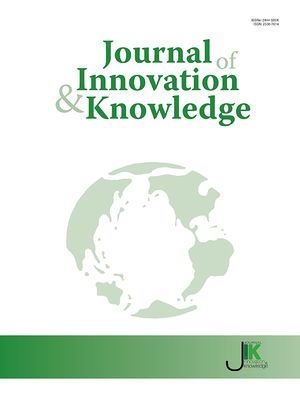
Editado por: Abbas Mardari
Más datosAfter the visions of Industry 5.0 and Society 5.0 were presented, a proliferation of artificial intelligence technologies have been applied to the financial field because AI develops fast, especially intelligent analysis methods for alternative financial data. However, the organic integration of the financial industry and the Internet of Things lacks relevant standards, and there is no appropriate work summary to coordinate the formulation of these standards. This work aims to effectively improve the reliability of information acquisition and the accuracy of data processing in the financial industry. In addition, this work also investigates papers and standards related to financial intelligence technology in recent years and statistically analyzes the evaluation indicators of AI research papers. Then, a standard evaluation framework is proposed for financial intelligence technology, which is evaluated for performance verification. The comparative experiments demonstrate that the prediction accuracy of the financial intelligence standard model reaches 95.44%, and the prediction error is substantially smaller than that of the other model algorithms. The financial intelligence standard model can make accurate predictions on alternative financial data and has high reliability and validity. The model can provide an experimental reference for intellectual development in the financial field and enable participants to improve work efficiency and standards throughout the process of developing intelligent financial technology.
Since its inception, Society 5.0 has been defined as a new society in the fifth stage, following the four early stages: hunting, agricultural, industrial, and information societies. It aims to create a people-centered society in which products and services are readily available to meet various potential needs and reduce economic and social disparities, enabling all people to live comfortable and vibrant lives (Romero-Castro et al., 2022). With the rapid development of science and technology, various industries are constantly improving themselves in keeping with the changes in science and technology. The development of science and technology also provides infinite power for the transformation of the times. Artificial Intelligence (AI), one of the most advanced technologies, has been widely used in various industries. Moreover, AI has become a technological weapon in daily life, achieving diversified applications, such as driverless cars, smart homes, and intelligent translation, fully realizing Industry 4.0. These are all masterpieces of AI, and AI has promoted a new round of industry and lifestyle changes (Wodecki, 2019). The current concept of Industry 5.0 is to harness the unique creativity of human experts in collaboration with powerful, intelligent, and precise machines. Many technology visionaries believe that Industry 5.0 will bring the human interest back to manufacturing (Guo et al., 2022). Industry 5.0 will significantly increase manufacturing efficiency, creating versatility between humans and machines, enabling interaction and responsibility for continuous monitoring of activities. Cooperation between humans and machines aims to promote production rapidly. Industry 5.0 can improve production quality by assigning repetitive and monotonous tasks to robots/machines and tasks requiring humans' critical thinking.
First of all, in industrial 5.0 production, the application of AI technology can save the use of the workforce and comprehensively improve production efficiency and production quality. The most critical AI technology has promoted the transformation of industrial production technology and the reform of industrial production. Secondly, the use of AI technology in the education industry can customize the personalized recommended teaching mode for students based on their characteristics and comprehensively transform the school's teaching mode, thereby improving the overall teaching quality of the education industry. Finally, the use of AI in the financial industry is also a breakthrough, providing crucial technical support for the development of the financial industry (Bossuyt et al., 2020; Saura, 2021).
In the context of Industry 5.0, the current high demand for information in the industry and the financial sector also put forward increasing requirements for AI. AI can quickly process big data and collect and process information in the financial industry. It simulates human functions to a large extent and even surpasses human parts, especially in the financial service industry (Shimada et al., 2020; David-West et al., 2020). First of all, in the service port, AI can provide customers with more appropriate and accurate services according to their needs, improve customers’ trust in financial service procedures, and increase the transaction rate of the business. Secondly, in terms of business operations, AI processes businesses more quickly and accurately and can take care of more businesses simultaneously, significantly reducing manual usage and improving business efficiency at the same time. Finally, in terms of risk management and control in the financial industry, AI can identify potential risks more quickly and accurately and deal with threats in a timely and effective manner, providing a solid backing for the steady development of the financial industry (Qi & Xiao, 2018).
Nowadays, driven by Industry 5.0, it is a breakthrough for intelligent analysis systems of financial investment in full bloom to formulate a unified standard based on alternative data. It is of great significance to improve the reliability of information acquisition in the financial industry and the accuracy of data processing. Using a systematic, standardized, and standardized financial investment intelligent analysis system can make the alternative data collected by Internet of Things (IoT) sensors accurate and effective; the core algorithm is flexible and adaptable; the decision-making results are rigorous and reliable so that the AI system is genuine. Therefore, it can serve the financial investment and achieve accurate data, high accuracy, and low risk. This article is based on alternative data collection to formulate a systematic, standardized, and standardized financial investment intelligent analysis system to improve the technical support for the development of the financial investment industry.
The overall structure of this research is as follows. Section 1 is the introduction to give a detailed description of the background of the development of financial intelligence and the application of AI algorithms and expound on the innovation and significance of this research. Section 2 is the literature review to analyze the research status of financial intelligence technology and detail the research progress of financial intelligence technology standards and the shortcomings and advantages in the research to highlight the research focus. Section 3 statistically analyzes the financial intelligence evaluation indicators from the perspectives of statistical hotspot time trend changes, keyword co-occurrence, etc. Section 4 constructs the financial intelligence standardization framework and evaluates the framework from the standpoint of evaluation principles, indicators, methods, and empirical analysis. Section 5 conducts a comparative experiment on the algorithm proposed here through empirical analysis and displays the recognition effect. Section 6 discusses the advantages and achievements of this study and explains its shortcomings and prospects.
Related workResearch Status of Financial Intelligence TechnologyIn the Society 5.0 vision, the demand for information and data in financial investment is enormous, and information and data in the financial investment industry are time-sensitive. Therefore, information collection and data processing need not only to be accurate but also to be fast (Li, 2020). The application of AI can meet the needs of the financial investment industry to a greater extent by collecting alternative data based on extensive data processing methods. Although the current financial industry's use of AI alternative data collection is imperfect, many studies have provided significant technical support (Zhao, 2021).
(Ho et al., 2018) conducted a questionnaire survey to obtain the data on agricultural value chains in Vietnam. They examined the relationship between market orientation and innovation by analyzing the data. (Arya & Rajkumar, 2019) showed that people's daily lives could not be separated from the financial field. People's production and life activities must deal with finance, which has important theoretical and practical significance for the research and analysis of financial markets. With the development of information technology, the economic field has accumulated a large amount of data in various formats. How to automatically analyze and process it effectively so that users are no longer submerged in the “ocean of data” and provide users with available information and knowledge that has significant value. In the research, (Leonov et al. 2020) mentioned that humanity had entered the era of the knowledge economy in the information society. Whoever has adequate information will become the ultimate winner, and the information in the financial field contains vast complex data from the past. In this case, but the form of the existence of this information is highly concealed, it is challenging to dig out useful information from many data cases. (Xiaojing, 2021) pointed out that alternative data is still a relatively unfamiliar concept in China. Compared with traditional data, alternative data refers to those that may affect investment decisions but are challenging to obtain from conventional mainstream financial data vendors. Especially in the economic field, alternative data has a tremendous supporting effect on the investment decision-making of financial institutions and can provide asset management companies with intelligent investment research services. (Caseiro & Coelho, 2019) took 228 start-ups from different European countries as samples to research the direct and indirect impact of business intelligence on performance through e-learning and innovation.
With technology development, alternative data has occupied an essential position in the financial industry, and artificial intelligence has also provided strong technical support for the financial sector. Therefore, the current research on the application of alternative data in financial intelligence technology is the leading technological channel for innovation and development in the financial industry. Thus, this work provides technical support for applying alternative data in financial intelligence technology and contributes to the invention and development of the financial sector in the vision of Industry 5.0 and Society 5.0.
Research Progress of Financial Intelligence Technology StandardsAI technology in the financial field has brought tremendous changes to the entire financial industry. Innovative financial services such as intelligent investment advisory, intelligent credit and monitoring, early warning, and intelligent customer service have expanded. Therefore, research on the application of AI in the financial industry is fundamental, and many studies have provided technical support for financial intelligence. Table 1 shows the application research of AI in the financial sector.
Research on the application of financial intelligence technology
No | Year | Authors | Name | Institution |
---|---|---|---|---|
1 | 2021 | (Fatehi & Hajiha, 2021) | The effect of financial intelligence on the four functions of operational managers and the financial performance of oil companies. | PalArch's Journal of Archaeology of Egypt/Egyptology |
2 | 2020 | (Magnuson, 2020) | Artificial financial intelligence. | Harv. Bus. L. Rev |
3 | 2021 | (Li & Xu, 2021) | Insights into financial technology (FinTech): a bibliometric and visual study. | Financial innovation |
4 | 2021 | (Jain, 2021) | How is AI transforming the financial sector? | Social Governance, Equity, and Justice |
5 | 2020 | (Wang et al., 2020) | Research on intelligence analysis technology of financial industry data based on genetic algorithm. | The Journal of Supercomputing |
6 | 2021 | (Corazza et al., 2021) | Predicting wet age-related macular degeneration (AMD) using DARC (detecting retinal apoptosis cells) AI (artificial intelligence) technology. | Expert review of molecular diagnostics |
7 | 2022 | (Goyal et al., 2022) | Artificial intelligence-assisted optical biopsies of colon polyps: Hype or reality? | Saudi Journal of Medicine and Medical Sciences |
8 | 2019 | (Pramanik et al., 2019) | The essence of digital transformation—Manifestations at large financial institutions from North America. | Future Generation Computer Systems |
9 | 2021 | (Grennan & Michaely, 2021) | Fintechs and the market for financial analysis. | Journal of Financial and Quantitative Analysis |
10 | 2021 | (Briggs, 2019) | Use AI to enhance human intelligence, not eliminate it. | Journal of Financial Planning |
As shown in Table 1, many studies have provided technical support for the development of financial intelligence and provided the primary reference information for the research on financial intelligence based on alternative data in this article.
Statistical Analysis of Financial Intelligence Evaluation IndicatorsTo be able to analyze the research related to AI evaluation indicators in the recent period, this work draws on the study of Zhu et al. “Deep Learning”, “Evaluation Index” and the author keyword index related to the evaluation index “Mean Absolute Error”, “Mean Square Error”, “Root Mean Square Error”, “Accuracy”, “Precision”, “Recall”, “F1 Score”, “Area Under Curve”, “Kolmogorov-Smirnov”, “mean Average Precision”, “Intersection over Union”, collected in the Web of Science core library and The 2152 literature data related to AI evaluation indicators are visualized and analyzed through Matplotlib, NetworkX, and Nxviz plotting libraries (Zhu et al., 2021; Hunter, 2007; Hagberg et al., 2008).
Analysis of the Time Trend Change of HotspotsBy analyzing the author's keyword frequency of 2152 documents in AI evaluation indicators, we selected the top 49 keywords with a word frequency of not less than 20. We carried out the word frequency statistics for these keywords according to different years—a heat map of the year distribution of word frequency. As shown in Figure 1, the frequencies higher than 1 in the figure have been marked with numbers, and different word frequencies are marked with different colors. The color bar from blue to red indicates that the frequency is from low to high. It can be seen from the figure that among these 49 high-frequency words, keywords related to AI (machine learning, deep learning, AI, neural networks, etc.), keywords related to evaluation indicators (accuracy, precision, AUC, recall, etc.) occupy the majority. In general, the keywords with a total word frequency of more than ten related to AI evaluation indicators are accuracy (331), precision (79), AUC (65), recall (45), RMSE (26), MSE (18), ROC (16), mean square error (15), mean absolute error (14), root mean square error (14), it can be seen that accuracy, precision, and AUC are the most commonly used AI evaluation indicators. However, these three indicators are often used in classification tasks. The three most commonly used AI evaluation indicators in regression tasks are root mean square error, mean square error, and mean absolute error (Jia et al., 2020; Verma & Pal, 2020; Lavanya & Parameswari, 2020). From the perspective of year distribution, as AI has been widely used in various industries in recent years, research on evaluation indicators has also increased year by year. The frequency of the keyword accuracy has increased significantly in the recent four years of research, and the frequency of each year in the past four years has exceeded 30. In addition, the frequency of precision, auc, and recall has also increased significantly in recent years (Pandey & Litoriya, 2020; Jacobs et al., 2020).
Keyword Co-occurrence AnalysisBased on the 49 author keywords with the highest word frequency, the work analyzed their relevance and obtained the keyword co-occurrence map by counting the number of times every two keywords in each article appeared together, as shown in Figure 2. The frequency of each keyword in the figure is different, and its color is also different. From blue to green, the frequency is from high to low. In addition, the number of co-occurrences between every two keywords is also represented by the color and thickness of the curve connecting them. The greener and thicker the curve means, the greater the number of co-occurrences between two words, and the yellower and thinner the curve means, the fewer the number of co-occurrences. The maximum number of co-occurrences is six keyword pairs: machine learning and precision medicine (155), machine learning and accuracy (106), precision medicine and AI (82), machine learning and precision agriculture (81), machine learning and deep learning (62), machine learning and AI (62). We found that these keyword pairs are mostly related to AI and AI application scenarios. Among them, machine learning and accuracy are related to AI evaluation indicators. Therefore, we can see that the most commonly used AI evaluation index in current research is accuracy. In addition, by analyzing the number of co-occurrences between keywords related to other evaluation indicators, we can also see that the top four keyword pairs with the most co-occurrences are precision and recall (35), accuracy and precision (22), accuracy and recall (11), accuracy and recall (10), it can be concluded that more of these evaluation indicators are used simultaneously in existing studies (Darabi et al., 2020; Koczkodaj & Wolny-Dominiak, 2017).
Financial Intelligence Standardization FrameworkNo AI algorithm is universally applicable to large and diverse datasets. Thus, people need to design algorithms according to the environment and the type of dataset in practical applications (Czako et al., 2018; Wolpert & Macready, 1997). Therefore, it is often difficult to evaluate the vastly different AI algorithms with a fixed data set or experimental environment. However, according to the characteristics of AI algorithms, we can still use deep learning as the object of AI algorithms and summarize a set of evaluation frameworks in a specific environment to play a particular guiding role for evaluation algorithms, as shown in Figure 3.
Evaluation PrinciplesWhen evaluating the pros and cons of an algorithm, it is often difficult to characterize an algorithm based on one or two indicators. The financial field has strict requirements on various performances of the algorithm. For example, in the prediction algorithm, the accuracy of the AI algorithm is very high. A vital evaluation index, and in classification algorithms, the error rate of AI algorithms is often placed first in the evaluation. As mentioned above, although it is difficult to form a set of universal evaluation standards for AI algorithms in different application scenarios, such as social platforms, when designing an evaluation plan, some formulation principles should be started (Czako et al., 2018; Tohka & van Gils, 2021) to guide developers to complete the evaluation plan. Formulate. Generally, the five elements of the algorithm's realizability principle, index priority principle, data availability principle, evaluation standard rationality principle, and evaluation result interpretability principle should be considered. The specific contents are as follows.
- (1)
The principle of realizability: The current financial problems that need to be solved should be suitable to be solved by AI algorithms. Otherwise, the following discussion will be meaningless. Generally speaking, the functions of AI algorithms include classification, clustering, and prediction. First, experts should evaluate whether the nature of the financial solution that needs to be customized from specific characteristics meets the characteristics of the above three problems, for example, for training, whether the data set has labels, whether the established functional relationship is linear or non-linear, how many calculation processes require manual intervention, and so on. The AI algorithm that needs to be designed to solve financial problems should be theoretically achievable. Otherwise, it will cause a waste of resources.
- (2)
Index priority principle: The types of AI algorithm indexes that can be evaluated are very diverse. Taking all evaluation indexes into account for a single project will significantly increase the workload, and it is also challenging to design an evaluation plan. Therefore, we should first select evaluation indicators for the algorithm. For example, prediction algorithms have high requirements for accuracy and real-time performance. When evaluating such algorithms, priority should be given to relevant evaluation indicators. In addition, the security of AI algorithms needs to be considered first for financial management. Therefore, the priority of evaluation indicators is often an essential basic work for a set of evaluation programs.
- (3)
Data availability principle: As an essential requirement for evaluating AI algorithms, sample training data sets are also necessary for financial AI algorithms. Since many financial-related data are compassionate, if the data is not processed as the primary content of the evaluation algorithm, it will cause the risk of data leakage to stakeholders. If the information is desensitized, the original relevance of the data will be affected. It will also make the data lose the function of the evaluation algorithm. Therefore, when processing data sets for evaluating AI algorithms, it is necessary to consider data desensitization and, more importantly, to keep the actual usability of the data.
- (4)
The principle of the rationality of evaluation standards in the social platform: There are many indicators to measure the performance of AI algorithms, such as the algorithm's efficiency, the accuracy of the classifier, the convergence speed of the function, and so on. When evaluating the current algorithm, you should select reasonable pros and cons metrics for evaluation so that the evaluation results are valid and effective for the application scenarios of the algorithm, instead of recommending available algorithms and using specific favorable metrics for assessment. In addition, the same evaluation indicators may have different importance in different application scenarios. For example, it is the same to verify the classification correctness of the classifier. The loss caused by some classification errors is minimal, and some may cause colossal market volatility. Therefore, the evaluation criteria for AI algorithms in financial applications should be carefully selected, and the evaluation criteria should not only include numerical indicators such as error and probability. However, they should also be ranked according to the specific application scenarios and effectively evaluate the pros and cons of the algorithm while reducing unnecessary workload.
- (5)
The principle of interpretability of evaluation results: The evaluation criteria of AI algorithms are often numerical indicators. These indicators are usually challenging to understand for people unfamiliar with related industries. For example, the effectiveness of financial AI algorithms does not only require recognition by algorithm engineers but also by stakeholders in financial-related industries. Therefore, the evaluation results should be explained in an easy-to-understand manner so that other relevant professionals can also have an intuitive understanding of the algorithm's performance.
Under the prospect of Industry 5.0 and Society 5.0, traditional financing channels, including financial markets and banks, provide companies with an essential source of funds. With the production of substantial alternative data, the financial industry is essentially a data-driven informatization war, which captures value in time from data. Trading signals (Allen et al., 2013). Because alternative data has the characteristics of a large amount of data, high real-time performance, and many types of data, it is also challenging to obtain. Financial institutions generally obtain alternative data in three ways: (1) Direct purchase from alternative data providers. (2) Obtained by self-built crawler team. (3) Obtained using data acquisition software. Among them, the data purchased from alternative data providers have reliability characteristics.
Types of Alternative DataCompared with traditional financial data such as fundamentals, finance, and historical market conditions, under Industry 5.0 and Society 5.0, alternative data is an essential supplement to conventional financial data, providing investors with broader trading ideas and more dimensional analysis perspectives. (Kolanovic & Krishnamachari, 2017) divided alternative data into several categories:
- •
Individual data, such as social media, news, Internet searches, etc.
- •
Business process data usually becomes business behavior, such as transaction behavior, company data, government agency, etc.
- •
Sensor data, such as satellites, geographic location, weather, etc.
- •
Currently, alternative data is mainly sensor data. Some satellite imagery or video feeds have been widely used. They include monitoring reports on oil tankers, tunnel traffic activities, or parking lot usage. Divide alternative data according to different types and stages:
- •
Large volume: The data scale and transmission volume are huge. According to an IDC report, there will be 33ZB of data worldwide in 2018. This number is expected to increase to 175ZB in 2025, which depends on improving computer computing power and the improvement of storage device technology (Yuan et al., 2020).
- •
High real-time: data acquisition and transmission are real-time or close to real-time. For example, the disclosure time of the annual report of a listed company was from January 1 to April 30 each year. With time delay. Through real-time monitoring of the company's online sales for the whole year of last year, investors will know the company's revenue in the previous year in January.
- •
Various types of data: There are many alternative data forms and structures. For example, include numeric values, pictures, text, audio, video, and other data types.
The demand stage of financial decision-making based on alternative data and assisted AI algorithms is to understand the specific requirements of users and project applications such as functions and performance through investigation and analysis, and finally determine the functional needs, non-functional requirements, and what should be met the stage of design constraints (Dessaint et al., 2021).
A structured analysis model of quality indicators is created based on considering alternative data's internal and external influences and the reliability of AI algorithms in the financial industry, combined with actual application scenarios. AI is used as a strategic policy based on multi-source heterogeneous financial data. According to the strategic approach, the quality target is determined. A set of reliability evaluation index systems is given in combination with the model, described in the next section.
Relevant Evaluation Index SystemFor the Classification Algorithm ModelAssuming that the sample software test set in the financial industry contains malware and standard software, all normal software needs to be marked as standard software by the intelligent detection system and vice versa. Among them are True Positive (TP), False Positive (FP), True Negative (TN), and False Negative (FN), as shown in Table 2.
- •
True Positive (TP): Normal software is correctly marked as normal software by the system.
- •
True Negative (TN): Malware is correctly marked as malware
- •
False-positive (FP): The intelligent detection system incorrectly keeps malware as regular software.
- •
False Negative (FN): The smart detection system incorrectly marks standard software as malware.
Accuracy is expressed as Eq. (1):
Precision is expressed as Eq. (2):
Recall is expressed as Eq. (3):
F1 is expressed as Eq. (4):
For Regression Algorithm ModelMean Absolute Error (MAE) is expressed as Eq. (5):
where actualk is the true value, and predictedk is the value predicted by the model algorithm, which usually reflects the actual situation of the predicted value error.Evaluation MethodAccording to the intelligent technology evaluation framework based on alternative financial data and the developed evaluation indicators, this section proposes a general evaluation method for the alternative financial data smart technology, from determining the evaluation object, forming the evaluation team, selecting the evaluation index, and constructing the evaluation. The system, the evaluation process's implementation, and the comprehensive results’ determination are carried out in 6 steps.
Determine the Evaluation ObjectDue to the complexity and diversity of alternative financial data, the significant flow rate, the variety of data, and the firm heterogeneity have led to a diverse range of AI technologies for alternative data (Rydberg, 2000). Therefore, we must first clarify the characteristics of alternative financial data applied by AI technology. The main criteria for classification are data source, data type and structure, data application direction, etc.
The reliability, confidence, response time, and other AI algorithms are evaluated according to the application scope and analysis requirements of alternative financial data. For example, alternative financial data is mainly summarized, which can reduce the weight of the algorithm at the corresponding time and increase the weight of confidence. According to the specific needs of alternative data analysis, adjust the standard evaluation weight of the algorithm and add detailed and flexible evaluation indicators.
Establish an Evaluation TeamFinancial alternative data is complex and interdisciplinary. When forming an evaluation team, selecting experts and technicians from multiple fields is necessary. The evaluation team comes from inside and outside the company, corresponding to self-evaluation and other evaluations.
Evaluation experts recommend the following tasks: algorithm architecture design, financial algorithm research, financial extensive data analysis, and other financial and interdisciplinary computer subjects. In addition, evaluators need to have a certain degree of understanding of alternative financial data and AI algorithms and master standard investigation methods and decision-making methods.
Selection of Evaluation IndicatorsWhen selecting evaluation indicators, the following principles should be followed:
- (1)
The value concept surrounds financial alternative data analysis.
- (2)
The first-level evaluation indicators set should remain unchanged, the second-level indicators should not be changed unless necessary, and the third-level indicators may have minor adjustments.
- (3)
The selected evaluation indicators should follow the evaluation principles and be scientific, manipulable, and expandable.
- (4)
The selected evaluation indicators are measurable, and attention should be paid to strengthening communication with relevant departments and personnel of the enterprise to determine the indicators jointly.
Based on the consideration of the internal and external influences of alternative data and the reliability of AI algorithms in the financial industry, combined with actual application scenarios, this standard draws a logical path analysis diagram of quality indicators, creates a structured analysis model of quality indicators, and combines the model to give a set of reliability Evaluation index system. Take AI based on multi-source heterogeneous financial data as a strategic policy, determine quality targets for the strategic approach, and create an indicator pool containing the first-level and second-level indicators embedded in algorithm evaluation. Use the following steps to determine the evaluation system:
- (1)
Determine the source of the alternative data and whether the data supplier has the right to sell the data, and provide a written statement of the supplier's privacy policy. After that, the alternative data is divided into different types and stages, and the alternative information is mainly sensor data.
- (2)
Concerning the evaluation system structure of this article, combined with the composition of financial data, the evaluation model is revised from the top to the bottom from the first level indicators to determine the level of evaluation standards and the subordination relationship between the indicators.
- (3)
The evaluation team members assign weights to each indicator in the evaluation system according to the standard level and data analysis focus. The distribution weight of each index is calculated by Delphi (Chunzhi & Qin, 2011), the analytic hierarchy process (Golden et al., 1989), network analysis, and other decision-making methods.
- (4)
Make specific definitions and explanations for each evaluation index and avoid the differences caused by different people when scoring. In a particular implementation, for quantitative indicators, you can establish a corresponding mapping relationship between the scoring value and the indicator measurement value or set up a corresponding interval; for qualitative indicators, multiple evaluation experts can use the Delphi method to score based on experience but Need to focus on the definition of evaluation indicators and scoring instructions.
The actual application scenario runs a stage that includes alternative data sets and AI algorithms. The reliability evaluation work for the operation phase of AI algorithms refers to analyzing the data used in the actual operating environment and evaluating the algorithm's correctness to determine the algorithm's operation to meet the reliability target requirements.
The input for carrying out reliability assessment work should include at least:
- (1)
Alternative data sets (mainly sensor data) and the reliability evaluation target of AI algorithms.
- (2)
Alternative data sets (mainly sensor data) and reliability evaluation reports of AI algorithm demand, design, and implementation phases.
- (3)
Real data used in the operation of AI algorithms.
The output results are alternative data sets (mainly sensor data) and reliability reports during the operation phase of AI algorithms.
According to the input and output content, the evaluation process is drawn up, as shown in Figure 4.
The following steps need to be taken when implementing each stage of the evaluation process:
- (1)
First, mobilize employees to actively cooperate with the evaluation work and correctly recognize the significance of carrying out evaluations to ensure the accuracy of the evaluation results.
- (2)
The evaluation team investigates the data source and background, understands the company's strategy and industry, has a certain degree of understanding of the content and application scenarios of alternative financial data, and then selects indicators to determine the evaluation system.
- (3)
The evaluation team conducts evaluation work. Specific use methods include field application, personnel interviews, questionnaires, meetings, etc., and scores are scored with the help of decision-making methods.
- (4)
By summarizing the scoring situation and the weight of the reference evaluation system, the total evaluation score of the AI algorithm at this stage is obtained. In this way, whether the AI algorithm can analyze the alternative financial data well can be judged. The advantages and disadvantages of the algorithm can be evaluated according to the score difference of specific scoring items.
According to AI, the algorithm's achieved effect on financial alternative data processing. A rough judgment can be made based on the scoring result. Since the evaluation team needs to set the scoring results and scoring standards, this article gives the characteristics corresponding to the general development stage and does not ask for specific scores.
The evaluation of AI algorithms can be distinguished according to 3 first-level indicators. The evaluation results are divided into eight categories below, as shown in Table 3.
Evaluation results of AI algorithms for alternative financial data.
In this work, the data of Shanghai and Shenzhen 300 stock index futures purchased from alternative data providers are selected to prove the advantages of the constructed financial intelligence standard model in applying to the financial market. The data are divided into a training set and a test set according to the ratio of 8:2. The training data are used to predict the test data to compare the performance of the traditional statistical model and neural network model in forecasting stock index futures data in the financial market. These models evaluate and identify the original data for stationarity judge. Besides, the stationary sequence is obtained through differential transformation to test the models’ results and assess the model's performance.
The financial intelligence standard model constructed here is compared with several representative time series prediction models and a model proposed by scholars in relevant fields recently, including the Autoregressive Integrated Moving Average model (ARIMA) model (Vo & Ślepaczuk, 2022), Back Propagation (BP) Neural Network model (Mohanty et al., 2022), Long Short-Term Memory (LSTM) Neural Network model (Hansun & Young, 2021), and the model algorithm proposed by (Xiaojing, 2021). The performance of the models is assessed through comparative experiments to reflect the actual situation in the financial field.
Interpretation of resultsAnalysis of the prediction accuracyThe financial intelligence standard model constructed here is compared with several representative time series prediction models and a model proposed by scholars in relevant fields recently. The prediction accuracy is analyzed from Accuracy, Precision, Recall, and F1-value perspectives. The results are shown in Figure 5, Figure 6, Figure 7, and Figure 8.
In Figure 5, Figure 6, Figure 7, and Figure 8, the financial intelligence standard model constructed here is compared with several representative time series prediction models and a model proposed by scholars in related fields from the perspectives of Accuracy, Precision, Recall, and F1-value. It can be found that the prediction Precision shows an increasing trend on the whole with the iteration. The algorithms reach a stable state when iterating about 70 times, and the prediction Precision is in descending order: the model reported here > the model proposed by (Xiaojing, 2021) > LSTM Neural Network model > ARIMA model > BP Neural Network model. The comparative analysis of accuracy suggests that the intelligent analysis framework's prediction accuracy is 95.44%, at least 2.54% higher than other neural network algorithms. At the same time, the Precision, Recall, and F1-value of the proposed model are the highest, with a difference of at least 3.19% compared with other algorithms. Thus, the financial intelligence standard model can predict alternative financial data more accurately than other neural network algorithms. The comparison and summary of Accuracy, Precision, Recall, and F1 values under different algorithms are shown in Table 4.
The comparison and summary of Accuracy, Precision, Recall, and F1 values under different algorithms
Accuracy | Precision | Recall | F1 | |
---|---|---|---|---|
The proposed algorithm | 95.44 | 88.57 | 78.28 | 75.22 |
(Xiaojing, 2021) | 92.90 | 85.38 | 73.17 | 70.38 |
LSTM | 90.44 | 83.40 | 71.01 | 66.86 |
ARIMA | 86.54 | 81.03 | 69.00 | 64.82 |
BP | 78.17 | 68.46 | 63.73 | 61.20 |
Furthermore, the relevant transaction data at half-hour intervals in the morning financial stock market opening time (9:30-11:30) are taken as the object to analyze the prediction errors under different algorithms, as shown in Figure 9.
As shown in Figure 9, it can be found that the performance of the models at different time points is different by analyzing the financial market transaction data in the period of 9:30-11:30. This phenomenon is caused by the various user transaction volume data densities at different time points. Therefore, the value of regression evaluation indicators decreases with the decline of the cumulative prediction error of models. On the contrary, with the growth of user transaction volume, the number of samples predicted by the model increases, the incremental error increases, and the value of such indicators also increases. Further comparison of the prediction errors of each algorithm shows that the different error values of the model proposed here are smaller than those offered by scholars in other related fields, which are generally stable at around 3. This result proves that the model's full use of alternative financial data improves the prediction accuracy and validates the effectiveness of the financial intelligence standard model in the forecasting process.
ConclusionSummaryIn the vision of Industry 5.0 and Society 5.0, increasing industries will further integrate AI technology into the process of industrial development. This article starts with literature analysis and formal analysis and provides a systematic, standardized, and standardized solution for formulating financial intelligence technology evaluation standards in social platforms. Furthermore, an example analysis was carried out on the framework proposed here. The results showed that the prediction accuracy of the framework reached 95.44%, and the error was significantly smaller, which could provide an experimental reference for the intellectual development of the financial field. There are the following specifications for each participant in the development process of financial intelligence technology:
- (1)
For program developers: There are clear regulations and standards for any development process. Developers take it as a reference, which will help improve work efficiency and shorten the development cycle; simultaneously, having a unified development standard can reduce the management staff's involvement in the development process—participation in improving the independent development ability of developers.
- (2)
For the financial investment system: raising the evaluation threshold of the development process will help ensure the standardization of the development process and the rigor and reliability of the output results, improve product quality, ensure system integrity and accuracy, and reduce subsequent software maintenance cost.
- (3)
For financial investors: On the one hand, the financial knowledge and laws contained in financial data can be refined more accurately to provide a solid theoretical basis for economic decision-making; on the other hand, the needs and use of data by investors are very different After the standards are unified, the financial investment system can be flexibly adjusted according to the actual needs of users to improve the applicability of the system. While reducing monetary costs, it can accurately and timely match investment needs and minimize investment risks.
- (4)
For the entire financial market: it is conducive to improving its stability and standardization and enhancing the risk prevention capabilities of the financial investment market.
Make the alternative data collected by IoT sensors accurate and effective. Make the core algorithm flexible and adaptable. Make decision-making results rigorous and reliable. The AI system can truly serve financial investment, achieving accurate data, high accuracy, and low risk. As a reference standard for related practitioners, it promotes the development and construction of the financial sector and drives the technological transformation and quality upgrade of associated industries.
Deep Thinking and ExplorationThe Authenticity and Reliability of DataAlgorithm design, feature engineering, and model parameters are essential steps that affect the accuracy of AI technology predictions. In addition to these, the authenticity and reliability of the original data will also affect the prediction results of the entire system architecture of the source. This is reflected in the outstanding performance of much AI research on public data sets, but the generalization ability in actual application scenarios is not exceptional. This is different from over-fitting. The main reason is the non-independent and identically distributed (Non-IID) problem of the input data. For two data sets with significant differences, their contradiction is reflected in the different model parameters and is more likely to be suitable models are also different. In addition, even if the most appropriate AI model is selected, the quality of the training data set is very high, and the performance of the validation set is also excellent. The expected results cannot be obtained after being attached to the production process. This is because the actual production data involves the core business secrets of the company, and these companies do not want to disclose the essential data to other institutions responsible for AI research and development. Hence, they desensitize the data before transmitting it, even adding noise. This is especially common in the field of intelligent finance.
Therefore, in addition to the development process of AI standards mentioned in this article, it is necessary to further study the standardization methods in the stages of data collection and data transmission and use privacy protection technologies, including blockchain, federated learning, and other technologies to unify data. The acquisition process guarantees the authenticity and reliability of AI data from the source. Of course, the financial intelligence reported here principally adopts the deep learning method to design the standard framework. In addition to the technique used here, some representative computational intelligence algorithms can also be used to solve these problems, such as monarch butterfly optimization (Wang et al., 2019), earthworm optimization algorithm (Wang et al., 2018), elephant herding optimization (Li, 2020), moth search algorithm (Feng, 2018), Slime mould algorithm (Li, 2020), hunger games search (Yang, 2021), Runge Kutta optimizer (Ahmadianfar, 2021), colony predation algorithm (Tu, 2022), and Harris hawks optimization (Heidari, 2019).
Regulation of AIThe AI system cannot analyze and process through the built-in algorithm of the system under the condition that the main body of power and responsibility cannot be defined, so the supervision process is faced with complex problems. Traditional and behavioral supervision principles can no longer be applied to the rapid development of AI finance, and there is a certain lag. In this case, specific regulatory standards are required to restrain financial companies from reducing their chances of taking high-risk speculation.
These constraints reduce the efficiency of the use of funds by financial institutions. At the same time, the operational efficiency of financial institutions when using AI has increased operating costs. Therefore, the future standard formulation will balance the two and formulate more detailed and in-depth regulatory standards.
Awareness of Financial Investment RisksFor financial investment, there must be risks when there are gains, and investors often do not have that in-depth understanding of risks. Investors are more interested in seeing these differences when they cannot choose by comparing the first-level risks that may arise. Level-level risks may lead to second-level or even multi-level risks. The current financial investment system lacks an assessment of the multi-level risks that potential risks may trigger and cannot provide investors with an in-depth and detailed investment analysis.
In the future, multi-level risk assessment standards will be formulated for the multi-level risk assessment system. To improve the practicability and accuracy of the evaluation results and their guiding significance for investors, there will be a unified standard for the in-depth evaluation of potential risks and the precision of the assessment.
Conflict of InterestThe authors declare no competing interests.
This work is supported by the Open Project (SKLMCPTS202103011) of State Key Laboratory of Media Convergence Production Technology and Systems, Xinhua News Agency, 100803 Beijing, China.