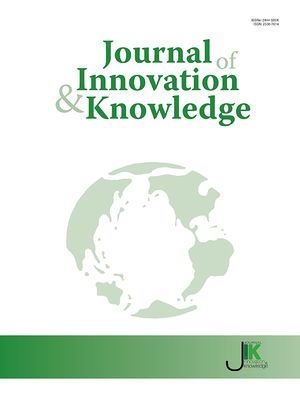
Editado por: Sascha Kraus, Alberto Ferraris, Alberto Bertello
Más datosSoftware development is an intensely knowledge-intensive industry that demands collaboration on behalf of everyone who participates in the process. Moreover, the successful accomplishment of the process depends on knowledge sharing and acquisition among the stakeholders. For this reason, organizations must understand how the knowledge-sharing process unrolls among employees. Nowadays, geographical distance is unavoidable, so researchers must consider subjective distance. This study provides an analysis of knowledge-sharing behavior within employees in software development roles in virtual teams, namely functional and technical, having subjective distance in mind. A research model based on a literature review was proposed to understand different dimensions of knowledge sharing. Afterward, the research model was empirically tested based on data collected in an organization with expertise in ERP software development through the implementation of a questionnaire. The method used to analyze the data was PLS-SEM. Results suggest that individuals share knowledge mainly to fulfill intrinsic needs; however, organizational culture also plays an essential role in knowledge sharing. The results are fundamental for both academics and practitioners to acknowledge how knowledge sharing occurs and, consequently, help in the decision-making processes regarding Knowledge Management within the companies.
Software development requires collaboration on behalf of everyone who participates in the process. Furthermore, the successful accomplishment depends upon knowledge acquisition, information sharing, and the minimization of communication breakdown (Ghobadi & D'Ambra, 2013). Given the cross-functional nature of software development, it is fundamental to understand the knowledge-sharing process among software development personnel. Knowledge sharing and using systems that allow digitalization of processes are part of the process of achieving innovation, thus part of digital transformation (Verhoef et al., 2021). Cross-functional teams are considered one of the company's internal enablers for developing dynamic digital transformation capabilities (Warner & Wäger, 2019). Currently, the geographical dispersion of team members is expected, which may neglect the socialization process as individuals cannot create shared mental presentations and routines (Nonaka & Toyama, 2015). Anwar, Rehman, Wang, Hashmani, and Shamim (2019) concluded that geographical distance negatively impacts knowledge sharing because it creates physical isolation. Given that geographical dispersion is unavoidable, Siebdrat, Hoegl, and Ernst (2014) introduced the subjective distance concept. Objective distance is measured in any unit of measure of distance, whereas subjective distance translates the team's perception of distance between its members (Siebdrat et al., 2014).
Although the impact of geographical distance has been studied, little evidence is available regarding the effects of subjective distance in software development. Hence, based on the subjective distance, the current research attempts to understand what factors affect the dynamics of knowledge sharing in the software development process in virtual teams, mainly in the context of global firms (Garro-Abarca, Palos-Sanchez, & Aguayo-Camacho, 2021; Skare & Riberio Soriano, 2021). The literature contributes to a more comprehensive picture of technology adoption in the context of COVID-19 by improving understanding of the dimensions' characteristics and interrelationships in times of crisis, namely by identifying ICT adoption pushed by the availability and readiness usage of technologies that support video-conferencing software, as well as the use of more sophisticated technology, that enables the alignment between company goals with technology goals (Wendt, Adam, Benlian, & Kraus, 2021). The best way to assess these dynamics is to study an organizational reality regarding multiple dimensions of knowledge sharing. Several authors have tried to understand knowledge sharing in organizations: however, understanding knowledge sharing in software development with subjective distance needs clarification. The study intends to analyze knowledge-sharing behavior in software development based on data collected in a software development organization with expertise in SAP Enterprise Resource Planning implementation.
The study aims to propose a research model capable of explaining the knowledge-sharing behavior in ERP software development based on a literature review, then empirically test the model to identify what represents the most determinant factors of the behavior. Afterward, the hypotheses were proposed. A structural equation model approach using Partial Least Squares (PLS) tested the hypotheses through the collected data. This study contributes to extending knowledge by proposing a model based on the theory to explain knowledge-sharing behavior. The results suggest that people are motivated by intrinsic factors when sharing knowledge. Nevertheless, organizational culture affected the knowledge-sharing process among knowledge workers. The findings provided by this study extend knowledge in the field and are useful to academics or practitioners. This work is organized as follows: Section 2 presents a literature review of knowledge sharing in organizations; Section 3 expounds on the proposed theoretical research model to explain the drivers of knowledge sharing; Section 4 includes the methodology used; Section 5 presents the analysis undertaken on the data collected, using PLS, and the discussion of findings, while Section 6 presents the conclusions. Finally, Section 7 identifies the limitations and recommendations for future works.
Literature reviewKnowledge workers' jobs require creating, distributing, and applying knowledge. Knowledge-intensive firms are organizations offering products and services based on the intellectual work of knowledge workers, which enable firms to achieve a competitive advantage (Hajishirzi, Costa, & Aparicio, 2022). Software development is an example of this industry. For this reason, organizations are starting to pay attention to Knowledge Management which allows individuals and teams to create, transmit and apply knowledge to overcome obstacles (North & Kumta, 2018). Besides, organizations not only process information but also generate knowledge through action and interaction with their organizational members (Sousa, Costa, & Aparicio, 2017).
Virtual teams are functioning teams having interdependent tasks (Gibson & Cohen, 2003). The members are geographically dispersed and accomplish their goals using technology-based tools. Regardless, virtual teams do not have to totally rely on technological tools to communicate and accomplish goals. They may meet face-to-face whenever necessary. Considering this, the degree of the virtuality of each team varies (i.e., comparing a team that meets face-to-face monthly and another that never meets face-to-face, the second one has a higher degree of virtuality).
Knowledge sharing is the process of transferring and sharing knowledge among individuals or teams to reduce the time spent on solving a business problem (Park & Lee, 2014). The quest for new knowledge requires personal commitment on behalf of the learner (Nonaka & Takeuchi, 1995). Nonetheless, barriers may arise in this process, e.g., people share knowledge with those close to them. Individuals interacting with one another engage in dialog and discussion that allow the creation of new perspectives (Nonaka & Takeuchi, 1995). New knowledge is generated by individual experiences and networks of individuals who meet and collaborate (McInerney, 2002). Researchers have found knowledge sharing brings benefits such as increased team performance and decision satisfaction (e.g., Mesmer-Magnus & DeChurch, 2009). Nevertheless, some authors defend that knowledge sharing is not always beneficial (e.g., McInerney, 2002). Personal and group ambitions must be met to avoid harm (Hendriks, 1999).
Software development requires expertise from all stakeholders, from business users to developers. Thus, knowledge sharing is fundamental to delivering quality software that meets the requirements of business users. Adapted from Ghobadi and D'Ambra's (2013) definition, cross-functional software development team members refer to people belonging to temporary workgroups responsible for completing a development project within a limited time frame and belonging to one of the two functional units (technical and functional departments). Functional personnel possesses knowledge about the system and the client's business processes. They collect the requirements through workshops with key users (Lech, 2014). They propose, develop, and configure documents and test business solutions according to the functional/technical requirements. Functional roles are responsible for writing requirement specifications, which act as a contract between the customer and developers.
On the other hand, technical personnel must understand the requirements of functional designs. They transform these requirements into technical designs under the supervision of functional personnel. Both functional and technical personnel require working cooperatively in a team, being critical to meeting the client's needs. The relationship between the corporation and the market is strengthened through increasing levels of social capital and transparency (De Bernardi, Bertello, Venuti, & Foscolo, 2020), and de Bem Machado, Secinaro, Calandra, and Lanzalonga (2021) relate that Industry 4.0 applications can impact the way companies distribute value to the market, and knowledge sharing within the organization.
Ghobadi (2015) presented drivers of knowledge sharing in software development teams used in conceptual models developed from 1993 to 2011 into categories and sub-categories. According to her research, people-related drivers are the most used to understand knowledge sharing in software development, while task-related drivers are the least used. The literature suggests that the various stages of KM (acquisition, conversion, application) are supported by the adoption of the digital business industry, promoted by management, leading to process innovation (Nwankpa, Roumani, & Datta, 2021). Nevertheless, this study lacks an understanding of how drivers impact knowledge sharing. For this reason, recent studies were explored. The papers proposing a conceptual model in multiple contexts were searched using the keywords (“knowledge sharing”) AND (Publication year ≥ 2012). Following that, a summary of drivers was performed (see Fig. 1).
Empirical studies using KS dimensions References.
The theoretical model proposed is based on different psychological theories and past studies. Table 1 summarizes the proposed model's constructs, definitions, and references. Afterward, the hypotheses are proposed based on the literature and its discussion.
Constructs, definitions, and references.
Construct | Definition | Reference |
---|---|---|
Subjective distance | An individual's perception of “how close or how far another person is.” | Siebdrat et al. (2014, p.2) |
Task interdependence | Each team member's tasks tend to depend on other team members' ability to accomplish their tasks since the tasks are interconnected. | Maruping & Magni (2015) |
Trust | State that allows building and maintaining relationships between people and, consequently, promotes KS behavior. | Park and Lee (2014) |
Intrinsic motivation | Individual's motivation to perform a certain behavior “for its own sake, out of interest, or for the pleasure and satisfaction derived from the experience.” | Lin (2007, p.3) |
Extrinsic motivation | Individuals’ motivation to perform a certain behavior is due to goal-driven reasons. | Lin (2007) |
Relational social capital | Refers to the “assets created and leveraged through relationships: trust, norms and sanctions, obligations and expectations, identity and identification.” | van den Hooff and Huysman (2009) |
Collaborative ICT infrastructure | Infrastructure facilitates the exchange of knowledge between people and overcoming social barriers. | |
Organizational KS practices | Practices that facilitate knowledge sharing in the organizational environment. | Hsu (2008) |
KS intentions | An individual is more prone to engage in some behavior when the intention to engage is stronger. | Ajzen (1991) |
Knowledge sharing | Process where the transferal of knowledge and experience occurs from one person to others to avoid repetition of mistakes. | Zheng et al. (2017) |
Individual impact | Perceived individual benefits that employees gain when sharing knowledge. | Urbach et al. (2010) |
Organizational impact | Perceived organizational benefits employees gain when sharing knowledge occur in the organization. |
Geographical distribution describes the team members' dispersion in different physical spaces (da Silva, Mosquera, & Soares, 2022; Ghobadi, 2015). Nonaka and Toyama (2015) suggested close physical interaction when sharing context and forming a common language among individuals for efficient knowledge creation and transfer. Moreover, the geographical distribution may harm trust-building among colleagues affecting knowledge sharing (Bouncken, Brem, & Kraus, 2016; Obsuwan, Chandrashekar, Kraus, Brem, & Bouncken, 2021) since these colleagues are uncomfortable with each other. Studies have found that the geographical distance of team members in software development negatively affects KS (Anwar et al., 2019; Rezaei, Ferraris, Busso, & Rizzato, 2021). Hendriks (1999) suggests that when correctly implemented by an organization, collaborative technologies overcome the difficulties regarding physical distance. Since geographical distance is more common, Siebdrat et al. (2014) proposed the concept of subjective distance. A study revealed the benefits and drawbacks of ICTs in terms of their ability to overcome physical distance and provide virtual work that connects people while also accommodating physical separation. Individuals may be concerned about the risk of infection from highly contagious diseases in crowded spaces, which are common in work spaces, even after physical distancing laws are removed and hybrid or completely physical labor is permitted (Wendt et al., 2021). Therefore, the following hypothesis is proposed:
H1. Subjective distance has a negative impact on trust.
Software development requires close interaction among team members because it has cohesive and integrated activities (Ghobadi & D'Ambra, 2013). These activities demand the ability to work cooperatively to meet the requirements. Task interdependence has significance to knowledge sharing because people consider goal interdependence (Pee, Kankanhalli, & Kim, 2010). Likewise, Pinjani and Palvia (2013) found high task interdependence allows team members to overcome their differences and build mutual trust. Organizations implement organizational wikis as knowledge management tools to enhance collaboration among the employees as wikis help employees who need guidance identify the people who possess the skills and knowledge they need to solve their business problems (Sousa, Aparicio, & Costa, 2010). These types of tools boost the socialization process as one may approach specialists easily and tackle the business problem with the specialist's help. Even though the individuals are not on the same project team, it is an opportunity for people to expand their network and build trust. Based on the discussion, the following hypotheses are presented:
H2a. Task interdependence has a positive effect on building trust.
H2b. Task interdependence has a positive effect on relational social capital.
Trust is a state that allows building and maintaining relationships between people and, consequently, promotes knowledge sharing (Park & Lee, 2014). Trust nurtures a substantial impact on knowledge sharing, positively affecting software development (Garro-Abarca et al., 2021; Wickramasinghe & Widyaratne, 2012). Team members trusting each other is positively related to knowledge sharing in virtual teams (Pinjani & Palvia, 2013). Given that virtual teams collaborate using collaborative technologies, Killingsworth, Xue, and Liu (2016) found that trust influences attitude toward knowledge sharing. Though, using vague definitions of trust may corrupt the research results. Care must be taken when comparing the studies where trust is involved since each study characterizes trust differently. In this study, trust is the state that allows building and maintaining relationships between people and, consequently, enables access to easily-built social capital. The following hypothesis is proposed:
H3. Trust has a positive effect on accessing relational social capital.
The motivator-hygiene theory explains what motivates employees' job satisfaction (Herzberg, 1976). Motivators are intrinsic factors that contribute to job satisfaction. Hygiene factors are extrinsic factors that contribute to job dissatisfaction. While motivator factors contribute to job satisfaction, hygiene factors will not contribute to job satisfaction. Nevertheless, when hygiene factors are unavailable, job satisfaction may be lower. In other words, adapting the theory, motivators contribute positively to certain behavior, and hygiene factors, when unavailable, contribute negatively to a certain behavior. Studies indicate meaning and enjoyment trigger knowledge sharing (Lin, 2007). Altruism (Obrenovic et al., 2020) and soft reward (Wickramasinghe & Widyaratne, 2012) are intrinsic motivations that impact knowledge sharing positively. Drivers such as reciprocity (e.g., Killingsworth et al., 2016) and hard rewards (Wickramasinghe & Widyaratne, 2012) are extrinsic motivations that positively impact knowledge sharing. Therefore, the following hypotheses are proposed:
H4. Intrinsic motivation has a positive effect on knowledge-sharing intentions.
H5. Extrinsic motivation has a positive effect on knowledge-sharing intentions.
Social capital refers to the actual and potential human resources available through the network of relationships owned by a person (van den Hooff & Huysman, 2009). Knowledge exchange in networks has multiple reasons: gaining esteem and exchanging favors (McInerney, 2002). Complex and interconnected business problems require collaboration across organizational boundaries, and thus managers and executives must focus on how to create and develop social networks. Organizations that promote a shared context where employees can engage with others, discuss new points of view, and dialog with each other often allow knowledge to circulate within the organization (Nonaka & Takeuchi, 1995). Apart from that, knowledge sharing involves two or more people to occur. According to van den Hooff and Huysman (2009), there are three facets to social dimension. This study focuses on relational social capital. The sense of community also positively impacts collaborative knowledge creation (Obsuwan et al., 2021; Rafique et al., 2022; Saleh Al-Omoush, Orero-Blat, & Ribeiro-Soriano, 2021). Additionally, it helps to build an identity and identification among the organization's employees by proving a common interest and an atmosphere of trust and appreciation of the value of knowledge. Communication and work style variations also affect the evolution of the innovation process in organizations (Bouncken et al., 2016). Ferraris, Mazzoleni, Devalle, and Couturier (2018) indicate that the use of Big data analytics has an impact on firm performance, proving the growing importance of IT-related talents to the overall competitiveness of modern and more digital businesses. The following hypothesis is proposed:
H6. Relational social capital has a positive effect on knowledge-sharing intentions.
Collaborative technologies allow connecting to other physically distant people fast and cheaply (Aparicio & Costa, 2012). According to Hendriks (1999), collaborative technologies are essential for helping share knowledge. Nevertheless, Hendriks (1999) emphasizes that strategies to leverage the benefits of collaborative technologies must be studied and adapted according to each organization. Information and Communications Technology (ICT) facilitates knowledge sharing when employees are familiar with the technology. Studies reported in the literature also indicate a relationship between the usage of ICT in the KM context as a digital innovation launching pad for new products, processes, and business models (Bresciani, Huarng, Malhotra, & Ferraris, 2021). Researchers have found that the intention to use collaborative technologies is connected to their perceived usefulness (Aparicio & Costa, 2012). Besides that, Sousa, Costa, and Aparicio (2017) found that perceived usefulness and perceived utility are determinant factors in the intention to use KM systems. A study by Sousa et al. (2010) found different clusters of employees who contribute to the organizational wiki. In the context of their research, the cluster that engaged the most in an organizational wiki was senior personnel.
On the other hand, junior personnel were the ones who contributed the least. When two or more team members are required to solve a business problem, these technologies help them achieve their objectives. Therefore, the following hypotheses are proposed:
H7a. Collaborative ICT infrastructure has a positive effect on task interdependence.
H7b. Collaborative ICT infrastructure has a positive effect on knowledge-sharing intentions.
Organizations must implement knowledge-sharing practices to allow the circulation of knowledge (Nonaka & Takeuchi, 1995). Hsu (2008) examined the impact of organizational knowledge-sharing practices on organizational performance and found that these practices positively affect organizational performance. Practices encouraging knowledge sharing benefit the organizations by increasing team performance and decision satisfaction (Mesmer-Magnus & DeChurch, 2009). Organizational knowledge-sharing practices aim to diffuse knowledge in the organization and develop human capital resources (Hsu, 2008). These practices also encourage knowledge sharing among employees, facilitating collaboration. Based on the discussion, the current study proposes the following hypotheses:
H8a. Organizational KS practices have a positive effect on task interdependence.
H8b. Organizational KS practices have a positive effect on knowledge-sharing intentions.
The theory of planned behavior attempts to explain human behavior (Ajzen, 1991). The theory argues behavioral intention is one of the factors explaining human behavior. An individual is more prone to engage in some behavior when the intention to engage is stronger. This intention derives from motivational factors. Additionally, non-motivational factors must be considered. Non-motivational factors represent individuals' actual control over their behavior. For instance, when a senior consultant wants to share knowledge with a trainee consultant but is unavailable, the intention does not turn into engaged behavior. The constructs depicted in this study represent both motivational and non-motivational factors. The findings of an investigation into the utilization of knowledge management systems (KMS) revealed that the goal of utilizing KMS favorably affected its usefulness in sharing information in their working duties (Sousa et al., 2017). The following hypothesis is proposed Considering the theory of planned behavior:
H9. Knowledge-sharing intentions have a positive effect on knowledge sharing.
People who engage in the processes of externalization and internalization must recognize the value of knowledge (Hendriks, 1999). As long as the person recognizes the value of knowledge, the person will commit to successfully transferring knowledge (Nonaka & Takeuchi, 1995). According to Lin (2007), enjoyment and meaning are why people share knowledge. Both parties involved in the knowledge-sharing process benefit from it (Zhu, Chiu, & Infante Holguin-Veras, 2018). The perceived benefits of people who engage in the knowledge-sharing process have been studied. KMS literature acknowledges the influence of digital innovation on company performance (Areekkuzhiyil, 2016; Di Vaio, Palladino, Pezzi, & Kalisz, 2021). When employing information systems, DeLone and McLean (1992; 2003) were among the first authors to establish these two levels of impact, which can positively affect individual and organizational performance. Knowledge exchange can have a positive impact on both levels. Individual impacts correspond to the individual worker's perception of the impact of information systems in this study for using knowledge-sharing platforms, as workers perceive that their usage influences their working performance. Likewise, regarding organizational impacts, this refers to how workers perceive that the use of information systems influences the performance of their colleagues. The individual's belief that their action towards information sharing influences the organization's overall performance correlates to the individual's experience with the organizational impact. In this study, the hypothesis can be drawn that knowledge-sharing behavior can positively affect the perceived individual and organizational impacts. Even during stressful periods, such as the Covid-19 pandemic, knowledge sharing improves employees' inventive performance and, consequently, the organization's performance (Rafique et al., 2022). The following hypotheses are proposed:
H10. Knowledge sharing has a positive effect on individual impact.
H11. Knowledge sharing has a positive effect on organizational impact.
Several researchers have studied the impact of knowledge sharing in organizations and found knowledge sharing brings personal and organizational benefits (e.g., Mesmer-Magnus & DeChurch, 2009). Nevertheless, people who engage in the knowledge-sharing process must recognize the value of knowledge to extract the maximum benefits (Hendriks, 1999). DeLone and McLean (1992) & Harr, vom Brocke, and Urbach (2019) tested the hypothesis that the effects of information technology (IT) on the performance of an individual would eventually have some impact on the organization. This line of reasoning is sound since a user who benefits from using a knowledge management system also helps an organization save time and effort when they do so. In organizational environments, only people who recognize the value of knowledge will commit to the knowledge-sharing process. Finally, the following hypothesis is proposed:
H12. Individual impact has a positive effect on organizational impact.
The proposed model has all types of driver constructs presented by Ghobadi (2015). Finally, Fig. 2 illustrates the conceptual model.
Research methodologyStructural equation modelingThere are two approaches to Structural Equation Modeling (SEM): covariance-based and the component-based PLS approach (Urbach & Ahlemann, 2010). The first approach requires a normal distribution of the data, while the latter works with any data distribution (Hair, Sarstedt, Hopkins, & Kuppelwieser, 2014). The primary reasons for using PLS-SEM are the acceptance of non-normal data, testing a complex model, and the usage of a small sample size (Hair, Hult, Ringle, & Sarstedt, 2016). For these reasons, the current research addresses the component-based approach PLS. A field survey was implemented to test the hypotheses. Therefore, this study required the sampling of knowledge workers in software development.
Sampling design processThe sampling design process contains five steps: target population definition, sampling frame determination, sampling technique selection, sample size determination, and sampling process execution (Malhotra, 2010). The target population was the knowledge workers in software development (with technical or functional roles). Then, the sampling unit was selected as a software development organization with expertise in SAP implementation. Mailing lists were used to retrieve the sampling frame. The selected sampling technique was the traditional sampling approach. The minimum sample size required for the proposed model was 169 (Hair et al., 2016). The survey occurred in a pandemic context, and the anonymous respondents belong to cross-functional, geographically dispersed teams.
Regarding the execution of the sampling process, a probability sampling, disproportionate stratified sampling, was selected. The target population was divided into two strata (stratification variable: role in the software development process). The main goal was to gather around 60% of the sample corresponding to functional roles and 40% to technical roles.
Research data collection instrumentThe measurement items were adapted from the literature and, when necessary, with further adjustments. Intrinsic motivation was adapted from Venkatesh, Speier, and Morris (2002); knowledge-sharing intentions and extrinsic motivation from Lin (2007); organizational knowledge-sharing practices from Hsu (2008); collaborative ICT infrastructure and relational social capital from van den Hooff and Huysman (2009); individual and organizational impacts from Urbach, Smolnik, and Riempp (2010); trust from Park and Lee (2014); subjective distance from Siebdrat et al. (2014); task interdependence from Maruping and Magni (2015); knowledge sharing from Zheng, Wu, and Xie (2017). A seven-point Likert scale ranging from 1 (strongly disagree) to 7 (strongly agree) was adopted in all constructs except the following, which are measured on a five-point Likert scale: collaborative ICT infrastructure, task interdependence, knowledge sharing, and subjective distance.
Measures were taken to minimize the measurement error (e.g., guarantee anonymity, share the questionnaire's duration). Besides this type of error, social desirability bias occurs when the respondent likes to appear to be something they are not to comply with moral and social responsibilities. After collecting the measurement items, the questionnaire was translated into Portuguese. A pilot study was conducted on ten people to gather improvement points. Respondents were requested to make notes about any questions they had difficulty with. After discussing the recommendations received, they were incorporated into the questionnaire. Finally, a prize draw of one Netflix card was announced.
Data collectionThe questionnaire was sent through e-mail during office hours. A total of 825 employees were contacted. After three weeks, a follow-up e-mail was sent to bring resistant participants into the study. As a direct result, a more reliable representation of the target population is possible. A total of 177 questionnaires were collected, of which seven were from people not belonging to the target population. The data collection lasted three months. A screening of the questionnaires collected was done to increase the accuracy and precision of the data. Afterward, each measurement item was codified. Statistical analysis was performed using SmartPLS 3. Of the sample of 170 respondents, 58% perform a functional role. Additionally, 105 respondents are male, and 64 respondents are female. Regarding job tenure, employees with tenure from two to four years were the most participative. Table 2 summarizes the profile of the respondents.
Results and discussionMeasurement model evaluationThe measurement model allows understanding the relationship between the model constructs and their indicators (Henseler, Hubona, & Ray, 2016). The Bias-Corrected and Accelerated (BCa) Bootstrap method was used as the data presents a high level of skewness. First, the indicator loadings were inspected to verify the items' reliability (Hair, Risher, Sarstedt, & Ringle, 2019). Item purification was done to one item. Regarding internal consistency reliability, Cronbach's alpha and Composite Reliability values had the recommended minimum threshold except for extrinsic motivation (see Table 3); however, it was kept in the study. Afterward, the convergent validity of the indicators was assessed through the average variance extracted (AVE). Values above 0.5 are considered good (Henseler et al., 2016) - AVE meets the minimum threshold (see Table 3).
Internal consistency reliability and convergent validity.
Then, discriminant validity was assessed. The shared variance for all constructs should not be larger than their AVE (Hair et al., 2019). Afterward, the heterotrait-monotrait (HTMT) ratio of the correlations was assessed. When HTMT values are above 0.90, discriminant validity problems are present. It was concluded that there are no discriminant validity problems. Furthermore, cross-loadings were assessed; every item presented high values of loadings regarding their construct than any other construct. After assessing the measurement model with satisfactory values, the structural model can be evaluated.
Structural model evaluationThe structural model allows understanding the hypothesized relationships between the constructs (Henseler et al., 2016). First, the variance inflation factor values of all sets of predictor constructs were analyzed to avoid collinearity issues; these presented satisfactory values (Hair et al., 2016). Afterward, the R2 values were examined as R2 measures the variance and reports the model's predictive power (Hair et al., 2019). Relational social capital and organizational impact present the highest values of R2 among all endogenous constructs (see Table 4). Nevertheless, R2 values of KS intentions and knowledge sharing are considered satisfactory as the model predicts human attitudes (Hair et al., 2019). Moreover, Table 4 presents the Q2 values of the endogenous constructs. Relational social capital has the highest Q2 value, followed by organizational impact. The results demonstrate clear evidence for the model's predictive relevance regarding the endogenous latent variables.
Fig. 3 illustrates the results of the structural model. The model explains 36% of the variance of the knowledge-sharing intentions. Intrinsic motivation (β^=0.41,p<0.01) and organizational KS practices (β^=0.20,p<0.01) have a positive impact on KS intentions. On the other hand, extrinsic motivation (β^=0.07) has no impact on KS intentions. The model explains 28% of the variance of KS. KS intentions (β^=0.52,p<0.01) impact positively on KS. Besides that, trust (β^=0.64,p<0.01) highly impacts relational social capital. Moreover, the findings provide empirical support for the mediating role of individual impact in the model. Knowledge sharing leads to individual impact; individual impact leads to organizational impact.
Regarding approximate model fit, the root mean square error correlation (RMStheta) is 0.14. According to Hair et al. (2016), values of RMStheta less than 0.12 indicate a good fit. Finally, Table 5 displays the summary of the findings.
Summary of the findings.
From the fifteen hypotheses proposed, ten hypotheses are empirically supported. Hypothesis 1 is supported (β^=0.51). Knowledge workers' perception of how close or how far another person is defines the degree of trust. Very little was found in the literature on the effect of subjective distance on trust; Siebdrat et al. (2014) suggest that subjective distance predicts better the degree of team collaboration than objective distance. Prior studies have noted the positive influence of trust on knowledge sharing (e.g., Kucharska, Kowalczyk, & Kucharski, 2017; (Rezaei et al., 2021)); however, little is known regarding the influence of task interdependence on trust. The findings suggested that task interdependence does not affect trust among the workers (H2a not supported). Nevertheless, the findings suggest task interdependence is associated with relational social capital (β^=0.18). Besides that, trust is strongly associated with relational social capital (β^=0.64). This experiment did not detect any evidence for the association of organizational KS practices with task interdependence (H8a not supported); however, collaborative ICT infrastructure is correlated with task interdependence (β^=0.21). This result may be explained by the fact that since the team members collaborate in virtual teams, collaborative ICT infrastructure allows the individuals to collaborate regarding interdependent tasks (Verhoef et al., 2021; Warner & Wäger, 2019). Interestingly organizational KS practices influence knowledge sharing and not ICT infrastructure; this might indicate that employees do not directly relate ICT with knowledge sharing but instead the formal practices existing in the way of working in virtual teams (Di Vaio et al., 2021; Nwankpa et al., 2021). Saleh Al-Omoush et al. (2021) found a significant and positive impact of an existent sense of community in collaboration and knowledge sharing (β^=0.487).
Referring to KS intentions, the findings did not detect any evidence for an extrinsic motivation to be associated with KS intentions (H5 not supported). This finding contradicts previous studies (e.g., Lin, 2007). Results indicate no relationship between relational social capital and KS intentions (H6 not supported); however, van den Hooff and Huysman (2009) suggest a small effect relationship between relational social capital and KS behavior. Moreover, Hau, Kim, Lee, and Kim (2013) findings indicate a correlation between social capital and KS intentions. As regards collaborative ICT infrastructure, hypothesis H7b is not supported, suggesting that it does not impact the KS intentions. The literature suggests that building capabilities (Warner & Wäger, 2019) leads to companies' competitiveness and performance (Ferraris et al., 2018), rather than just the simple existence of ICT adoption is a result of companies' goals with the market's goals about the business (Wendt et al., 2021). H8b is supported; organizational KS practices are correlated with KS intentions (β^=0.20). Finally, the most determinant driver of KS intentions is intrinsic motivation (H4 supported; β^=0.41). This finding broadly supports the work of other studies linking intrinsic motivation with KS intentions (e.g., Hau et al., 2013). The findings suggest there is a strong relationship between KS intentions and KS behavior (H9 supported; β^=0.52). Regarding the impact of the KS behavior, knowledge sharing has a strong relationship with individual impact (β^=0.33) and has an even stronger relationship with organizational impact (β^=0.47). Finally, the individual impact has a medium effect on organizational impact (β^=0.34).
Theoretical contributionsThe results suggest that intrinsic motivation is the most important driver in explaining knowledge-sharing intentions, followed by organizational knowledge-sharing practices. According to the study conducted by Urbach, Smolnik, and Riempp (2011), the quality of cooperation, as measured by the utilization of an employee portal, is the only quality characteristic that significantly affects both use and user happiness, and subsequently, individual impacts. Therefore, the quality of the collaborative capabilities offered by an employee portal appears to be a key success factor that influences an individual's level of performance. Al-Okaily (2021) found that only three antecedent factors are essential to the success of Enterprise Content Management Systems (ECMS): the quality of the information, the quality of the system, and the quality of the process. However, in their study, process quality is the only factor that influences ECMS usage as well as users' satisfaction with the ECMS. Regarding the three aspects of organizational impact, our findings imply that evaluating the success of an ECMS based on specific ECM objectives is important. Our contribution extends the understanding of knowledge sharing in digitized teams, as intrinsic motivation, extrinsic motivation, relational social capital, collaborative ICT infrastructure, and organizational knowledge-sharing practices explain 36% of the variance of knowledge-sharing intentions, knowledge-sharing intentions explain only 28% of the variance of knowledge-sharing behavior. It can be concluded that the intention to share knowledge is not the only reason that triggers the knowledge-sharing behavior. The findings suggest that collaborative ICT infrastructure helps in task interdependence; however, organizational KS practices do not contribute to task interdependence.
Moreover, the results do not support the effect of interdependent tasks on building trust. Nevertheless, the perceived distance of how far or close the colleague remains a crucial factor in building trust. Finally, as the study focuses on only one organizational reality, it can be concluded that organizational culture plays an enormous role in knowledge-sharing practices.
Practical implicationsThe findings of this study suggest intrinsic motivation is an important driver of knowledge-sharing intentions. Besides intrinsic motivation, organizational knowledge-sharing practices also contribute to knowledge-sharing intentions. Moreover, knowledge-sharing processes have a considerable impact on the organization's success. For this reason, human resources and managers must cooperate to understand the motivations of a potential employee to align the organization's goals with the worker's goals. Furthermore, and without exception, it is crucial to perceive if the potential worker fits the organizational culture. As a climate of trust is essential for an organization to prosper, managers should understand that the frequency of online meetings when teleworking does not reduce the perception of distance among the team members. Managers must focus on implementing measures to enhance trust in the organization. Besides that, the organization studied implements knowledge-sharing measures (e.g., seniors mentoring junior personnel) that successfully increase the knowledge-sharing intentions. Finally, the focus of management should be on how to mitigate the perceived distance among the workers. Because remote working is the new reality for organizations, adaptation is the key to success.
ConclusionsThe main goals of the research were to examine the knowledge-sharing behavior in software development in virtual teams and to identify the most determinant drivers of knowledge sharing. The objectives were achieved by proposing a research model based on the literature review and then empirically testing the proposed model in an organization with expertise in software development. Intrinsic motivation and organizational KS practices emerged as the most determinant drivers of knowledge-sharing intentions. Extrinsic motivation made no significant difference to the process. The results support the idea that knowledge workers share knowledge to fulfill their psychological needs. The findings of this investigation complement those of earlier studies. Moreover, evidence from this research suggests that knowledge workers do not share knowledge to achieve extrinsic goals, which is closely parallel with previous findings.
Furthermore, this study lays the groundwork for future research into understanding how knowledge workers perceive the impact of their contribution to knowledge sharing in organizations. Hence for future studies, it would be important to include corporate culture as a possible determinant of knowledge sharing. A limitation of this study is that it only analyzes one organizational reality based on a small sample; therefore, generalizing the findings must be carried out cautiously. Although the current research is based on a small sample, the findings suggest intrinsic motivation is the primary driver of knowledge-sharing intentions. For example, future research could help practitioners, and researchers better understand the sabotage of knowledge, particularly to uncover the characteristics that contribute to a greater understanding of the antecedents that may impede or enhance intra-organizational information exchange (Perotti, Ferraris, Candelo, & Busso, 2022). According to Connelly, Connelly, Černe, Dysvik, and Škerlavaj (2019), organizational cynicism stemming from employees hiding information from their coworkers worsens the unfavorable correlation between knowledge concealing and psychological safety. This finding supports their theory. A "knowledge saboteur," according to other research (Serenko, 2019), is someone who is driven by vengeance against a coworker or management they believe is useless or unproductive. Due to these recent findings, new avenues of study in a digitalized workplace, where the atmosphere may not be conducive to adequate information sharing, are suggested. Many workers started new careers in different fields during and after the first waves of a pandemic. Some also changed companies or even countries, as they were working remotely. This scenario encompasses new paradigms that need to be studied urgently, including how people adapt to new work environments and whether knowledge sharing through virtual offices helps them. How can businesses and organizations, in general, keep their IT staff from jumping ship at the first sign of a better offer from the market? How do individuals conduct themselves when working in nomadic environments? How can businesses compete with the IT giants when it comes to luring employees with expertise in information management, information systems, and data science to work for them? These are only some of the new paradigms that researchers need to investigate for science to contribute to the solution of the challenges posed by the features of the future of work.
We are gratefully acknowledged financial support from FCT- Fundação para a Ciencia e Tecnologia, I.P., Portugal, national funding through research grant UIDB/04152/2020 - Centro de Investigação em Gestão de Informação (MagIC).