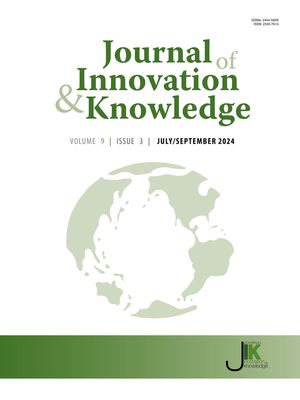
Editado por: Brij B. Gupta, Kwok Tai Chui
Más datosThe influence of digital industry and firm digitization on enterprise innovation has emerged as a critical research topic. To assess the impact of digital transformation on enhancing innovation output, we propose a game model of two organisations investing in digital transformation, analyze the index of enterprise digitalization level with Python tools for text analysis, and employ a fixed effect model. The findings indicate that firm digitalization and the level of regional digital industry innovation can both promote firm innovation. However, the regional digital industry innovation level can have a negative moderating effect on the firm digitalization innovation effect. Furthermore, the impact of firm digitalization on innovation is more visible in digital-related service industries. In other industries, the regional digital industry innovation level has a greater impact on innovation promotion. Due to firms' free-riding tendency in technology adoption, this study shows that the higher the level of digital industrialization in the region where the firm is located, the lower the marginal innovation efficiency of the firm's digital investment. When the level of development of digital industrialization in the region where a firm is located is higher, the "competitive effect" improves the marginal innovation efficiency of firms in adjacent areas, implying that digital industrialization has a spatial spillover effect. The relevant robustness test further verifies the conclusion of the empirical analysis. As a result, the digital industry should be given more attention and financial support.
The digital economy, as the "new engine" for the world's economic evolution, has created new sectors and empowered traditional industries. It is also an essential way for countries to improve the quality of their economic development. The United States, Germany, France, Canada, India, and other countries have successively made digital transformation the focal point of their national economic development strategies. Digital transformation has become a requirement for survival and long-term development in all walks of life. Digital transformation refers to the process of reconstructing business models using a combination of emerging digital technologies such as artificial intelligence, cloud computing, blockchain, and big data. The use of digital transformation to improve the production and innovation efficiency of enterprises has already achieved initial results in some enterprises.
Enterprises are embracing digital transformation at an exponential rate in an effort to spur development through the adoption of new business models and digital technology. For enhancing the customer experience, Kumbhojkar and Menon (2022) suggested using the Integrated Predictive Experience Management Framework (IPEMF). Through the customer journey, IPEMF assists businesses in creating intuitive, trustworthy connections and hyper-personalized client experiences. Utilizing semantic web technologies is more effective at resolving issues with metadata storage and data integration in digital archives, which improves search accuracy and results relevancy (Pandolfo & Pulina, 2021). Pashchenko (2020) believed that digitalization in the software sector provides a continuous flow of varied data, while also presenting new chances to make process organizations more competitive. Sarkar and Kundu (2020) proposed cloud-based high-performance storage techniques. Jamil and Yukongdi (2020) investigated the function of emotional trust as a moderator of the link between creative work behavior and participative management, as well as the moderating impact of supervisor-subordinate guanxi. Then, discovered that information systems help businesses stay competitive. Ramaru, Garg and Chakraborty (2022) attempted to address the merging of existing systems with the goal of optimizing operational productivity based on various hardware and software.
The significant expansion of the digital industry and the ongoing process of firm digitalization are two indicators of the digital transformation's success. The development of the digital economy has become a strategic choice in order to capitalize on the new opportunities presented by the new round of technological revolution and industrial transformation, and numerous studies have confirmed the impact of enterprise digital transformation on innovation. (Chen, Kumara & Sivakumar, 2021; Gaglio, Kraemer-Mbula & Lorenz, 2022; Peng & Tao, 2022; Sedera, Tan & Xu, 2022; Wen, Zhong & Lee, 2022). Digital industry provides digital technologies, products, services, infrastructure and solutions for the development of firm digitalization. This means that the level of regional digital industry innovation is important in the innovation effect of firm digitalization. So, what are the specific roles of firm digitalization and the level of regional digital industry innovation in promoting firm innovation? These are significant research hypotheses.
Most existing research focuses on the impact of enterprises’ digitalization on themselves, but in fact, the development and innovation level of the regional digital industry may help enterprises to realize more innovations, and it is very easy to make positive spillovers and form “free-riding” effect. The majority of current research on the impact of the digital industry on businesses is still qualitative. So, we construct a theoretical model based on existing research and apply empirical studies on the effects of firm digitalization and regional digital industry innovation level on the firm innovation. The marginal contribution of the paper has two main points: First, in contrast to previous literature, which only focuses on the degree of digital transformation of the enterprise itself, this paper divides digital transformation into two perspectives: enterprise digitization and regional digital industry innovation level. Second, a theoretical game model is developed to explain why businesses choose to free-ride in regions with developed digital industries. It enriched the relevant theoretical background.
The rest of the paper is organised as follows: The literature review in Section 2 introduces influential factors of firm innovation and the technological innovation model. The game model and research hypothesis are presented in Section 3. Section 4 conducts an empirical analysis to determine whether digital transformation has an impact on firm innovation, which includes the construction of the measurement model and the explanation of variables and data. Section 5 analyses the spatial spillover effects of regional digital industry innovation levels. Section 6 concludes the findings.
Literature reviewTechnological innovationDepending on the source of technological innovation, there are two types of technological innovation: independent innovation and cooperative innovation (Felin & Zenger, 2014; Li, Zhou & Huang, 2021). The former refers to firms that develop new technologies or new products based on their own technical resources and knowledge reserves, while being able to provide follow-up support for technologies, products, and services. The latter means that firms obtain corresponding technological innovation results through cooperative research and development, division of labor and collaboration with other firms, universities or scientific research institutes and other technological partners. Firms that innovate independently will inevitably face risks such as R&D failure and excessive costs and so on. Risks associated with cooperative innovation include technology leakage, technology plagiarism, and technology imitation. As a result, the choice of a firm's technological innovation model is influenced by a variety of factors, including the firm's location in the innovation environment and its internal and external resources. (Ma, Zheng, Chen & Yin, 2021; Nason, Wiklund, McKelvie, Hitt & Yu, 2019).
Technological start-ups are a complex combination of new technologies and knowledge. In the era of the digital economy, they are an important carrier for disruptive technological innovation (Sheng et al., 2022a, 2022b). When choosing different technological innovation models, technology-based entrepreneurial firms will comprehensively weigh the costs, benefits, and risks of the innovation model (Kang, Baek & Lee, 2017; Si, Li & Huang, 2021). The company mainly consider the following factors: (1) Technological innovation resources and capabilities. When technology-based startup firms have abundant reserves of resources, strong innovation capabilities, and low dependence on external resources, they are more likely to accumulate knowledge and strengthen competitive advantages through independent technical innovation, to avoid “technical catch-up traps”. (2) Innovation costs and benefits. Independent innovation by technology-based entrepreneurial firms is typically more expensive than cooperative innovation. However, long technology development cycles, unclear innovation risks, and lagged innovation returns are all considerations in independent innovation. (3) Innovation risk. On the one hand, some technology-based start-ups will evaluate the risks of independent and cooperative invention and select the technological innovation model with the highest returns and the lowest risks based on their specific situations. The level of risk associated with cooperative innovation, on the other hand, is closely related to technological differences. (Ritala, Olander, Michailova & Husted, 2015; Wang, Zhang & An, 2018; Yan et al., 2021; Zheng & Yin, 2022; Zheng et al., 2021).
The influential factors of firm innovationThe factors affecting firm innovation include government subsidies, R&D investment, and equity structure. The debate over the positive and “trap” effects of government subsidies has been going on for a long time. Government subsidies help make up for market failures in the innovation process, drive innovation investment at the firm level, and promote firm technological innovation activities (Lin & Luan, 2020; Liu, Zhao & Wang, 2020). Scholars who hold the view of “negative effects” of government subsidies believed that government selective subsidies will have a crowding out effect on firm innovation (Wu et al., 2022). Firm's R&D investment has a positive impact on innovation, which is the research conclusion of many scholars (Alam, Uddin, Yazdifar, Shafique & Lartey, 2020; Xu, Wang & Liu, 2021). Ownership structure affects firm innovation, and the preference of actual controlling shareholders influence the company's choice of innovation strategy. The stability of major shareholders provides major shareholders with sufficient motivation and power to supervise the behavior of corporate managers, obtain more benefits from technological innovation, take the initiative to assume innovation risks, increase innovation investment, and carry out technological accumulation.
The effect of digital transformation on firm innovationThe early research on the impact of the digital economy on firm innovation mainly revolved around the Internet. The widespread use of the Internet can quickly promote the dissemination of knowledge and information while also lowering firm costs in the process of information search, copying, transportation, tracking and verification, which is conducive to promoting firm innovation (Goldfarb & Tucker, 2019; Fang, Razzaq, Mohsin & Irfan, 2022). Digital communication technologies, such as social media and business mobile Internet access, have a positive impact on innovation, and innovation that is conditional on the use of these technologies has a positive impact on labor productivity. (Gaglio et al., 2022). Some scholars have conducted empirical tests on the internal mechanism of firm innovation from different perspectives. Firm with stronger absorptive capacity and higher efficiency can often obtain more innovative benefits from the use of network (Müller, Buliga & Voigt, 2021). According to previous study, it showed that the implementation of digital transformation plays a significant role in promoting economic benefits (Zhang, Shi, Shi & Chen, 2022). Internet e-commerce can significantly promote corporate R&D investment (Gherghina, Botezatu & Simionescu, 2021). That means there is a significant and positive relationship between digital platforms capability and innovation performance (Jun et al., 2021). Digital transformation of enterprises includes the overall transformation of enterprise strategy, marketing, commodities, business model, management and corporate culture. So, digital transformation can reduce the communication cost of firm, improve the connectivity of innovation networks, accelerate the speed of digital integration, expand the scope of integration, increase the knowledge heterogeneity and integration requirements of innovation networks, and reshape the creation and sharing of knowledge in innovation networks. Digital capabilities positively influence firm performance only through technological capabilities. Furthermore, enterprises in the mature stage of their product life cycle benefit the most from digital transformation. (Zhai, Yang & Chan, 2022). Numerous studies indicate that the more companies use digital technology, the more potentially innovative they are. However, some authors insist that digital technology has very little impact on innovation performance, and that R&D expenses are the most reliable predictor of innovation. (Usai et al., 2021)
To sum up, the existing literature on the impact of enterprise innovation elements and innovation mode selection of research is quite broad, and studies on the impact of digital transformation on enterprise innovation, are mostly based on the macro or digital transformation of enterprise level, but in fact, local digital industry innovation ability to impact on the rest of the enterprise innovation, is likely to be more important than the enterprise's own digital capability. However, there aren't many studies on this topic, and the mechanism analysis of the impact of digital transformation on enterprise innovation is relatively lacking. To examine the effects of both on enterprise innovation, we divided digital transformation into two categories regional digital industry innovation level and enterprise digitalization. We also explore the spatial spillover effects of the level of innovation in regional digital industries.
Theoretical analysis and research hypothesisTheoretical modelAssumptionThe digital transformation includes digital industrialization and firm digitalization. Digital industrialization provides the foundation for firm digitalization. At the same time, the development of the digital economy blurs the boundaries of the industry. The digital industry has become an important subject of innovation, and the firm digitalization is the extended application of the innovation. The two are closely related to the choice of innovation and application.
We label digital industrialization and firm digitalization as firm group 1 and firm group 2. To facilitate analysis, firm A is the representative company in group 1, and firm B is the representative company in group 1. A and B are the main players participating in the game.
There is an innovation project, the investment cost of enterprise A and enterprise B is T, and the total income generated by this project is M, M>T. Company A is better at investing in R&D and innovation, while company B is better at scaling up the use of innovative products. The ratio of company A's revenue to total revenue is λ, and the ratio of company B's revenue is 1-λ. In fact, we assume that innovation investment has a positive impact on the level of enterprise innovation.
When enterprise A undertakes the R&D and application of innovation project independently, the investment is αT (α>2) and the income is M due to the professional barrier. The capital required by enterprise B to independently undertake innovation project R&D and application is αT (α>2), and the income is M.
Game evolutionWhen both firm A and firm B choose to invest in terms of their comparative advantages, they share the cost of innovation input and application. At this time, firm A's income is λ(M-T), and firm B's income is (1-λ)(M-T). When firm A chooses to invest independently and exclusively, the income of firm A is M-αT and the income of firm B is 0. When only firm B chooses to invest, the income of firm A is 0 and the income of firm B is M-αT. When both firm A and firm B choose not to invest, their returns are both 0. Table 1 shows the profit matrix of the strategic choices of the two types of firms.
Where x is the probability that firm A invests and y is the probability that firm B invests. Therefore, the income of firm A's choice of investment, the income of non-investment, and the expected income are:
The income of firm B's choice of investment, the income of non-investment, and the expected income are:
According to the replication dynamic equation of the evolutionary game, the replication dynamic equations of firm A and firm B are:
Set F(x)=F(y)=0 to obtain five equilibrium points of the evolutionary game, respectively: A(0,0),B(1,0),C(1,1),D(0,1),O(αT−M(1−λ)(M−T)+(αT−M),αT−Mλ(M−T)+(αT−M)).
Using the research method of Friedman (1998), we analyze the stability of the system composed of the copy equations of the two parties in the game. In the two cases of αT-M>0 and αT-M<0, we analyze the evolutionary and stable results of the two types of firm selection modes. Fig. 1 is the evolution phase diagram under the condition of αT-M>0. When the initial state falls in zone I and converges to point A(0,0), both parties in the game choose not to invest. When the initial state falls in area II and converges to point C(1,1), both players in the game choose to invest. Fig. 2 is the evolution phase diagram under the condition of αT-M<0. No matter how the investment strategies of the two parties in the game choose, they will eventually converge to the point C(1,1), so the decision-making steady state is joint investment. It is conceivable that when digital transformation in one place leads to improved innovation performance, surrounding areas will follow suit and compete. Through “competition effect”, digital transformation within a region can improve the innovation performance of surrounding regions.
Under the condition of αT-M>0, the final evolutionary stable strategy of both parties in the game is to choose to invest or not to invest, depending on the size of SⅠ(ABOD) and SⅡ(BCDO) in Fig. 1. If the area of SⅠ(ABOD) is larger, the two parties are more likely to choose not to invest. If the area of SⅡ(BCDO) is larger, the two parties are more likely to choose investment.
Given the coordinates of saddle point O, the area of SⅡ(BCDO) can be known from Fig. 1:
According to Eq. (9), ∂SI/∂M>0, that is, as M increases, the probability that both parties choose to invest increases.∂SI/∂T<0, that is, as T increases, the probability that both parties choosing to invest decreases. When 0<λ<1/2, ∂SI/∂λ>0, that is, with the increase of λ, the probability that both sides choose to invest increases. When 1/2<λ<1, ∂SI/∂λ<0, that is, with the increase of λ, the probability that both sides will choose to invest decreases. It means that λ=1/2 is an equilibrium state. It can be imagined that when enterprises in the region choose to free ride, that is, the equilibrium state λ=1/2 is broken, which is bound to be not conducive to the improvement of innovation level.
Research hypothesisImpact of firm digitalization on innovationFirm digitalization affects the firms’ innovation through four channels. (1) Cost-saving channel. Firm digitalization accelerates the flow of information, knowledge, manpower, and other elements. Firm with digitalization can obtain massive, high-quality and fully disclosed resources smoothly, instead of spending efforts on information search, copying, transmission, tracking and verification. It also helps to expand the innovation space from the internal organization to an open space without institutional barriers. (2) Advanced technology application. Digitalization empowers firm to require massive data and calculation capabilities. It provides unprecedented efficient tools to achieve promising R&D outcomes and innovative products, which improves the decision-making efficiency and success rate of innovation. (3) Resource allocation efficiency. Firm with digitalization overcomes information asymmetry and allows the firm to organize fragmented resources. Then the firm is able to allocate the key resources on the core technology and innovation activities. (4) Diversified innovation ways. Firm digitalization trigger more varied innovation modes such as mass innovation, mass intelligence, micro-innovation and iterative innovation, which inject new impetus into firm innovation. Therefore, we propose Hypothesis 1.
H1. Firm digitalization can promote firm innovation.
The digital industry serves as the technological foundation and driving force behind firm digitalization. That is, digital industrialization supports local firms with basic digital equipment and services, resulting in increased investment in digitalization and innovation expansion. However, as an individual firm, it is more likely to rely on the existing environment created by pioneers' technologies and engage in "free-riding" behaviours rather than committing to its own innovation, making innovation a high-risk and high-cost process where R&D failure and excessive expenditure are unavoidable. The stronger the digital industrialization innovation environment, the more likely the firm will have “free-riding” behavior. This kind of “free-riding” makes it impossible or difficult for innovators to recover the high cost of investment in the innovation process and weakens the role of firm digitalization in promoting firm innovation. Therefore, we propose Hypothesis 2.
H2. Regional digital industry innovation level promotes firm innovation, but it plays a negative moderating effect in the innovation effect of firm digitalization.
The digital transformation has a spatial impact on firms’ innovation. Through empowerment, resource allocation and innovation agglomeration, the spatial overflow of regional innovation activities of firm has been formed. For example, the Internet platform firm is an innovation ecosystem with typical bilateral market characteristics. To improve network externality, platform firm need to acquire a large number of developers, users and providers of digital products. In order to lower the threshold of innovation activities, they will encourage developers and suppliers in different regions to input into innovation by components and modular methods. This “platform + empowers + developers” innovation model improves the efficiency of innovation activities, enhances the inclusiveness of the process, and establishes a space overflow for digital innovation. In terms of resource allocation, innovative entities integrate factors of production across regions, overcoming resource mismatch and lowering transaction costs, all of which have a positive impact on innovation activities across multiple regions. From the standpoint of innovation agglomeration, it will "siphon" the surrounding areas' innovation resources to strengthen a concentration of innovation elements such as talents, technology, and capital, which will be beneficial for innovation activities. Therefore, we propose Hypothesis 3.
H3. Regional digital industry innovation level has a positive impact on firm innovation in surrounding areas through a spatial spillover effect.
Based on theoretical analysis and previous studies, firm digitalization and the innovation level of digital industrialization in the area both have the positive effects on the firms’ innovation. In addition, some variables such as government subsidies and R&D investment would affect the innovation performance of enterprises. So, we constructed the following econometric model.
Where i and t represent firm and year respectively. einle is the level of firm innovation. endig represents the level of firm digitalization. digen is the innovation level of digital industry in the area where the firm is located. wdigen is the spatial weight matrix of regional digital industry innovation level.
Control variables includes firm-level and regional-level variables. The variables at the firm level mainly include government subsidies, R&D investment, total assets and the shareholding ratio of the largest shareholder. Regional-level variables include regional GDP, per GDP, total population at the end of the year, the proportion of the secondary industry in GDP, and the proportion of the provincial digital industry population. year represents the fixed time effect of the firm. ownership is the nature of equity. Since the time span of some corporate samples in the regression is small, controlling the time fixed effect and the individual effect, the influence of the variable with a small change over time will be covered by the individual effect, so it needs to add the dummy variable ownership. If it is a state-owned firm, set it to 1, otherwise it is 0. ε is a random disturbance term.
Variables- (1)
Dependent variable. The dependent variable is the level of corporate innovation (einle). Due to invention patents having a higher technical content than other types of patents, We utilize invention patent applications to assess the extent of firm innovation, and utilize the total number of patent applications of firms (the sum of invention patents, utility models, and design patents) as a substitution variable in the robustness analysis. To avoid obtaining a number less than zero after doing the logarithm, the logarithm is performed after adding one to the original data.
- (2)
Independent variables. The key independent variable is the level of firm digitalization (endig). Former studies have measured the digital economy based on the macro level or the Internet development level as an alternative indicator but failed to subdivide the digital economy. We adopt the keyword frequency analysis method and use the frequency of firm digitalization keywords in the company's annual report as a proxy indicator of the company's digital investment level. The text information of the company's annual report comes from the PDF file of the company's annual report on www.cninfo.com.cn. Limited to data availability and integrity, we used crawler technology to obtain all the PDF files of all listed companies’ annual reports from 2011 to 2017, and the corresponding software is used to recognize them as plain text data. In order to define the firm's digitization, we selected 90 linked keywords. To determine the percentage of the total length of the annual report text that represents the firm's rate of digitalization, or firm digitalization level, the frequency of occurrence is finally added. The header and page number information from the yearly report file are deleted while still recognizing the content in order to maintain data accuracy.
- (3)
Moderating variable. Our moderating variable is regional digital industry innovation level (digen) in the area where the firm is located. We used the total number of valid invention patent applications of all listed companies related to the digital economy in the province as a measure of the level of inter-provincial digital industry innovation level. According to the industries corresponding to the products that meet the characteristics of digital infrastructure in the “Product Classification Catalog for Statistics”, digital industries include telecommunications and other information transmission services, computer information services, software services, communication equipment, computers and other electronic equipment.
Fig. 3 shows the peaks of the innovation level of China's provincial digital industries, depicting the annual trend. From the change of peak position from 2011 to 2017, the overall innovation level of provincial digital industry has improved year by year, and the dispersion degree has been reduced. That indicates that the gap between the digital industry in each region is narrowing when the innovation ability of digital industry is improved.
- (4)
Other variables. Variables at the corporate and regional levels are also included. Firm-level variables include government subsidies, R&D investment, total assets, and the shareholding ratio of the largest shareholder. Government subsidies include bonuses, tax incentives, industrial support, technological transformation, scientific research funding, and talent introduction. R&D investment refers to the financial investment of a scientific research project in the research and development stages, including equipment costs, material costs, testing and processing costs, fuel and power costs, travel costs, international cooperation and exchange costs, intellectual property affairs costs, labor costs, and experts’ consultation fees, management fees and other expenses.
Because the statistical standards for patent data after 2017 is different, and the data lacks firm digital indicators before 2011, we selected China's A-share listed companies from 2011 to 2017 as the research sample, and the data comes from the China Stock Market Accounting Research (CSMAR). After excluding some samples with severe data missing, we obtain 8709 valid sample observations (Variable definitions and Descriptive statistics is in APPENDIX A). All variables except ratios have been processed with logarithms.
Empirical resultsBaseline resultsThe baseline results are shown in Table 3. Model (1) shows that the estimated coefficient of the company's digital level is 2.490, which is significantly positive, and shows that firm digitalization has a significant role in promoting the level of company innovation. Model (2) demonstrates that the estimated coefficient of digital industry innovation level is 0.148, which is significantly positive, indicating that digital industry innovation level has a significant role in promoting firms’ innovation. Model (3) incorporates the impact of firm digitalization and digital industry innovation level on firm innovation. The estimated coefficients of company's digital level and digital industry innovation level are 2.464 and 0.145, respectively. The coefficients are both significantly positive, confirming Hypothesis 1.
Model (4) includes the impact of the firm's digitalization level and digital industry innovation level interactive items on the firm's innovation level. The result shows that the coefficient of the interaction term is −0.623 and significantly negative. The influence coefficients of the level of corporate digitalization and digital industry innovation level are 7.541 and 0.160, respectively, which are both significantly positive. This proves that the marginal impact of firm digitalization on innovation level will decrease as digital industry innovation level increases, that is, the stronger the innovation environment of the province in which the firm is located, the lower the marginal innovation efficiency of its digital investment. In other words, a good digital industry innovation environment provides opportunities for other local enterprises to “free ride”, and enterprises are more willing to borrow the technology and innovation of digital enterprises, which reduces the efficiency of their own digitalization, thus leading to the decreasing marginal effect of enterprise digital transformation. The result verifies the Hypothesis 2.
Robustness testsWe replace the number of invention patent applications with the number of patent applications. The results of the robustness analysis are shown in Table 3. Model (5) shows that the estimated coefficient of the company's digital level is 1.210, which is significantly positive. Model (6) demonstrates that the estimated coefficient of digital industry innovation level is 0.166, which is significantly positive. Model (7) shows that the estimated coefficients of company's digital level and digital industry innovation level are 1.180 and 0.164, respectively. Model (8) shows that the coefficient of the interaction term of firm's digitalization level and digital industry innovation level is −1.294, and significantly negative. The findings show that the level of firm digitalization and digital industry innovation level continue to have a significant positive effect on the number of firm patent applications, and the interaction between the two is also significantly negative, and digital industry innovation level has a significant negative adjustment impact on the digital level of firms’ innovation effect. The finding of the robustness regression is mostly consistent with the conclusion of Table 2.
Baseline regression results.
(1)***p<0.01; **p<0.05; *p<0.1. (2) Standard errors are in parentheses.
Results of robustness tests.
(1)***p<0.01; **p<0.05; *p<0.1. (2) Standard errors are in parentheses.
To investigate the impact of firm digitalization and regional digital industry innovation level on firm innovation level, we use several quantile regressions of firm innovation level. The quantile regression results are shown in Table 4. The results show that at the lowest quintile of 0.05, that is, in the sample of companies with the least amount of patent applications, the estimated coefficients of company's digital level and digital industry innovation level are −0.127 and −0.040, respectively. It shows that the level of corporate digitalization and digital industry innovation level does not significantly promote the level of firm innovation. Starting from 0.20 quantile, the promotion of enterprise digitization and digital industry innovation level to enterprise innovation is significantly positive, which is consistent with the conclusion of baseline regression. The coefficient increases as the quantile value increases, indicating that the greater the enterprise innovation level, the greater the influence of enterprise digitalization and digital industry innovation level on firms’ innovation. This implies that companies with strong innovation capabilities should invest in digital transformation and locate in more developed digital industries.
Results of quantile regression.
(1)***p<0.01; **p<0.05; *p<0.1. (2) Standard errors are in parentheses.
The impact of firm digitalization and digital industry innovation level on firm innovation may differ due to industry heterogeneity among firms. According to the China Securities Regulatory Commission's secondary industry classification standard (2012), this article divides the samples into five categories: Internet-related companies (model 16); Equipment manufacturing (model 17); Digital-related manufacturing (model 18); Digital-related services Industry (model 19); Other industries (model 20). Table 5 shows the results of the regression by industry. Model (17), model (18) and model (19) show that the estimated coefficients of company's digital level are 3.195, 3.109 and 2.659, respectively. The result shows that the digital level of firms plays an important role in stimulating innovation in the equipment manufacturing industry, digitalization-related manufacturing, and digitalization-related service industries. Model (17), model (19) and model (20) show that the estimated coefficients of digital industry innovation level are 0.212, 0.492 and 0.068, respectively. The findings indicate that the regional digital industry innovation level has a significant impact on stimulating innovation in the equipment manufacturing industry, digital-related service industries, and other industries.
Results of regression by industry.
(1)***p<0.01; **p<0.05; *p<0.1. (2) Standard errors are in parentheses.
According to the above empirical findings, the stronger the innovation level of provincial enterprises, the lower the marginal innovation level of firm digitalization. It can be found that digital industry innovation level in the region where an enterprise is located may have a substitution relationship with the digitalization level of the enterprise. Therefore, this paper constructs the following econometric model:
Table 6 shows the results of mechanism analysis. The estimated coefficient of company's digital level is 0.003. The digital industry innovation level in the region where the company is located has a significant positive impact on the level of digitalization of the company. The enhancement of regional industry digitalization, on the one hand, promotes the degree of enterprise digitalization. The motivation for businesses to realize innovation through their own digital level will be reduced as a result of the "free-riding" phenomena, which allows businesses to immediately benefit from the patented technologies of other local digital industries by purchasing services or products. Therefore, it shows that the interactive items of enterprise digitalization and digital industry innovation level have a significant negative impact on enterprise innovation level. It is further speculated that there are not many opportunities for enterprises in regions with poor digital industry innovation level to “free-riding”. In order to improve their operational capabilities and innovation capabilities, they use limited digital investments to carry out R&D and innovation with maximum efficiency by themselves.
Spatial spillover effectsSpatial spillover effect analysisThe level of digital industry innovation level in a region not only affects the innovation level of firms in the region, but also has a spillover effect that affects the innovation level of firms in other regions. If the spatial spillover effect is ignored, the outcome may be biased. Therefore, we create a geographical adjacency matrix, using the SLX model (Spatial Lag of X model) in spatial measurement to lag the provincial digital industry innovation level, and create a new variable (wdigen) to test the existence of spatial spillover effects. Table 7 shows that wdigen’s coefficients are 0.219, 0.418, 0.306 and 0.264, respectively. They are all significantly positive, which means that digital industry innovation level has a significant promotion effect on the innovation level of firms in surrounding areas, that is, the spillover effect exists. endig × wdigen is significantly positive, and coefficients of endig × wdigen are 5.198 and 6.398, respectively. The results indicate that the surrounding area has a strong digital innovation atmosphere, which not only gives firms in the surrounding area “free-riding” opportunities, but also brings a “competitive effect”, which forces the local enterprise to strengthen the degree of reliance on their own digital construction and promotes the marginal innovation level of firm digitalization.
Spatial spillover effects of regional digital industry innovation level.
(1)***p<0.01; **p<0.05; *p<0.1. (2) Standard errors are in parentheses.
Table 7 shows that the digitalization level of firms in the region and the digital industry innovation level of surrounding areas have formed a “synergistic effect”. In order to further verify the possible mechanism of synergy, we constructed the following measurement model.
Table 8 shows that coefficient of wdigen is −0.023, and significantly negative, which indicates that the digital industry innovation level of the surrounding region can promote the innovation level of local enterprises, but also inhibit the digitalization level of enterprises in the region. The digital industry innovation level in the surrounding area will have a “siphon effect” on the regional digital industry, that is, attract a large number of service providers and scientific and technological talents, which reduces the regional digital industry innovation level and the chance of “free-riding” for local enterprises.
ConclusionsThe regional digital industry innovation level data, as well as the digital and patent data of the listed firms, are applied to investigate the effects of digital transformation on firm innovation. The spillover effects of digital industry innovation level are also further analyzed. According to the findings of the study, corporate digitization and digital industry innovation level have a beneficial impact on firm innovation. Furthermore, digital industry innovation level has a negative moderating influence on the digital level of enterprises’ innovation. The greater the innovation environment of the region in which the firm is located, the lower the marginal innovation efficiency of the firm's digital level. The amount of firm digitalization and digital industry innovation level will have a bigger influence on the level of firm innovation for firms with stronger innovative capabilities. Furthermore, the level of innovation in the digital industry has a spillover effect on company innovation in adjacent areas. When the region's digital industry innovation level is high, companies in the region prefer to “free-ride”, but the innovation efforts of firms in the surrounding region are enhanced, resulting in an increase in the marginal innovation effect of digital level of the enterprises in the surrounding region. Digital industry innovation level has a spillover impact on corporate innovation in adjacent regions.
Recommendations and implicationsBased on the research conclusions, it is suggested that the digital industry should be given more attention and financial support. To improve the effects of digital transformation on firms’ innovation. Several pieces of advice is given as following: To begin, regional governments should vigorously develop the digital industry, leading to the provision of technical support for the digital transformation of a firm. Secondly, to ensure that the digital industry's innovation has a positive impact on regional enterprises. If enterprises' "free-riding" behavior is to be sustainable, the state must actively research and develop relevant laws and regulations to effectively protect intellectual property rights. Finally, digital enterprises can collaborate with other enterprises to establish models of digital innovation partnership to solve problems of digital technology. This study explains the ways, mechanisms and motives for enterprises to get help for innovation from regional digital industries through “free-riding” behavior in the process of digitalization. This work also contributes to the strategic direction of digital transformation of enterprises.
Limitations and future research directionsThis study has certain limitations that should be addressed in future research. There are still some shortcomings in this paper. Firstly, the sample time span is insufficient due to data availability. If more comprehensive data becomes available in the near future, we will be able to study the time trend of the impact of digital transformation on innovation. Secondly, digital industry innovation level includes a variety of sub-industries. Thus, it would be meaningful for future studies to investigate how the impact of the specific digital industry promotes the firms’ innovations. Thirdly, in this paper, the innovation level of the digital industry is measured by the number of patent applications in the digital industry. However, the relevant services provided by the digital industry for other industries can already promote innovation. In the future, other indicators could be used to measure the impact of the digital industry more accurately on the innovation of local enterprises. Finally, rather than the direct effects on firm innovation, future research could focus on the effects of digital transformation on the elements of innovation. (Eqn (1)-8, 10-14, Table A1-A2).
Variable definitions.
Descriptive statistics.
This project was funded by Deanship of Scientific Research (DSR) at King Abdulaziz University, Jeddah under grant No. (KEP-PhD-5-611-38)], the authors, therefore, acknowledge with thanks DSR technical and financial support.