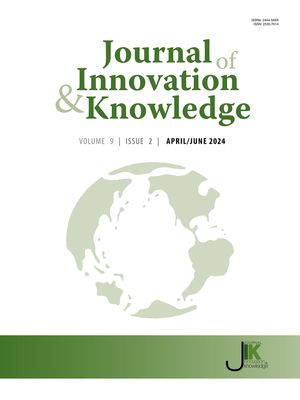
Editado por: Brij B. Gupta, Kwok Tai Chui
Más datosGeneral purpose technologies (GPTs) are regarded as a major source of productivity advancement and economic growth. As a kind of platform technology, GPTs have strong knowledge spillovers, which causes a single subject to lack R&D motivation and adopt a wait-and-see strategy. Cooperation R&D is an effective mode choice for GPTs. For this, three models based on upstream-led, downstream-led and balanced power structures were constructed to study the cooperation R&D modes of GPTs and influencing factors from a technology chain perspective. This study aims to reveal the effects of fairness concerns and power structures on three models. This study also focuses on the roles of knowledge spillovers and government support. The results indicate that different power structures will lead to an unequal distribution of profits between firm U and firm D in the technology chain. The balanced power structure should be the preferred model. The profits of firms in the leading position are always higher than those of firms in the following position. In addition, fairness concerns negatively impact the performance of firms, which may improve the bargaining ability of firms in the following position, but this does not bring a sustainable benefit. Government support (e.g., knowledge and technology support and R&D subsidies) and knowledge spillovers are two key factors influencing the decisions and outcomes of the technology chain. When a firm's relative innovation contribution level is greater, its profits in the leading position are the highest, followed by those in the balanced power structure, and they are lowest in the following position. In contrast, profits under balanced power are the highest, and those in the following position are still the lowest. This study enables a theoretical understanding of how and why the R&D process of GPTs can be regarded as a technology chain. It also sheds light on the fact that the balance power structure model should be the preferred choice and that both fairness concerns and government support should be considered for improving the R&D efficiency of GPT cooperation R&D in practice.
In the context of industrial revolution 4.0, the breakthroughs and application of key GPTs, such as semiconductors (Bresnahan & Trajtenberg, 1995), artificial intelligence (Rasskazov, 2020), blockchain Filippova (2020) and microcircuits, have become an important means for countries to seize the commanding heights of a new round of international competition. GPTs, which are recognized as a platform technology for further and continuous innovations that could spread across different sectors (Coccia, 2017), have been regarded as the ‘Engines of growth’ (Bresnahan & Trajtenberg, 1995) and a major source of productivity advancement and economic growth (Strohmaier & Rainer, 2016). However, GPTs have strong knowledge spillovers, which causes a single subject to lack R&D motivation and adopt a wait-and-see strategy and requires multiple subjects with their own advantages to carry out cooperative R&D. Hence, several important issues are raised: Which kind of GPT cooperation R&D model under the power structure is dominant? How can the key influencing factors and mechanisms of GPT cooperation R&D be revealed? These are very important questions in the GPT cooperation R&D fields. However, few studies (e.g., Strohmaier et al., 2016; Zheng et al., 2021) have addressed these issues systematically from a technology chain perspective.
To better understand the questions of GPT cooperation R&D, prior studies have discussed the mode of GPT cooperation R&D from two perspectives. First, the quasi-public goods characteristics of GPTs determine the characteristics of long period, continuous investment and knowledge spillovers with GPT R&D, which inevitably lead to “insufficient investment” in GPTs for firms that represents private interests under market mechanisms (Tassey, 2005). The model of GPT cooperation R&D with government support (e.g., subsidies, special plans, science and technology policies) can effectively alleviate the “insufficient investment” problems of GPT R&D (Tassey, 2005; Zuo et al., 2019; Zheng et al., 2021). Second, GPT R&D has obvious process characteristics (Zheng & Ren, 2020; Zheng et al., 2019; Zheng & Qin, 2021), which include two links: identifying and supply and commercial development. After GPTs are identified and supplied, commercial development activities can be launched and then release the potential of “engines of growth” for GPTs, which also requires firms in two links to cooperate vertically to prevent the “fracturing” of the GPT R&D process. Becker et al. (2004), Chen et al. (2019) and Fiori et al. (2022) found that the cooperation R&D is an effective mode choice for innovation activities. However, few studies (e.g., Zheng et al., 2020; Cen et al., 2021) have discussed GPT cooperation R&D from a technology chain perspective. Studies that consider factors such as power structures, knowledge spillovers and fairness concerns are even rarer.
We believe that the factors mentioned in the above paragraph should be critically considered from the technology chain perspective in GPT cooperation R&D. Although the models based on a technology chain are applicable in the discussion of the existing cooperation R&D frameworks (Belderbos et al., 2004; Fiori et al., 2022), prior studies seldom regard the R&D process of GPTs as a special technology chain and discuss the issues of GPT cooperation R&D between firm U and firm D from a technology chain perspective. Furthermore, power structures amongst cooperating firms are commonly considered in the technology chain framework (Perrons, 2009; Meng et al., 2018; Tang & Yang, 2020). Firms also pay considerable attention to knowledge spillovers (Audretsch & Belitski, 2020; Bernal et al., 2022) and fairness concerns (Pavlov & Katok, 2011; Ranjbar et al., 2020; Shen et al., 2021; Liu et al., 2021; Ren et al., 2022), which are the characteristics that cannot be ignored in cooperative innovation activities, and GPT cooperation R&D is no exception. However, there are almost no studies on power structures, knowledge spillovers and fairness concerns within the same framework. In addition, the quasi-public goods nature of GPTs has led to underinvestment in GPTs, and appropriate government R&D policies need to be developed on the basis of trade-offs between the expected benefits and risks of current and proposed R&D portfolios (Tassey, 2005). This dependency on policies has been evidenced in existing studies, for example, ICTs (Liao et al., 2016; Lee et al., 2022). Therefore, government support is also considered in our model.
While addressing the above limitations, this study grasps the chain relationship of successive dependencies between the identifying and supply and commercial development links of the GPT R&D process and establishes a theoretical framework of GPT cooperation R&D from a technology chain perspective. Accordingly, we construct models of upstream-led, downstream-led and balanced power structures by using the Stackelberg game theory to study the cooperation R&D modes of GPTs and influencing factors. Specifically, the technology chain is used as a theoretical lens to view the cooperation R&D process of GPT, which includes 1) the upstream firm (firm U), which identifies and supplies GPTs required by downstream firms, and 2) the downstream firm (firm D), which develops proprietary technology, processes or products with exclusive benefits based on GPTs offered by firm U (Zheng & Ren, 2020). Based on this, this paper studies the influencing factors of GPT cooperation R&D and the choice of cooperation R&D modes by establishing a Stackelberg game model considering power structures, knowledge spillovers and fairness concerns from the technology chain perspective.
The aims of our study are to examine the influence of power structures on the cooperation R&D process of GPTs, to examine the influence of fairness concerns on the R&D process of GPTs and to identify and determine the influences of other key factors, such as government support and knowledge spillovers, on the performance of the R&D process of GPTs. For this purpose, our model considers the cooperation process of GPTs between firm U, which identifies and supplies GPTs, and firm D, which conducts commercial development. Three models based on upstream-led (DU(s)), downstream-led (DU(s)) and balanced power structures (BE(s)) were constructed and examined with and without fairness concerns. In addition, this study reveals the effects of fairness concerns and power structures on three models of upstream-led (UD(s)), downstream-led (DU(s)) and balanced power structures (BE(s)), which also focuses on the roles of knowledge spillovers and government support. The results show that different power structures will lead to an unequal distribution of profits between firm U and firm D in the technology chain. Fairness concerns will reduce the efficiency and profits of the cooperation R&D process of GPTs. Government support and knowledge spillovers are the factors that cannot be ignored in the technology chain. In addition, the effects of firm's relative innovation contribution level on the cooperative R&D process of GPTs also deserve attention.
This study makes several theoretical contributions to the relevant literature. First, this study proposes an analytical framework of GPT cooperation R&D from a technology chain perspective and analyses the issues of GPT cooperation R&D by building Stackelberg game models under the UD(s), DU(s) and BE(s) scenarios. Second, we consider how factors such as power structures, fairness concerns, knowledge spillovers and government support contribute to achieving better R&D performance for GPTs, which helps address the dilemma of "double uncertainties" (Kokshagina et al., 2017; Zheng & Qin, 2021). More specifically, this study pays attention to the “fracturing” of the identifying and supply and commercial development links involved the GPT R&D process triggered by uncertainties in both technologies and markets. In addition, this study regards the R&D process of GPTs as a technology chain and introduces relevant research on power structures, fairness concerns, and knowledge spillovers (Ranjbar et al., 2020; Caldas et al., 2021; Niu et al., 2022) to GPT R&D fields and then places government support (Tassey, 2005; Zuo et al., 2019; Zheng et al., 2021) under the same framework, which enriches and promotes relevant research in GPT R&D fields (Zheng & Ren, 2020; Zheng & Qin, 2021; Cen et al., 2021; Ren et al., 2022). For practitioners, the present results can help firms systematically understand how and why the R&D process of GPTs can be regarded as a technology chain. It also provides a reference for promoting GPT cooperation in R&D practice.
The rest of this paper is organized as follows. In the next section, we review the relevant literature on GPTs and cooperative R&D. Based on this, we present the theoretical framework of our research by drawing figures and specify our assumptions and different research scenarios, which lay the foundation for game modelling. We then present the optimal solutions for players included in the R&D process of GPTs under different scenarios. Thereafter, the optimal solutions under different scenarios are analysed comparatively. Accordingly, we further explore the impact factors and mechanisms of effort levels and profits involved in GPT cooperation R&D by numeric analysis. Finally, we provide our theoretical implications and conclusions, implications for future research and practice, and the limitations of our study and suggestions for future research.
Literature reviewOur study concerns cooperation R&D for GPTs. Due to the risky nature of innovation, firms that participate in cooperative R&D activities have attracted attention from management studies (Lei et al., 2021; Fiori et al., 2022). The existing studies have provided evidence regarding the effectiveness and types of cooperation R&D (Becker & Dietz, 2004). As far as GPT R&D is concerned, researchers have examined the effects of GPTs on technological progress and economic growth (Lipsey et al., 2005; Andergassen et al., 2017; Petralia, 2021), where GPTs have been taken as an endogenous factor of production and studies on their mechanisms of promoting endogenous economic growth using the economic equilibrium paradigm from the perspective of macroeconomic effects. In addition, the R&D process of GPTs has also been a concern of researchers. Zheng et al. (2019, 2020, 2021) pointed out that GPT R&D has obvious process characteristics, which involve the identification and supply of GPTs and commercial development based on the GPTs identified and supplied, and these two links form an interdependent technology link relationship and can be regarded as a technology chain. Considering the quasi-public goods characteristics of GPTs (Tassey, 1992, 2005; Zheng et al., 2019), the mode of cooperation R&D amongst multiple subjects with their own advantages is an effective mode choice for GPTs. However, few studies (e.g., Zheng et al., 2020) discuss GPT cooperation R&D between firm U and firm D from a technology chain perspective. For the GPT cooperation R&D relationship to work, our study considers power structures, knowledge spillovers and fairness concerns to be the main influential factors in the context of GPT cooperation R&D.
The concept of power structure is a key topic in cooperation R&D, and prior studies mainly focus on the field of supply chain management. Wang et al. (2019) studied a balanced power structure and argued that this model is often conducive to the whole supply chain but might not perform very well in a supply chain with isoelastic demand and additive shock. In a wider context, Ranjbar et al. (2020) constructed a game model of different power structures in a three-level closed-loop supply chain and pointed out that the decentralized retailer leadership model is often the most effective scenario. Similarly, Shen et al. (2021) studied the value of collaborative innovation and decisions considering supplier-led and manufacturer-led innovation games. Niu et al. (2022) developed models with power in a dyadic supply chain to investigate whether a manufacturer should invest in uncertain decarbonizing innovation and how power affects investment incentives, firm profitability, consumer surplus, and system performance. Although these studies mainly focus on supply chain relations, we argue that relationships based on the supply chain are similar to GPT cooperation R&D processes, especially when using the technology chain for conceptualization. Here, GPTs identified and supplied could be viewed as supplies in the supply chain. Applying power structure in modelling GPT cooperation R&D activities is not common in the current literature. An exception is a recent study from Zheng and Qin (2021)) that examined the impact of different government subsidies on the sustainable R&D behaviour of GPT under different power structures. Therefore, the supply chain concept is applied to the R&D process of GPT cooperation, which is regarded as a technology chain. For this purpose, Stackelberg game models for GPTs with UD(s), DU(s) and BE(s) power structures are built to study the influencing factors and model selection of GPT cooperation R&D.
Knowledge spillovers, which mean an unintentional share of knowledge (Sun et al., 2021), are likely to occur in GPT cooperation R&D between firm U and firm D from the technology chain. In understanding the impact of knowledge spillovers, Caldas et al. (2021) showed that spillovers are the most relevant external knowledge source in explaining the product innovation performance and growth of small and medium-sized firms; meanwhile, they could play a crucial role in the outcomes of large firms. Further support for the importance of knowledge spillovers is given by Audretsch and Belitski (2020), who show that complementarities between R&D and knowledge spillovers are strongly associated with firm productivity rather than with firm innovation. Specifically, regarding the cooperation R&D relationship, Bernal et al. (2022) investigated the interplay of formal collaboration and incoming spillovers by distinguishing four collaboration-spillover scenarios and pointed out that incoming knowledge spillovers may amplify or limit collaboration but that they only partly substitute formal collaboration in regard to impacts on performance. As far as GPTs are concerned, Lo and Sutthiphisal (2010) took electronic technology as an example and found that knowledge spillovers between industries had little influence on the geography of crossover inventions and the speed and productivity of crossover inventors. Cen et al. (2021) studied the impact of patent cooperation network characteristics on GPT spillover and found that the relative centrality of the patent cooperation network has a significant positive impact on GPT spillover. Bettiol et al. (2022) made a first attempt to link groups of ICT to groups of Industry 4.0 technologies, the finding shows strong path dependency amongst ICT, Industry 4.0 and knowledge performance. As a result, it can be argued that knowledge spillovers are a broad concern for technological cooperation and innovation and also applies to cooperation between firm U and firm D in the technology chain, especially for GPTs, where there are double uncertainties regarding GPT R&D. Consequently, the concept of knowledge spillovers completes our thinking of the GPT cooperation R&D model from a technology chain perspective.
In addition, existing studies are also dedicated to factors such as different effort inputs and the distribution of outcomes, which have been conceptualized as fairness concerns. Kumar and Steenkamp (1995) found that vulnerable resellers’ perceptions of both distributive and procedural fairness enhance their relationship quality. Liu et al. (2020) analysed the impacts of fairness concerns on supply chain decisions under centralized and decentralized scenarios and designed a coordination mechanism through cost sharing. It is also shown in Liu et al. (2021) that fairness concerns affect cooperation relationships in a three-party sustainable supply chain; accordingly, the authors developed a novel coordination method addressing this issue. Yang and Sun (2022) studied prices and low-carbon strategies considering dual fairness concerns and different competitive behaviours in a two-echelon supply chain. Their research findings show that regardless of the type of pattern that retailers adopt, the distributional fairness concerns of the manufacturer have a negative impact on the CER (carbon emission reduction) level but have a positive effect on wholesale and retail prices, while peer-induced fairness increases the CER level and reduces wholesale and retail prices. Ren et al. (2022) studied the effects of fairness concerns on price and quality decisions made in an IT service supply chain and pointed out that the existence of a firm's fairness concerns not only reduces its own profit but also decreases the profit of the partner, which results in a loss of IT service supply chain performance. Consequently, it is evidenced that fairness concerns have a large impact on cooperation relationships, especially when factors such as uncertainty and information asymmetry are significant. However, fairness concerns have not received attention in work on GPT cooperation R&D even though this is a very important research topic. Due to the need for GPT R&D to address "double uncertainties" (Terwiesch & Xu, 2008; Hooge et al., 2012; Kokshagina et al., 2017), fairness concerns are a key factor affecting the behaviour of firm U and firm D of the technology chain in our model.
Government support is also an important topic in GPT R&D. GPTs are pervasive (Bresnahan & Trajtenberg, 1995) and can also be called quasi-public goods binding firms that represent private interests to "insufficient investment" in GPTs under the market mechanism (Tassey, 2005). Governments that act on behalf of social interests can effectively balance "insufficient investment" through R&D subsidies, special plans, and science and technology policies (Tassey, 1996, 1997, 2005; Zuo et al., 2019; Zheng et al., 2019, 2021). For example, Tassey (2005) pointed out that the public goods nature of GPTs has led to underinvestment, and appropriate government R&D support policies need to be developed on the basis of trade-offs between the expected benefits and risks of current and proposed R&D portfolios. Zuo et al. (2019) applied three-way decision theory to address the mechanism design of government support, which represents subsidies, delayed decision-making and no subsidies, under the R&D of new energy vehicle industry GPTs in China. Zheng et al. (2021) pointed out that government subsidies, knowledge and policy support can help alleviate the failure of insufficient investment in GPTs and that when government support is not sufficient or is improper, a “failure of government intervention” can easily result. However, the literature has not systematically studied the role of government support in GPT cooperation R&D considering power structures, fairness concerns and knowledge spillovers within the technology chain, which is an important focus of our study.
Theoretical framework and modellingTheoretical frameworkWe viewed the GPT cooperation R&D process, which involves two interdependent links, including the identification and supply of GPTs and commercial development based on the GPTs identified and supplied, as a special technology chain and considered relevant factors that may impact this process. The theoretical framework of our study is shown in Fig. 1.
As shown in Fig. 1, firm U and firm D in the technology chain constitute the main subjects of the GPT cooperation R&D process. Firm U is responsible for identifying and supplying GPTs and provides GPTs for firm D to promote commercial development, and then firm D adopts the GPTs from firm U; conducts commercial development to realize market value based on GPTs; and, at the same time, provides information and knowledge and technology support to support firm U's work. For the GPT cooperation R&D process, this raises the following question: who is the leader of the technology chain? To address this problem, considering the importance of the power structure to GPT cooperation R&D, three models based on DU(s), DU(s) and BE(s) were constructed and examined both with and without fairness concerns. In addition, this study focuses on the effect of knowledge spillovers and government support on GPT cooperation R&D. Accordingly, from a technology chain perspective, we construct GPT cooperation R&D models considering power structures, fairness concerns, knowledge spillovers and government support to analyse the impacts of different power structures on optimal R&D strategies and profits.
Basic assumptions and modelling- (1)
We denote the effort level of firm U and firm D as euanded, respectively, representing the knowledge and resource inputs of the firms. Similar to prior literature (e.g., Atasu & Subramanian, 2012; Ranjan & Jha, 2019), we assume that the costs of the firms' effort are Cu=ηueu2/2 and Cd=ηded2/2, respectively, whereηi>0is the effort cost multiplier for firm i(i=u,d)and where the smaller the multiplier is, the higher the degree of R&D efficiency is.
- (2)
We assume that the unit operating costs of firm U and firm D are cuand cd, respectively. As shown in Fig. 1, knowledge spillovers exist in GPT cooperation R&D that can be transferred from firm D to firm U and from firm U to firm D in the technology chain. Letβidenote the knowledge spillovers of firmito firmj, wherei,j=u,d,i≠j, satisfying cu,cd>0,0<βi<1. Then, the unit operating costs of firm U and firm D under GPT cooperation R&D between firm U and firm D are cu−βdedand cd−βueu, respectively.
- (3)
Assume that firm U and firm D in the technology chain sign a GPT patent licensing contract. GPTs have the characteristics of quasi-public goods, which depend on the externality in GPT cooperation R&D. This feature can be characterized by the commonness degree of GPTs (Zheng & Wang, 2019), denoted asλ, 0<λ<1. The patent licensing pricew(w>0) of GPTs is determined by firm U and is affected by the commonness degreeλ. A largerλmeans more externality amongst GPTs, and firm D will pay less to obtain GPTs with a high commonness level. Thus, we assume that the unit patent licensing price is (1−λ)w.
- (4)
Assume that the market size isD0and the final product sell unit price isp. The product innovation efforts made by the manufacturer can create demand and increase consumer utility (Chen et al. 2017). According to this, the demand function is given byD=D0−bp+α(eu+ed), whereαis the effect of effort levels on market demand, which denotes the product innovation return based on GPTs, and b denotes the price elasticity of demand, satisfying α>0, 0Chen et al., 2017; Yenipazarli, 2017).
- (5)
The government provides policy guidance and subsidies to encourage GPT R&D activities by, for example, guiding and supporting relevant industries (such as the AI, blockchain and chip industries) and firms to develop key GPTs based on national strategic needs. We consider the operational cost per unit of firm U to be (1−g)cu−βded, wheregis the government's support for identifying and supplying GPTs, satisfying0
- (6)
GPT R&D is the coexistence process of cooperation and competition between firm U and firm D from the technology chain perspective. First, firm U and firm D jointly decide on R&D efforts to maximize the total profits of the technology chain. Second, firm U and firm D make operational decisions on patent licensing pricewand sell pricep; the sequence of decisions depends on the power structures in the technology chain. Specifically, there are three scenarios and game time sequences.
In the UD scenario, firm U is the leader of the technology chain. The game time sequence can be stated as follows: in step 1, the government sets subsidiesθi; in step 2, firm U and firm D jointly decide on R&D efforts to maximize the total profits of the technology chain; in step 3, firm U offers a licensing pricew; and in step 4, firm D decides on its sell pricepin response.
In the DU scenario, firm D is the leader of the technology chain. The game time sequence can be stated as follows: in step 1, the government sets subsidiesθi; in step 2, firm U and firm D jointly decide on R&D efforts to maximize the total profits of the technology chain; in step 3, firm D decides on its sell pricep; and in step 4, firm U sets the licensing pricewin response.
In the BE scenario, firm U and firm D are in an equal position. The game time sequence can be stated as follows: in step 1, the government sets subsidies θi; in step 2, firm U and firm D jointly decide on R&D efforts to maximize the total profits of the technology chain; and in step 3, firm U and firm D decide on their licensing price w and sell price p.
The profit functions of firm U, firm D and the revenue of government G are as follows:
The total profits of the technology chain are obtained from Eqs. (1) and (2):
- (1)
Assume that both firm U and firm D in the technology chain consider fairness concerns and aim to maximize their own profits by referring to the profits distributed by the other firm. To simplify the calculation without losing generality, we assume that the fairness concern levels of firm U and firm D areN(0≤N≤1). Similar to previous literature (Liu et al., 2020; Nie & Du, 2017), the utility function of firm U and firm D can be expressed as follows:
It can be seen from Eqs. (1)-(6) that the profits of firm U and firm D are composed of patent income (sales income), the cost of R&D effort, and government subsidies. The government's profit function consists of four parts: the profits of firm U and firm D, the total subsidies provided by government and consumer surplus. Regarding fairness concerns, the utility of firm U and firm D is composed of their own profits and the profits of the other firm. For ease of exposition, we let {UD,DU,BE} denote the UD, DU, and BE scenarios without fairness concerns and{UDs,DUs,BEs}denote the corresponding three situations where fairness concerns are considered. Table 1 provides a list of key notations used in this paper.
Summary of key notations.
According to assumption (6), firm U is the leader of the technology chain. The specific solving procedure used is shown in Appendix A.
The UD scenario without fairness concerns (UD)In this case, firm U and firm D both make decisions to maximize their own profits, and the optimal effort levels of firms in the UD scenario are as follows:
The optimal government subsidies and optimal price strategies of firm U and firm D are as follows:
The optimal profits for game parties are as follows:
where RuUD=(α+bβu)2(6+b)/6bηu,RdUD=(α+bβd)2(6+b)/6bηd, and we assume that RuUD<169, RdUD<89 to ensure that firms’ effort levels and outputs are positive. Rik(s) represents the innovation contribution level of the GPT R&D of firmi(Chen et al., 2019; Ge et al., 2014), k∈{UD(s),DU(s),BE(s)},i∈{u,d}.The UD scenario with fairness concerns (UDs)In the UD scenario, firm U and firm D make decisions to maximize their own utility, and the optimal effort levels of firms in the UD scenario are as follows:
The optimal government subsidy and the optimal price strategies of firm U and firm D are as follows:
The optimal profits for game parties are as follows:
The utilities of firm U and firm D are as follows:
where TUDs=(2(N+2)2−(3+2N)RuUDs−(3+2N)RdUDs), H=[D0−b(1−g)cu−bcd], RuUDs=(α+bβu)2(2(3+2N)+b)2(3+2N)bηu and RdUDs=(α+bβd)2(2(3+2N)+b)2(3+2N)bηd are the innovation contribution levels of firm U and firm D in the UD scenario, where 2(1+N)2(N+2)2(2N+1)b−(3+2N)22bRuUDs>0, (2N2+4N+1)(N+2)2(2N+1)b−(3+2N)22bRdUDs>0.The DU scenarioAccording to assumption (6), firm D is the leader of the technology chain. The specific solving procedure is shown in Appendix A.
The DU scenario without fairness concerns (DU)In this case, firm U and firm D make decisions to maximize their own profits, and the optimal effort levels of firms in the DU scenario are as follows:
The optimal government subsidies and optimal price strategies of firm U and firm D are as follows:
The optimal profits for game parties are as follows:
where RuDU=(α+bβu)2(6+b)6bηu and RdDU=(α+bβd)2(6+b)6bηd are the innovation contribution levels of firm U and firm D, respectively, which satisfy the condition 8−9RuDU>0, 16−9RdDU>0.The DU scenario with fairness concerns (DUs)In the DU scenario, firm U and firm D make decisions to maximize their own profits, and the optimal effort levels of firms are as follows:
The optimal government subsidies and the optimal price of firm U and firm D are as follows:
The optimal profits for game parties are as follows:
The utilities of firm U and firm D are as follows:
where TDUs=[8(1+N)2−(3+4N)RuDUs−(3+4N)RdDUs],H=[D0−b(1−g)cu−bcd].RuDUs=(α+bβu)2(2(3+4N)+b)2(3+4N)bηu and RdDUs=(α+bβd)2(2(3+4N)+b)2(3+4N)bηd are the innovation contribution levels of firm U and firm D in the DU scenario, which satisfy 4(1+4N)(1+N)3(1+2N)b−(3+4N)2RuDUs2b>0 and 4(4N2+5N+2)(1+N)2(1+2N)b−(3+4N)2RdDUs2b>0.
The BE scenarioIn the BE scenario, firm U and firm D are in an equal position. The specific solving procedure is shown in Appendix A.
The BE scenario without fairness concerns (BE)In this case, firm U and firm D make decisions to maximize their own profits, and the optimal effort levels of firms in the BE scenario are as follows:
The optimal government subsidies and the optimal price strategies of firm U and firm D are as follows:
The optimal profits for game parties are as follows:
where RuBE=(α+bβu)2(4+b)/4bηu and RdBE=(α+bβu)2(4+b)/4bηd are the innovation contribution levels of firm U and firm D, which satisfy 9−8RuBE>0, 9−8RdBE>0.The BE scenario with fairness concerns (BEs)In the BE scenario, firm U and firm D make decisions to maximize their own utility, and the optimal effort levels of firms in the BEs scenario are as follows:
The optimal government subsidy and the optimal price strategies of firm U and firm D are as follows:
The optimal profits for game parties are as follows:
where TBEs=[9(1+N)2−2(2+3N)RuBEs−2(2+3N)RdBEs],H=[D0−b(1−g)cu−bcd].RuBEs=(α+bβu)2(2(2+3N)+b)2(2+3N)bηu and RdBEs=(α+bβd)2(2(2+3N)+b)2(2+3N)bηd denote the innovation contribution level in the BEs scenario of firm U and firm D, satisfying 9(1+3N)(1+N)3(1+2N)b−2(2+3N)2RuBEsb>0, 9(3N2+3N+1)(1+N)2(1+2N)b−2(2+3N)2RdBEsb>0.
Model analysisComparison of GPT cooperation R&D modelsThe specific solving procedures of Propositions 1-7 are shown in Appendix B.
Proposition 1 The government subsidies, the optimal effort level of firm U and firm D, the total R&D profits of the technology chain and the expected revenue of the government with fairness concerns are lower than those without fairness concerns, i.e.,θks<θk,eiks
Proposition 1 indicates that compared to not considering fairness concerns, the ratio of government subsidies, the optimal effort level of firm U and firm D, the total profit of R&D in the technology chain and the expected revenue of the government are lower when considering fairness concerns. This is because firms may pay more attention to the profits of the cooperating firm when considering fairness concerns. Firm D may bargain with firm U by using the commercialization of GPTs for bargaining to obtain higher R&D profits. In contrast, firm U will resist bargaining behaviour by raising the transfer price of GPTs or reducing the level of effort to save costs, which restricts the efficiency of GPT cooperation in R&D. As a result, fairness concerns have a negative impact on GPT cooperation R&D and reduce R&D profits.
Proposition 2 Whether or not fairness concerns are taken into account, the R&D effort level of firms, the ratio of government subsidies, government expected revenue and the total profits of the technology chain in the UD(s) and DU(s) scenarios are lower than those in the BE(s) scenarios. Specifically, When not considering fairness concerns, the R&D effort level of firms, the ratio of government subsidies, government expected revenue and R&D profits of the technology chain in the UD and DU scenarios are equal, i.e.,eiBE>eiUD=eiDU,θBE>θUD=θDU,πGBE>πGUD=πGDU and πTBE>πTUD=πTDU, i∈{u,d}. When considering fairness concerns, the R&D effort level of firms, the ratio of government subsidies, government expected revenue and R&D profits of the technology chain in the UD scenario are greater than those in the DU scenario, i.e.,eiBEs>eiUDs>eiDUs, θBEs>θUDs>θDUs, πGBEs>πGUDs>πGDUs,πTBEs>πTUDs>πTDUs, i∈{u,d}.
From Proposition 2, power structures will lead to unequal profit distribution between firm U and firm D and affect the effort level of firms and thus the total profits of the technology chain. Specifically, the effort level of firms and the total profits of the technology chain are improved in the BE(s) scenarios. This is because in GPT cooperation R&D, if the positions of firm U and firm D in the technology chain are not equal, they will have an incentive to participate in the leadership struggle to obtain greater profits, causing the “friction” of cooperation R&D amongst GPTs to increase and then leading to a loss of R&D profits. In addition, the ratio of government subsidies and the expected revenue of the government are higher than those in the UD and DU scenarios. When not considering fairness concerns, both firm U and firm D will independently pursue their own profit maximization. Considering that firms in the leading position have more bargaining power, both firms will actively compete for leadership. As a result, when the game is optimal, the effort level and R&D profit of firm U and firm D are equal, and the ratio of government subsidies and the expected revenue of the government are also equal in the UD and DU scenarios. When considering fairness concerns, both firm U and firm D will be concerned about the profits of the cooperating firms. Because the commercial development of firm D depends on the GPTs identified and supplied by firm U, compared to firm D, firm U has the advantage of fighting for leadership to obtain higher profits. Therefore, the R&D profits of firm U and firm D in the UD scenario are higher than those in the DU scenario, and the ratio of government subsidies and the expected revenue of the government are also higher.
Proposition 3 The R&D profits of the firm in the leading position are always greater than those in the following position in the technology chain. When the innovation contribution level of firms is lower, the profits of the firm in BE(s) scenarios are highest, followed by those of the firm in the leading position, and the profits of the firm in the following position are lowest, i.e., πuBE(s)>πuUD(s)>πuDU(s), πdBE(s)>πdDU(s)>πdUD(s). When the innovation contribution level of firms is higher, the R&D profits of firms in the leading position are highest, followed by those of the BE(s) scenarios, and the profits of the firm in the following position are lowest, i.e.,πuUD(s)>πuBE(s)>πuDU(s), πdDU(s)>πdBE(s)>πdUD(s).
From Proposition 3, the firms in the leading position can obtain higher profits in the UD and DU scenarios because the leading firms have more bargaining power than the following firms, and then firm U can identify and supply GPTs according to its own advantages and market demand such that the leading firms can obtain higher profits. When the relative contribution of firms to innovation is greater, the GPT R&D profits of firms in the leading position are the highest because, at this point, firms have the most power in the technology chain. In BE(s) scenarios, firms tend to fight for leadership to obtain greater profits, which will affect the smooth development of GPT cooperation R&D and then affect R&D profits. When the relative contribution of firms to innovation is lower, the firms will also actively fight for leadership because of their similar contributions to GPT R&D. When the game is optimal, GPT cooperation R&D will be carried out in BE(s) scenarios. The above conclusions show once again that power structures will affect the profit distribution between firm U and firm D in the technology chain.
Analysis of factors affecting decisions and profits of firmsProposition 4 Government supportgfor GPTs can improve the effort level and profits of firms. The government's subsidies to the effort costs of firm U and firm D are equal and are affected by the price elasticity of demandband the level of fairness concernsN. The greaterbis, the greater the ratio of effort cost subsidies is, and the greaterNis, the smaller the ratio of effort cost subsidies is.
Proposition 4 illustrates that information and knowledge and technology support, which are provided by the government to firm U for identifying and supplying GPTs, can, to a certain extent, compensate for the lack of firms’ innovation resources and then improve firm effort level and R&D profits. In addition, because the process nature of GPT R&D determines the characteristics of the long period and continuous investment that GPT R&D faces, the government gives equal subsidies for the effort costs of firm U and firm D to ensure the smooth development of the GPT R&D process. The greater the price elasticity of demand is, the more sensitive consumers will be to GPT-based products. At this point, increasing the ratio of government subsidies is required to share the R&D risks faced by firms, which is equivalent to putting an "approval label" on GPT-based products (Feldman & Kelley, 2006) and which helps increase consumers’ trust in products based on GPTs and then lowers the price elasticity of demand. Moreover, because the profits distributed by the cooperating firm are used as a reference by firms to maximize their own profits, the behaviour of fairness concerns will lead to interest conflicts in firms’ GPT cooperation R&D, and then the R&D effort level and price strategy of firms will tend to increase their own profits, which will reduce the market expectations of GPT R&D. Therefore, as fairness concerns increase, government subsidies decrease.
Proposition 5 Knowledge spilloversβiand the product innovation returnαbased on GPTs are helpful to improve the R&D effort level of firms, the total R&D profits of the technology chain and the expected revenue of the government. However, an increase in the effort cost multipliersηiof firms will lead to a decrease in the effort levels of firms, the total profits of the technology chain and government expected revenue, i.e.,∂eik(s)∂βi>0,∂πTk(s)∂βi>0,∂πGk(s)∂βi>0,∂eik(s)∂α>0,∂πTk(s)∂α>0,∂πGk(s)∂α>0,∂eik(s)∂ηi<0,∂πTk(s)∂ηi<0,∂πGk(s)∂ηi<0,i∈{u,d},k∈{UD,DU,BE}.
From Proposition 5, the process of sharing innovation knowledge is essential to GPT cooperation R&D between firm U and firm D. With the increase in knowledge spilloversβi, firm U can obtain more market information and innovation resources from firm D, and firm D can also acquire knowledge of GPTs that is beneficial to commercial development, which stimulates the level of R&D effort of firm U and firm D and then increases the total profit of the technology chain and the expected revenue of the government. The increase in the product innovation returnαincreases the expected returns of GPT cooperation in R&D, which encourages firms to increase investment in GPTs and promotes an increase in the total profit of the technology chain and the expected revenue of the government. The increase in R&D effort costs directly dampens enthusiasm for GPT R&D efforts amongst firms and reduces the total profit of the technology chain and the expected revenue of the government.
Proposition 6 The influences of knowledge spillovers, effort costs and product innovation return on the R&D profit of firm U and firm D are affected by the power structures. In any power structure, the firm's knowledge spilloversβi are conducive to improving the profits of firms, i.e.,πjk. In contrast, the firm's effort cost multipliersηiwill reduce the profits of the cooperating firmπjk, i.e., ∂πjk(s)∂βi>0, ∂πjk(s)∂ηi<0, i,j∈{u,d}, k∈{UD,DU,BE}. When not considering fairness concerns, the R&D profits πik of firms in the leading position are positively correlated with their own knowledge spilloversβi and are negatively correlated with their own effort cost multipliers ηi, i.e.,∂πuUD∂βu>0,∂πuUD∂ηu<0,∂πdDU∂βd>0,∂πdDU∂ηd<0. The R&D profitsπikof firms in the following position are negatively correlated with their own knowledge spilloversβiand positively correlated with their own effort cost multipliersηi, i.e.,∂πdUD∂βd<0, ∂πdUD∂ηd>0, ∂πuDU∂βu<0, ∂πuDU∂ηu>0. In BE scenarios, when the relative innovation contribution levelRiBEof firms is lower, their R&D profits are positively correlated with their own knowledge spilloversβiand negatively correlated with their own effort cost multipliersηi. When the relative innovation contribution level RiBE of firms is higher, their R&D profit is negatively correlated with their own knowledge spilloversβiand positively correlated with their own effort cost multipliers ηi, i.e., WhenRdBE>RuBE, ∂πuBE∂βu>0, ∂πuBE∂ηu<0, ∂πdBE∂βd<0, ∂πdBE∂ηd>0; When RdBE Considering fairness concerns, whenRuUDs As far as the product innovation return α is concerned, when not considering fairness concerns, the profits of firms in the leading position increase with α, while the profits of firms in the following position only increase with α when the innovation contribution level of the leading firm is higher. When considering fairness concerns or BE(s) scenarios, the profits of firms only increase withαwhen the cooperating firm's innovation contribution level is higher; otherwise, such profits decrease with α.
Proposition 6 illustrates that the R&D profits of firm U and firm D are affected by knowledge spillovers, effort costs and product innovation returns, and this influence is also affected by the power structure. First, the knowledge spillovers of firms are conducive to improving the R&D profits of cooperating firms, while an increase in the cost coefficient of firms’ efforts will reduce their R&D profits. This is the case because the knowledge spillovers of identifying and supplying GPTs are beneficial to the commercial development of firm D, and the knowledge spillovers from firm D, such as market information, also help identify and supply GPTs, which help improve the total profits of the technology chain (see Proposition 5) and thus the R&D profits of the cooperating firms. Similarly, the increase in the effort cost coefficient dampens the enthusiasm of firms to invest in GPT R&D and erodes the total profits of the technology chain (see Proposition 5) and then the R&D profits of the cooperating firms.
Second, when not considering fairness concerns, firms will not consider the profits earned by the cooperating firm when pursuing their own profit maximization. The knowledge spillovers of the firms in the leading and following positions are beneficial to improving the R&D profits of the cooperating firms (see Proposition 6(1)), which help improve the R&D profits of firms in the leading position. Effort costs have the opposite effect. The knowledge spillovers of firms in the following position are at a disadvantage in the technology chain, and their knowledge spillovers further reduce the bargaining ability of the cooperating firm and thus reduce its R&D Profits. At this time, the firm in the following position may make more efforts to absorb the knowledge of the leading firms, which increases the R&D effort costs and improves the R&D profits of GPTs. When the power of firm U and firm D is balanced in the technology chain, the effect of their knowledge spillovers and effort costs on the R&D profits of firms is affected by the relative innovation contribution level of firms. Because firm U and firm D compete with each other on an equal position, if the innovation contribution level of firms is lower, it is evidenced that knowledge spillovers helps improve the R&D profits of the cooperating firms (see Proposition 6(1)) and then the state of cooperative R&D, which can increase the total profits of the technology chain (see Proposition 5) and thus also enhance the R&D profits of firms themselves. Similarly, effort costs erode R&D profits. If the relative innovation contribution level is higher, the loss of knowledge spillovers of firms may be difficult for cooperating firms to address. A possible way for firms to improve their R&D profits is to increase their R&D efforts to reduce knowledge spillovers to enhance their bargaining ability.
Third, when considering fairness concerns, the influences of knowledge spillovers and the effort cost coefficient on the R&D profits of firms are impacted by the power structure and the relative innovation contribution level of firms. Although fairness concerns are taken into account, leading firms can obtain higher R&D profits from more influence and dominance that they have. Similarly, effort costs reduce firms' R&D profits. Because firms in the following position do not have dominance in the technology chain, knowledge spillovers may damage their own profits. Rational firms may actively absorb knowledge from leading firms by increasing their GPT R&D efforts and strengthening GPT cooperation R&D to improve their own R&D profits. When the relative innovation contribution level of firms in the leading position exceeds a certain threshold, the increase in knowledge spillovers may weaken the bargaining ability of firms and reduce R&D profits. At this point, firms should put more effort into reducing knowledge spillovers, which in turn may improve the R&D profits of firms. Similarly, in the BE scenario, when the relative innovation contribution level of firm U is lower than a certain threshold, the R&D profits of the firm are positively related to its knowledge spillovers and negatively related to the effort cost multiplier. With an increase in the relative innovation contribution level, when it exceeds the threshold, the correlation relationship between the R&D profits of firms and their knowledge spillovers changes from positive to negative. At this point, this can improve profits through more investment in R&D efforts.
Finally, the production innovation return can improve a firm's effort level and the total profits of the technology chain (see Proposition 5), but its impacts on the R&D profits of firms are regulated by the power structure and the relative innovation contribution level of firms. When not considering fairness concerns, the product innovation return is beneficial for increasing market demand for products based on GPTs. The R&D profits of firms in the leading position increase with the increase in the production innovation return. If the relative innovation contribution level of the leading firms is higher, firms in the following position can share the profits of GPT cooperation R&D without investing too much. Therefore, the R&D profit of the firms also increases with the increase in the production innovation return. When considering fairness concerns or a balanced power structure, if the relative innovation contribution level of the cooperating firms is higher and that of firms themselves is lower, they will raise the R&D effort levels of GPTs by referring to the profits of the cooperating firm and its own input. With the increase in product innovation returns, the total profits of the technology chain will increase, and the R&D profits of firms will increase correspondingly. If GPT cooperation R&D is perceived to be unfair, firms will reduce the level of their R&D efforts and then affect the smooth development of GPT cooperation R&D. At this point, despite the increase in the production innovation return, the GPT R&D profits of firms decline.
Proposition 7 The impacts of fairness concerns on the price strategy, R&D effort level and profits of firms are as follows. Firm D's price increases with fairness concern level N. Firm U's patent licensing price per unitwdecreases with fairness concerns when firm U is in the leading position of the technology chain and increases with fairness concerns when firm U is in the following position or in the BE scenario. In the UD and DU scenarios, the profits of leading firms are always negatively correlated with the fairness concern level, and the profits of following firms first increase and then decrease with the fairness concern level. Under the balanced power structure, the profits of both firm U and firm D are negatively correlated with the level of fairness concerns. In any R&D scenario, the effort level of firm U and firm D, the total R&D profits of the technology chain, and the expected revenue of the government are negatively correlated with the fairness concern level.
Proposition 7 illustrates that the fairness concern behaviour of firms will reduce the total profits of the technology chain and the effort level of firms. When a firm pays more attention to the cooperating firm's profits, its profits are more obviously affected by the cooperating firm. As far as firm D is concerned, an increase in the level of fairness concerns under any R&D scenario would lead to higher prices. Firm U has the advantage of making profits when it is in the leading position. To avoid reducing R&D efficiency, firm U will make appropriate price concessions to firm D as its fairness concern level increases. When firm U is in the following position or in the BE scenario, firm U will not easily make concessions to the product price because there is no longer the advantage of making profits. Because fairness concerns increase the bargaining power of firms in the following position, the profits of the following firms first increase and then decrease with the level of fairness concerns. In general, fairness concerns have a negative impact on the R&D profits of firms in the technology chain and the R&D efficiency of GPTs. Our conclusions are similar to those drawn by Liu et al. (2021) in their research on a fairness coordination mechanism in the supply chain context.
Numeric analysis of the modelIn this section, we analyse the impact factors and mechanisms of the relevant parameters for effort levels and profits in the technology chain using numeric analysis. Satisfying relevant assumptions and constraint conditions, let the market size be D0=20, the price elasticity of demand be b=0.3, the unit operating cost of firm U and firm D be cu=cd=2, the product innovation return based on GPTs be α=0.3, the government's support be g=0.3for identifying and supplying GPTs, the fairness concern level be N=0.3, knowledge spillovers be βi∈(0,1), and the effort cost multipliers of firms be ηi∈[1.5,3]. Referring to Chui et al. (2021), we provide a list of the values and ranges of the above parameters (see Table 2). When analysing the impact of a parameter, other parameter values remain unchanged unless otherwise specified.
First, we analyse the impacts of government supportgfor identifying and supplying GPTs, the product innovation returnαand power structures on firms’ effort level and total profits of the technology chain, as shown in Fig. 2.
As shown in Fig. 2, taking the profits of the technology chainπTas an example, πT increases asgandαincrease. As shown in Fig. 2(a), πTwithout considering fairness concerns is higher than that considering fairness concerns; that is, fairness concerns will reduce the R&D efficiency and profits of GPTs. Furthermore, as shown in Fig. 2(b), πTis equal to that of the UD/DU scenarios but lower than that of the BE scenario. Similarly, when considering fairness concerns, the effort level of firms and profits of the technology chain are highest in the BE scenario, followed by those of the UD scenario, and they are the lowest in the DU scenario. The impacts ofgon firms’ effort level and government revenue are similar to those shown in Fig. 2. The effects of the price elasticity of demand b and fairness concern level Non the ratio of effort cost subsidies can be analysed similarly but is not repeated here. Thus, Propositions 1, 2, and 4 and parts of Proposition 5 are verified.
As shown in Fig. 3, taking the state not considering fairness concerns as an example, the profits of firms under different power structures are impacted by their relative innovation contribution levelRi(i=u,d). When Rd of firm D is higher (Ru/Rd=0.26), as shown in Fig. 3(a), theπuof firm U under the BE scenario is the largest. However, whenRddecreases (Ru/Rd=1), the πuof firm U under the UD scenario is the largest. In addition, the πuof firm U in the UD(s) scenarios is always greater than that in the DU scenario, which indicates that when Rd is larger, the πuof firm U in the balanced power structure is greater than that in the unbalanced power structure, and the πuof firm U in the leading position is greater than that in the following position. Fig. 3(b) shows that the situation of firm D is similar to that of firm U. In addition, the impacts of the profits of firm U and firm D when considering fairness concerns are similar to those when fairness concerns are not considered. Proposition 3 is verified.
Next, we analyse the impacts of knowledge spilloversβiand effort cost multipliersηion firms’ effort level, the profits of the technology chain and government expected revenue. We use the total profits of the technology chain πT as an example, as shown in Fig. 4 and Fig. 5.
Whether or not fairness concerns or power structure are considered, as shown in Fig. 5, the total profits πT of the technology chain increase with βiand decrease with a firm's ηi. Furthermore, it can be seen from Fig. 4 and Fig. 5 that the total profit relation of the technology chain isπTBE>πTUD=πTDUwhen considering fairness concerns and πTBE>πTUD>πTDUwhen not considering fairness concerns. The comparisons of the effort levels of firms and the ratio of government subsidies and government revenue can be analysed similarly. From these findings and combined with Fig. 2(b), Proposition 2 and Proposition 5 are verified.
Furthermore, as shown in Fig. 6, we analyse the impacts of βi and ηi on the R&D profits πjof the cooperating firms. As shown in Fig. 6, whether or not fairness concerns are considered, the profitsπuof firm U increase with theβdof firm D and decrease with the ηd of firm D. The profits of firm D also increase with theβuof firm U and decrease with theηuof firm U. Therefore, Proposition 6(1) is verified. In addition, as shown in Fig. 7, when not considering fairness concerns, the R&D profits of firms in the leading position are positively related to their βi and negatively related to their ηi. In contrast, the R&D profits of firms in the following position are negatively related to theirβiand positively related to theirηi. Furthermore, as shown in Fig. 7(c)−7(d), when theRuof firm U is lower than theRdof firm D, the R&D profits of firm U are positively correlated withβuand negatively correlated withηu, while the opposite applies for firm D. As a result, under the balanced power structure without considering fairness concerns, when theRiof firms is lower, the R&D profits have a positive correlation withβiand a negative correlation with ηi. When theRiof firms is higher, the R&D profits are negatively correlated withβiand positively correlated withηi. Thus, Proposition 6(2) is verified.
As shown in Fig. 8, we use the UD scenario as an example, which at this point satisfies RuUDs
Finally, as shown in Fig. 10 and Fig. 11, we analyse the impacts of fairness concerns level Non the total profits of the technology chain and firms’ profits and prices. As shown in Fig. 10, the effort level of firms and the total profits of the technology chain are negatively correlated with N, which means thatNwill reduce a firm's effort levels and the total profits of the technology chain. Furthermore, as shown in Fig. 11(a), the greater the fairness concernNis, the higher the product pricepbased on GPTs determined by firm D is. When firm U is in the leading position, the patent licensing pricewdecreases withN, which shows that firm D has stronger bargaining ability whenNis higher. When firm U is in the following position or a balanced power structure, pricewincreases withN. Moreover, as shown in Fig. 11(b), when firm U (D) is in the leading position, the R&D profits of firm U(D) increase withN, while the profits of firm D(U) first increase and then decrease withN. Therefore, Proposition 7 is verified.
General discussion and implicationsTheoretical implication and conclusionsGPT cooperation R&D has obvious process characteristics. Bresnahan and Trajtenberg (1995) studied semiconductors and their applications in hearing aids, radios, television sets, computers and other products, which can be regarded as part of an earlier literature on the GPT R&D process. Recently, Zheng et al. (2019, 2021) proposed the GPT R&D process framework of “supply→ diffusion (adoption→commercial development→market benefit realization)” in which the interdependent relationship between supply and diffusion is analysed. Based on this, referring to the idea of the supply chain, this study regards the GPT R&D process as a technology chain; that is, the supply of GPTs and diffusion based on GPTs supplied form an interdependent technology link relationship, which expands the research on the process of GPT R&D. In addition, this study introduces the concepts of power structures, fairness concerns, and knowledge spillovers (Ranjbar et al., 2020; Caldas et al., 2021; Niu et al., 2022), which are combined with government support (Tassey, 2005; Zuo et al., 2019) in the same framework to establish GPT cooperation R&D models between firm U and firm D with UD(s), DU(s) and BE(s) scenarios from a technology chain perspective to enrich the relevant research related to GPT cooperation R&D. Furthermore, research on the key influencing factors and mechanisms of GPT cooperation in R&D enables a theoretical understanding of how and why the effective operation of GPT cooperation in R&D is regarded as a technology chain. The following conclusions can be drawn from the aims stated in the introduction based on Propositions 1-7 and numeric analysis:
First, the optimal effort level of firm U and firm D, the ratio of government subsidies, the expected revenue of the government and the total R&D profits of the technology chain are lower when considering fairness concerns. Whether or not fairness concerns are taken into account, the R&D effort level of firms, the ratio of government subsidies, the government's expected revenue and the total profits of the technology chain, which are equal in the UD and DU scenarios when not considering fairness concerns and are higher in the UDs scenario than that in the DUs scenario when considering fairness concerns, are all higher in the BE(s) scenarios than in the UD(s) and DU(s) scenarios. The R&D profits of firms in the leading position are always greater than those of firms in the following position. When the innovation contribution level of firms is lower, the profits of firms in the BE(s) scenario are highest, followed by those in the leading position, and the profits of firms in the following position are lowest. When the innovation contribution level of firms is higher, the R&D profits of firms in the leading position are highest, followed by those in the BE(s) scenarios, and the profits of firms in the following position are lowest.
Second, government support for GPTs can improve the effort level and profits of firms. government subsidies to the effort costs of both firm U and firm D are equal, and the ratio of government subsidies increases with an increase in the price elasticity of demand or (and) a decrease in fairness concerns. For the production innovation return based on GPTs, when not considering fairness concerns, the profits of firms in the leading position increase with it, while the profits of firms in the following position only increase with it when the innovation contribution level of the leading firm is higher. When considering fairness concerns or under the BE(s) scenarios, the profits of firms only increase when the cooperating firm's innovation contribution level is higher; otherwise, it decreases with it. Knowledge spillovers between firm U and firm D and the product innovation return help improve the R&D effort level of firms, the total profits of the technology chain and the expected revenue of the government. However, the effort cost multipliers of firms have the opposite effect.
Third, knowledge spillovers are conducive to improving the profits of firms in any power structure. The firm's effort cost multipliers will reduce profits. When not considering fairness concerns, the R&D profits of firms in the leading position (in the following position) of the technology chain are positively (negatively) correlated with their own knowledge spillovers and are negatively (positively) correlated with their own effort cost multipliers. In the BE scenario, when the relative innovation contribution level of firms is lower (higher), their R&D profits are positively (negatively) correlated with their own knowledge spillovers and negatively (positively) correlated with their own effort cost multipliers. When considering fairness concerns, if the relative innovation contribution level of firms in the leading position is less (greater) than a certain threshold, the R&D profits of firms in the leading position are positively (negatively) correlated with their knowledge spillovers and negatively (positively) correlated with their effort cost multipliers. Otherwise, knowledge spillovers and effort cost multipliers have opposite effects on the R&D profits of firms in the leading and following positions. In the BE scenario, when the relative innovation contribution level of firm U is less (greater) than a certain threshold, the R&D profits of firms are positively (negatively) related to their knowledge spillovers and negatively (positively) related to their effort cost multipliers.
Finally, the price of firm D increases with the level of fairness concerns. The patent licensing price of firm U decreases with fairness concerns when firm U is in the leading position of the technology chain and increases with fairness concerns when firm U is a follower or under BE conditions. In the UD and DU scenarios, the profits of firms in the leading position are always negatively correlated with the fairness concern level, and the profits of firms in the following position first increase and then decrease with the fairness concern level. In the BE scenario, the profits of both firm U and firm D are negatively correlated with the level of fairness concerns. In any GPT R&D scenario, the effort level of firm U and firm D in the technology chain, the total R&D profits of the technology chain, and the expected revenue of the government are all negatively correlated with the fairness concern level.
Implications for practitionersThe above results have important implications for GPT cooperation in R&D. Specifically, the R&D process of GPTs is viewed as a special technology chain in our study, which leads to the following implications for practitioners.
The first implication is that the balanced power structure is preferred to achieve a win‒win situation of GPT cooperation in R&D between firm U and firm D. However, an unbalanced structure encourages firms in the leading position to maximize their own profits, which does not necessarily result in the optimal outcome of the technology chain. Therefore, a balanced power structure should be the dominant mode of GPT cooperation R&D activities. Meanwhile, knowledge spillovers are a key factor in the process of leadership allocation, which can improve the effort level of both firm U and firm D and thus the total profits of the technology chain. To reduce interest conflicts between the individual firm and the entire value chain, which may lead to competition between firm U and firm D for leadership in the technology chain, firm U and firm D should establish an appropriate trust mechanism to promote knowledge sharing between firms in the technology chain under a balanced power structure.
The second implication of our results is that behaviours based on fairness concerns should be given more attention to improve the efficiency of GPT cooperation in R&D in the technology chain. The R&D efficiency and profits of firms with fairness concerns are lower than those of firms without fairness concerns. Therefore, both firm U and firm D in the technology chain should develop a GPT cooperation R&D mode that focuses on overall benefits instead of individual benefits to avoid malignant competition and double marginalization. In addition, fairness concerns can increase the bargaining power of firms in the following position because of an unfair income distribution under the unbalanced power structure, which is emphasized in decision-making. With this competition rather than corporation mindset, the overall R&D efficiency and profits of the technology chain will be lower, regardless of the power structure. Consequently, these findings call for firm U and firm D to build a performance system that extends beyond individual interests and to further embrace the overall interests that GPT cooperation R&D can bring. By doing so, the adverse impacts of fairness concerns in decision-making are mitigated, which allows for better capturing of the value of GPT cooperation in R&D from a technology chain perspective.
Our models also consider support from the government and thus have some implications for policy-makers. It is already known that government support plays a crucial role in R&D projects involving GPTs. Our results reinforce this understanding and further demonstrate that government support policies should be made to adopt different power structures. The aims of government support policies should minimize the negative effects of fairness concerns in the GPT cooperation R&D relationship by, for example, offering R&D subsidies under a balanced power structure to promote GPT cooperation R&D. In addition to subsidies, our study emphasizes that information and knowledge and technology support are necessary in the identification and supply of GPTs, which can compensate for a potential lack of R&D resources and reduce the high R&D risk associated with GPTs. When the price elasticity of demand is higher, the government can share the R&D risk of firms by increasing R&D effort cost subsidies, which is equivalent to granting “approval labels” to products based on GPTs and promoting the smooth development of GPT cooperation in R&D activities.
Limitations and future researchThis study is not free from limitations. As an early attempt at GPT cooperation R&D from the technology chain, future studies could explore more dimensions of GPT cooperation R&D questions based on our model. First, our research results that fairness concerns have a negative impact on the R&D efficiency and profits of firms. However, fairness concerns are an important topic that cannot be avoided in GPT cooperation R&D between firm U and firm D. Therefore, it would be valuable to design a mechanism to minimize these negative impacts, which is neglected in our research. Second, our study also shows that the balanced power structure is a preferred choice for GPT cooperation in R&D considering its benefit of improving the overall performance of the technology chain. However, unbalanced power structures are more common in the technology chain. Thus, more work needs to be done to establish an optimization mechanism to make the profits of firm U and firm D close or equal to those of balanced power structures, which is a direction of further in-depth study based on our model. Third, it will also be a significant research topic in theory and practice to consider the horizontal synergy of upstream or (and) downstream links and vertical collaboration from upstream to downstream in the technology chain to explore the sustainable cooperation R&D mechanism of GPTs, which is another research topic that needs to be studied.
This work was supported by the Chongqing Natural Science Foundation (Basic Research and Frontier Exploration Special Project) Surface Project (cstc2019jcyj-msxmX0112), China Postdoctoral Science Foundation Funded Project (2020M673154), Chongqing Graduate Innovation Project (CYS21387), Graduate Innovation Project of Chongqing Technology and Business University (yjscxx2021-112-19,yjscxx2021-112-76) and The Deanship of Scientific Research (DSR) at King Abdulaziz University (KAU), Jeddah, Saudi Arabia, has funded this project, under grant no. (RG-6-611-43).
Proof of optimal solutions of the UD scenario: Satisfied with a feedback Nash equilibrium, we first obtain the optimal market price set by firm D by solving the first-order condition forp; asπd is concave inp, we let∂πd∂p=0, obtainp(w)=D0+α(eu+ed)+b(1−λ)w+bcd−bβueu2b, and replacep(w) in Eq. (1), as πu is concave in w, and we let ∂πu∂w=0. We then obtain
By replacing w inp(w), we obtain the following:
We replace w and p in πT and let ∂πT∂eu=0 and ∂πT∂ed=0; then, we obtain the following:
where Ru=(α+bβu)2bηu(1−θu), Rd=(α+bβd)2b(1−θd). We replace the optimal eu and ed in πG, and we solve ∂πG∂θu=0 and ∂πG∂θd=0 for an optimal θuUD and θdUD, i.e., θuUD=θdUD=b6+b.We replace θuUD=θdUD=b6+b with eu and ed, we can obtain the optimal eu and ed under the UD scenario:
where RuUD=(α+bβu)2(6+b)6bηu, RdUD=(α+bβd)2(6+b)6bηd.We replace (A4) and (A5) with (A1) and (A2), we can obtain the optimal price strategies of firm U and firm D:
We replace euUD,edUD,wUDandpUDin Eqs. (1)-(4) and obtain the optimal profits πiUD(i=u,d,T,G)under the UD scenario.
Proof of the optimal solutions of the DU scenario:
p=(1−λ)w+m0 and we replace p=(1−λ)w+m0 in Eq. (1). We solve ∂πu∂w=0 to obtain w(p)=D0−bp+α(eu+ed)+b(1−g)cu−bβdedb(1−λ), replace w(p) in Eq. (2) and obtain p by solving ∂πd∂p=0:
We replace Eq. (A7) inw(p) to obtain the following:
We replace (A7) in Eq. (4) and let ∂πT∂eu=0and ∂πT∂ed=0 to obtain the following:
where Ru=(α+bβu)2bηu(1−θu) andRd=(α+bβd)2b(1−θd) and we replace eu and ed above in Eq. (3) to solve the optimal government subsidies; we let ∂πG∂θu=0and ∂πG∂θd=0, and we obtain the optimal solutions:We replace (A9) in euand ed above and obtain the following euDU and edDU:
where RuDU=(α+bβu)2(6+b)6bηu and RdDU=(α+bβd)2(6+b)6bηd.We replace euDUand edDU in Eqs. (A7) and (A8) and obtain the optimal price strategies of firm U and firm D:
We replace euDU,edDU,wDUandpDUin Eqs. (1)-(4) and obtain the optimal profits πiDU(i=u,d,T,G)under the DU scenario.
Proof of the optimal solutions of the DU scenario: Satisfied with a feedback Nash equilibrium, we first solve the first-order optimal condition for w andp. Note that πu is concave in wand πd is concave in p. Let ∂πu∂w=0and ∂πd∂p=0.
By replacing w and pin Eq. (4) and solving the first-order optimality condition for eu and ed, we obtain
where Ru=(α+bβu)2bηu(1−θu)andRd=(α+bβd)2b(1−θd). By replacing eu and ed in Eq. (3) and letting ∂πG∂θu=0 and ∂πG∂θd=0, we obtain the optimal solutions:By replacing (A16) with eu and ed, we can obtain the following:
whereRuBE=(α+bβu)2(4+b)4bηu and RdDU=(α+bβd)2(4+b)4bηd.By replacing Eqs. (A17) and (A18) in Eqs. (A14) and (A15), we can obtain the optimal price strategies of firm U and firm D:
By replacing euBE, edBE, wBE and pBE in Eqs. (1)-(4), we can obtain the optimal profits πiBE(i=u,d,T,G) under the BE scenario. It should be noted that the optimal solutions under the UD, DU and BE scenarios are similar to those under the UD, DU and BE scenarios, so they are not repeated here.
Proposition 1 We first compare the scenarios considering and not considering fairness concerns, taking the UD and UD scenarios as examples. From the optimal solutions of different scenarios, we can obtain the following:
Since 8(2(3+2N)+b)<2(2+N)2(6+b), we find that euUDseuUD=edUDsedUD<1; similarly, the following hold:
Therefore, we obtain euUDs<euUD, edUDs<edUD, πGUDs<πGUD and πTUDs<πTUD; thus, we find that under the UD scenario, the effort level and profits of firms and government revenue with fairness concerns are lower than those without fairness concerns. The proof of conclusions for the DU(s) and BE(s) scenarios are similar to those above; hence, we omit the details for brevity.
Proposition 2 We first compare government subsidies and expected outcomes, firms’ effort levels and the profits of the technology chain under different power structures when not considering fairness concerns. Furthermore, we can easily obtainθiUD=θiDU=b6+b<θiBE=b4+b(i∈{u,d}) fromEq. (A9), which shows that the ratio of government subsidies is equal under the unbalanced power structure and lower than that under the balanced power structure.
Then, we compare effort levels. From the optimal effort solutions, we obtain euUD=euDU, edUD=edDUandeuBEeuUD=16(4+b)−6(4+b)RuUD−6(4+b)RdUD9(6+b)−6(4+b)RuUD−6(4+b)RdUD. Since 16(4+b)>9(6+b), we obtain euBEeuUD>1. Similarly, edBEedUD>1, so we have euBE>euUD=euDU,edBE>edUD=edDU.
Then, we compare the expected revenue of the government and the profits of the technology chain. We obtain πGUD=πGDU, πGBEπGUD=16(4+b)−6(4+b)RuUD−6(4+b)RdUD9(6+b)−6(4+b)RuUD−6(4+b)RdUD>1. Thus, we have πGBE>πGUD=πGDU. Similarly, πTBE>πTUD=πTDU.
The proof when considering fairness concerns is similar to that above; hence, we omit the associated details for brevity.
Proposition 3 We first compare the profits of firms under different power structures when not considering fairness concerns. From the optimal profits, we haveπuUDπuDU=16−9RuUD8−9RuUD>1,πdUDπdDU=8−9RdUD16−9RdUD<1, and thenπuUD>πuDU,πdDU>πdUD. Similarly,πuBE>πuDU,πdBE>πdUD.
Upon observing the optimal solutions of the R&D profits of firm U and firm D, we can see that the R&D profits of firms are mainly affected by the relative innovation contribution level of firms. Therefore, we focus on proving the impacts of firms’ innovation contribution levels on the comparison of profits under different power structures. As ∂πuUD∂RuUD>0, ∂πuUD∂RdUD>0, ∂πuBE∂RuBE<0 and ∂πuBE∂RdBE>0, we conclude that the R&D profits of firm U in the BE scenario decrease with an increase in its own innovation contribution level and increase with an increase in the innovation contribution level of firm D. In the UD scenario, profits increase with an increase in the innovation contribution level of both firm U and firm D. Therefore, with an increase in firm U's relative innovation contribution level, its profits in the UD scenario are higher than those in the BE scenario. Hence, Proposition 3 is proved.
Proposition 4 From the optimal solutions, we obtain∂euUD∂g=3RuUDbcu(α+bβu)(8−3RuUD−3RdUD)>0. Similarly,∂eik(s)∂g>0,∂πik(s)∂g>0,∂πTk(s)∂g>0, and∂πGk(s)∂g>0. Therefore, the government's knowledge and technology support in the identification and supply link of GPTs can effectively improve the efficiency of GPT cooperation in R&D as well as the profits of all parties. As∂θuUD∂b=6(6+b)2>0,∂θuUDs∂N=−4b(2(3+2N)+b)2<0,∂θik(s)∂b>0,∂θiks∂N<0, wherei∈{u,d},k∈{UD,DU,BE}. Therefore, the ratio of government subsidies to the level of R&D effort is positively correlated with demand price elasticity and negatively correlated with the level of fairness concerns. Therefore,Proposition 4is proved.
Proposition 5 Taking the impacts of knowledge spillovers in the UD scenario as an example, as∂euUD∂βu=(D0−b(1−g)cu−bcd)(b(6+b)(8−3RuUD−3RdUD)+6b(α+bβu)2(6+b)2)2bηu6bηu(8−3RuUD−3RdUD)2>0,∂euUD∂βd=(D0−b(1−g)cu−bcd)(α+bβd)(6+b)2bηuηd(8−3RuUD−3RdUD)2>0, similarly,∂edUD∂βd>0,∂edUD∂βu>0. Therefore, the firm's effort level is positively correlated with knowledge spillovers. As∂πTUD∂βu=3(D0−b(1−g)cu−bcd)2(α+bβd)(6+b)2bηu(8−3RuUD−3RdUD)2>0, similarly,∂πTUD∂βd>0,∂πGUD∂βu>0,∂πGUD∂βd>0. Therefore, the total profits of the technology chain and government revenue are positively correlated with knowledge spillovers. In other scenarios, the dependant variables are also positively correlated with knowledge spillovers. The proof of the impacts of effort cost multipliers and product innovation return based on GPTs are similar to those above, and we omit the associated details for brevity. Hence,Proposition 5is proved.
Proposition 6 As in the proof ofProposition 5, we use the UD scenario as an example.
As ∂πuUD∂βd=(D0−b(1−g)cu−bcd)2(α+bβd)(6+b)(16−9RuUD)bηd(8−3RuUD−3RdUD)3>0, ∂πdUD∂βu=(D0−b(1−g)cu−bcd)2(α+bβu)(8−9RdUD)bηu(8−3RuUD−3RdUD)3>0, ∂πuUD∂ηd=−(D0−b(1−g)cu−bcd)2(α+bβd)2(6+b)(16−9RuUD)2b2ηd2(8−3RuUD−3RdUD)3<0, ∂πdUD∂ηu=−(D0−b(1−g)cu−bcd)2(α+bβu)2(6+b)(8−9RdUD)2b2ηu2(8−3RuUD−3RdUD)3<0, similarly, ∂πi∂βj>0,∂πi∂ηj<0. Therefore, firm knowledge spillovers βi are conducive to improving firm profits, i.e., πjk. In contrast, a firm's effort cost multipliersηiwill reduce the profits of the cooperating firm πjk. Hence, Proposition 6(1) is proved.
When not considering fairness concerns, as∂πuUD∂βu=(D0−b(1−g)cu−bcd)2(α+bβu)(6+b)(8−9RuUD+9RdUD)2bηu(8−3RuUD−3RdUD)3>0,∂πuUD∂ηu=−(D0−b(1−g)cu−bcd)2(α+bβu)2(6+b)(8−9RuUD+9RdUD)2b2ηu2(8−3RuUD−3RdUD)3<0, similarly, ∂πdUD∂βd<0,∂πdUD∂ηd>0,∂πuDU∂βu<0,∂πuDU∂ηu>0,∂πdDU∂βd>0,∂πdDU∂ηd<0. Thus, in the DU and UD scenarios, the R&D profits of firms in the leading position are positively correlated with their own knowledge spillovers, and the R&D profits of firms in the following position are negatively correlated with their own knowledge spillovers. As ∂πuBE∂βu=[D0−b(1−g)cu−bcd]2(α+bβu)(4+b)16(Rd−Ru)bηu(9−4Ru−4Rd)3, i.e., when Rd>Ru, ∂πuBE∂βu>0, when Rd<Ru, ∂πuBE∂βu<0. Therefore, in the BE scenario, when the relative innovation contribution level of the cooperating firm is higher, the R&D profits of the firm are positively correlated with its knowledge spillovers; when its relative innovation contribution level is lower, the R&D profits of the firm are negatively correlated with its knowledge spillover. Thus, proposition 6(2) is proved.
When considering fairness concerns, in UD and DU scenarios, taking the UD scenario as an example, ∂πuUDs∂βu=(D0−b(1−g)cu−bcd)2(α+bβu)(2(3+2N)+b)2bηu(2(2+N)2−(3+2N)RuUDs−(3+2N)RdUDs)3×(2(2+N)21+2N−(3+2N)2(RuUDs−RdUDs)), ∂πuUDs∂ηu=−(D0−b(1−g)cu−bcd)2(α+bβu)(2(3+2N)+b)4b2ηu2(2(2+N)2−(3+2N)RuUDs−(3+2N)RdUDs)3×(2(2+N)2(1+2N)−(3+2N)2(RuUDs−RdUDs)). Therefore, whenRuUDs<RdUDs+2(2+N)2(1+2N)(3+2N)2, ∂πuUDs∂βu>0,∂πuUDs∂ηu<0. Similarly, whenRuUDs<RdUDs+2(2+N)2(1+2N)(3+2N)2, ∂πdUDs∂βd<0, ∂πdUDs∂ηd>0. WhenRdDUs<RuDUs+8(1+N)2(1+2N)(3+4N)2, ∂πdDUs∂βd>0,∂πdDUs∂ηd<0, ∂πuDUs∂βu<0, ∂πuDUs∂ηu>0. Therefore, when considering fairness concerns, if the relative innovation contribution level of firms in the leading position is lower than a certain threshold, the R&D profits of firms in the leading position (in the following position) of the technology chain are positively (negatively) correlated with their knowledge spillovers and negatively (positively) correlated with their effort cost multipliers. Similarly, in the BE scenario,
Therefore, whenRuBEs−RdBEs<9N(1+N)22(1+2N)(2+3N)2,∂πuBEs∂βu>0,∂πuBEs∂ηu<0. Similarly, whenRuBEs<RdBEs+9N(1+N)22(1+2N)(2+3N)2,∂πdBEs∂βd<0,∂πdBEs∂ηd>0; when RuBEs>RdBEs+9N(1+N)22(1+2N)(2+3N)2, ∂πuBEs∂βu<0, ∂πuBEs∂ηu>0, ∂πdBEs∂βd>0, ∂πdBEs∂ηd<0. As a result, when the relative innovation contribution level of firm U is lower than a certain threshold, the R&D profits of the firm are positively related to its knowledge spillovers and negatively related to the effort cost multiplier. In contrast, the R&D profits of the following firms are negatively correlated with their knowledge spillovers and positively correlated with the effort cost multiplier. Proposition 6(3) is proved.
When not considering fairness concerns, we prove the impacts of the product innovation return αon the profits of firms by taking the UD scenario as an example, as ∂πuUD∂α=[(D0−b(1−g)cu−bcd)2b(8−3RuUD−3RdUD)3×((α+bβu)(6+b)2bηu(8−9(RuUD−RuUD))+(α+bβd)(6+b)bηd(16−9RuUD))]>0,
∂πuUD∂α=[(D0−b(1−g)cu−bcd)2b(8−3RuUD−3RdUD)3×(8A−4B−(9A+92B)RdUD+92BRuUD)], where A=(α+bβu)(6+b)bηu, B=(α+bβd)(6+b)bηd. Therefore, the profits of firms in the leading position increase withα, while the profits of firms in the following position only increase with the production innovation returnαwhen the innovation contribution level RuUDof the leading firm is higher, i.e., ∂πuUD∂α>0. Similarly, when considering fairness concerns under BE(s) scenarios, the profits of firms only increase withαwhen the cooperating firm's innovation contribution level is higher; otherwise, they decrease with α, i.e., ∂πj∂α>0, ∂πi∂α<0. Thus, Proposition 6(4) is proved.
Proposition 7 This proof is similar to the proofs ofPropositions 4-6. Hence, we omit the associated details for brevity.