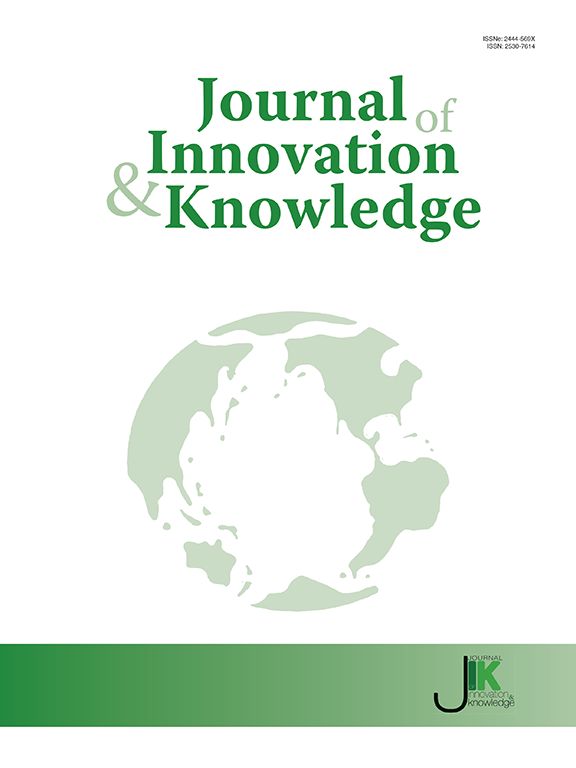
Editado por: Brij B. Gupta, Kwok Tai Chui
Última actualización: Julio 2022
Más datosIn the wake of the global disruption caused by the coronavirus pandemic, digital channels have emerged as a significant driver of business sales. As such, the value of digital business management has received considerable attention from industrial practitioners. Beyond conventional promotion techniques, collaboration with digital market influencers has shown significant potential for business organisations by creating a circle of trust. However, managing influencers in the business-to-business market is far more complex than in business-to-consumer markets, as it involves balancing business processes, competitive advantages, company image and risks rather than solely motivating customer engagement. It is, therefore, critical for organisations to carefully select key opinion leaders (KOLs). To address this challenge, this study introduces an intelligent multi-criteria KOL analytics framework that embeds the fuzzy best-worst method and the fuzzy technique for order preference by similarity to the ideal solution. The framework converts subjective and linguistic criteria, determines the attribute most relevant to the business, and then selects the most suitable KOLs for the campaign. A case study was conducted in partnership with a digital marketing agency in Hong Kong to demonstrate the feasibility and advantages of this approach. This model facilitates the evolution of digital business management by systematically identifying and assessing KOLs, contributing to significant changes in the digital business environment for companies.
The advent of the ‘digital business’ era has sparked a transformation in organisations, with emerging technologies and new analytics methods being implemented across various industries. Digital technologies have been incorporated into business practices and activities, significantly changing daily operations for many organisations (Martín-Peña et al., 2019; Reim et al., 2022). For instance, business transactions, information exchange and communication are now conducted via digital channels and platforms (Chatzoglou & Chatzoudes, 2016). Meanwhile, supplier relationships have substantially transformed due to outsourcing, globalisation and digitalisation (Gadde & Snehota, 2019). The preventive measures required by the COVID-19 pandemic, such as social distancing and working from home, have accelerated the evolution of digital business. Transactions and operations completed via online platforms – whether business-to-business (B2B) or business-to-consumer (B2C) – represent a shift towards digitalisation (Seetharaman, 2020). Therefore, digital business management is crucial for business transformation and survival, helping to maintain customer-perceived value in this turbulent environment.
Successful organisations typically start their digital journeys by focusing on marketing, leveraging digital marketing to reach customers across geographical boundaries (Saura, 2021; Verhoef & Bijmolt, 2019). The term ‘digital marketing’ refers to the adoption of digital technologies to acquire and retain customers, analyse customer preferences, promote brands and increase sales. More specifically, digital marketing is an adaptive, technology-enabled process of jointly creating, communicating, delivering and sustaining value for all stakeholders in business networks. Incorporating key opinion leaders (KOLs) is an effective digital marketing technique because KOLs can influence target audiences, boost sales and contribute to a positive company image (Cartwright et al., 2022). Over the past few years, the significant expansion of B2C e-commerce has led to the increasingly sophisticated utilisation of KOLs in B2C marketing. Utilising KOLs offers advantages such as building trust, reaching specific audiences, tailoring content and increasing profits. However, relatively few studies have discussed KOLs in the context of B2B digital business management. B2B digital marketing differs fundamentally from B2C digital marketing, focusing on organisational requirements and fostering strategic partnerships within B2B networks.
Managing digital businesses is more complex and dynamic for B2B than B2C businesses. For example, in the B2B context, customer involvement entails mobilising resources and competencies distributed across multiple organisations (La Rocca et al., 2016) while B2C focuses on leveraging customer emotions to drive purchasing behaviour, B2B emphasises cultivating long-term, loyal relationships, thus building crucial trust between stakeholders (Håkansson & Snehota, 2017). Ford et al. (2017) underscored the significance of sales and technical managers in developing relationships between companies, noting that such relationships cannot be developed unilaterally. In the digital realm, establishing KOLs representing specific domains of expertise has become an essential element of B2B digital business management. This approach is known as ‘influencer marketing’ (Backaler, 2018). Therefore, marketing strategies amongst B2B enterprises have shifted from traditional advertisement to the adoption of industry-specific influencers to cultivate a credible online presence. Table 1 compares B2B and B2C digital business management (Iankova et al., 2019; Pandey et al., 2020).
Differences between B2B and B2C digital business management in marketing.
Digital Business Management in Marketing | ||
---|---|---|
Aspect | B2B | B2C |
Objective | Focus on business performance, operational feasibility and domain expertise | Provide entertainment and emotional connections to drive sales |
Decision drivers | Business logic and financial considerations | Emotion |
Decision complexity | High; confirmation from different stakeholders (e.g. account managers) needed | Low; direct purchases made |
Customer relationship | Long-term; reliable relationship with a long sales cycle for supplying the products and services expected | Short-term; spontaneous, with one-time purchases expected |
Marketing measures | Social media for businesses, webinars, professional associations, online advisory services | Social media for leisure, video platforms, blogs |
Given their importance in B2B marketing, it is critical to establish appropriate criteria for selecting KOLs. One effective approach involves using data-driven methodologies to identify KOLs capable of influencing and shaping customer opinions (Bamakan et al., 2019). The multi-criteria intelligence aid (MCIA) methodology is valuable for effectively analysing linguistic data through quantitative analysis. By combining fuzzy set theory, artificial intelligence (AI) and multi-criteria decision aid (MCDA), MCIA offers a promising method for intuitively measuring relative importance in constructed hierarchies of various alternatives.
Consequently, it is worth exploring the utility of MCIA in digital business management to facilitate KOL identification and evaluation. Accordingly, this study investigates the role of business model innovations in the digital realm, proposing an intelligent multi-criteria KOL analytics framework (iMcKAF) for identifying and assessing KOLs to facilitate digital business management. Incorporating fuzzy set theory into MCDA enhances comprehension of the vagueness of human thinking and eliminates uncertainty in pairwise comparisons. When formulating practical applications, uncertainty is typically associated with applying the fuzzy best-worst method (BWM) and the fuzzy technique for order preference by similarity to the ideal solution (TOPSIS). The hybridisation of the fuzzy BWM and the fuzzy TOPSIS in the proposed iMcKAF, coupled with applying an AI methodology (i.e. fuzzy set theory), makes MCDA a more adaptable, practical and flexible method of evaluating KOLs. Therefore, the proposed framework provides a systematic method for selecting the most appropriate KOL to enhance B2B marketing, bolster B2B sales and optimise digital business management. In terms of analysis criteria, the expertise, novelty, influence and activity (ENIA) framework and performance metrics for digital business management enable the establishment of a comprehensive hierarchical structure for the MCIA (Li et al., 2013; Silva et al., 2020; Vieira et al., 2019).
Utilising KOLs in B2B marketing has enormous potential. However, a substantial research gap exists in understanding the appropriate criteria for identifying and selecting the most suitable KOL to promote B2B activity. Selecting the right KOL is a critical factor influencing the success of a marketing initiative built on this promotion strategy. Companies must rely solely on the decision-maker's knowledge and subjective experience without effective selection criteria. This process could lead to selecting a KOL unsuitable for collaboration, risking the campaign's success. This study aims to develop a structured system for categorising and identifying relationships between criteria, e.g. expertise, background, market experience and personal characteristics. In addressing this research gap, two issues emerge: (i) a dearth of literature focused on KOL selection in a B2B digital context with relevant selection criteria and (ii) the absence of a systematic approach for identifying and evaluating appropriate KOLs in the B2B digital market. To address these issues, the following research questions are posed:
RQ1
What is the hierarchical structure for quantifying KOL selection in B2B digital business management?
RQ2
What is the systematic mechanism for identifying and assessing appropriate KOLs to facilitate B2B digital business management?
This study makes the following contributions: (i) it develops a systematic framework for identifying and classifying relevant criteria for KOL selection in B2B digital business, and (ii) it establishes a decision support model to help B2B companies choose the most appropriate KOL for product promotion based on the selection criteria.
The remainder of the paper is organised as follows. Section “Literature review” reviews related research on digital business management, KOL selection and MCDA. Section “Research methodology” presents the architecture of the proposed iMcKAF. Section “Case study analysis” provides a case study that implements the proposed framework. Section “Results and discussion” reviews the results and discusses the framework's performance, comparing the proposed approach with the fuzzy analytic hierarchy process (AHP). Finally, Section “Conclusion” presents the conclusions of this study.
Literature reviewThis section reviews recent studies concerning (i) digital marketing models, (ii) KOL selection and (iii) MCIA. These represent the foundations for a theoretical framework of KOL analytics.
Overview of the differences between B2B and B2C digital marketing modelsThe rapid growth of digital business culture has created promising business development opportunities for companies to effectively and efficiently introduce new products and services (Guo et al., 2022). Many organisations are now turning to digital marketing to facilitate customer acquisition and establish a positive image both publicly and within business networks. Unlike traditional marketing, B2B digital marketing offers interactive online platforms that enable business marketers to accurately identify prospective customers, enhance information flow and foster trust in the business ecosystem (Krishna & Singh, 2018; Pandey & Gudipudi, 2019). Moreover, there is a distinction between B2B and B2C digital marketing at the strategic level. Most companies participating in B2B transactions are knowledgeable, tech-savvy and have solid business acumen (Lilien, 2016). B2B digital marketing focuses on value chain creation, professional content delivery, lead segmentation and customer engagement.
Conversely, B2C digital marketing focuses on brand building and end-consumer activities (Järvinen & Taiminen, 2016; Kannan & Li, 2017). In the digital age, strategies for achieving effective B2B digital marketing include business website development, search engine optimisation, online-to-office integration, social media promotion, pay-per-click campaigns and demographic targeting. The COVID-19 pandemic has accelerated the e-business transformation of B2B-centric organisations, with digital marketing adding value in this context.
Effectively leveraging social media and digital tools to promote a business to other companies is particularly crucial for driving sales (Fraccastoro et al., 2021). In recent years, KOLs have been discussed at length in the context of social media, with the term broadly referring to influencers who shape the decision-making processes and behaviours of a targeted group of customers (Lin et al., 2018). Influencers generally demonstrate high credibility, trustworthiness and professional expertise in their industry (Flodgren et al., 2019). According to Xue et al. (2023), KOLs in B2C digital markets strongly influence customer purchasing behaviours. However, research on KOLs in B2B digital marketing is still nascent (Crisafulli & Singh, 2022; Hudders et al., 2021). According to Mero et al. (2023), over 50 % of B2B companies lack the knowledge and ability to incorporate influencers into their business, as B2C strategies do not directly translate to the B2B context. This finding highlights a gap in understanding B2B digital marketing, particularly in selecting KOLs who can most effectively influence target customers and drive novel business competition.
KOL selection ontologyIn digital marketing, selecting the appropriate KOLs to promote a company's products and services is crucial. According to Oueslati et al. (2020), the KOL-identification process is complex, requiring a seven-phase framework: (i) understanding the domain, (ii) specifying available features, (iii) collecting data, (iv) constructing social networks, (v) modelling data, (vi) identifying KOLs and (vii) evaluating their performance. Effective KOL identification methods can employ six different approaches: descriptive, statistical and stochastic, diffusion process–based, topological, data mining and machine learning, and hybrid content mining (Bamakan et al., 2019). Cho et al. (2012) adopted a diffusion process–based approach, considering both diffusion speed and the increasing number of consumers who trust recommended purchasing decisions. Zhang et al. (2020) employed data mining to divide user leadership into user influence and activeness and design an action chain for opinion leaders. Park and Kaye (2017) proposed a hybrid content mining approach to examine Twitter content, aiming to identify connections between opinion leaders regarding network size and civil engagement.
Pozzi et al. (2016) outlined the major challenges surrounding KOL identification for B2B-centric organisations, which include identifying intrinsic and extrinsic characteristics and evaluating their market effectiveness and influence. This underscores the importance of considering multidimensional factors such as user activities, the follower–following network structure and the flow of influential power. Conducting an in-depth study that quantifies multiple criteria becomes essential for systematically identifying, evaluating and ranking KOLs for specific business organisations (Lin et al., 2016; Zhou et al., 2018).
Multi-criteria decision-making approachesMCDA approaches have been extensively developed to aid multi-criteria decision-making processes. These include service assessment (Langemeyer et al., 2018), portfolio evaluation (Pasaoglu et al., 2018) and the ranking of global market regions (Haddad et al., 2020). Four widely recognised methods of achieving multi-criteria decision-making have garnered significant attention from scholars: AHP, BWM, preference ranking organisation method for enrichment evaluations II, and elimination and choice expressing reality III. The selection of MCDA methods depends on problem features such as the nature of the alternative set, type of input set, nature of information, type of preference mode and type of decision. The decision process is also influenced by method features such as alternative ordering, criteria measure scale, preference structure, software availability and ease of use. While MCDA methods can robustly evaluate a set of alternatives using pairwise comparisons, they are less effective at intuitively capturing user preferences and understanding human thinking. The MCIA methodology – a composite of the MCDA, AI and fuzzy theories – is better equipped to handle imprecise knowledge in uncertain circumstances, making it suitable and practical for human-machine interactions (Frini, 2017). Fuzzy set theory can be embedded in existing MCDA methods to capture human intuition when making pairwise comparisons, with human expression modelled through fuzzy membership functions. For example, Sassi et al. (2015) applied MCIA to predict competitor decisions in complex business environments. The fuzzy BWM has also attracted increased attention due to its proficiency in pairwise comparisons and its ability to maintain consistency between judgements (Dong et al., 2021). This technique aims to capture the subjective judgements of decision-makers by using linguistic terms to specify the relative importance of criteria using fuzzy numbers (Amiri et al., 2021). In addition, the hybridisation of different decision analysis methods, such as AHP and TOPSIS (Bait et al., 2021; Bianchini, 2018; Leung et al., 2021), can effectively weight decision criteria to obtain a final ranking of alternatives.
Further improvements in these techniques, such as fuzzy TOPSIS, have incorporated multi-criteria decision-making (Nădăban et al., 2016; Nazim et al., 2022; Salih et al., 2019). Fuzzy TOPSIS was developed to evaluate multiple criteria of alternatives and rank them based on their similarity to an ideal solution, aiding the decision-maker in selecting the alternative that aligns most closely with their objectives (Solangi et al., 2021). Compared to typical MCDA methods, the MCIA approach provides a more robust mechanism for evaluating human intuition, which can be integrated with decision analysis methods. Therefore, they represent the ‘new era’ of multi-criteria decision-making.
Summary of literature reviewKOL selection is a significant research focus in digital business management due to its value in sustaining business growth and development, particularly in the current digitalised environment. Professionals well-versed in digital markets can extend their impact beyond physical sales and marketing activities, significantly influencing other stakeholders and supporting the development of holistic digital business solutions. Currently, KOL selection relies heavily on human knowledge and experience, resulting in subjective decisions that can lead to inappropriate KOL selection. The scarcity of literature identifying potential KOL selection criteria in the digital B2B market exacerbates this issue. Without the support of a comprehensive decision-making system, selecting the most suitable KOL can be complicated, difficult and discouraging for businesses. To address this research gap, this study expands the scope of the MCIA methodology to encompass the selection and assessment of KOLs in the digital B2B context. This contribution aims to enhance sales and build trust within business networks.
Research methodologyAn innovative business model was designed for KOL selection to facilitate digital business management. An appropriate selection mechanism can help boost sales and build trust within digital markets. The details of the research methodology appear in Fig. 1, identifying the three phases of the research: (i) identification and knowledge goal definition, (ii) design of the iMcKAF and (iii) case study and system evaluation.
Phase 1: problem identification and knowledge goal definitionIn the first phase, in-depth consultations with managers across various companies were conducted to identify the existing challenges in digital business management and solicit opinions concerning selecting suitable KOLs. One common concern highlighted by these discussions was the importance of selecting KOLs with relevant knowledge and experience in advertising and selling B2B products and services as a company representative. This is a key consideration, as the experience of some KOLs is limited to B2C advertising and sales. In response to the identified problems, specific knowledge goals were defined to facilitate decision-making in the KOL selection process. These data were then stored in the cloud database to design the iMcKAF.
Phase 2: design of the iMcKAFTo simplify pairwise comparisons when ranking potential KOLs, fuzzy BWM was integrated with fuzzy TOPSIS. Specifically, the weights of core and sub-criteria were assessed using fuzzy BWM, and KOLs were ranked and assessed using optimised weights and fuzzy TOPSIS. This allowed for the development of an efficient method of ranking a set of potential KOLs with fewer pairwise comparisons. Fig. 2 shows the three-tiered iMcKAF architecture.
Phase 3: case study and system evaluationIn Phase 3, a case study was conducted to evaluate and validate the iMcKAF model, with the case study company implementing the proposed model to support KOL selection. Subsequently, performance evaluation and validation were conducted for the iMcKAF to compare the reliability and consistency of fuzzy BWM with another traditional MCDA approach.
Tier 1: construction of a hierarchical structure for KOL analyticsBefore deploying the MCIA methodology, a hierarchical structure for KOL selection and evaluation was developed. The essential elements for building the hierarchical structure were established by reviewing B2B digital marketing, theories concerning opinion and market leaders and KOL performance measurements. To comprehensively evaluate the knowledge, skills and experience of potential KOLs, five criteria and 19 sub-criteria for assessing KOLs in the context of B2B digital marketing were defined (Table 2). Additionally, an industry investigation was conducted to identify potential KOLs by studying existing B2B business models and the availability of KOLs in the market. The MCIA methodology, combining fuzzy BWM and fuzzy TOPSIS, can be implemented as a practical and intelligent approach to ranking and selecting appropriate KOLs for B2B companies.
Criteria and sub-criteria for KOL selection.
Criterion | Sub-Criterion | Description | Refs. |
---|---|---|---|
C1: Domain of expertise | SC11: General business and marketing | Attraction of potential target users/organisations to increase companies’ profitability | Grissa (2016), Liu et al., 2019 |
SC12: Political science | Interest in launching political campaigns for promoting political missions | Horio and Shedd (2016), Nisbet and Kotcher (2009), Rocha et al. (2016) | |
SC13: Public health | Dissemination of information on public health and precautionary measures | Engez and Aarikka-Stenroos (2023), Flodgren et al. (2019), Gentina et al. (2017), Gupta et al. (2023), Srivastava et al. (2022) | |
SC14: Education | Provision of a broad spectrum of knowledge and tactics to the general public and business organisations | Koeslag-Kreunen et al. (2018), Li et al. (2013), Stylianou et al. (2022) | |
C2: Digital marketing experience | SC21: Products/services advertisement | Level of experience advertising and selling products and services as a company representative | Litterio et al. (2017), Singh et al. (2022) |
SC22: Business network | Level of experience building business networks with internal and external customers | Andersson and Axelsson (2018), Cartwright et al. (2021) | |
SC23: Social media | Level of experience using various types of social media tools and platforms | Korzynski et al. (2023), Rollins et al. (2014), Wang et al. (2017) | |
C3: Personal characteristics | SC31: Business connection | Ability to connect to specific business customer segments | Crisafulli and Singh (2022), Palo et al., 2019 |
SC32: Innovativeness | Ability to promote products/services in an innovative manner | Rollins et al. (2014), Wang et al. (2016) | |
SC33: Leadership | Leadership in the market influencing business customers in purchasing decisions | Fitriati et al. (2023), Krasikova et al. (2013), Nagy et al. (2017) | |
SC34: Responsiveness | Speed of customer interactions and responses to customer enquiries | Andersson and Axelsson (2018), Holliman and Rowley (2014) | |
C4: Message distribution channel | SC41: Webinar | Ability to hold online seminars with presentations and real-time interaction | Bussières et al. (2017), Terho et al. (2022) |
SC42: Website | Setup of a business website to build a positive image and reputation | Drivas et al.(2017), Simoes et al. (2015) | |
SC43: Online advisory services | Provision of highly customised professional advisory and consultancy services | Kajwang (2022), Nagy et al. (2017), Racherla and Friske (2012) | |
SC44: Social media hub | Adoption of digital social media hubs for content aggregation to effectively control brand image and exposure | Alavi (2016) | |
C5: Customer engagement | SC51: Positive comments | Positive engagement and interaction with customers regarding content related to products/services | Hanna et al. (2011) |
SC52: ‘Likes’ rate | Degree to which business customers engage with content | Itani et al. (2022) | |
SC53: ‘Shares’ rate | Willingness to share and recommend to others in their business network | Vieira et al. (2019) | |
SC54: Click-through rate | Ratio of views by customers interested in specific content | Corstjens and Umblijs (2012), Cowan et al. (2022), Tsimonis and Dimitriadis (2014) |
As the criteria are identified, each criterion is assigned a different weight based on relevance and importance. In Tier 2, a set of adjusted weights and vectors for criteria and sub-criteria is listed in the hierarchical structure. Weighting is determined via the fuzzy BWM, specifically through pairwise comparisons conducted with targeted business customers based on formulated linguistic terms. This facilitates the subsequent quantification of market preferences regarding the criteria employed in selecting KOLs.
According to the hierarchical structure shown in Table 2, the pairwise comparisons at levels 1 and 2 were conducted for criteria K and sub-criteria SK, where K = {K1, K2, …, Kn}, SK = {SK1, SK2, …, SKn}, SKi = {Ki1,…,Kini} and i = 1, 2, …, n. The comparisons amongst criteria or sub-criteria were formulated using the triangular fuzzy number Fi = {li, mi, ui}, where li, mi and ui denote the lower bound, midpoint and upper bounds of the linguistic term i for the triangular fuzzy numbers. According to Wang (2021), fuzzy triangular numbers can more accurately handle complex and multi-criteria decision problems. For example, the linguistic term ‘equally important’ is represented by the triangular fuzzy number (0, 1, 2). Table 3 shows the fuzziness transformation between linguistic terms and the triangular fuzzy numbers used in the fuzzy BWM, which includes graded mean integration representation (GMIR) and consistency index (CI) for the triangular fuzzy number. The GMIR refers to the graded λ-preference integration representation, where λ = 1/2, as Eq. (1) shows, and the CI is used to measure the consistency ratio (CR) of the pairwise comparison to ensure measurement consistency.
The pairwise comparisons at levels 1 and 2 consider two scenarios representing differences between (i) the five major criteria and (ii) the 19 sub-criteria. For every pairwise comparison, the best (most influential) and worst (least influential) criteria (i.e. Kb and Kw for major criteria and SKib and SKiw for sub-criteria) were identified. In contrast, the best-to-others and others-to-worst pairwise comparisons were conducted using defined linguistic terms, as shown in Fig. 3. Two evaluation vectors were subsequently formulated to perform (2n−3) comparisons between criteria or sub-criteria, where n is the number of criteria or sub-criteria. A nonlinear optimisation problem was formulated to determine the adjusted weights of criteria and sub-criteria. First, it was necessary to minimise the absolute gap ξ in the differences between ωb/ωi and the triangular fuzzy number of the best criterion Kb, and between ωi/ωw and the triangular fuzzy number of the worst criterion Kw. The values ωi, ωb and ωw denote the fuzzy weights for criterion i, the best criterion and the worst criterion. The corresponding objective function for minimising ξ is formulated in Eq. (2). The constraints for this minimisation problem are as follows: (i) constraint (3) assesses the absolute gap between the ωb/ωi and the triangular fuzzy number of the best criterion Kb, which is less than ξ; (ii) constraint (4) determines the absolute gap between ωi/ωw and the triangular fuzzy number of the worst criterion Kw, which is also less than ξ; (iii) constraint (5) stipulates that the sum of GMIR values for the fuzzy weights of all criteria is equal to 1; (iv) constraint (6) ensures that the sequence of triangular fuzzy number (l, m, u) is maintained; (v) constraint (7) ensures the non-negative integrality of the lower bounds of triangular fuzzy numbers and ξ.
Subject to:
When using fuzzy BWM, the CR can be measured to determine the consistency of the results. For example, a small CR value (i.e. ≤ 0.1) is preferred. The CR is calculated by dividing the obtained optimal ξ* value by the CI, where the CI is the maximum possible ξ value in a pairwise comparison. CI values between 0 and 1 can be determined by solving a quadratic equation at the highest level of a linguistic term used in the pairwise comparison, as shown in Eq. (8), where u0 denotes the largest of the upper bound fuzzy numbers of the linguistic terms used in a pairwise comparison.
The output of the above approach determines the optimal value of ξ and the fuzzy weights of the major criteria and sub-criteria in the hierarchical structure. This enables the derivation of the sets of adjusted weights for criteria and sub-criteria. The notations used in the fuzzy BWM model are summarised in Table 4.
Notations used in the fuzzy BWM model.
Notation | Description |
---|---|
K | Set of criteria |
Ki | The ith criterion |
SK | Set of sub-criteria |
SKi | The ith sub-criterion |
Kij | The jth sub-criterion of the ith criterion |
ni | The number of sub-criteria in the ith criterion |
Fi | The ith triangular fuzzy number |
li | The lower bound of Fi |
mi | The midpoint of Fi |
ui | The upper bound of Fi |
GMIR | Graded mean integration representation |
λ | Graded preference of the graded mean integration representation |
CI | Consistency index |
CR | Consistency ratio |
Kib | The best ith criterion |
Kiw | The worst ith criterion |
SKib | The best ith sub-criterion |
SKiw | The worst ith sub-criterion |
ωi | The fuzzy weight for criterion i |
ωb | The fuzzy weight for the best criterion |
ωw | The fuzzy weight for the worst criterion |
ξ | Objective value in nonlinear form |
R(wj) | GMIR values of criterion j |
u0 | The largest of the upper bound fuzzy numbers in the pairwise comparison |
ξ* | The optimal objective value |
Fuzzy TOPSIS (rather than the fuzzy BWM) was employed to determine the suitability of the KOLs for the designated business customers. This reduced the complexity associated with ranking a large group of KOLs based on the major criteria and sub-criteria. As with the fuzzy BWM, a conversion table between linguistic terms and triangular fuzzy numbers was required for fuzzy TOPSIS (Table 5).
In the fuzzy TOPSIS process, decision-makers at B2B digital marketing service providers evaluate a set of KOLs using the 19 level-2 sub-criteria to formulate the fuzzy decision matrix. Table 6 presents the notations used for fuzzy TOPSIS. The identified sub-criteria were then classified as benefit or cost characteristics. This refers to whether they are favourable or unfavourable in terms of the goal of the MCIA, namely, KOL selection in the B2B market of interest. Next, the normalised fuzzy decision matrix {npq} can be established, as in Eq. (9), where p and q represent the sets of KOL alternatives and sub-criteria. This is then multiplied by the fuzzy weights of the sub-criteria obtained via fuzzy BWM to establish the weighted normalised fuzzy decision matrix, as in Eq. (10).
Notations in the fuzzy TOPSIS model.
Notation | Description |
---|---|
ωq* | Adjusted weights for criteria q |
lq* | The lower bound of the adjusted weights for criteria q |
mq* | The midpoint of the adjusted weights for criteria q |
uq* | The upper bound of the adjusted weights for criteria q |
npq | Entries to the normalised fuzzy decision matrix |
l~pq | The normalised lower bound |
m~pq | The normalised midpoint of Fi |
u~pq | The normalised upper bound of Fi |
vpq | Entries to the weighted normalised fuzzy decision matrix |
E+ | The fuzzy positive ideal solution |
E− | The fuzzy negative ideal solution |
dp+ | Distance between E+ and alternative p |
dp− | Distance between E− and alternative p |
CCi | Closeness coefficient for KOL i |
Subsequently, the fuzzy positive ideal solution (PIS) and fuzzy negative ideal solution (NIS) can be computed, as in Eq. (11). This enables two vectors, E+ and E−, to be obtained as a collection of fuzzy PISs and fuzzy NISs for all sub-criteria.
The distance matrices for (i) E+ and each alternative and (ii) E− and each alternative can also be measured, allowing evaluation of the distances d+ and d− by summing the distances between all sub-criteria, as in Eq. (12), where d(vps,vs+/−)=13[(lpsk−lsk+/−)2+(mpsk−msk+/−)2+(upsk−usk+/−)2]. Thus, vectors d+and d− can be determined for all KOL alternatives.
Finally, the closeness coefficient CCi can be computed using Eq. (13) for the set of potential alternative KOLs. According to the derived CCi value, the decision-maker can rank potential KOLs for designated business customers. This enables the decision-maker to select the most appropriate KOL to promote the company's products and services and establish trust with target customers.
Case study analysisThis section presents a case study in which the effectiveness and feasibility of the proposed iMcKAF were tested through its implementation at a marketing agency in Hong Kong specialising in B2B digital marketing. The agency adopted the proposed framework to select the most appropriate KOL for maximising customer value in B2B transactions, business performance and brand building.
Industrial background and motivationDigital marketing is more complex for B2B than for B2C businesses. D.M. Digital Marketing Company Limited (an alias, hereafter D.M.), the B2B digital marketing agency under investigation in this case study, is a renowned digital marketing agency headquartered in Hong Kong, offering customised integrated digital marketing, social media marketing, creative marketing campaigns and influencer marketing solutions. According to D.M., B2B digital marketing demands professionalism and clarity. The company aims to establish a professional and trustworthy image amongst its intended business clientele, fostering collaboration and encouraging transactions. Regarding business growth, companies exclusively focused on B2B services and transactions prioritise profitability.
Moreover, effective B2B digital marketing strategies should motivate and persuade entire stakeholder groups and target customers to consider increasing marketing campaign budgets. Marketing agencies traditionally identify KOLs for specific business networks through professional associations, business exhibitions, conferences and face-to-face meetings. In the wake of the COVID-19 pandemic and its impact on global technological development, KOL identification has expanded to business-orientated social media (e.g. LinkedIn). While D.M. has adopted effective measures for identifying KOLs, recommending the most appropriate KOL to maximise benefits in the B2B market remains challenging. Within the B2B sector, establishing a systematic method for ranking potential KOLs and selecting the most appropriate one can effectively foster business collaborations between KOLs and companies, thereby enhancing synergy across business networks.
Implementing the iMcKAFThe criteria and sub-criteria for KOL selection (Table 2) were integrated into the analytical process for implementing the iMcKAF at the case study company. Subsequently, KOL selection can be incorporated as a service available on B2B digital marketing platforms. The overall implementation process requires four core steps: (i) collecting data from the B2B company, (ii) determining the adjusted weights for criteria and sub-criteria, (iii) collecting data from the decision-maker and (iv) ranking potential KOLs and selecting a suitable KOL.
Step 1: collecting data from B2B companiesFirst, the selection criteria and sub-criteria preferences of B2B companies were collected. These preferences indicate a company's intention to deploy the KOL for business development. B2B digital marketing agencies must understand the needs of B2B companies to position KOLs within these companies more effectively. To demonstrate the analytics process, T.C. Training Company Limited (an alias, hereafter T.C.), a B2B company that promotes training and certification programs to corporations via B2B digital marketing channels, was selected to rate the criteria and sub-criteria using linguistic expressions. T.C. suggested that criterion C1 was the best and criterion C4 was the worst. Table 7 summarises T.C.’s best-to-others and others-to-worst pairwise comparisons.
T.C.’s pairwise comparisons of the KOL selection criteria.
Linguistic evaluation of C1, C2, C3, C4 and C5 | |||||
---|---|---|---|---|---|
Best-to-others | C1 | C2 | C3 | C4 | C5 |
- C1 (Best) | – | SI | VI | AI | CI |
Others-to-worst | C1 | C2 | C3 | C4 | C5 |
- C4 (Worst) | AI | CI | SI | – | CI |
Note: The code names for the criteria and linguistic terms appear in Tables 2 and 3.
After rating the criteria, the five groups of sub-criteria were individually assessed to understand the detailed requirements of KOL selection. Table 8 summarises the linguistic evaluations of the sub-criteria within the five core criteria. After collecting opinions on the criteria and sub-criteria, a systematic approach (the fuzzy BWM) was applied to obtain the corresponding adjusted vectors supporting the fuzzy TOPSIS–based KOL selection process.
T.C.’s pairwise comparisons of the KOL selection sub-criteria.
Linguistic evaluation of SC11, SC12, SC13 and SC14 (C1) | ||||
---|---|---|---|---|
Best-to-others | SC11 | SC12 | SC13 | SC14 |
- SC14 (Best) | EI | VI | SI | – |
Others-to-worst | SC11 | SC12 | SC13 | SC14 |
- SC12 (Worst) | CI | – | EI | VI |
Linguistic evaluation of SC21, SC22 and SC23 (C2) | ||||
Best-to-others | SC21 | SC22 | SC23 | |
- SC23 (Best) | AI | SI | – | |
Others-to-worst | SC21 | SC22 | SC23 | |
- SC21 (Worst) | – | CI | VI | |
Linguistic evaluation of SC31, SC32, SC33 and SC34 (C3) | ||||
Best-to-others | SC31 | SC32 | SC33 | SC34 |
- C32 (Best) | CI | – | SI | VI |
Others-to-worst | SC31 | SC32 | SC33 | SC34 |
- C34 (Worst) | EI | VI | SI | – |
Linguistic evaluation of SC41, SC42, SC43 and SC44 (C4) | ||||
Best-to-others | SC41 | SC42 | SC43 | SC44 |
- C44 (Best) | VI | AI | SI | – |
Others-to-worst | SC41 | SC42 | SC43 | SC44 |
- C42 (Worst) | EI | – | SI | AI |
Linguistic evaluation of SC51, SC52, SC53 and SC54 (C5) | ||||
Best-to-others | SC51 | SC52 | SC53 | SC54 |
- C53 (Best) | SI | EI | – | VI |
Others-to-worst | SC51 | SC52 | SC53 | SC54 |
- C54 (Worst) | EI | CI | VI | – |
Note: The code names for the criteria and linguistic terms appear in Tables 2 and 3.
Based on the pairwise comparisons of the criteria and sub-criteria, the arrays of adjusted weights were computed by solving the optimisation problem mentioned in Section "Phase 2: design of the iMcKAF". For the core criteria, the fuzzy weights aggregated using GMIR are 0.5274, 0.1621, 0.1023, 0.0495 and 0.1587, which refer to specific fuzzy weights for C1, C2, C3, C4 and C5, respectively, with ξ* equalling 1.73. The GMIR weights of the sub-criteria for the five groups can thus be obtained. Table 9 shows that SC23, SC32 and SC44 were the criteria of greatest concern to T.C. The corresponding ξ* values for the pairwise comparisons of sub-criteria in the groups C1, C2, C3, C4 and C5 are 0.95, 1.00, 0.58, 0.69 and 0.95. The resulting fuzzy composite weights of the sub-criteria were calculated accordingly for the further fuzzy TOPSIS–based analysis, where the two membership functions were multiplied by each other. For example, the composite fuzzy weight of the sub-criterion SC11 is [0.4763, 0.5307, 0.5652] ⨂ [0.2606, 0.2720, 0.4068] = [0.1241, 0.1444, 0.2299].
Summary of composite fuzzy weights of sub-criteria.
Sub-criteria | Weight and vectors of core criteria | Fuzzy weight of sub-criteria | Composite fuzzy weights |
---|---|---|---|
SC11 | GMIR = 0.5274,[0.4763, 0.5307, 0.5652] | [0.2606, 0.272, 0.4068] | [0.1241, 0.1444, 0.2299] |
SC12 | [0.0672, 0.0672, 0.0955] | [0.032, 0.0357, 0.054] | |
SC13 | [0.0909, 0.1311, 0.1983] | [0.0433, 0.0696, 0.1121] | |
SC14 | [0.3869, 0.518, 0.5408] | [0.1843, 0.2749, 0.3056] | |
SC21 | GMIR = 0.1621,[0.0902, 0.1621, 0.2343] | [0.0682, 0.0783, 0.0852] | [0.0061, 0.0127, 0.02] |
SC22 | [0.1705, 0.3134, 0.4091] | [0.0154, 0.0508, 0.0958] | |
SC23 | [0.5114, 0.6268, 0.6818] | [0.0461, 0.1016, 0.1597] | |
SC31 | GMIR = 0.1023, [0.0662, 0.1006, 0.1454] | [0.0779, 0.1058, 0.1811] | [0.0052, 0.0106, 0.0263] |
SC32 | [0.5904, 0.5904, 0.5904] | [0.0391, 0.0594, 0.0859] | |
SC33 | [0.1337, 0.1883, 0.3732] | [0.0088, 0.0189, 0.0543] | |
SC34 | [0.0701, 0.0779, 0.1337] | [0.0046, 0.0078, 0.0194] | |
SC41 | GMIR = 0.0495, [0.0444, 0.0495, 0.0546] | [0.0638, 0.0918, 0.1345] | [0.0028, 0.0045, 0.0073] |
SC42 | [0.06, 0.0697, 0.0918] | [0.0027, 0.0034, 0.005] | |
SC43 | [0.1436, 0.2512, 0.3417] | [0.0064, 0.0124, 0.0187] | |
SC44 | [0.5791, 0.5791, 0.6184] | [0.0257, 0.0287, 0.0338] | |
SC51 | GMIR = 0.1587, [0.0696, 0.1621, 0.2343] | [0.0857, 0.1236, 0.1869] | [0.006, 0.02, 0.0438] |
SC52 | [0.1845, 0.3769, 0.4014] | [0.0128, 0.0611, 0.0941] | |
SC53 | [0.3647, 0.4646, 0.5098] | [0.0254, 0.0753, 0.1194] | |
SC54 | [0.0633, 0.0633, 0.0901] | [0.0044, 0.0103, 0.0211] |
After T.C.’s preferences had been collected, the decision-makers at D.M. selected the appropriate KOL. In this scenario, 10 potential KOLs underwent final ranking and evaluation based on 19 defined sub-criteria, enabling D.M. to assign appropriate linguistic expressions (Table 3) to each item. Table 10 summarises the linguistic terms assigned to these 10 potential KOLs by the decision-makers. According to the results, only the SC34 criterion characterises the cost feature (−), with all other criteria characterising the benefit feature (+). Linguistic expressions are represented by corresponding membership functions that can be analysed using fuzzy TOPSIS, as discussed in Section "Phase 3: case study and system evaluation".
Evaluations of KOLs by decision-makers based on defined sub-criteria.
Potential KOLs | ||||||||||
---|---|---|---|---|---|---|---|---|---|---|
1 | 2 | 3 | 4 | 5 | 6 | 7 | 8 | 9 | 10 | |
SC11 (+) | WO | VO | WO | SO | WO | MO | VO | LO | SO | MO |
SC12 (+) | MO | SO | MO | MO | WO | VO | LO | WO | WO | VO |
SC13 (+) | VO | VO | VO | WO | WO | MO | SO | LO | WO | LO |
SC14 (+) | VO | MO | VO | WO | VO | MO | WO | WO | LO | MO |
SC21 (+) | SO | MO | LO | LO | WO | WO | MO | MO | SO | VO |
SC22 (+) | LO | MO | SO | WO | LO | LO | LO | VO | SO | SO |
SC23 (+) | VO | MO | MO | VO | SO | LO | WO | MO | WO | VO |
SC31 (+) | SO | VO | SO | WO | LO | MO | VO | LO | VO | LO |
SC32 (+) | LO | VO | MO | SO | MO | MO | WO | MO | VO | WO |
SC33 (+) | WO | WO | SO | LO | VO | LO | WO | WO | LO | LO |
SC34 (−) | WO | VO | SO | LO | VO | SO | MO | LO | WO | VO |
SC41 (+) | VO | VO | MO | MO | VO | LO | MO | SO | WO | WO |
SC42 (+) | LO | VO | SO | WO | MO | LO | MO | VO | VO | WO |
SC43 (+) | WO | MO | VO | SO | LO | WO | WO | MO | VO | LO |
SC44 (+) | VO | MO | VO | LO | SO | WO | LO | WO | VO | MO |
SC51 (+) | MO | VO | LO | MO | LO | LO | VO | SO | VO | MO |
SC52 (+) | VO | LO | SO | WO | MO | VO | MO | WO | LO | LO |
SC53 (+) | WO | LO | MO | WO | MO | SO | VO | MO | SO | MO |
SC54 (+) | SO | WO | LO | VO | SO | MO | VO | VO | LO | VO |
Weighted normalised decision matrices were determined to analyse the ratings documented in Table 10, allowing the formulation of two vectors of fuzzy PIS and fuzzy NIS (i.e. E+ and E−). Based on E+ and E−, d+ and d− (representing the distance vectors from all sub-criteria to the fuzzy PIS or fuzzy NIS, respectively) were calculated. The closeness coefficients for all sub-criteria were obtained by aggregating the results of d+ and d− (Table 11) and ranking the potential KOLs accordingly. This process revealed that KOL 3 is the most appropriate choice for T.C.
Results and discussionThe feasibility of the proposed framework (iMcKAF) was verified using the case study analysis. This section investigates three aspects of the research outcomes: (i) performance evaluation, (ii) implications for KOL selection and (iii) theoretical and practical contributions.
Performance evaluation of the iMcKAFBuilding on the various MCDA methodologies, the proposed framework creates a hybrid of fuzzy BWM and fuzzy TOPSIS to achieve systematic functionality in KOL selection. Traditionally, integrating fuzzy AHP and fuzzy TOPSIS has garnered significant attention in solving multi-criteria selection problems. This MCDA approach proves promising, easy to apply, adaptable and effective in facilitating the selection process in business environments and addressing various industrial challenges (Agrawal et al., 2019; Bait et al., 2021; Leung et al., 2021; Sirisawat & Kiatcharoenpol, 2018). However, fuzzy AHP has several drawbacks, including its unsuitability for pairwise comparisons and difficulty maintaining measurement consistency (Guo & Zhao, 2017). When fuzzy AHP is replaced with fuzzy BWM, pairwise comparisons decrease from n(n − 1)/2 to 2n−3, improving the ease of using the MCIA methodology and enhancing measurement reliability and consistency. The case study also involved a comparative analysis of CRs between fuzzy AHP and fuzzy BWM (Fig. 4) that revealed that the reliability and consistency of the pairwise comparison using fuzzy BWM outperforms the pairwise comparison using fuzzy AHP. Therefore, the integration of fuzzy BWM and fuzzy TOPSIS warrants further investigation as an alternative to fuzzy AHP.
Implications for KOL selectionThe proposed framework empowers marketing agencies to recommend KOLs who are best suited to B2B companies based on their domain expertise, digital marketing experience, personal characteristics, message distribution channel and customer engagement. The case study results indicate that the proposed framework can effectively resolve existing challenges in KOL selection while systematically collecting and evaluating the perspectives and needs of both the B2B company and decision-makers at the marketing agency. Building on existing approaches to KOL identification in business networks, this study analysed the challenge of identifying KOLs in the B2B context, considering multidimensional factors. Rather than simply applying multi-criteria decision-making approaches, an element of AI (i.e. data fuzziness) was embedded to develop the MCIA approach. This approach strengthened the methodology's capacity to understand linguistic expressions by businesspeople and decision-makers, embedding intelligence in decision aid for KOL selection. This study has developed intelligent platforms that allow B2B digital marketing agencies to provide comprehensive solutions for business promotion, brand building and opportunity exploration by facilitating the selection of the right KOL to act as the company's public representative (Fig. 5). Combined with existing KOL identification mechanisms and digital transformations, such as robotic process automation, intelligent agents can be designed and developed to effectively and consistently contribute to decision-making processes in alignment with prominent initiatives of the digital age.
Theoretical and practical contributionsThis study synthesised the hierarchical structure of KOL selection via an extensive investigation of the recent literature, leading to the selection of five core criteria and 19 corresponding sub-criteria to contribute to a critical decision-making process in B2B digital marketing: KOL ranking and selection. A novel integration of the fuzzy BWM with fuzzy TOPSIS was proposed as an MCIA methodology to achieve this goal. This approach offers advantages, including simplifying pairwise comparisons, assessing data fuzziness, and improving measurement consistency. This integration can be extended to other B2B digital marketing domains that must consider multidimensional criteria, such as marketing strategy selection, market evaluation and performance measurement. Most prominently, this study has explored intelligent solutions for KOL selection, a critical step in B2B digital marketing for ranking KOLs and identifying the most suitable KOL to represent specific companies. This systematic approach generates ranking and selection results with high levels of consistency and reliability. A business philosophy involving appropriate market influencers can enable a company to effectively communicate with targeted customer segments and establish a circle of trust to induce synergistic business collaboration.
ConclusionSelecting appropriate KOLs to represent specific companies and organisations has consistently posed a challenge for digital business management. Due to the sophisticated and subjective nature of KOL identification, a systematic and intelligent approach is needed to effectively classify and select KOLs while also considering the requirements and preferences of B2B company stakeholders. This study resolves this challenge by introducing an approach to ranking and selecting KOLs using an MCIA methodology. By integrating fuzzy BWM and fuzzy TOPSIS, the proposed iMcKAF can help companies and decision-makers better understand the hierarchical structure of KOL evaluation and selection in digital marketing. In particular, the proposed approach transforms uncertain and subjective linguistic expressions into weights for evaluation criteria using fuzzy BWM. Then, fuzzy TOPSIS is used to facilitate ranking. A case study was conducted using a Hong Kong B2B digital marketing agency to test the performance of the proposed model. Using the proposed framework for KOL identification, the agency demonstrated its ability to assist companies in choosing the most suitable KOL to represent them. By enhancing KOL selection accuracy, companies can efficiently devise more effective marketing strategies to drive product sales, bolster their image and foster trust within business networks, leading to sustained benefits in the digital market.
However, this study has two limitations. First, as a proof-of-concept, the study only compared the results of the proposed framework with those obtained through fuzzy AHP and focused on past KOL data. Further investigation into the long-term performance of marketing campaigns built around the selected KOL is critical to obtain deeper insight into the usefulness of this framework in selecting KOLs. Future research should develop real-time monitoring and update KOL performance metrics. This could entail leveraging machine learning algorithms or predictive analytics to analyse the evolving influence of KOLs in dynamic contexts. Second, only five criteria and 19 sub-criteria were established to identify KOLs. Given the complex and dynamic nature of market environments, companies’ requirements for KOLs are subject to change. Incorporating additional criteria or sub-criteria would better align with companies' diverse goals and audience preferences across various industries and situations. This would increase the comprehensiveness, accuracy and precision of KOL selection while maintaining the adaptability of the model. Future research should incorporate a broader range of criteria to aid B2B companies in selecting KOLs.
CRediT authorship contribution statementH.Y. Lam: Conceptualization, Methodology, Writing – original draft. V. Tang: Data curation, Investigation, Writing – original draft. C.H. Wu: Formal analysis, Project administration, Writing – review & editing. V. Cho: Resources, Supervision, Validation.
The work described in this paper was partially supported by the Research Grants Council, Hong Kong SAR, under the Faculty Development Scheme (Project Ref. No.: UGC/FDS14/E04/21) and the Research Matching Grant Scheme (RMGS Project Acc. No.: 700011 & 700043), and the Big Data Intelligence Centre at the Hang Seng University of Hong Kong. Special thanks go to Dr P. Tsang, Dr P.P.L. Leung, and Mr Thomas Chen for their support.