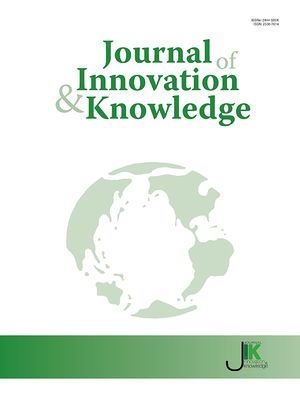
Edited by: Abbas Mardari
More infoIn recent years, the data trading industry has thrived on data assetization. However, given the increasing privacy concerns of the main data providers—individual users—their willingness to disclose data is in decline. Meanwhile, enterprises may violate privacy laws by profiting from co-creation data without user permission, blocking a significant amount of co-creation data from full circulation. This study establishes the utility function of users under three choices—purchase, sale, and maintaining the status quo—that quantify the privacy calculus mechanism and introduce privacy-related variables, employing references and interviews with Tencent employees and users. Accordingly, examining the decision-making behavior of users helped establish a data property rights two-way transaction model comprising six scenarios and, hence, six corresponding platform decisions. Indeed, the model can solve the noted dilemma and realize a Pareto improvement of the utilities of the platform and users. This study contributes a new perspective, constructs a data property rights two-way transaction model, expands the pricing theory boundary, and provides a decision reference for data trading practice, allowing enterprises to establish new business models.
The advent of the Internet, big data, cloud computing, and artificial intelligence have popularized various intelligent terminals. People use different platforms that record significant amounts of structured and unstructured data generated during the use process (Mačiulienė & Skaržauskienė, 2020). The amount of personal user information collected by large Internet and social network platforms has reached unprecedented levels (Mai, 2016). Data released by Tencent Holdings Limited (2021) on March 24, 2021, show that WeChat has more than 1.2 billion active monthly accounts, and more than 120 million users post content in the form of WeChat Moments daily. Approximately 360 million users open WeChat official accounts daily for browsing, and 400 million open the applet (Tencent Holdings Limited, 2021). Such user behaviors produce a large amount of data that are collected, processed, and stored on the platform. This study terms the data co-created via platform-individual interactions as “platform-individual” co-creation data. The data platforms collect include personal details that can directly identify users (e.g., name, biometric information, ID number, and mobile phone number) (Xiong et al., 2022). The generation of such data does not depend on the platform; thus, they are not considered co-creation data.
However, recent years have seen frequent instances of platforms infringing on users’ personal information. On July 24, 2019, the Federal Trade Commission (FTC) imposed a $5 billion penalty and sweeping new privacy restrictions on Facebook because it charged that the company violated a 2012 FTC order by deceiving users about their ability to control the privacy of their personal information.1 In July 2021, European privacy regulators fined Amazon 746 million euros ($887 million) for breaching the EU's General Data Protection Regulation.2 The level of user privacy concerns has since heightened. In the absence of incentives, most individuals do not take the initiative to allow platforms to commercialize their data. Thus, platforms, as direct storage facilities for “platform-individual” co-creation data, cannot use them freely.
In this context, co-creation data transactions can improve the situation of both parties and solve existing challenges. However, unclear data ownership issues hinder data transactions (Yu & Zhao, 2019). The clarification of data property rights is also the premise of data accounting and trading (Liang & Yuan, 2021). Arguably, platforms and individuals share co-creation data property rights, which lays the foundation for co-creation data trading. Currently, many pricing mechanisms for data transactions exist. Liang et al. (2018) categorize pricing models into economic- and game-theory-based pricing models. These pricing models are suitable for different situations, but few consider the willingness of individual data providers to disclose data or privacy-related factors. Moreover, previous studies seldom address “platform-individual” co-creation data transactions.
Thus, this study constructs user utility functions under different decisions based on the privacy calculus mechanism and value co-creation effect to establish a two-way transaction model of “platform-individual” co-creation data property rights. Hence, it answers the following research questions: How can a pricing model be built based on the user utility function? What choices do users make under different pricing scenarios? How does the platform make pricing decisions based on the model to maximize revenue? This study establishes users’ utility functions under three different decisions to construct a two-way transaction pricing model via co-creation data property rights. Further, it proposes the platform's pricing decisions under six scenarios to ascertain six corresponding platform decisions.
This study contributes to the literature as follows. From a theoretical perspective, this study has a three-fold contribution. First, the privacy calculus mechanism of users is quantified using three utility functions containing privacy-related factors to describe users’ mental process of measuring utility. Second, this study provides a new research perspective for data pricing from the perspective of the utility of personal data providers, introducing privacy-related factors to study individual decision-making behavior. Third, it constructs a two-way transaction model of co-creation data property rights. Evidently, existing studies focus only on one-way transactions. In the process of co-creating data with the platform, users are value creators and users. Therefore, this study establishes a two-way transaction model. Users can sell a portion of the value created by themselves as value creators or buy a portion created by the platform as consumers. The two-way transaction model is a theoretical innovation that furnishes new data transaction possibilities.
The rest of this paper is organized as follows. Section 2 reviews the literature on data association, data pricing, privacy concerns, and value co-creation. Section 3 presents the model variables and assumptions from the literature reviews and interview results. Section 4 constructs the two-way transaction model of co-creation data property rights. Section 5 conducts a numerical simulation of the model by referring to specific data in the interview to observe a specific model situation and improve the pricing strategy. Section 6 concludes the study with a summary of the findings, implications, limitations, and scope for future research.
Literature reviewData transactions hinge on data assetization. While prior studies propose many data pricing models, few focus on pricing co-creation data. Few also associate the privacy concern mechanism with the value co-creation effect to create a two-way transaction model of co-creation data property rights. This study reviews data assetization, data pricing, privacy concerns, and value co-creation studies.
Data assetizationData can comprise assets that are valuable and tradable (Xiong et al., 2022). Accordingly, data is considered “the new oil” of the digital age (Sadowski, 2019). Today, the data-rich environment has induced new opportunities and challenges (Orlandi, 2016). Thus, firms are increasingly developing data-driven strategies (Saura, 2021). The information technological advances have improved data analysis capabilities, which supports innovation.
Many countries realize that big data describe a new type of strategic resource and a crucial strategic asset (Yu & Zhao, 2019). Hence, some governments have begun to promote data asset trading as a legitimate business activity (Xiong et al., 2022). However, from a company perspective, data assets remain poorly utilized and must be governed systematically (Adamides & Karacapilidis, 2020; Hannila et al., 2022). Indeed, Zhang et al. (2022) and Abraham et al. (2019) consider data governance as the disciplined and legitimate management of data into a strategic enterprise asset. Zhang et al. (2022) define the process of governing the data-by-data standards to obtain clean and practical data as data assetization. The study focuses on co-creation data; that is, data generated by individuals and collected, cleaned, and stored by the platform. From the noted studies, co-creation data are valuable and tradable assets with property rights shared by platforms and individual users.
Data pricingCurrently, many data markets have emerged, accompanied by a wide range of pricing mechanisms for data transactions (Driessen et al., 2022). The influencing factors of the data transaction price also vary (Liang & Yuan, 2021). As per Liang et al. (2018) and Badewitz et al. (2022), pricing models can be categorized into several approaches, including cost-, perception-, game-theory-, programming-, and query-based pricing models. Although these models have different applicable conditions, barely any of them consider two-way data property rights transactions, and few consider the impact of the data provider's privacy attitude on pricing. Existing pricing cannot address the research questions. However, the pricing model based on perception provides a vital research reference. Harmon et al. (2009) propose that the data performance perceived by data consumers is among the main factors affecting data pricing, and the key indicator to measure data performance is utility. Accordingly, this study can start from the perspective of data providers and establish a co-creation data transaction model based on utility functions.
Privacy concernsIn recent years, companies have increasingly developed data-driven innovation strategies (Saura, 2021). However, the large-scale analysis of user-generated data has induced user privacy concerns (Saura et al., 2021; Ying et al., 2023), making users more sensitive to user-generated data collection by platforms. Privacy concerns in this research context refer to Internet privacy concerns; that is, users’ concerns about a website's collection and use of their personal information, reflecting their perception of the disparity between users’ expectations of privacy protection and service providers’ privacy-related actions (Hong & Thong, 2013).
Privacy concerns affect users in different ways. From the perspective of psychology and cognition, users with high-level privacy concerns have a greater perceived privacy disclosure risk (Malhotra et al., 2004) and show distrust toward enterprises requiring privacy disclosure (Miltgen et al., 2016). From the behavioral response perspective, an increase in privacy concerns induces a decrease in users’ behavior of disclosing personal information (Bansal et al., 2016). Previous studies show that consumers want to pay to protect their data from being used by platforms given privacy concerns (Kummer & Schulte, 2019; Savage & Waldman, 2015). Meanwhile, some studies highlight the phenomenon of the privacy paradox, noting the discrepancies between users’ privacy concerns and behaviors (Acquisti & Grossklags, 2005; Li et al., 2023; Debatin et al., 2009; Smith et al., 2011). Prior studies argue that before making privacy disclosure decisions, users consciously weigh the expected losses and potential benefits; that is, privacy calculus (Culnan & Armstrong, 1999; Li et al., 2023; Debatin et al., 2009; Dienlin & Metzger, 2016; Krasnova et al., 2010; Krasnova & Veltri, 2010). Through a systematic literature review of the privacy paradox, Barth and De Jong (2017) determine that the result of the risk-benefit evaluation generally drives users’ privacy disclosure willingness. This study ignores the deviation in the estimation of gains and losses by individual cognitive bias (Lee et al., 2013) and assumes users are “rational people” who take action after risk-benefit evaluation (Karwatzki et al., 2017).
Value co-creationThe individual-platform data co-creation produces a value co-creation effect, where consumers participate and cooperate with firms to create value (Humphreys & Grayson, 2008; Prahalad & Ramaswamy, 2004), potentially blurring the line between consumers and producers. Humphreys and Grayson (2008) argue that when individuals produce exchange value for companies in value co-creation, their roles fundamentally change. The blurring effect provides a theoretical basis for constructing a two-way transaction pricing model of data property rights. Norton et al. (2012) find that consumers overestimate the value of products in which they have invested; that is, the value of co-creation goods. Moreover, value co-creation effects can occur in goods and service purchases (Alves et al., 2016; Ajmal et al., 2023; Auh et al., 2007; Etgar, 2008; Evangelista et al., 2022). Existing studies show the widespread value co-creation effects in customer engagement processes. From the theoretical modeling view, Wang and Fan (2020) and Basu and Bhaskaran (2018) construct utility functions to analyze the value co-creation effect. Studies on value co-creation rarely probe co-creation data transactions. Therefore, utility functions should be constructed by associating the privacy calculus mechanism and value co-creation effect per existing parameters.
Research methodResearch frameworkFig. 1. Research framework presents the research framework to articulate the research design and process. This study employs employee and user interviews in four distinct steps. The introductory section overviews the relevant background information. The second part discusses privacy attitudes; it explores participants’ attitudes and concerns regarding data privacy through interviews. The third part aims to understand participants’ co-creation data comprehension and their evaluation of its value. The fourth part constructs the hypothesized scenario of two-way transactions in co-creation data and examines participants’ attitudes within this context.
Building on the insights from the interviews, the study conducted a literature review on data assetization, data pricing, privacy concerns, and value co-creation. Subsequently, it developed a research model based on the interviews and existing literature. In the final stage of the research, the study performed numerical simulations using data collected from interviews. Observing the simulation results revealed a deeper understanding of “platform-individual” co-creation data transactions, providing valuable insights for relevant decision-making and practices.
InterviewsFurther, to understand co-creation data and construct the transaction model, this study takes the WeChat platform as the research object and interviews four Tencent employees (managers from different departments) and 38 users. Employee interviews investigated employee attitudes toward co-creation data and the current data application situation of the enterprise. User interviews examined users’ privacy concerns and their attitudes toward co-creation data transactions. The interviews provide a realistic foundation for the two-way transaction model of co-creation data.
Following the expert interviews by Gimpel et al. (2018), this study interviewed four Tencent managers from different departments, each lasting approximately 60 min. This study adopted a semi-structured approach to interviewing employees and developed follow-up questions per respondents’ answers. The interview comprised four parts. The first part introduced the research background to reveal the data problem. The second part asked respondents’ about their understanding and perception of data privacy. The third step examined respondents’ views on co-creation data, as they evaluated the value of their co-creation data from the enterprise perspective. Finally, assuming a two-way trading situation, respondents evaluated the potential benefits of data trading.
Analyzing the employee interviews induced the following inspirations. First, all employees agreed that the value of user accounts is a joint platform-individual creation. However, employees had diverse views on the proportion of the value created by the platform relative to the total co-creation data value, with their assessments ranging from 27% to 60%. When asked about the current data application situation, all employees agreed that WeChat uses only part of the co-creation data to make profits. The platform has the incentive to obtain complete co-creation data. However, employees had different views on the proportion of data used and the evaluation of profit margins. Co-creation data have the following features: The platform and individuals share the property rights of co-creation data; co-creation data have economic value and can guide individuals or enterprises in making decisions; and data integrity is an essential index with which to evaluate data quality and value (Liang & Yuan, 2021).
The user interview also comprises four parts. However, because employees and users have different perspectives and understandings of data practices, there are some differences between user and employee interviews regarding specific questions. Following the interview sample size of related studies (Liyanaarachchi, 2021; Parks et al., 2017), we interviewed 38 users, including 14 under 24 years old, 16 between 25 and 34 years old, five between 35 and 44 years old, and three over 45 years old. The first step of the interview briefly introduced the research background and data types used by the WeChat platform (including contacts, location, and search history). Users were then briefed on the concept of co-creating data to facilitate follow-up questions.
The second step of the interview askedrespondents’ privacy-related situations when using various platforms. This part refers mainly to the studies of Hong and Thong (2013) and Gimpel et al. (2018), where most users experienced data privacy violations when using Internet platforms. Their degree of discomfort is generally intensive. Examples cited by respondents include the following. (1) The platform pushes “content you may be interested in” per users’ browsing data, making people feel that platforms monitor their browsing behavior. (2) Some platforms frequently ask users for certain permissions, such as their geographical location, which is unsafe to expose. (3) After searching for a particular product on e-commerce platforms, relevant advertisements appear on other non-shopping platforms, such as Douban and Zhihu, making people uncomfortable. (4) Platforms such as Weibo obtain geographic location information and push advertisements for nearby businesses. (5) Some platforms monitor user conversations through microphones, infringe upon their chat information privacy, and push related advertisements. (6) Some platforms receive user identity information and recommend advertisements for postgraduate entrance examination courses and certificate training.
Regarding the summary of data privacy concerns and measures in Gimpel et al. (2018), user responses involve various privacy concerns generated in the process of using platforms, such as data collection, internal secondary usage, and external secondary usage. In such cases, users’ discomfort is the perceived privacy risk (Hajli & Lin, 2016; Maseeh et al., 2021; Wiese et al., 2020), a fear that the platform infringes upon the value of the data they create. Thus, the third step of the interview ascertained the specific situation of using the WeChat platform. Users were given some reference information to evaluate the value of the co-creation data they generate every year and estimate how much they created themselves. Apparently, most users overestimated the value of the co-creation data of their accounts, thinking that the value they create accounts for more than 50% of all value created.
Finally, the interviews assume a data property rights two-way transaction scenario: first, users buy co-creation data from the platform. Assuming the WeChat platform launches a private membership service, then members’ data are protected, not used for profit, and not shared with other platforms, except for data related to special needs such as public security. Further, members can independently manage all co-creation data, for which users must pay some fees. Second, the platform purchases from users. Currently, WeChat cannot use all co-creation data to make profits. Thus, assumedly, WeChat hopes to use all co-creation data to make profits and is willing to provide users with some form of compensation. Given the two-way trading scenario, the interviews examined users’ attitudes and expected decisions under different degrees of compensation and pricing. Consistent with the literature on privacy calculus (Culnan & Armstrong, 1999; Debatin et al., 2009; Dienlin & Metzger, 2016; Krasnova et al., 2010; Krasnova & Veltri, 2010), the interviews show that users make privacy-related decisions based on cost-benefit tradeoffs. Given users’ different privacy risk assessment levels, they make three decisions based on their estimate of utilities: buy, sell, or maintain the status quo. Some users willingly pay fees to make purchases, after which they obtain a sense of security that their data are protected. The intensity of this sense of security varies among people. The stronger the current feeling of discomfort, the stronger the sense of security post-purchase. Users who sell bear feelings of discomfort and offer data for compensation, while those who choose to maintain the status quo do not provide data or ask for compensation.
Variables and assumptionsTable 1 describes all the notations used in this study.
Notations and descriptions.
V represents the total value of the co-creation data. The user interviews show that people have similar habits in using the WeChat platform, and the data generated are similar in volume. The employee interviews show that the dimensions of data collected by the platform from each user are similar, and the value contributed by each user is similar. Therefore, the total value of the co-creation data for most users’ accounts is relatively similar. Regardless of extreme values, this study assumes that vi→v¯. Further, to simplify the model, we normalize vi by dividing it by v¯.3 As vi→v¯, V=vi/v¯→1. Therefore, without loss of generality, V is set to 1.
N represents the total number of users. For a platform in the mature stage, the number of users typically exhibits a stable trend and does not undergo significant fluctuations in the short term. For example, data released by Tencent Holdings Limited (2022) indicate that the combined monthly active users of Weixin and WeChat exhibited a quarter-over-quarter increase of 0.4% in Q4 2021, suggesting a relatively stable user base. As this study does not focus on how the number of users affects the model, N is set to 1; thus, the users are treated as a whole, and the total number of users does not change. Instead of focusing on the number of users, this study focuses on the percentage of users making different decisions.
The study then assumes that each user contributes a ratio, φ, to the co-creation data of their account. As the value created by customers accounts for less than 100%, φ ranges from (0, 1). As this study sets V to 1, φ also represents the value created by users. Accordingly, the value created by the platform is (1−φ). From value co-creation theory and interview results, most users tend to overestimate the value of the data with which they participate in co-creation. Therefore, this study defines θ to represent the coefficient of overestimation. Regarding users’ perception, the value created is φ(1+θ), and that created by the platform is (1−φ)(1+θ).
The interviews show that most users have experienced data privacy violations when using Internet platforms. This discomfort stems from a worry that the data value they create with co-creation data will be infringed upon. This study assumes the discomfort to be −φ(1+θ)r. φ(1+θ) represents the perceived value of the data created by users, and r represents the perceived risk coefficient. The intensity of each user's discomfort indicates the degree of their perceived privacy risks. The maximum loss users may suffer corresponds to that of the perceived value they created themselves, represented by −φ(1+θ). Hence, this study assumes r follows an even distribution in the interval [0, 1]. The interviews show that, under transaction scenarios, users gain a sense of security after purchasing data, and the degree of this sense of security closely relates to the amount of discomfort they feel. Further, to simplify the model, we assume the sense of security generated after purchasing data is φ(1+θ)r.
In the two-way transaction scenario, user decisions range between three choices, assuming their utility under the purchase decision is U1, their utility under the sales decision is U2, and their utility under the status quo decision is U3. p1 represents the proportion of the cost paid by the user to the total value. p2 represents the ratio of the platform purchase price (compensation given to the user) to the total value. This study assumes p1 and p2 are continuous variables that are greater than or equal to 0. As the marginal cost of data collection is relatively low, it is assumed to be 0. Finally, this study assumes that when the platform cannot make profits from all co-creation data, the ratio of revenue obtained from each user's account to the total value is M, ranging from (0 1). As this study sets V to 1, p1, p2, and M, can represent p1V, p2V, and MV, respectively.
Model constructionConstruction of utility functionsIn two-way transactions of co-creation data, a single user may make decisions from three choices: purchase, sell, or maintain the status quo. Fig. 2. Trading model shows the schematic diagram of the trading model.
Under the purchase decision, the user pays p1 and obtains the value (1−φ)V created by the platform, which the user perceives as (1−φ)(1+θ). The interviews show that users gain a sense of security after purchasing data, perceived as φ(1+θ)r. The utility function U1 is shown in (4–1). Under the selling decision, users obtain compensation p2 and lose the value of the data they created, perceived as φ(1+θ). Moreover, users still suffer discomfort. The utility function U2 is shown in (4–2). Users continue to suffer discomfort when they decide to maintain the status quo. The utility function U3 is shown in (4–3).
Boundary conditions for users’ choicesWhen U1>U3 and U1>U2, the utility of making a purchase is the largest, and the user makes a purchase. Substituting the functions into the calculation, we can obtain the following:
This study defines A=p1+φ+θφ−θ−12φ(1+θ) and B=p1+p2−θ−12φ(1+θ). The value range (4–4) indicates that if users’ perceived risk coefficient is greater than A and B, then they will choose U1 to make the purchase decision. When U2>U1 and U2>U3, the user makes a sales decision. The functions are then substituted into the calculation as follows:
When U3>U1 and U3>U2, users choose to maintain the status quo. The functions are then substituted into the calculation as follows:
Considering critical cases, for users with r=B, the two decisions, U1 and U2, have the same utility. In fact, in the hypothetical situation of this work, the decisions of users whose r equals a specific value will not significantly impact platform revenue. Without loss of generality, this study assumes that when U2>U3, users with r=B, which means U1=U2, choose U2. When U2≤U3, users with r=B, which means U1=U2 choose U3. Similarly, for users with r=A, the two decisions, U1 and U3, have the same utility. This study assumes that when U2>U3 users with r=A, which means U1=U3, choose U2. When U2≤U3, users with r=A, which means U1=U3, choose U3. Whenp2=φ+θφ,U2=U3 and A=B. The two decisions, U2 and U3, have the same utility. Without loss of generality, this study assumes that when p2=φ+θφ, users will not consider U3 but will choose between U1 and U2. Specifically, when p2=φ+θφ, users with r>B will choose U1, while those with r≤B will choose U2.
User decisions and platform revenue analysisThe discussions are classified per the values of A and B. When A,
p2>φ+θφ and U2>U3. From the analysis, in this situation, users with r>B will choose U1, while those with r≤B will choose U2. When A=B,p2=φ+θφ and U2=U3. As analyzed, users with r>B will choose U1, while those with r≤B will choose U2. As users’ decisions when A=B and AFig. 3).Further classification discussions are organized according to the value of B.
WhenA≤BandB>1When A≤B and B>1, all users choose U2 (see Fig. 4). Users sell their data, and the platform compensates them with p2.
After the transaction, the platform obtains users’ complete data, and the platform's cost is p2. The platform's revenue function is as follows:
As A≤B and B>1, the following constraints can be obtained:
Using the graphical method to solve the above linear programming problem, when p2=φ+θφ, the platform's revenue reaches the maximum. In this case, π=1−φ−θφ, and p1>φ+θ+θφ+1.
WhenA≤BandB<0When A≤B and B<0, all users choose U1 (see Fig. 5). Users pay p1 to purchase the value created by the platform. In this case, each user brings p1 income to the platform.
The platform's revenue function is as follows:
As B<0 and A≤B, the following constraints can be obtained:
Using the graphical method to solve the above linear programming problem reveals that π<1+θ−φ−θφ.
WhenA≤Band0≤B≤1When A≤B and 0≤B≤1, users with B
The platform's revenue function is as follows:
Further, to calculate and simplify the revenue function,
As 0≤B≤1 and A≤B, the following restraints can be obtained:
This study defines F(p)=p12+p22+2p1p2−(2φ+2θφ+θ+2)p1−(θ+2)p2+θ+1. The platform's revenue reaches the maximum when F(p) is the minimum. The problem is then transformed into the following constrained programming problem:
After the calculation, the Hessian matrix is obtained as follows:
The Hessian matrix is employed to determine the concavity and convexity of a function (Rardin, 1998). H is a positive semidefinite matrix; thus, F(p) is a convex function, and this problem is a convex optimization problem. We apply the Karush–Kuhn–Tucker (KKT) condition to solve the optimization value. The constraints are as follows:
First, the gradient is calculated as follows:
According to whether λi is zero, there are eight combination cases, five of which can be easily excluded according to the dual-feasibility condition. The remaining three cases are analyzed below.
1. When λ3≠0 and λ1,λ2=0, according to the KKT condition, when θ≤2φ+2θφ and 2φ+θ+2θφ≥0 (always holds), the minimum value of F(p) is obtained when p2=φ+θφ and p1=12(θ+2), respectively. In this case, λ3=2φ+2θφ (constantly greater than 0), and the maximum value of π is as follows:
2. When λ1,λ3≠0 and λ2=0, according to (4-16), when θ>2φ+2θφ and 2φ+2θφ≥0 (always holds), the minimum value of F(p) is obtained when p2=φ+θφ and p1=1+θ−φ−θφ, respectively. In this case, B=0 and π=1+θ−φ−θφ.
3. When λ2,λ3≠0 and λ1=0, according to (4-16), the minimum value of F(p) is obtained when p2=φ+θφ and p1=1+θ+φ+θφ. In this case, λ3=2φ+2θφ, and λ2=−2φ−θ−2θφ<0. Therefore, the case is not valid.
Thus, when A>B, p2<φ+θφ, and U2
Further classification discussions are organized according to the value of A.
WhenA>BandA>1When A>B and A>1, all users choose U3 (see Fig. 8). The platform can use only part of the data to obtain revenue M.
The constraints are as follows:
WhenA>BandA<0When A>B and A<0, all users choose U1 (see Fig. 9) to pay p1 to purchase the value created by the platform.
The platform's revenue function is as follows:
In this case, constraints can be obtained as follows:
Using the graphical method to solve the above linear programming problem reveals that π<1+θ−φ−θφ.
WhenA>Band0≤A≤1When A>B and 0≤A≤1, users with A
The platform's revenue function is as follows:
After the calculation, the following function can be obtained:
As A>B and 0≤A≤1, the following constraints can be obtained:
When p1=12(φ+θ+M+1+θφ) conforms to the constraints, the maximum value of the platform's revenue is as follows:
In this case, M must meet the following constraints:
Accordingly, M≤1+θ+φ+θφ is always true. When M<1+θ−3φ−3θφ, the platform's revenue reaches its maximum at p1=θ+1−φ−θφ:
Platform decisionsIn the actual situation, users evaluate the utility value per p1 and p2 given by the platform and make decisions accordingly. From the above calculation, there are six different pricing decisions as the values of p1 and p2 change (see Fig. 11).
From the analysis, the definition domain can be divided into six parts, corresponding to six different decisions. As in Fig. 11, this study annotates the domains of the six decisions.
Decision 1: all users choose to sellThe platform sets p1 and p2 according to the following constraints:
In this case, the revenue function of the platform is π=1−p2. When p2=φ+θφ and p1>φ+θ+θφ+1, π reaches the maximum: π=1−φ−θφ. Under these constraints, all users choose to sell their data. This decision indicates that once the value of p2 is larger than or equal to a boundary value, users do not consider U3.
Decision 2: all users maintain the status quoThe platform sets p1 and p2 according to the following constraints:
In this case, the platform's revenue is always M, which means p1 (p2) is too large (small) under these constraints. For all users, the utility of maintaining the status quo is the largest.
Decision 3: all users choose to purchaseThe platform sets p1 and p2 according to the following constraints:
In this case, the platform's revenue function is π=p1. Under the constraints, p1<1+θ−φ−θφ, and π<1+θ−φ−θφ. For all users, the utility of U1 is the largest.
Decision 4: all users choose to purchaseThe platform sets p1 and p2 according to the following constraints:
The platform's revenue function is π=p1. Under the constraints, p1<1+θ−φ−θφ, π<1+θ−φ−θφ. Users’ decisions under Decisions 3 and 4 are the same, indicating that once p1 and p1+p2 are less than their respective critical values, all users will choose U1.
Decision 5: some users buy, and others sellThe platform sets p1 and p2 according to the following constraints:
In this case, some users choose to buy, while others choose to sell. Under this decision, the platform's revenue function is π=p1(1−B)+(1−p2)B. If inequality θ≤2φ+2θφ is satisfied, then the maximum value of π is obtained when p2=φ+θφ and p1=12(θ+2) as follows:
If θ>2φ+2θφ, then π reaches the maximum when p2=φ+θφ and p1=1+θ−φ−θφ, which is 1+θ−φ−θφ. As p2=φ+θφ and p1=1+θ−φ−θφ, A=B=0. In this case, the extreme point lies at the junction of the feasible regions of Decisions 3, 4, and 5 (see Fig. 11).
Decision 6: some users buy, and others maintain the status quoThe platform sets p1 and p2 according to the following constraints:
Under these constraints, some people choose to buy, while others choose to maintain the status quo. The revenue function of the platform is π=p1(1−A)+MA. If inequality M≥1+θ−3φ−3θφ can be satisfied, then the platform's revenue maximum is obtained when p1=12(φ+θ+M+1+θφ):
If M<1+θ−3φ−3θφ, then the platform's revenue reaches the maximum when p1=θ+1−φ−θφ as follows:
Summary of the decisionsTable 2 summarizes the six decisions, describing users’ behaviors and platform revenue thereunder in-depth. Under different choices, the p1 and p2 values affect the values of A and B. Moreover, they influence users’ decisions. The platform should make its optimal decision based on the current situation and specific needs and set p1 and p2 according to the corresponding constraints.
Summary of users’ behaviors and platform revenue under different decisions.
We first briefly presented the research background in the user interviews in Section 3.1. Next, users described the data privacy violation problems they encounter when using various platforms. We then considered the specific situation of the WeChat platform, assuming a data property rights two-way transaction scenario. In this part of the interviews, beyond exploring psychological changes in different situations and studying what factors affect user behaviors, respondents were asked to conduct a numerical evaluation. We recorded the data and calculated the mean value to conduct the numerical simulation. The overestimation coefficient calculated based on the value assessed by users was approximately 14.81%, rounded to 15% (θ=0.15). The share of the value created by users is approximately 57%, rounded to 60% (φ=0.6). As assumed, the platform's revenue from each user while users maintain the status quo (M) is a constant within the interval (0, 1).
Simulation resultsSimulation of decision 1Under this decision, p1 and p2 meet the following constraints:
The revenue function of the platform is π=1−p2. When p2=0.69, the revenue of the platform reaches the maximum. In this case, π=0.31, and p1>1.84. When the revenue reaches the maximum, p2 reaches the boundary value; thus, users receive sufficiently high compensation if they choose to sell. Moreover, the value of p1 is too large, resulting in no user being willing to make the purchase decision.
Simulation of decision 2Under this decision, p1 and p2 meet the following constraints:
The revenue of the platform is M. As the value of p2 does not reach the boundary, and the value of p1 is too large, all users decide to maintain the status quo.
Simulation of decision 3Under this decision, p1 and p2 meet the following constraints:
Under this decision, the revenue function of the platform is π=p1. As p1<0.46, π<0.46. For all the users, the utility of U1 is the largest in this case.
Simulation of decision 4Decision 4 can be combined with the above decision. In this case, p1 and p2 have the following constraints:
The revenue function of the platform is π=p1. As p1<0.46, π<0.46. Users’ decisions under Decisions 3 and 4 are the same, indicating that once p1 and p1+p2 are less than their respective critical values, all users will choose U1.
Simulation of decision 5Under this decision, p1 and p2 meet the following constraints:
The revenue function of the platform is π=p1(1−B)+(1−p2)B. Substituting values into inequality θ≤2φ+2θφ reveals that 0.15≤1.38 is always true. Therefore, the platform's revenue reaches the maximum when p2=0.69 and p1=1.075:
Under the numerical simulation, F(p)=p12+p22+2p1p2−3.53p1−2.15p2+1.15 and π=−F(p)1.38. Based on the numerical simulation, we plot part of the F(p) graph (see Fig. 12).
The trend of the function graph reveals that the F(p) surface is inclined, and its extreme value is obtained at the section where p2 reaches the critical value of 0.69. We draw the cross-section of F(p) when p2 takes the critical value to further illustrate the extreme point (see Fig. 13). The function expression of the section is F(p)=p12−2.15p1+0.1426. The minimum value of the function is p1=1.075, meaning that the platform's revenue reaches the maximum when p1=1.075. Therefore, the observation results are consistent with the calculation results.
Simulation of decision 6Under this decision, p1 and p2 meet the following constraints:
The revenue function of the platform is as follows:
Substituting the value into inequality M≥1+θ−3φ−3θφ yields M≥−0.92. As this inequality always holds, the platform's revenue reaches the maximum when p1=12(1.84+M):
Since the value range of M is (0, 1), the following inequality always holds:
The inequality indicates that the platform benefit is higher than that under Decision 2.
Summary of the simulationsTable 3 provides a comprehensive summary of the user behaviors and platform revenue for the six different decisions under numerical simulation. Comparing the decisions reveals that the value of M is important for decision-making. From the numerical simulation results, the benefits of Decisions 1, 3, and 4 are less than those of Decision 5. Moreover, the benefits of Decision 2 are less than those of Decision 6. M affects the revenue of Decision 6. The platform's revenue under Decisions 5 and 6 should be compared to find the optimal choice under numerical simulation. We compare (5–9) and (5–6) to obtain the critical value:
Summary of user behavior and platform revenue for different decisions under numerical simulation.
Accordingly, when M>0.30983, the most profitable strategy is Decision 6. In Section 5.1, this study concretizes the model through numerical simulation, analyzes the specific platform situation under six different pricing decisions, and calculates the platform's revenue. Decisions 5 and 6 in the simulation scenario are better than the other decisions. Moreover, which of the two decisions is optimal depends on M. If M>0.30983, the revenue of Decision 6 is greater than that of Decision 5. Further, M determines whether other decisions are feasible. Decisions with revenue greater than M benefit the platform more than the decision to maintain the status quo.
The numerical simulation verifies that the model solves the research problem adequately. Based on the numerical simulation, this study presents some critical values of variables, such as p2 and M, and provides relevant guidance and suggestions. It also shows that the pricing model of the “platform-individual” co-creation data has good explanatory power for user behaviors under different decisions and can provide a theoretical basis for practice.
ConclusionSummary and implications of the findingsTaking the WeChat platform as the study object, this study establishes a two-way transaction model of co-creation data property rights, which offers a new economic approach to the current data problem. The transactions between the platform and users help enterprises avoid this problem and understand the value of platform-individual co-creation data. Based on the utility functions of users’ three choices (purchase, sell, and maintain the status quo), the model studies the decision-making behavior of users and identifies six scenarios corresponding to the six decisions of the platform, (see Table 4 User decision and behavior s).
User decision and behavior summary.
In different decision scenarios, users make different choices, and the platform benefits differently. Therefore, from the simulation results emerges certain conclusions and management implications, discussed as follows. First, the platform should pay more attention to the value of M. Under Decision 2, all users choose to maintain the status quo, and revenue Mis an evaluation standard. Decisions with revenue greater than Mare feasible. Second, the platform should focus on Decisions 5 and 6. In the simulation (Section 5), the two decisions are better than the others. Which of these two decisions is optimal depends on the value of M. If M>0.30983, then the benefit of Decision 6 is greater than that of Decision 5. If the platform's goal is to maximize revenue, then it should focus on Decisions 5 and 6 and set p1 and p2 based on price constraints. Third, the platform should find a balance between the amount of data collected and revenue. Maximizing revenue may not be the best decision for the platform because revenue measures only economic value and ignores the business efficiency gains that can be provided by large-scale data. The platform must make the correct decision based on its data needs.
Overall, this study promotes the development and perfection of data transactions and makes possible two-way data property rights transactions between platforms and individuals, benefiting both parties. Moreover, this research on “platform-individual” co-creation data pricing can provide decision support to platforms and users. Platforms can design new products following the study model and launch services such as private membership. Platforms can also expand their business, establish new models for data transactions, and develop data-transaction-related industries. This study can help users better understand the value and privacy disclosure mechanism of co-creation data. Third, it provides an economic solution to the data dilemma between the platform and users. Currently, the main means to solve the data dilemma problem are administrative. The government establishes restrictive policies, supervises enterprises, and penalizes those that violate regulations. This study provides a practical economic approach that can help solve this problem.
Limitations and directions for future researchDespite the implications, the study has notable limitations that give scope for future studies. First, when setting the variables, the study addresses some restrictions by employing certain assumptions. For example, for the model construction, the values of the co-creation data of each user's account are assumed to be the same. This study also assumes that each user's overestimation coefficient θ is the same and that the risk coefficient r is evenly distributed between [0, 1]. Though such assumptions contribute to model clarity, they inevitably impose some limitations on the model. Future research can curb some of the assumptions to make the model more universal. Furthermore, as inequality affects subjective well-being (He et al., 2022), future research can explore the impact of the relevant variables on pricing. Second, this study assumes that the platform obtains the same value every time it obtains data from a user. Nevertheless, the value of the data available to the platform may have a cumulative effect. The platform's marginal revenue may change with the change in the total amount of data available. Future studies can change the assumption of the fixed marginal revenue of the platform. Third, there are different types of platforms, most of which co-create some data with their users. The model construction in this work is based on the actual use case of WeChat, which has many users. Although the data co-creation pattern and psychological state of users in transactions are similar on different platforms, there are also inevitable differences. Therefore, the research scope can be expanded to cover different platform types. Finally, this study employs data from China. However, as people from different countries have different attitudes toward privacy, future studies can collect data from multiple countries to compare diverse attitudes toward privacy disclosure.
Authors contributionsConceptualization, J.L.; Methodology, J.L. and R.L.; Software, R.L.; Validation, J.L. and R.L.; Formal Analysis, R.L.; Investigation, R.L.; Data Curation, R.L.; Writing – Original Draft Preparation, R.L.; Writing – Review & Editing, J.L.; Visualization, R.L.; Supervision, J.L.; Project Administration, J.L.; Funding Acquisition, J.L. All authors have read and approved the final manuscript.
FundingThis work was supported by the National Social Science Fund of China (18BGL110).
We thank Elsevier for English language editing services.
Junfeng Liao received his bachelor's degree in Finance from Sun Yat-Sen University in 2002, his MSc degree in Electronic Business Management from the University of Warwick, UK in 2004, and his Ph.D. degree in Marketing from the South China University of Technology, in 2014. He also visited the School of Hospitality and Tourism Management at Purdue University, US, in 2018 and 2019. He is an Associate Professor in the Department of Electronic Business in the South China University of Technology. His research interests are in the area of electronic business and marketing, focusing on national branding, consumer behavior, cross-cultural study, and internet finance. He has published in journals and conferences indexed by SCI, SSCI, CSSCI and EI, such as Academic Research, Social Sciences in Guangdong, and Information Sciences in China.