag model. Model 3 echoes the results of Model 1, while Model 4 echoes the results of Model 2. This suggests that the results of this study do not alter the spatial model settings due to changes in the spatial model settings. Therefore, it has good robustness.
In addition, to further test the robustness and examine the impact of sharing economy development on carbon emissions in the neighboring cities, here the spatial lag terms of the dependent variable are replaced by the spatial lag terms of Credit and Digit in formula (2), respectively, and the results are summarized in Table 4.
Results of empirical estimation with spatial lag independent variable
Note: *, **, and *** are statistically significant at 0.1, 0.05, and 0.001, respectively.
Except for the regression coefficient of the spatial lag term, the regression results of Models 5 and 6 in Table 4 are consistent with the regression results of Model 2 in Table 3, again indicating the good robustness of the results of this study. The regression coefficients of the Credit and Digit spatial lag terms are both significantly negative, which indicates that the city's development of personal credit information services and digital finance will spill over to neighboring cities and promote the development of the sharing economy in neighboring cities, thereby effectively inhibiting carbon emissions in neighboring cities. Thus, Hypothesis 5b and Hypothesis 5c are confirmed here.
Regarding the endogeneity problem, the results are robust after controlling for observable characteristics at the city level and various unobservable characteristics such as time and city fixed effects. However, even if these strategies were used to handle the empirical problem of potential endogeneity, endogeneity might still be a problem due to reverse causality.
DiscussionThis study provides theoretical implications for different streams of literature. First, the findings in this paper contribute to the sharing economy literature based on previous evidence from ride‑hailing (Zhu et al., 2018), bike-sharing (Ding et al., 2020; Luna et al., 2020; Zhang & Mi, 2018), sharing of household chores (Lackner et al., 2020), and sharing delivery-pickup (Asghari & Al-E-Hashem, 2020). This study takes those studies a step further and explores the impact of the sharing economy on carbon emissions from two essential components of a sharing platform: personal credit services and digital finance. No kind of sharing economy can be separated from social trust mechanism (Moriuchi, 2020; Stemler, 2017) and digital finance (Munkoe, 2017; Zhou & Wan, 2021). This study has found that the development of both personal credit services and digital finance can help reduce urban carbon emissions. Hence, this paper sheds light on the importance of properly promoting Internet-based third-party personal credit services and digital financial services to develop the sharing economy and curb carbon emissions.
Second, this paper contributes to the literature on energy efficiency by focusing on the moderating role of technological innovation in the relationship between economic development and carbon emissions. Issues of the sharing economy and energy efficiency have attracted widespread attention (Hou et al., 2021). A very important reason for this benign situation is that technological innovation improves energy efficiency in the operation of the sharing economy (Filipovi et al., 2019; Lombardi & Schwabe, 2017; Tietze, 2020). This study found that technological innovation not only helps to reduce urban carbon emissions (Liu et al., 2021; Yang et al., 2014), but also has a negative moderating effect on the relationship between economic development and carbon emissions. In the case of a relatively strong technological innovation capability, carbon emission reduction can also be achieved in parallel with economic development. Therefore, this study provides a better understanding of the role of technological innovation in the economic development-carbon emission nexus, thus extending the study of energy efficiency in the sharing economy.
Finally, this study also found that factors at the neighborhood level affect carbon emissions. Carbon emissions will spread to neighboring cities (Cui et al., 2019). This shift in carbon emissions suggests a strategic interaction between neighboring cities (Lu et al., 2021). This leads to a spatial accumulation of carbon emissions (Huang et al., 2019). Of more concern than the spatial spillover effects of carbon emissions is that the sharing economy also has a spatial spillover effect. Moran's I test is a commonly used method to detect spatial spillover effects (Bai et al., 2022). Moran's I analysis was performed separately for Credit and Digit, and the results are summarized in Table 5.
Results of spatial autocorrelation analysis
Note: **, and *** are statistically significant at 0.05, and 0.001, respectively.
According to Table 5, the Moran's I values for Credit and Digit have been positive and significant for many years. This shows that the development of a city's personal credit services and digital financial services will soon spill over to neighboring cities, which in turn will promote the development of neighboring cities in these two aspects and contribute to the development of the sharing economy in neighboring cities. The development of the sharing economy helps curb carbon emissions as it does so. Therefore, the spatial spillover of personal credit services and digital financial services to neighboring cities contributes to the control and reduction of carbon emissions in neighboring cities. Indeed, this study reveals the spatial spillover effects of the sharing economy and the impact of neighborhood-level factors on carbon emissions, which is an emerging field that has so far received relatively little attention from scholars.
In addition to theoretical implications, this study has important practical implications. China has certain characteristics in developing sharing economy and controlling carbon emissions. However, we can generalize this evidence to other countries because these problems are also commonly faced by developing and developed countries. To reduce carbon emissions from cities, approximately 1050 cities in the United States, 40 cities in India, 100 cities in China, and 83 cities in Japan have reportedly established low-carbon development goals (Su et al., 2013). However, how to reduce urban carbon emissions as a whole remains a common challenge facing cities around the world. This study provides an important sustainable pathway for reducing urban carbon emissions through the development of a sharing economy. A cross-city and cross-regional coordination mechanism is necessary to develop the urban sharing economy and curb urban carbon emissions. In terms of specific measures, it is important to consider not only the suppressive effect of the sharing economy on carbon emissions in individual cities, but also the impact on carbon emission reduction in neighboring cities. The neighboring cities should share effective energy-saving and emission-reduction measures, jointly promote the sharing economy, establish a unified carbon emission reduction governance mechanism, and form a regional carbon emission synergy effect. These experiences are not only useful for developing countries, but also have important reference value for developed countries to achieve carbon neutrality. Therefore, the results of this study and policy recommendations are applicable to other countries.
Although this study has achieved the above valuable research results, there are also limitations. First, there is a lack of data on carbon emissions from 2017 onward. This study may be updated if NGDC publishes data on urban carbon emissions beyond 2017. Second, this study explores the impact of Internet-based third-party personal credit services and digital financial services closely related to sharing platforms on carbon emissions, but does not explore the specific path of action. In the future, appropriate mediating variables can be introduced to further explore the impact paths of the sharing economy on carbon emissions. Finally, this study explores the impact of energy efficiency on carbon emissions through the moderating role of technological innovation. In the future, energy efficiency in the sharing economy can be first calculated by data envelopment analysis (DEA), and then the direct impact of energy efficiency on carbon emissions can be examined.
ConclusionsUnder the vision of carbon neutrality, the role of cities as the “main position” of carbon emission control will be further highlighted. Based on a panel data of 275 prefecture-level cities in China from 2011 to 2017, this paper empirically examines the impact of the sharing economy on urban carbon emissions. The development of personal credit information business and digital finance, which are directly related to the sharing economy, has been empirically verified to promote the reduction of urban carbon emissions. Technological innovation not only suppresses urban carbon emissions, but also negatively moderates the relationship between economic growth and carbon emissions by improving energy efficiency, so that economic growth does not necessarily come at the expense of carbon emissions. There is a positive spatial spillover effect of carbon emissions between neighboring cities, which shows the importance of regional carbon emission reduction policies. Spatial spillover effects of the sharing economy can help curb carbon emissions in neighboring cities.
Note: **, and *** are statistically significant at 0.05, and 0.001, respectively.