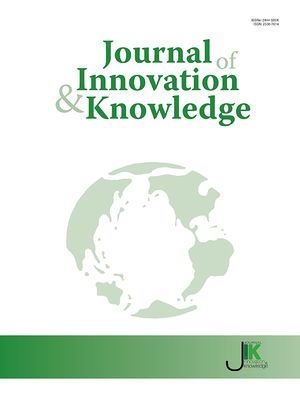
Edited by: Brij B. Gupta, Kwok Tai Chui
More infoThe ongoing Corona Virus Disease 2019 (COVID-19) pandemic presents several challenges to the education system including technical, cognitive, managerial, and behavioral ones. As a result of these pressures, education systems are undergoing dramatic changes. The persistent state of the pandemic leading to anincrease in connectivity between teachers and students' devices, and the growth of online learning, is changing how students learn and the risks they have to manage themselves. The education sector typically employs some technical models to assess students' attitudes. Moreover, there is an ongoing intention to use online learning. In addition to technological factors, psychological factors were incorporated into the assessment. And intentions and attitudes are from a cognitive standpoint. Based on empirical research on online learning conducted among university students under epidemic normalization, the main goal of this paper is to examine the relationship between self-awareness and the willingness to use it continuously. During COVID-19 pandemic, the research framework created for this study was tested on 429 college students. The integrated Technology Acceptance Model (TAM) and Theory of Planned Behavior (TPB) model's hypotheses were empirically examined. It was found that self-awareness and the intention to use online learning during the epidemic are consistently related. Self-awareness profoundly and significantly impacts the decision to continue using online learning. The study's findings can gauge participants' intent to continue. This study's result can help assess the intention to continue to use online learning during COVID-19.This can help provide more valid assessment results beneficial for the management of online learning.
Benefitting from the rapid development of ICT, the Internet has become an essential part of our daily lives. It can link us to the outside world, irrespective of time and space. People are increasingly reliant on the Internet for information and also to fulfill other social needs. Scholars have conducted a series of studies based on big data on the Internet, such as sentiment analysis using big data to forewarn users of mental health problems (Bouarara, 2021; Mohammed et al., 2022), account security (Masud et al., 2020), construction of identification systems (Campomanes-Alvarez et al., 2019), conducting academic research (Fayoumi & Hajjar, 2020; Noor et al., 2020; Tembhurne et al., 2022), and even to predict global sustainable development goals (Chopra et al., 2022), and a host of other issues.
Internet technology develops rapidly, which accelerates the various industries’ integration. This has triggered a revolutionary wave in the field of education, bringing disruptive changes to the traditional education industry. In contrast to traditional learning in the classroom, online learning is more elastic, expanding the field of education, is not restricted by time and space (Cheng, 2012), and affects students positively (Allen et al., 2001). However, it also has some limitations. For example, students’ motivation and studying outcomes may decrease when studying online (Tang & Hew, 2019). Nevertheless, in spite of its shortcoming, online learning was seen as a potential alternative to traditional classroom learning before the pandemic (Liu et al., 2010), an adjunct to regular instruction.
However, after COVID-19 spread rapidly, online learning became the recognized way of teaching and learning, which also ushered in some challenges. The Newcastle pneumonia epidemic has interrupted the schooling of 1.6 billion students (UNESCO, 2020). This has forced educators to turn to online teaching and virtual learning overnight. Educational institutions around the world not only started offering online learning but also developed different tools to continue classes during the period of COVID-19. The evolution of these online-learning tools can facilitate effective learning. Consequently, it has become the primary teaching and learning approach since COVID-19 (Anderson et al., 2020).
Due to the COVID-19, most universities in China started distance learning under the government's requirement for uninterrupted teaching. Consequently, Students had to take online classes from home due to millions of teachers fast transitioning to teaching in front of computers (Bao, 2020). However, providing students with online learning tools does not ensure that the online learning process will be successful. The physical classroom interactions between students and teachers, which help with material comprehension, learning, and knowledge absorption, are eliminated by online learning. Assuming that all students are cognitively aware of what is expected of them is another foundational tenet of online learning. However, this assumption is not always valid.
Many attractions could be discovered on the same digital devices used for online learning, according to Cole et al. (2004), such as social media, entertainment, and other barriers that divert students' attention. In addition, not all learners are efficient or disciplined and therefore procrastinate rather than actively participate in the learning process. It is often observed that they are prone to cheating on assignments and exams and have a low value for learning. Considering the above, we realized that students are the main subjects of learning and that the study of online learning must take into account students' self-awareness to assess its effectiveness in providing them with the necessary knowledge and skills. In particular, the role of self-awareness is better reflected in students' learning attitudes and behaviors in a context where epidemics are normalized and students are not influenced by the teacher's supervision and the learning climate. Previous distance education studies have focused on students' attitudes and willingness to learn online, but there is relatively little insight into how students' self-awareness functions.
During COVID-19, Adedoyin et. (2020), Ali (2020), Bao (2020), Dhawan (2020), and others have analyzed the imperatives, implementation techniques, and strategies encountered associated with online learningby reviewing the previous literature. Without using a theoretical framework, several authors have examined the viewpoints, usage, performance, and attitudes of university students regarding online learning with a focus on the significance of online learning during the 2019 coronavirus outbreak (Aguilera-Hermida, 2020; Demuyakor, 2020; Al-Salman & Haider, 2021; Elzainy et al., 2020). Thus, it can be argued that several studies have used a theoretical framework to analyze their results, despite many studies looking into the application of online learning among students during the pandemic (Aguilera-Hermida, 2020; Demuyakor, 2020; Al-Salman & Haider, 2021; Elzainy et al., 2020), which limits their conclusions or insights and makes them challenging to replicate. There is an intense desire not only to provide scientific measurements about online teaching impact factors but also to employ rational models to interpret the causes.
Hence, this research seeks to address these questions, as follows.
- 1.
What's the individual's continued using intention of online-learning when adopted in the context of epidemic normalization?
- 2.
How does self-awareness affect the continued usingthe intention of online- learning consistently in the context of epidemic normalization?
- 3.
Can the strength of the new model explored be better interpreted?
TAM, one of the most influential frameworks for exploring technology acceptance and rejection issues (Davis, 1989a, 1989b), has been increasingly adopted in instructional settings (Al-Emran et al., 2018). The Planned Behavior Modelis a successful method of predicting intentions and behaviors. The difference is that the two models are explained in terms of individual and technological factors, respectively (Weskott, 2008; Awa, et al., 2012). Thus, the two models are complementary. The increasing number of studies have focused on integrating to study Internet and IT usage, such as predicting mobile banking adoption (Aldammagh, 2021) M-learning Acceptance (Gómez-Ramirez et al., 2019), and online shopping intention (Ha, 2020). Researchers have concluded that integrated models perform better than TAMs and TPBs separately (Bosnjak et al., 2006; Chen et al., 2007; Joo, 2013).
For these reasons, this study will consider merging the TAM and TPB to obtain more research results and practical insights. Meanwhile, using self-awareness as an external variable, we will explore the mechanism of self-awareness in online learning during epidemic normalization from the perspective of self-perception. Although China is currently one of the best-controlled countries in the world, local outbreaks still occur occasionally. Due to the frequent outbreaks in the province of Henan in 2021, it will be seriously affected. At the same time, it has a large student population base, and its level of educational facilities falls in the lower middle of the country, so it is more representative to study the behavioral willingness of Henan university students to receive distance education (Wang et al., 2021). Therefore, this study investigated the attitudes, continued use intentions, and influencing factors of distance education among college students in Henan Province. Further, the development of distance education in China provided new teaching models to underdeveloped countries during the epidemic.
The remaining portions of this essay are organized as follows: Section 2 reviews the theoretical foundation of the integration of self-awareness, the TAM, the TPB, and other related studies. Section 3 provides a comprehensive description of the study methodologies, including the research model, data usage, and variables used. Section 4 provides a summary of the findings, and Section 5 discusses the contributions, ideas, constraints, and suggestions for further research.
Literature reviewSelf-awareness and technology acceptance model (TAM)(1) Self-awareness
Self-awareness is a concept widely used in psychology and is the core of a person's self-consciousness. A defining characteristic of self-awareness is found in almost all conceptualizations of self-awareness i.e., individuals evaluate themselves according to salient criteria or goals, according to the theory of self-awareness (Duval & Wicklund,1972; Wicklund & Duval,1971). Researchers have shown that self-awareness activates self-relevant or self-evaluating thoughts in memory (Geller & Shaver, 1976), and the self is often used as an evaluation tool. Self-awareness helps a person experience negative or positive effects depending on whether the attention is directed negatively or positively (Wicklund, 1975). Thus, it is characterized by behavior, introspection, and self-evaluation. It is not difficult to see that self-awareness can help realize the attitudes and beliefs held by an individual (Gibbons, 1990).
People whois higher self-awareness are likely to act in normative ways than those without self-awareness. Increased self-awareness increases the likelihood of people engaging in behaviors that they perceive as normative in this context (Diener & Wallbom, 1976; Duval & Wicklund, 1972). Increasing self-awareness leads to enhanced consistency in attitudes and behaviors, lower self-esteem, greater motivation to complete tasks, and an associated increase in attributions of personal responsibility (Wicklund & Duval, 1971). For example, when self-awareness is present, students engage in fewer counter-normative behaviors such as cheating on tests. Furthermore, increasing self-awareness increases the likelihood of people engaging in behaviors they perceive as normative (Diener & Wallbom, 1976). Individuals with higher levels of self-awareness are more likely to take behavior into account when predicting the future, so their self-perceptions become more reliable and valid (Nasby, 1989). In contrast, individuals with low self-awareness experience poor career development, workderailment, and negative attitudes toward their work (Ashford et al., 1989). Self-awareness helps individuals recognize their stress to prevent burnout, become more self-disciplined, deepen their understanding of others (Burnard, 1988), improve self-efficacy (Engin & Cam, 2009), respond to others, discern their actions, and manage difficult situations (Rasheed, 2015).
(2) Technology Acceptance Model (TAM)
TAM is a critically important theory in understanding technology adoption by humans. Davis (1989a) proposed two critical variables:(a) perceived usefulness and (b) perceived ease of use. Specifically, they refer to the attributes of the technology that influence technology adoption or implementation (Venkatesh & Davis., 2000). Perceived usefulness (PU) and perceived ease of use (PEU) are both influenced by external variables, whereas attitudes influence actual system use. As defined by Davis (1989a, 1989b), perceived ease of use is an individual's belief that the use of a particular system would be free of physical and mental effort, and perceived usefulness is determined by the degree to which an individual believes a particular system will improve their job performance. As a general rule, TAM predicts that users will embrace new technology if they perceive its ease of use and usefulness as positive.
A review of the relevant literature reveals that, in many fields, TAM continues to be widely used among the various frameworks available because it is a cost-saving model and highly effective and robust in most technology acceptance studies (Wang et al., 2022; King & He, 2006; Lee et al., 2003). In educational contexts, it is a crucial model for explaining and predicting potential acceptance or rejection of IT technologies for emerging applications, it assumes both PU and PEU can accurately predict users' acceptance (Davis, 1989a).
(3) Self-awareness and TAM
TAM is rarely associated with psychological factors. Some researchers have tried to construct psychological variables of TAM, such as perceived satisfaction, learning style, and self-efficacy. Relevant studies have shown that the extended model proved appropriate, though studies have found no significant psychological differences in student satisfaction and acceptance of online learning, research tables clarify that TAM is affected by factors such as self-efficacy, enjoyment behaviors, and anxiety (Abdullah & Ward, 2016).
An extended general TAM for online learning was also suggested, and it was also discovered how these elements affected students' perceptions of it. According to Yu (2020) and Cheng (2019), sustained intention, perceived easiness, perceived usefulness, and enjoyment were all positively and directly caused by conformity behavior and self-esteem. According to social psychology studies, self-awareness is a fundamental component of self-esteem (William John Ickes et al., 1973).
Self-awareness as a psychological factor influencing TAM has been infrequently studied. Notably, some researchers have argued that perceived awareness and PU predict intention to use ICT, and there is a correlation between perceived awareness and PU (Sharma, 2020). A study of mobile banking intention use also argued that awareness positively correlates (Raza et al., 2017). In this era of information technology, university students are highly educated. Students with high levels of self-awareness also have strong learning abilities. In the event of an epidemic, online learning is the only means of learning without interruption. Students with a high level of self-awareness can perceive online learning and maintain their willingness to continue using it.
Based on the above studies, we incorporate self-awareness into TAM to extend past related research with examine the continued using intention of online- learning in terms of the theory. Accordingly, there are four hypotheses:
H1: Self-awareness positively affects PEU toward online -learning.
H2: Self-awareness positively affects PU of online- learning.
H3: Self-awareness positively affects the attitude toward online learning.
H4:Self-awareness positively affects the continued use intention of online -learning.
TAM was used to examine the behavioral intentions that affect online learning, focusing on the following determinants: PU, PEU, and attitude Critical factors for behavioral intentions are PU and PEU (Davis & Venkatesh, 1996). Researchers have found that PEU and PU influence teachers' attitudes toward technology (Lazim et al., 2021; Qu & Chen, 2021; Teo, 2012). In particular, PEU predicts the PU in online learning environments and e-learning systems (Lazim et al., 2021; Liu et al., 2010; Qu & Chen, 2021; Badri et al., 2016). Based on TAM-related studies, PU and PEU shape users' beliefs about the technology, predict users' attitudes toward the technology, and consequently, influence behavioral intentions. Current online learning platforms are designed with user-friendliness in mind and, therefore, generally accommodate a user-friendly and easy to use interface. PEU, regardless of the presence of the pandemic, boosts students' confidence and promotes positive attitudes toward online learning. Furthermore, the perceived ease of use of online learning during the pandemic normalization can help students overcome online's disadvantages learning and facilitate its perceived usefulness.
An extended general TAM for online learning was also suggested, and it was also discovered how these elements affected students' perceptions of it. According to Yu (2020) and Cheng (2019), sustained intention, perceived easiness, perceived usefulness, and enjoyment were all positively and directly caused by conformity behavior and self-esteem. Similarly, students' attitudes about online learning and their willingness to continue using it are positively influenced by how useful they feel it to be. Consequently, we put up the following hypotheses:
H5: PEU positively affects the attitude to accept online- learning.
H6: PEU positively affects PU.
H7: PU positively affects the attitude toward online learning.
H8: PU positively affects the continued use intention of online learning
Self-awareness and theory of planned behaviorTheory of planned behavior (TPB)The TPB was developed by Ajzen based on the TRA theory (Ajzen, 1991). It has successfully predicted and explained human behavior using various information technologies (Ajzen, 1991, 2002). Moreover, in TPB, some individual-level factors have been demonstrated as potential influences on the final behavior. As a result, TPB has been extensively used in many domains of personal behavior, including automotive and transportation, such as automotive and transportation (Fett et al., 2018; Tu & Yang, 2019), healthcare (Khayeri et al., 2019; Rahimdel et al., 2019) and education (Asadi et al., 2020; Wang et al., 2020).
According to this hypothesis, attitudes, arbitrary standards, and perceived behavioral control are all capable of predicting intentions. A person's favorable or unfavorable evaluation of the action in question is indicated by their attitude (A). Positive or negative attitudes also directly impact behavior intensity and beliefs about potential outcomes. The level of one's willingness to put forth effort when engaging in particular behaviors is measured by behavioral intention. Subjective norms are individuals' perceptions of action expectations. In contrast, behaviors are predicted by intentions and perceived behavioral control. Furthermore, the link between intentions and conduct is moderated by perceived behavioral control.
Specifically, subjective norms are related to others’ expectations. When an epidemic is normalized and an individual finds that everyone around them is engaged in online learning, he/she can perceive it as pressure and prefer to persist in the same behavior. A person's assessment of how simple or difficult it is to carry out the targeted behavior is captured by their perceived behavioral control (PBC) (Ajzen, 1991). It involves beliefs about the control factors that may facilitate or hinder them. As a result, control perceptions about resources and opportunities are critical factors in how behavioral control is believed to be exerted.
Self-awareness and TPBThe TPB examines the connection between attitudes, subjective norms, perceived behavioral control, behavioral goals, and conduct. It is a highly influential model for the study of human behavior prediction.– can predict behavioral intentions (Sheeran & Taylor, 1999). Other researchers, such as Ostroff (1993), have suggested the potential for environmental and personal factors influencing an individual's salient behavior, and normative and controlling beliefs about that behavior. Beliefs are often regarded as essential influences or prerequisites that affect attitudes (Engel, Blackwell, & Miniard, 1995; Han & Kim, 2010; Han & Kim, 2010). Specifically, individuals will have to confront the beliefs they hold when exposed to the blockade, the inconvenience of life, and the changes in learning styles in the pandemic environment. Self-awareness can make people aware of their attitude and belief which is why we incorporated it into the TPB model. In experimental social psychology, it has been demonstrated that increased self-awareness enhances the likelihood that individuals will engage in normative behavior, especially when they perceive themselves as brutal (Diener & Wallbom, 1976; Duval & Wicklund, 1972). Previous research has established that the essential variable of self-awareness, self-esteem, is an important indicator of attitudes, arbitrary standards, and perceived behavioral control (Cheng et al., 2016). Researchers argue that self-awareness can also have the same predictive effect on the above variables. During epidemic normalization, students with high levels of self-awareness were willing to put time and effort into their studies to finish their learning tasksby online-learning and reduce the studying pressure they perceived to obtain a sense of pleasure. Therefore, we propose hypotheses, as follows:
H9: Self-awareness positively affects the subjective norms toward online learning.
H10: Self-awareness positively affects the perceived behavioral control toward online learning.
Perceived behavioral control, in terms of students' ability to use online technologies, predicted their interest in using such technologies and intention to collaborate online. According to previous research, students' intentions to use information technology have been demonstrated to be significantly predicted by their attitudes (Taylor & Todd, 1995). It has been demonstrated that subjective norms may predict college students' intent to use online technologies, as well as pre-service teachers' intentions (Cheng et al., 2016; Teo, 2012). Moreover, it also found that perceived behavioral control predicted students' interest in using online tools and intention to collaborate electronically (Woo et al., 2011; Cheng et al., 2016). When the pandemic was normalized, the more students valued learning, the more positive their attitude toward online learning, the easier it was to complete their learning tasks, and the stronger intention to use consistently. Based on related studies, we will propose the following hypotheses:
H11: Attitudes positively affect the continued use intention toward online learning.
H12: Subjective norms positively affect the continued use intention toward online learning.
H13: Perceived behavioral control has a beneficial impact on continued use intention toward online learning.
In summary, we designed the structure of the study as shown in Fig. 1.
MethodData collectionWe performed the questionnaire to gather samples. Thus, An on-site survey was challenging to carry out during the COVID-19 pandemic. As a result, they were made available online via the survey. The questionnaire was administered to 500 university students from eight universities in Henan province, where the new crown epidemic was severe in 2021.
There were two sections to the questionnaire. First, there were seven-point Likert scale questions that ranged from 1 (strongly disagree) to 7 (strongly agree). The second part was composed of single-choice questions in which we investigated the basic demographic information, including gender, educational background, major, and income. Table 1 shows the respondents’ demographics to elucidate the structure. The questionnaire survey period was from January 25, 2022, to January 30, 2022. A total of 500 questionnaires were collected, of which 429 were used for data analysis, with 61.5% of females and 38.5% of males. Most of the students (79.3%) were from the countryside. Regarding educational background, 67.8% were science and engineering students, and 32.2% were literature and history students.
Table showing basic sample data.
Due to the epidemic, questionnaires were collected using Questionnaire Star online, usually using a cell phone based on a URL sent by the researcher. Online surveys have the following benefits: (1) No geographic restrictions on sampling; (2) Lower costs; (3) Quicker recovery questionnaire. Meanwhile, in the preface of the questionnaire, interviewees were notified that all data were used for academic research.
Therefore, students must complete the questionnaire truthfully to improve their reliability. Simultaneously, we used the IP addresses of intelligent communication devices to screen duplicate samples. To stop participants from returning to the survey many times. In addition, a few useless questionnaires that were either randomly completed, too brief, or contained duplicate values were eliminated.
In addition to removing unusable responses, the large sample size of this study significantly improved the statistical analysis' power and robustness. Content validity was confirmed by designing a core model based on previous correlation structures. Based on the literature and pre-validation scales, the items were developed for this questionnaire. The questionnaire was rigorously validated by several front-line teachers and statistical experts with extensive practical experience in online learning. Therefore, it was confirmed that the questionnaire had sufficient content validity.
Definition of variablesBased on the research objectives, the measurement items were research framework, operational definitions, and relevant literature. The core variables of the TAM adopt the concept of Davis (1989a) and the core variables of the TPB adopt the concepts of Ajzen (1991) and Taylor (1995). Self-awareness adopts the concepts of Zettle, and Govern & Rolffs. Thedetails are shown in Table 2.
Measurement items of constructs.
Research variable | Operability definition | Reference scale |
---|---|---|
Self-awareness (SA) | The capacity to recognize one's state, possible actions and the result of these actions on itself and its environment. | Zettle et al., 2018; Govern & Marsch, 2001; Rolffs et al., 2018. |
Attitude (AT) | Positive or negative psychological tendency towards online education | Ajzen,1991; Taylor & Todd, 1995 |
Subjective norm (SN) | Describing how a student reacts to other people's opinions of him or her when using online learning. | Ajzen,1991; Taylor & Todd, 1995 |
Perceived behavioral control (PBC) | The degree of behavior execution under subjective judgment. | Ajzen,1991; Taylor & Todd, 1995 |
Perceived ease of use (PEU) | The degree to which a student perceives online education to be user-friendly | Davis, 1989a |
Perceived usefulness (PU) | The degree to which a student thinks online education is helpful for learning is perceived usefulness. | Davis, 1989a |
Continued use intention (CUI) | The possibility of usage of online education by students in the future. | Ajzen,1991; Taylor & Todd, 1995 |
The partial least squares (PLS-SEM) method was used to analyze the data in this study.PLS-SEMis an iterative estimation that combines principal component analysis with multiple regression, and it is also a method of causal modeling. PLS-SEM is usually applicable to the following research: (a) research aimed at the development of exploratory theory; (b) studies whose research purpose is predictive analysis, (c) studies whose structural models are more complex, (d) studies that include one or more structural models that form conformations, (e) studies with small sample sizes based on fewer matrices, (f) studies whose data distribution does not conform to normal requirements. And (g) potential scores for subsequent analysis when the study requires them (Gefen & Straub, 2011; Shiau et al., 2019; Chin & Newsted,1999).
The PLS is a better tool for exploring causal relationships between structural variables than other approaches, and can deal with the model structure and measurement items (Hair et al., 2019; Petter et al., 2007). Furthermore, this method is suitable for dealing with the relationships between variables in anomalous data distributions because of its more relaxed requirements for variable normality and randomness. Aside from that, it has the capability of analyzing complex prediction models(Gefen&Straub, 2011; Hair et al., 2019; Chin & Newsted, 1999; Petter et al., 2007; Khan et al., 2019). These were also the reasons why PLS-SEM was chosen as the essential data analysis tool in this study. The PLS-SEM analysis and estimation in this study were performed in two stages. An analysis of the reliability and validity of the questionnaire was conducted in the first stage, and the passing coefficient and explanatory power of the structural model were calculated and verified in the second stage. These two stages were designed to verify structural relationships (Anderson & Gerbing, 1988; Hulland,1999). The present study explored the causal relationships among Self-awareness, subjective norms, perceived behavioral control, attitudes, behavioral intentions,perceived ease of use, and perceived usefulness; however, in the past literature, each construct contained many measures. Thus, the PLS is more appropriate than other SEM methods for revealing causal relationships among relevant variables, reducing measurement error, and avoiding covariance. Furthermore, it is suggested tha the sample size should be equal to 5–10 times the maximum number of model paths (Majchrzak et al., 2005). In this study, there were 429samples, and the maximum number of paths was 13, which met the requirements.
Outer model and scale validationGenerally, external model tests included the reliability for each item as well as the internal consistency, convergent validity, and discriminant validity for each construct. The reliability of the items was verified by loading them into the associated questions. Factor loading indicates the extent to which the structure can be measured through several questions with a threshold value of 0.6 for individual reliability (Hair et al., 2010). The combined reliability of each structure is listed in the following Table 3.
Reliability and AVE of the outer model.
According to Chin (1998), all constructs had composite reliability (CR) values greater than 0.7 overall, displaying internally consistent constructs.
Considering convergent validity, AVE for each construct was applied as an evaluation index for each measurement factor loading and composite reliability. According to Fornell & Larcker(1981), if AVE was > 0.5, the construct indicated an excellent convergent validity. The results showed that the AVEs of the latent variables in Table 2 ranged from 0.778 to 0.888, indicating that the study had good convergent validity.
To avoid common methods, the researcher took preventive measures (CMV). CMV may be reduced, resulting from a single respondent's cognitive information, using a self-reported scale. This study not only adopts an anonymous survey but also randomly assigned the items and attempts to hide the meaning of each item as much as possible. Obviously, the results of Tables 3 and 4 indicate that construct validity was considerable. In this regard, they also showed that CMV had no significant influence on the results.A further test used in this study was Harman's One-Factor Test, which can be used to determine the severity of CMV (Podsakoff & Organ, 1986). According to the exploratory factor analysis of the 26 items in this study, the first factor contributed 16.95% to the explanatory variance,and that it was a non-integrated factor. Therefore, CMV did not have an extensive impact on the outcome of this study.
Standardized factor loadings and cross loadings of the outer model.
Note 1: Yellow cells represent the factor loadings of scale items for each construct.
Moreover, we calculated the Goodness of Fit (GOF) to evaluate the overall quality of the model (Tenenhaus et al., 2005). GOF was calculated as follows:
GOF=⁎⁎It has been estimated that the GOF for this effect size is 0.659, more significant than the cut-off criterion of 0.36 (Wetzels et al., 2009).
Inner model and hypotheses testingA PLS analysis in this study was performed on the inner model to evaluate the hypotheses .To test the path of the coefficients and R², the researcher used an internal model. Path coefficients, which describe the strength and direction of the variable correlations, demonstrate the cause and effect, whereas the R²alludes to the percentage that explains the dependent variable, exhibiting the model's predictive capacity. Therefore, each of the significant path coefficients was valued using bootstrapping in this study. A resampling of the data was used because the values tested were found to be more accurate than commonly used approximate limits (Purvis et al., 2001). Hence, this strategy was utilized in this study to assess the significant correlations between variables.
Table 5 and Fig.2 display that self-awareness positively and significantly affects the perceived ease of use, attitude, subjective norm, perceived behavioral control, and H1,H3,H9, and H10 were supported (self-awareness→perceived ease of use: β = 0.421, t-value = 7.087; Self-awareness→attitude: β = 0.095, t-value = 2.919; self-awareness→subjective norm: β = 0.505, t-value = 9.680; Self-awareness→perceived behavioral control: β = 0.524, t-value = 11.565).However, self-awareness had no significant direct effect on perceived usefulness and continued use intention. Thus, H2 and H4 were not supported (self-awareness → perceived usefulness, β = 0.098, t-value = 1.887; self-awareness → continued use intention, β = −0.016, t-value = 0.459). According to the analysis, perception of ease of use affected attitude and perceived usefulness, supporting H5 and H6.(perceived ease of use → attitude: β = 0.388, t-value = 2.551; perceived ease of use → perceived usefulness: β = 0.842, t-value = 15.039). H7 and H8 were also supported in this study (Perceived usefulness → attitude: β = 0.519, t-value = 3.434; Perceived usefulness → continued use intention: β = 0.354, t-value = 3.282). The analysis results indicated that attitude and perceived behavioral control had a positive effect on continued use intention (attitude → continued use intention: β = 0.384, t-value = 3.241; perceived behavioral control → continued use intention: β = 0.178, t-value = 0.027).However, H12 was not supported (subjective norm→ continued use intention, β = 0.057, t-value = 0.460).
Summary of inner model results.
Hypothesis | Relationship | Path coefficient | t Values | P Values | Support hypothesis (Yes/No) |
---|---|---|---|---|---|
H1 | Self-awareness -> Perceived ease of use | 0.421⁎⁎⁎ | 7.087 | 0.000 | Yes |
H2 | Self-awareness -> Perceived usefulness | 0.098 | 1.887 | 0.059 | No |
H3 | Self-awareness->Attitude | 0.095⁎⁎ | 2.919 | 0.004 | Yes |
H4 | Self-awareness ->Continued use intention | −0.016 | 0.459 | 0.646 | No |
H5 | Perceived ease of use -> Attitude | 0.388* | 2.551 | 0.011 | Yes |
H6 | Perceived ease of use -> Perceived usefulness | 0.842⁎⁎⁎ | 15.039 | 0.000 | Yes |
H7 | Perceived usefulness -> Attitude | 0.519⁎⁎ | 3.434 | 0.001 | Yes |
H8 | Perceived usefulness -> Continued use intention | 0.354⁎⁎ | 3.282 | 0.001 | Yes |
H9 | Self-awareness -> Subjective norm | 0.505⁎⁎⁎ | 9.680 | 0.000 | Yes |
H10 | Self-awareness -> Perceived behavioral control | 0.524⁎⁎⁎ | 11.565 | 0.000 | Yes |
H11 | Attitude -> Continued use intention | 0.384⁎⁎ | 3.241 | 0.001 | Yes |
H12 | Subjective norm -> Continued use intention | 0.057 | 0.740 | 0.460 | No |
H13 | Perceived behavioral control-> Continued use intention | 0.178* | 2.211 | 0.027 | Yes |
Note 1:
An R2 analysis was performed to determine the combined effect of self-awareness on continued use intention. According to Fig 2, the R2 of continued use intention was 83.6%, which implies that self-awareness has an impact of 83.6% on continued use intention to use consistently through the TAM and TPB integrated model.
ConclusionDiscussionA novel model for predicting online learners' continued use intention was developed using the TAM and TPB merged. In addition, it investigated during the pandemic how self-awareness influences the continued use intention of online learning by using the TAM and TPB integrated model route. The results in this study demonstrated that self-awareness did not impact the continued use intention through perceived usefulness. However, perceived usefulness and attitudes of college students toward online learning might have a direct impact on the continued use intention. Additionally, self-awareness can indirectly influence individuals' willingness to continuously use online learning through perceived ease of use, perceived control behaviors and attitudes toward online learning. The model also explains, to a certain extent, the influence of self-awareness on the propensity to use online learning continuously.
The score of the continued use intention was 5.56 ± 1.24. Employing self-awareness as an external variable, this study explored a new model to identify the influence of self-awareness toward the continued use intention of online learning. It indicates that while self-awareness does not positively affect perceived usefulness, it does boost perceived usefulness via perceived ease of use. This might be attributed to the post-Epidemic period, when online learning became the primary instructional method. Students were required to take courses that required credit completion, so they did not have to consider whether or not they were helpful. Instead, perceived ease of use was more likely to engage students in their studies and thus maintaining their continued use intention. Similarly, while self-awareness positively influences the subjective norms, subjective norms do not positively influence the continued use intention i.e. students cannot achieve sustainability of online learning by external influences, in contrast to the perceived behavioral control, which can positively influence the willingness to continue using online learning. The differences between the two are external motivation and intrinsic motivation.
In addition, the R2 of the continued use intention was 83.6%, which is better than that of the TAM model (R2 = 0.475), and the TPB model alone (R2 = 0.683) (Cheng, 2019).
Instead, perceived simplicity of use was more likely to interest students in learning and hence sustain their intention to continue using the product.
Contributions and suggestionThis study investigates college students' continuous intention to use online learning during the epidemic in Henan, China, and also the reasons for it, and offering two critical contributions. First, previous studies on online learning have extensively reviewed individuals' attitudes toward online education as well as their tendency to use it consistently in terms of technical aspects such as platform characteristics, course characteristics, and perceived experiences, with little research on psychological influences. The perception of technology dimensions is desirable for short-term learning behavior (Chow et al., 2012). Furthermore, when online learning becomes the norm, students not only have to suffer from the negative emotions of epidemic stress but also need to overcome the non-favorable experiences associated with the shortcomings of online learning, such as instructor-learner distance, insufficient intimacy between learners, content-centered lectures, nonverbal communication constraints, unstable systems and the misuse of microphones (Han, 2021). Therefore, there needs to be increased attention given to students’ attitudes and beliefs about online learning. Second, the state of online learning as the primary mode of learning during the pandemic provides an excellent opportunity for scholars to analyse the effect of self-awareness on it. This study confirmed that during the pandemic, the psychological qualities of individuals were more prominent in their contribution to the willingness to continue using online learning. It also clarified that self-awareness had a substantial impact on perceived ease of use, attitudes, and perceived behavioral control, all of which influenced participants' propensity to continue utilizing online learning.
In response to the above findings, we propose the following strategies:
Communication can improve self-awareness. During the pandemic, teachers can understand the students’ attitudes and intentions toward online learning courses through communicating with them. Solving problems according to the student feedback can minimize the disadvantages of online learning. Teachers can help students recognize their perceptions, emotions, beliefs, and evaluations, and improve their self-awareness to enhance their willingness to continue using online learning.
Course quality and structured learning can ensure positive learning outcomes. Online learning is the future trend, and the pandemic has accelerated its popularity and development.In the future, with the same classroom atmosphere, the quality of the courses and the degree of individual engagement will define the quality of online learning and the future growth of individuals.
Improving the quality of distance courses and people's perception of the experience has become a point of consensus. However, as this study has confirmed, an individual's psychological quality determines the depth of participation in online learning. Thus, the impact of psychological aspects on online learning will become an important topic of study.
And future researchDespite the researchers' attempts to establish a rigorous study organization, research methodology, and data collecting, limitations were identified that might be addressed in future research.
First, there may be bias in data collection in a totally autonomous environment, affecting the external validity of the results, when utilizing an online questionnaire to gather data, Second, this study is essentially a cross-sectional study. As a result, the findings of the investigation can only account for individuals' existing propensity to use online learning on an ongoing basis. However, because different types of online learning carriers have different functional characteristics and match individuals in varied ways, individuals' long-term willingness to use online learning requires long-term observation and comparison. Comparing online learning based on different platforms can better assess psychological factors and correlations.
Third, since the variables in this study are all popular variables, no mediation analysis was performed. Lastly, given the differences in the effects of the epidemic prevention and control in China compared with other countries, more in-depth studies need to explore whether the research in this paper can be validated in other parts of the world.