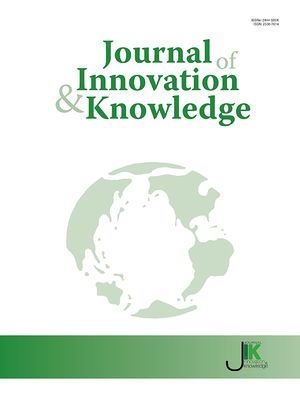
Edited by: Marcin W. Staniewski
More infoThe adoption of the sharing economy by organizations is of central importance to energy efficiency. However, previous studies ignore the role of user adoption of the sharing economy. Therefore, the objective of this study is to investigate the role of energy efficiency through user adoption of the sharing economy to promote environmental sustainability. This objective is achieved using a quantitative research method. Data collection is managed using a survey questionnaire. Area cluster sampling is used for the data collection because this sampling technique is suitable for dealing with a widespread population. The sample size of the study is 178. Scale items taken from previous studies are used to measure the relationships between herd behaviour, perceived usefulness, perceived ease of use, trust, behavioural intention, and environmental sustainability. The results show that energy efficiency through user adoption of the sharing economy is of key importance in the behavioural intention of users. User adoption of the sharing economy has the potential to promote environmental sustainability. The results provide valuable insights for practitioners designing strategies for energy efficiency and the sharing economy.
Over the recent decade, the concept of energy efficiency has grown in importance due to its influential role in environmental sustainability (Mhadhbi et al., 2021; Ponce & Khan, 2021; Zhao et al., 2022). Energy efficiency is the use of less energy to accomplish a task or produce an outcome. Using less energy has the potential to save the environment from various harmful substances, which has a number of benefits, including a reduction in greenhouse gas emissions, a reduction in demand for energy imports, and lower costs for households at an economy-wide level (Chege & Wang, 2020). However, achieving a satisfactory level of energy efficiency is one of the main challenges for nations. The use of less energy to perform specific tasks requires a technology adoption model (Sendawula et al., 2020). The current study proposes that the sharing economy is an influential factor in promoting energy efficiency. Sharing economies are socio-economic systems built around the sharing of resources in the community (Adnan et al., 2021; Alfayad, 2021; Dang et al., 2021; Mouratidis et al., 2021).
The concept of a sharing economy is prevalent in China in relation to energy efficiency (Dabbous & Tarhini, 2021). However, the current study considers a unique aspect of the sharing economy in China, bicycle sharing, which is an important element of a sharing economy, leading to energy efficiency and environmental sustainability. Bicycle-sharing applications are common in China, which decrease energy use, having a significant effect on environmental health (Zheng et al., 2019). Bicycle sharing in China is increasing, and is higher than in the United States of America (USA), United Kingdom (UK), and Brazil, as shown in Fig. 1. A study carried out by Liu and Yang (2018) highlights bicycle-sharing applications in China as part of the sharing economy, but does not address it in relation to environmental sustainability (Al-Abri & Mydin, 2021; Aldoghan, 2021; Alshareef et al., 2021; Fakhri, 2021).
However, user adoption of the sharing economy is still a challenge in China. Although the concept of sharing is important for energy efficiency and environmental sustainability, it requires maximum user adoption. This study explores whether user adoption of the sharing economy can be promoted through behavioural intention (BI), the internal motivation that colours behaviour. The more strongly one intends to engage in a behaviour, the more probable it is that one will do so. BI is discussed along with the sharing economy (Ek Styvén & Mariani, 2020; Olya et al., 2018), but is not reported in relation to energy efficiency in terms of Chinese bicycle-sharing applications. The current study proposes that BI for user adoption in relation to the sharing economy can be promoted through herd behaviour. Herd behaviour occurs when individuals in a group function together without centralized guidance, and has the potential to influence perceived usefulness (PU), (the extent to which a person thinks that utilizing a particular technology or method would improve their ability to perform a task), perceived ease of use (PEU) (the degree to which people believe that using the technology or adopting a system is simple), and trust. Therefore, the roles of PU, PEU, and trust are important in influencing user adoption. Hence, the objective of this study is to investigate the role of energy efficiency through user adoption of the sharing economy to promote environmental sustainability. The results have the potential to enhance strategy to promote energy efficiency through the sharing economy (Aljazzazen & Schmuck, 2021; Alrabadi et al., 2021; Mhmood et al., 2021).
China is a country that emits a huge amount of pollution. The major cause of pollution is the increase in human activity, which requires energy in large amounts. In order to control pollution in the country, many policies and business strategies have been designed, such as the sharing economy and energy efficiency. But the implementation is not yet sufficient to overcome environmental pollution. This study, by analysing the relationships between herd behaviour, PU, PEU, trust, BI, and environmental sustainability in the context of energy efficiency and the sharing economy, clarifies how pollution can be controlled and a sustainable environment be established.
Literature reviewThe study proposes a framework (Fig. 1) to examine energy efficiency through user acceptance of the sharing economy and its role in environmental sustainability (Baradaran Motie & Biolsi, 2021; Bari et al., 2021). User acceptance of the sharing economy comes about through a combination of variables which influence the BI of people (Buturache & Stancu, 2021; Cernikova & Hyblerova, 2021).
Herd behaviourHerding can be described as the phenomenon of individuals determining to follow others or copy group behaviours, as opposed to deciding independently on the grounds of their own private information. Herd behaviour is the behaviour of individuals in a group acting without central direction. Various previous studies consider herd behaviour (Liu et al., 2018; Shi et al., 2022), but not in relation to energy efficiency, the sharing economy, or bicycling-sharing applications in China. There are studies which show that herd behaviour has an influence on PU, PEU, and trust (Akpan et al., 2021; Chen et al., 2021; Duke, 2020; Fabbro & Tonchia, 2021).
According to Davis (1989), PU is the subjective perception of users, through which they believe that using certain technologies can advance performance. Jahmani et al. (2018) examine the relationship between herd behaviour, knowledge content, and PU in a sharing economy. A survey of 442 healthcare establishments in Jordan, and structural equation modelling (SEM) are applied to empirically analyse herd behaviour, knowledge content, and PU in a sharing economy. The study finds that the herd behaviour of an individual has an influence on PU. As herding behaviour is based on the behaviour of the group acting collectively, it has an effect on the perception of the user. Liu and Yang (2018) find subjective norms and imitating others to be the key elements of herd behaviour which influence PU. Other studies show the relationship between herd behaviour and user perception (Kassas & Nayga, 2021; Kim & Petrick, 2021; Luo et al., 2021). Kang et al. (2020) investigate the relationship between herd behaviour and PEU in a sharing economy. Empirical data on herd behaviour and PEU is collected from Korea and analysed using SEM modelling with SPSS and AMOS. Herd behaviour is shown to have an influence on PEU, which is a user's perception of being comfortable with a technology. PEU is very important to the adoption of any new technology. In a sharing economy, any change based on technology requires ease of use, making it very important. Herding behaviour and PEU are positively linked. Qiao et al. (2018) examine the herd behaviour and PEU relationship in a sharing economy, taking evidence from 56 Chinese commercial banks collected through questionnaires. The current study considers PEU is in relation to the adoption of a sharing economy through bicycle-sharing applications in China. The behaviour of people in a group, shapes the behaviour of the individual. Therefore, herd behaviour should positively influence the use of the bicycle-sharing applications. Trust is another important factor which has an influence on individuals (Danilwan et al., 2020), particularly, trust of technology is important for adopting a sharing economy (Armey et al., 2022; Hmelak et al., 2022; Hussain et al., 2022). A lack of trust in any new innovation can decrease its implementation. Therefore, any innovative element used to promote a sharing economy or enhance energy efficiency has to be able to inspire trust. It is the most important element of user acceptance of a sharing economy (Yasa et al., 2022). Trust is linked to herding behaviour, and the literature shows a significant connection (Armansyah, 2021; Vedadi & Greer, 2021). In the context of the current study, trust in the bicycle-sharing application is key to user adoption and promoting the sharing economy in China. The relationship between herd behaviour and trust is the most significant part of the sharing economy. The literature proves a significant association between the herding behaviour of people and trust. Hence, the following hypotheses are proposed:
Hypothesis 1 Herd behaviour has a positive effect on PU.
Hypothesis 2 Herd behaviour has a positive effect on PEU.
Hypothesis 3 Herd behaviour has a positive effect on trust.
Behavioural intention (BI) is a significant factor that has an influence on the adoption of sharing economy interventions. It is the motivational elements that affect a given behaviour, as the higher the intention to engage in the behaviour, the more likely the behaviour is. The BI of users is influenced by herd behaviour (Yi et al., 2020). According to Hwang and Lee (2020), herd behaviour has a major relationship with the intentions of people, and any change in herd behaviour has an effect on BI (Yang et al., 2019). Herd behaviour has a positive effect on shaping the intentions of people towards energy efficiency through a sharing economy (Adamides & Karacapilidis, 2020; Borges et al., 2020).
This discussion shows that herd behaviour influences PU, PEU, and trust, and the previous section highlights that PU, PEU, and trust influence BI. When implementing technology, the usefulness of the technology is most significant, and has an influence on its adoption by users. A higher level of usefulness leads to positive behaviour by people (Shao et al., 2020), and BI has a relationship with user adoption (Peng et al., 2012; Son et al., 2015). Similarly, the ease of use of any technology builds positive behaviour and has a major influence on its adoption. Technology related to bicycle-sharing applications can promote a sharing economy based on the ease of use of the applications (Liu & Yang, 2018). PEU has a key role in influencing BI, as proven by previous studies (Dymek et al., 2022; Jin & Chen, 2022; Suki & Suki, 2011), but this is not proven in relation to energy efficiency through a sharing economy. Trust is another important factor that has an influence on BI, as trust in a technology can lead to an increase in its adoption. Similarly, trust of a bicycle-sharing application could lead to higher user adoption and promote a sharing economy.
Vedadi and Warkentin (2020) examine the impact of herd behaviour on BI in a sharing economy. Data are collected for two group experiments from more than 100 graduate and undergraduate business students at a public university in the US. The study posits that herd behaviour positively influences BI for energy-efficient technologies. Chen and Aklikokou (2020) investigate the impact of PU on BI, applying a questionnaire survey to collect empirical information on PU and BI from 482 Togolese respondents. The study finds that the PU of energy-efficient technologies has a positive impact on BI in a sharing economy. Hansen et al. (2018a) shed light on risk, trust, PEU, and BI, applying the theory of planned behaviour (TPB) and technology acceptance model (TAM). They find that PEU improves BI, and thus PEU has a positive relation with BI. Hooda et al. (2022) examine the impact of trust on BI in a sharing economy. Meta-analytic SEM methods and the unified theory of acceptance and use of technology (UTAUT) model are applied, and data is gathered from 90 previous studies on e-government. The results show that trust plays a crucial role in forming BI for using technologies in a sharing economy in the context of e-government.
Wijaya et al. (2019) analyse the relationships between herd behaviour, PU, and BI, applying the customer experience (CE) model, and expectation confirmation model (ECM). The empirical data are acquired from 311 users of social media commerce. The study posits that herd behaviour gives users a positive perception of the usefulness of energy-efficient technologies and helps them attain environmental and economic goals. The improved perception of usefulness improves users’ intentions to apply the technologies. Hence, PU has a meditating impact on the relationship between herd behaviour and BI. Moslehpour et al. (2018) examine the relationship between personality traits, PU, PEU, and BI in e-commerce. The technology acceptance model (TAM) is applied, and data are collected from Taiwanese online customers. The study claims that the herd behaviour of the users improves their perception of the ease and comfort they may have interacting with the technologies. If users already have positive perceptions of technologies, they have the motivation to interact with, and benefit from, them. This confirms the mediating role of PEU between herd behaviour and BI. Al-Rasheed (2020) analyses the relationship between herd behaviour, trust, and BI, through an online public survey collecting empirical data from 679 participants from Kuwait. According to the findings, herd behaviour develops general accepting behaviour and accelerates user trust. Trust increases user confidence in the sharing economy's usefulness and performance, thereby improving customer BI. Therefore, trust mediates the relationship between herd behaviour and BI. The following hypotheses are proposed:
Hypothesis 4 Herd behaviour has a positive effect on BI.
Hypothesis 5 PU has a positive effect on BI.
Hypothesis 6 PEU has a positive effect on BI.
Hypothesis 7 Trust has a positive effect on BI.
Hypothesis 8 PU mediates the relationship between herd behaviour and BI.
Hypothesis 9 PEU mediates the relationship between herd behaviour and BI.
Hypothesis 10 Trust mediates the relationship between herd behaviour and BI.
Environmental sustainability is described as responsible communication with the environment to avoid the reduction or degradation of natural resources and permit long-term environmental quality. With increasing use of energy, environmental degradation is increasing. However, protection of the environment is majorly dependent on energy efficiency (Mont et al., 2020). The sharing economy has an important contribution to make to the environment (Moreno-Izquierdo et al., 2019). Although previous studies address the sharing economy in relation to the environment, it is not considered in relation to the BI of people to adopt the sharing economy for energy efficiency. The behaviour of sharing economy users plays a major role in maintaining a healthy environment. The literature shows the relationship between BI and environmental sustainability (Martínez et al., 2019; Mialon & Nesson, 2020; Özer et al., 2020; Tiawon, 2020). Pan et al. (2018) investigate the relationship of BI and environmental sustainability using a questionnaire-based survey at nine universities in Taiwan, collecting empirical data for BI and environmental sustainability from 390 students in the tourism departments. After analysis, the authors confirm that the users who have a positive BI to adopt energy-efficient technologies and processes in a sharing economy, better contribute to environmental sustainability. Thus, the following hypothesis is proposed:
Hypothesis 11 BI has a positive effect on environmental sustainability.
Environmental sustainability is not a novel topic among researchers. Many studies discuss environmental sustainability, but the present study proves its novelty by addressing some literary gaps. Firstly, in the existing literature, the relationship between herd behaviour, PU, PEU, trust, BI, and environmental sustainability is discussed, with diverse opinions from various authors. The current study shows a positive relationship between herd behaviour, PU, PEU, trust, BI, and environmental sustainability in the context of energy efficiency and the sharing economy. Secondly, previous studies only link herd behaviour to PU, PEU, trust, and PU, PEU, and trust, to BI for environmental sustainability. In the present study, the analysis of the mediating role of PU, PEU, and trust between herd behaviour and BI for environmental sustainability addresses the literary gap. Thirdly, the previous literature discusses the relationship between herd behaviour, PU, PEU, trust, BI, and environmental sustainability in the context of energy efficiency and the sharing economy of diverse economies, while little attention is paid to these factors and their relationship to the environmental sustainability of China, the greatest pollution emitter. The current study fills this gap by analysing these factors in terms of the environmental sustainability of China. In past literature, various econometric and statistical approaches are applied to analyse the relationship between herd behaviour, PU, PEU, trust, BI, and environmental sustainability. The current study applies a survey questionnaire technique with area cluster sampling to give empirical and valid results.
Research methodologyResearch designThis study considers energy efficiency through user adoption of the sharing economy. The relationship between herd behaviour, PU, PEU, trust, BI, and environmental sustainability is considered. The study adopts quantitative research methods, which are most suitable for the nature of the relationship and the objectives. The study uses a cross-sectional research design instead of a longitudinal design, because the researchers suppose that the behaviour of the respondents could change over time. Thus, only a one-time data collection is made (Liao et al., 2020). The study takes herd behaviour as the independent variable, while PU, PEU and trust are used as the mediating variables, and behavioural intention and environmental sustainability are the dependent variables. The variables and their relationships are presented in Fig. 2.
Questionnaire developmentThis study measures six variables: herd behaviour, PU, PEU, trust, BI, and environmental sustainability. All the variables are measured using available scale items taken from previous studies. The measures of environmental sustainability are six scale items adapted from Ponce & Khan (2021). Herd Behaviour is measured by using five scale items adapted from Liu and Yang (2018). PU is measured using four scale items from Davis (1989) and Venkatesh and Davis (2000). PEU is measured using four scale items adapted from Davis (1989). Trust is measured using three scale items adapted from Liu and Yang (2018), and BI is measured using five scale items adapted from Liu and Yang (2018). All the measures are given in Table 1.
Scale items.
Sr. N. | Variable | Measure | Source |
---|---|---|---|
1 | Environmental Sustainability |
| Ponce & Khan (2021) |
2 | Herd Behaviour |
| Liu and Yang (2018) |
3 | PU |
| Davis (1989) and Venkatesh and Davis (2000) |
4 | PEU |
| Davis (1989) |
5 | Trust |
| Liu and Yang (2018) |
6 | BI |
| Liu and Yang (2018) |
In China, a number of people use bicycle-sharing applications, and the facility is common in most cities. The population of the study is individuals using bicycle-sharing applications in China. Thus, the respondents are people who use bicycle-sharing applications in China. Questionnaires are distributed to areas where bicycle-sharing applications are used. The study uses an online survey to carry out the data collection. Area cluster sampling is used for data collection, because this sampling technique is suitable for dealing with a widespread population (Hameed et al., 2020). Hence, questionnaires are filled by users who have experience of using bicycle-sharing applications, which is a typical application in the sharing economy. The data are collected online, from people at locations with numerous shared bicycles.
Data collectionThe data collection process started by distributing the questionnaires among people at locations with many shared bicycles. Along with the questionnaires the researcher provided instructions on how to fill out the questionnaire online. The objective of the study was explained to all respondents. A total of 400 questionnaires were distributed, and reminders were sent to the respondents after fifteen days. Finally, the researcher received 180 valid responses. Following the data collection, the data were entered into an Excel spreadsheet for analysis. After the data entry, the initial data screening was carried out, which is important to remove errors in the data, such as missing values and outliers (Ahmad Mahmoud et al., 2018), which may lead to inaccurate results. Three missing values were found in environmental sustainability, and six in herd behaviour. Five outliers were found in PU. All the missing values and outliers were removed from the data. The data statistics are reported in Table 2.
Data statistics.
Note: HB = herd behaviour, PU = perceived usefulness, PEU = perceived ease of use, TRU = trust, BI = behavioural intention, ES = environmental sustainability.
There are several data analysis techniques proposed by researchers. The implementation of suitable data analysis techniques is crucial for accurate results. Therefore, the study employs partial least square structural equation modelling (PLS-SEM), which is a popular and recommended data analysis technique, using SmartPLS software (Hair et al., 2020; Hooi et al., 2018; Purwanto & Sudargini, 2021). PLS-SEM is one of the latest data analysis techniques and is recommended for analysing primary data collected through questionnaire (Hair et al., 2017). PLS-SEM is divided into two steps (Davis, 1989): 1) a measurement model; and 2) a structural model. The measurement model is used to examine the factor loadings, composite reliability (CR), average variance extracted (AVE), convergent validity, and discriminant validity. The structural model is used to examine the relationships between variables in order to test the hypotheses.
Data analysisFig. 3 shows the PLS-SEM measurement model. The factor loadings are given in Fig. 3 and Table 3. According to previous studies, factor loadings should not be less than 0.7 (Hair et al., 2017). The results of the measurement model show that: herd behaviour has factor loadings between 0.883 and 0.927; PU has factor loadings between 0.897 and 0.815; PEU has factor loadings between 0.887 and 0.915; trust has factor loadings between 0.929 and 0.953; BI has factor loadings between 0.868 and 0.903; and environmental sustainability has factor loadings between 0.889 and 0.927. Hence, all the scale items have factor loadings higher than 0.7.
Factor loadings and convergent validity.
Note: HB = herd behaviour, PU = perceived usefulness, PEU = perceived ease of use, TRU = trust, BI = behavioural intention, ES = environmental sustainability.
Previous studies propose that convergent validity can be tested using CR and AVE. A CR value higher than 0.7 and an AVE value higher than 0.5 is the confirmation for convergent validity achievement (Ringle et al., 2015). All the values of CR and AVE are given in Table 3. The CR and AVE of herd behaviour, PU, PEU, trust, BI, and environmental sustainability are higher than 0.7 and 0.5, respectively, hence the study achieves convergent validity. The study also tests for discriminant validity (Hyland et al., 2019). The discriminant validity is tested using two methods: 1) the heterotrait-monotrait ratio of correlations (HTMT); and 2) the cross-loadings. For HTMT, given in Table 4, none of the values should be higher than 0.9. Along with the cross-loadings, given in Table 5, both methods confirm the discriminant validity.
Cross-loadings.
Note: HB = herd behaviour, PU = perceived usefulness, PEU = perceived ease of use, TRU = trust, BI = behavioural intention, ES = environmental sustainability.
After the assessment of the measurement model, the study applies the structural model (Hair et al., 2021, 2020). The structural model is used to examine the relationships between the variables, evaluated through direct and indirect effect hypotheses. The t-statistic values should be larger than 1.96, and the p-values should be less than 0.05 for associations between the variables to be significant. The structural model, given in Fig. 4, shows the relationships between the independent variable, dependent variables, and mediating variables. The effect of herd behaviour is considered in relation to PU, PEU, and trust; the effects of PU, PEU, and trust are considered on BI; and the effect of BI is considered on environmental sustainability. It is found that: herd behaviour has a significant effect on PU, with a t-value of 100.771; herd behaviour has a significant effect on PEU, with a t-value of 14.2; herd behaviour has a significant effect on trust, with a t-value of 16.449; PU has a significant effect on BI, with a t-value of 1.989; PEU has a significant effect on BI, a t-value of 8.899; trust has a significant effect on BI, with a t-value of 5.365; herd behaviour has a significant effect on BI, with a t-value of 2.01; and BI has a significant effect on environmental sustainability, with a t-value of 93.246. The results are given in Table 6.
Direct effect results.
The study considers three mediating effects. Firstly, the mediating effect of PU is examined between herd behaviour and BI. Secondly, the mediating effect of PEU is considered between herd behaviour and BI. Thirdly, the mediating effect of trust is considered between herd behaviour and BI. The t-statistic values should be larger than 1.96, and p-values should be less than 0.05 for the associations among the variables to be significant. The mediating effects are given in Table 7. The mediating effect of PU between herd behaviour and BI is insignificant as the t-value of 0.341 is less than 1.96. The mediating effect of PEU between herd behaviour and BI is significant, with a t-value of 9.027. The mediating effect of trust between herd behaviour and BI is significant, with a t-value of 4.853.
Indirect effect results.
The mediating effect of PEU between herd behaviour and BI is given in Fig. 5. The mediating effect of trust between herd behaviour and BI is given in Fig. 6. Table 7 shows all the indirect effects. The current study considers three indirect effects related to Hypothesis 8, Hypothesis 9, and Hypothesis 11.
Finally, the study considers the r-square value to examine the variance in BI and environmental sustainability explained by the other variables. Fig. 3 shows that BI has an r-square value of 0.885, indicating that herd behaviour, PU, PEU, and trust are expected to account for 88.5% of the change in BI. The dependent variable, environmental sustainability, has an r-square value of 0.860, indicating that herd behaviour, PU, PEU, trust, and BI are expected to account for 86% of the change in environmental sustainability. The variance in BI and environmental sustainability explained by the other variables is considered strong, as the r-square value is above 0.6 (Ahmad et al., 2018; Purwanto & Sudargini, 2021).
DiscussionThe results of the study show that herd behaviour has a positive effect on PU. An increase in the behaviour of people towards energy efficiency and the sharing economy can increase PU. Several other studies in the literature highlight the positive relationship between the behaviour of people and PU in various other contexts (Al-Gasawneh et al., 2022). Therefore, the results of the study are consistent with previous studies which highlight similar relationships between behaviour and PU. In the current study, this relationship is Hypothesis 1. Hypothesis 2 concerns the effect of herd behaviour on PEU. PEU is an important factor in relation to energy efficiency and the sharing economy. PEU has a significant relationship with the sharing economy and energy efficiency, and this study finds that herd behaviour has a positive effect on PEU, as positive behaviour by people can lead to PEU, which has a significant role in promoting the sharing economy in relation to energy efficiency. Furthermore, results show that the relationship between herd behaviour and trust, proposed by Hypothesis 3, is significant and positive. Similarly to the other two hypotheses, herd behaviour affects trust, and an increase in the positive behaviour of people can increase the level of trust in the sharing economy. Furthermore, the study highlights the relationship between herd behaviour and BI, the subject of Hypothesis 4. The results prove that herd behaviour has a direct effect on the BI of people. The intention of people to use the bicycle-sharing applications is influenced by herd behaviour. The behaviour of other people makes a significant contribution to the intention of the individual to use sharing applications. The next three hypotheses, Hypothesis 5, Hypothesis 6, and Hypothesis 7, concern the direct effects of PU, PEU, and trust on BI. People play a role in adopting the sharing economy by sharing bicycles. The results prove that PU has a positive effect on BI. An increase in PU can increase the BI of people to use these applications, which leads to energy efficiency through the sharing economy. In addition, PEU has the potential to promote BI, because the results show that it has a positive effect on BI. The easiness of using bicycle applications can lead to an increase in the BI of people to use and share bicycles. Trust is also found to be positively correlated with BI. This means that an increase in the level of trust among people can increase their intention. Previous studies highlight the effect of PU, PEU, and trust on BI (Hansen et al., 2018b; Nowacki, 2021; Suleman & Zuniarti, 2019). Therefore, the literature is in line with the current study. Finally, considering the direct effects, the study proposes a direct relationship between BI and environmental sustainability in Hypothesis 8. This relationship is found to be significant and positive, which shows that BI has a positive effect on environmental sustainability. Several previous studies also highlight the BI of people and its relationship to the environment (Hong & Kim, 2021; Saari et al., 2021).
After considering the direct effects, the study considers the mediating effects. Three mediation hypotheses are proposed, relating to the mediating effects of PU, PEU, and trust between herd behaviour and BI, Hypothesis 9, Hypostasis 10 and Hypothesis 11. The results show that PU is not a mediating variable between herd behaviour and BI. This means that user adoption does not reflect the effect of herd behaviour on BI. On the other hand, PEU and trust are mediating variables between herd behaviour and BI. This shows that PEU and trust have the potential to transfer the positive effect of herd behaviour onto the BI of people in relation to energy efficiency through the sharing economy. Therefore, along with the direct positive effect of herd behaviour on BI, there is also an indirect effect of PEU and trust.
Finally, the study concludes that user adoption of the sharing economy is important in environmental sustainability. The sharing economy, which leads to energy efficiency, can be achieved with the help of user adoption. Users must adopt sharing economy practices for energy efficiency, which may lead to sustainable development and protection of the environment. It is proven that user adoption of the sharing economy has a potential role in increasing environmental performance. The results of the study prove that herd behaviour has the potential to improve the behaviour intention of people towards energy efficiency through the sharing economy with the influence of PU, PEU, and trust. These three elements have a significant role in sustainable development and energy efficiency through user adoption of the sharing economy.
ConclusionThe objective of this study is to investigate the role of energy efficiency through user adoption of the sharing economy in order to promote environmental sustainability. A survey questionnaire is used for data collection to examine the relationship between herd behaviour, PU, PEU, trust, BI, and environmental sustainability. PLS-SEM is used to test 11 hypotheses, of which eight are direct hypotheses, and three are based on the mediation effect of PU, PEU, and trust. The results show that herd behaviour positively influences PU, PEU, and trust for energy efficiency in the sharing economy. If individuals are influenced by herd behaviour, they accept change in others and try to adopt these changes. The results show that people who move with the herd try to understand new technologies, resources, and processes, giving them confidence and a good perception of the usefulness of new technologies and techniques. This results in a higher perception of the ease of use. Herd behaviour motivates people to trust themselves to achieve tasks, trust others to be cooperative and loyal, and trust initiatives to work while adopting energy efficiency in the sharing economy. According to the findings, PU, PEU, and trust positively influence BI. The results show that in the sharing economy, if users have a high perception of the usefulness of the technologies and techniques to be adopted, they adopt positive behaviour to employ energy-efficient technologies and processes. The results reveal that, in the sharing economy, if users have a high perception that it will be easy to use energy-efficient technologies and techniques, they have a behaviour intention to employ energy-efficient technologies and processes. The results show that, in the sharing economy if users have high trust, they have behavioural intention to employ energy-efficient technologies. The study concludes that, in the sharing economy, herd behaviour enhances the PU, PEU, and trust of users of energy-efficient technologies and techniques, and increased PU, PEU, and trust shapes BI. The study findings state that BI and environmental sustainability have a positive association. An increased BI for energy-efficient technologies and techniques enhances environmental sustainability.
Policy implicationsTheoretical implicationsThe authors find much to learn from this study to improve future academic work. This study investigates the relationship between herd behaviour, PU, PEU, trust, BI, and environmental sustainability. The study is a great addition to the literature because it explores this relationship in the context of energy efficiency and the sharing economy. The direct relationships of PU, PEU, and trust with BI are considered. The study tests PU, PEU, and trust as mediators between herd behaviour and BI, contributing to the literature. Very limited studies consider the relationship between herd behaviour, PU, PEU, trust, BI, and environmental sustainability for energy efficiency in the sharing economy in China. The authors consider this research to add to the literature.
Practical implicationsPractically, the current study makes important insights for the management of various companies and other practitioners promoting energy efficiency through the sharing economy. The study highlights user adoption as the most important aspect of the sharing economy. Practitioners must consider user adoption in the promotion of energy efficiency through the sharing economy. Practitioners must promote the factors which lead to an increase in user adoption. For instance, practitioners should promote PU, PEU, and trust among people, as these factors ultimately have the potential to increase BI. Practitioners should promote bicycle-sharing applications by emphasizing PU, PEU, and trust, which have the potential to lead to energy efficiency. Additionally, environmental performance has the potential to increase with the help of energy efficiency through a sharing economy. Therefore, practitioners should consider the sharing economy as part of energy efficiency to promote environmental sustainability. The favourable intention of people to engage in the sharing economy can promote environmental performance, which is proved by the results. Therefore, the use of the sharing economy by people should be promoted using various factors to enhance environmental sustainability.
Limitations and future directionsThere are several factors that affect the BI of people in relation to energy efficiency through the sharing economy. However, the current study considers only a limited number of these factors, PU, PEU, and trust. Future studies should also consider other factors which could influence the BI of people to use the sharing economy and lead to environmental sustainability. Furthermore, the current study considers bicycle-sharing applications in relation to energy efficiency and the sharing economy. However, there are a number of other dimensions of the sharing economy which should be considered by future studies to extend the current study framework.