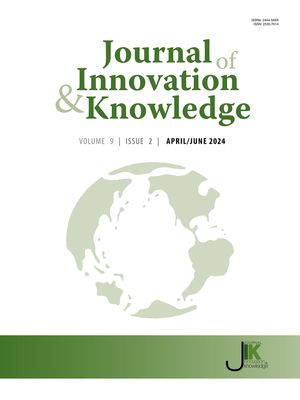
Editado por: Sascha Kraus, Alberto Ferraris, Alberto Bertello
Más datosThis study aims to explore the impact of the implementation of artificial intelligence (AI) in the healthcare sector on overall human resource (HR) practices and organizational performance. We seek to highlight the added value that adopting AI techniques in human resource management (HRM) in the healthcare sector offers to the entire ecosystem in financial and organizational terms. To tackle the research question, we conducted an explorative qualitative analysis investigating a Lebanese international healthcare center in Dubai. To obtain data triangulation, we used both primary and secondary data as a source of evidence. The study offers interesting insights into implementing AI tools in HRM across the healthcare sector and to what extent this will contribute a useful tool to gather better organizational performance. Despite the unique characteristics of the case study in the industry and country settings, this study is not without limitations. It is difficult to extend the results to the entire population. This study provides several theoretical, managerial, and policy implications that give concrete insights into how implementing AI will affect HRM processes and company performance.
The phenomenon of digital transformation, which is defined as "a process aimed at improving an entity by triggering major changes to its attributes through combinations of information, processing, communication, and networking technologies," has an impact on many elements of businesses (Verhoef et al., 2021; Vial, 2021). Healthcare is one of the key industries where this phenomenon has taken root, thereby generating new business opportunities and revolutionizing established paradigms (Kraus et al., 2021). The healthcare emergence triggered by COVID-19 underlined the critical importance of healthcare institutions attaining a high degree of digital maturity (Fletcher & Griffiths, 2020; Kraus et al., 2021). Healthcare facilities have had to respond to the need to ensure continuity of care despite physical distance between doctor and patient, as well as to streamline the flow of patients within the hospital, guaranteeing an effective and efficient service to the large pool of patients treated (Ting et al., 2020).
In such an emergency, the healthcare ecosystem was called upon to modify and improve its performance to maintain high-quality standards of care by rationalizing and allocating scarce resources (Khalifa & Zabani, 2016), managing HR, and monitoring their performance, all of which proved to be essential (Cuccurullo & Pennarola, 2017). Therefore, digitalization with the development of sophisticated technologies, such as AI, significantly impacts the labor market and how employees are managed (Frey & Osborne, 2017). While human judgment and management of HR still heavily rely on human input, businesses are increasingly digitizing more of these processes and integrating AI (Köchling et al., 2022). Such technologies provide the opportunity to achieve high levels of efficiency in managing and screening candidates or reduce the time to hire and overall recruitment costs (Woods et al., 2020).
HRM plays a crucial role in competitive challenges, particularly in the healthcare sector (Olan et al., 2022). The four dimensions through which AI is categorized (mechanical, analytical, empathetic, and intuitive) make this technology a valuable tool to support organizations (Huang & Rust, 2018) by providing a vast amount of data and automating many steps of business processes to speed up and optimize all phases of HRM (Frey & Osborne, 2017Neufeind et al., 2018; Tambe et al., 2019; Bresciani et al., 2021a; 2021b). Despite several studies on the implementation of AI in healthcare (Jiang et al., 2017; Davenport & Ravi, 2019; Rong et al., 2020; Manne & Sneha, 2021), there persists a gap in the literature regarding the relationship between using such technology and employee performance. In this vein, the research question concerns how implementing digital solutions based on AI can impact HR performance within healthcare facilities.
This study aims to explore the topic of HRM in healthcare and employee performance. Specifically, this study seeks to determine the potential benefits of implementing innovative and digital solutions in healthcare institutions and the impact of these technologies on HR performance. To highlight the relevance of AI implementation, we refer to the “theory of resistance” (Ram, 1987; Zaltman & Wallendorf, 1983; Kaur et al., 2020; Talwar et al., 2020; Khalil et al., 2022). Reluctance in employees may hinder the implementation of digital solutions. Our analysis aims to emphasize that the AI benefits outweigh the costs and that its implementation necessary to optimize performance.
The novelty of this study is to observe a phenomenon from a different perspective to unveil nuances. To tackle the research question, this paper used an exploratory approach based on an investigation of the Clemenceau Medical Center (CMC) in Dubai, one of the top private hospitals in the United Arab Emirates. Its excellent patient-centered approach has led Johns Hopkins Medicine International (JHMI) to select the CMC as one of its global affiliate medical institutions. It was feasible to acquire results that witness AI's critical role in HR performance based on the study of primary and secondary data.
From a theoretical point of view, this study provides new insights into the transformation and digitalization of HRM practices. From a managerial perspective, it offers healthcare managers a valuable tool to innovate their business models and HRM processes. It also highlights the need for policymakers to promote the implementation of digital healthcare to improve overall performance and achieve the highly desired balance between providing quality care and minimizing costs. This article is structured as follows. In Section 2, we analyze the role of AI in HRM and performance evaluation. Section 3 describes the methodology used, the data collection, and the different stages of analysis. In Section 4, we analyze the results and in Section 5, we discuss them thoroughly. Additionally, we have also highlighted some implications for academics, practitioners, and policymakers. Finally, we discussed the limitations of the analysis conducted and proposed various ideas for future research.
Theoretical backgroundHuman resource management and digitalizationDigitilization offers the possibility of understanding how information or digital technology can be applied to modify current company operations. (Verhoef et al., 2021; Mostaghel et al., 2022). The massive expansion of digital power is also changing the labor market. (Neufeind et al., 2018). As the “digital” phenomenon spreads, companies are pushed to innovate their business models to exploit new opportunities. To optimize performance and base growth on digitalization, companies must be open to knowledge exchange and collaboration, which implies rethinking work and HRM processes (Bouncken et al., 2021).
The creation of more hybrid skill sets, a decrease in repetitive activities, and a reduction in workplace risks are just a few effects that result from the development of new technologies, all of which have a significant impact on employment (Frey & Osborne, 2017; Neufeind et al., 2018) and on how HR is managed within an organization (Tambe et al., 2019; Bresciani et al., 2021a; 2021b). HRM is "the acquisition and retention of new skills, capacities, and competencies in an organization through its personnel through the use of various management strategies" (Tambe et al., 2019). The adoption of digital technologies may impact both recruitment management procedures and HRM. AI allows companies to optimize information sharing to manage HR risk. As Kumar et al. (2022) stated, “intelligent HR management leads to increased economic growth.”
These technologies can hold and process a vast amount of data. As a result, HR managers can identify recruitment trends and automatically choose the best candidates (Hamilton et al., 2020). They offer the possibility of making all phases of the production “funnel” more efficient. By analyzing a large amount of data, digital solutions represent valuable tools to predict consumer needs, build highly customized offers, and optimize after-sales services (Paschen et al., 2020). They also provide the opportunity to determine how to raise employee knowledge and abilities to create a competitive advantage, and enhance the performance of the business (Olan et al., 2022). When the practices in the HRM process align with a business's competitive strategy, people can represent a valuable and nonreplicable resource for the firm. As a result, individuals are a vital source of benefit for an organization challenging competitors to match (Amarakoon et al., 2018).
In this vein, performance evaluation is crucial (Sardi et al., 2020). Indeed, performance appraisal is one of the essential practices in the HRM process regarding employee behavior aligned with corporate goals (DeNisi & Murphy, 2017). A performance evaluation may signal to staff members the value of their inventive actions and accomplishments, particularly in a world that is increasingly digital. Studies that emphasize electronic performance management systems show how employee behavior and efficiency are impacted by digital assessment (Yin & Qin, 2019; Kamble et al., 2020; Frederico et al., 2020). Others stress the significance of focusing on objective outputs and excellent staff development in a digital setting to assist employees in meeting the competence standards of digital work (Nijp et al., 2016).
As previously mentioned, digitization has led to the adoption of “new ways of working,” pushing companies to promote collaboration, exchange ideas, and decouple work from the business environment itself. As Gerards et al. (2021) affirm, this leads employees to engage in intrapreneurial behavior, ensuring high levels of individual performance for the organization and resulting in improved overall performance. Companies must develop the capacity to react more swiftly to rival actions in a dynamic digital environment. As a result, standard linear management and performance measurement systems must also be transformed. Mainly, they must be created to be used in highly dynamic circumstances with consideration to requirements in a digital environment (Sahlin & Angelis, 2019). Therefore, it is essential to establish a performance measuring system that considers technological changes, the business environment, and organizational procedures. This notion is based on the understanding that numerous performance measurement functions must be established through technical advancements. (Yin & Qin, 2019; Kamble et al., 2020; Frederico et al., 2020). With the rise of digitalization, the conventional idea that corporate strategies shape technologies needs to be rethought entirely as digital technologies increasingly influence corporate strategy (Li et al., 2021) and organizational contexts.
Artificial intelligence in performance measurementOrganizational performance has always significantly impacted business decisions (Samson & Bhanugopan, 2022). The specificities of a firm influence a company's performance, making an unambiguous description of a "performance measuring system" impossible (Shane, 2000). This concept can be examined from various perspectives. From an operational point of view, a performance measurement system is a "collection of metrics used to assess both the efficiency and efficacy of actions." and can also be used to give staff feedback. From a strategic standpoint, it reflects the organization's methods of implementing the strategy (Horváth et al., 2019).
The ability of this tool to strike a balance between economic performance, growth and control, and opportunity underlies the significance of performance monitoring and measuring systems, allowing managers to meet predetermined corporate objectives (Simons, 2000). It is necessary to create a control system since the company is a dynamic enterprise that operates in a continually changing environment (Bititci et al., 2018). In the digital context, firms must face various complex changes that underline the need to achieve a valid performance monitoring system (F. Schiavone et al., 2022).
In this light, cutting-edge technologies, such as those based on Industry 4.0 and AI, may come into play (Yin & Qin, 2019; Kamble et al., 2020; Frederico et al., 2020). AI is "the capacity of a digital machine or computer-controlled robot to carry out functions typically associated with intelligent beings" (Plastino & Purdy, 2018; Haenlein & Kaplan, 2019; Copeland, 2020). AI technologies enable companies to create more value for their customers by applying a proactive, uncertainty-management approach, thereby improving cost efficiency and increasing revenues (Åström et al., 2022; Kumar et al., 2022). At the same time, their disruptive nature pushes companies to rethink and adapt their business models (Bouncken et al., 2021; Trischler & Ying, 2022).
One of the main barriers to the use of such technologies is HR. As Khalil et al. (2022) stated, people often adopt behaviors of resistance to technological change. The theory of resistance is widely addressed in the literature (Zaltman & Wallendorf, 1983; Ram, 1987; Kaur et al., 2020; Talwar et al., 2020; Khalil et al., 2022). "Resistance to change may be defined as any conduct that serves to maintain status quo in the face of pressure to alter the status quo" (Zaltman & Wallendorf, 1983) and is “associated with the degree to which individuals feel threatened by change” (Ram, 1987). Employees may be afraid of being replaced by AI. They could be reluctant to adopt these technologies in their day-to-day work (Huang & Rust, 2018). Additionally, lack of technological and digital skills may lead them to refuse to use AI solutions altogether (Du et al., 2019). Employee resistance may also be due to a lack of perception of the value of the new technology (Jebarajakirthy & Shankar, 2021).
AI technologies can improve several necessary performance measures. Accelerating processing decreases bottlenecks and boosts overall operational effectiveness (Akter et al., 2020; Rana et al., 2022). Finally, AI enables better decision-making by extending the range of knowledge that top management and other essential decision-makers typically access, especially information that would otherwise be impossible to locate (Lindebaum et al., 2020; Araujo et al., 2020; Buçinca et al., 2021; Kumar et al., 2022). AI makes it possible to make decisions based on a deeper understanding of management dynamics by making it easier to manage unstructured information and build sophisticated decision-making models (Martins et al., 2020).
Such data can significantly impact performance results, allowing organizations to cut costs, extend their goods and service offerings, and target customers with more individualized offers (Wamba-Taguimdje et al., 2020; Mikalef & Gupta, 2021; Kumar et al., 2022). Digitalization has firmly established itself in a complicated sector such as healthcare (Kraus et al., 2021). The emergence of COVID-19 has pushed many organizations to achieve digital maturity (Kraus et al., 2020) and has underlined the need for firms to adjust swiftly to external events and thereby gain organizational flexibility (Fletcher et al., 2020). Using AI in healthcare enables cocreation processes to occur. Implementing new technologies allows healthcare facilities to adopt a patient-based approach and build personalized care pathways, ensuring high-quality care (Leone et al., 2021).
Strategic performance in healthcare can be defined as the attempt “to achieve medical effectiveness and appropriateness of services, avoid or reduce inequalities in access to services, ensure job satisfaction for professionals and appropriate treatment for patients” (Prager et al., 2018; Baeten et al., 2018; Bombard et al., 2018). Performance evaluation is an essential component of enhancing the efficiency of care delivery services (Agarwal et al., 2019). Given the complexities of healthcare systems, this is a significant challenge. Indeed, healthcare performance is multidimensional as it results from the efforts of several organizations and people (Barbazza et al., 2021). The endeavor to analyze health performance extensively using general indicators has resulted in the emergence of many indicators, emphasizing the importance of developing an effective performance monitoring system (F. Schiavone et al., 2022). The high predictive potential of AI could make this technology a valuable tool for evaluating and improving healthcare performance in terms of knowledge management of healthcare workers, cost reduction, and optimization of care (Yu et al., 2018).
MethodologyTo tackle the research question, we conducted a qualitative analysis using the case study methodology (Eisenhardt, 1989) investigating the Clemenceau Medical Center in Dubai. The CMC in Dubai is a tertiary, state-of-the-art facility designed to offer the city one of the most innovative treatments and technologies available in the region. Medical professionals worldwide come together to deliver a great patient experience driven by passion, experience, and expertise. The hospital is passionate about its mission to provide healthcare services that go above and beyond anything that patients have ever experienced. From medical innovation to outpatient care, the legacy of excellence shows that the CMC is committed to bringing a new standard of excellence for patient-first healthcare to the region. The hospital knowledge transfer matrix further enables us to tap into top-tier medical professionals worldwide, ensuring that patients have access to the best care from start to finish.
The hospital caters to all services on the market, including but not limited to cardiology, radiology, laboratory and pathology, and minor and complicated surgeries. Despite being new in Dubai, the hospital managed to position itself in a very reputable position according to patients and physicians. Most top-notch physicians in Dubai operate part-time or full-time in CMC Dubai. In such a modern and revolutionary hospital, it is urgent to implement AI tools in the human resources management system to contribute to the overall hospital performance and create an environment of trust and engagement for current and future employees. The objective is to ensure that all hospital functions, clinical and administrative, benefit from AI techniques and work in harmony.
We used deliberate and theoretical sampling to search for a case rich in manifestations of our fundamental theoretical notions (Yin, 2009). Our goal is to represent the complexity of social behavior by developing concepts and meaningful descriptions that are neither unduly simplistic nor prejudiced. To guarantee data triangulation and reinforce our analysis, we used multiple sources of evidence, such as semistructured interviews, archival data, industry reports, newspaper and magazine articles, and internal documents (Yin, 2009). Data collection and analysis were conducted in the second semester of 2022. We prepared an interview guide to ensure the same primary lines of inquiry (Bäckström et al., 2018; Cao & Henderson, 2021). Table 1 presents information about the respondents.
List of interviewees.
The interviewer transcribed each interview in the same week it occurred. A second researcher checked all interview transcripts by listening to recordings. Since our research addresses the impact of AI tools on human resource performance, we gathered our data from multiple sources, primarily focusing on the human resources department, which includes different functions that will all be impacted by AI tools. We conducted our interviews with the human resources director, compensation and benefits manager, talent acquisition manager, onboarding specialist, and government relations officer to clearly understand how AI tools would impact their scope of work and overall performance from a financial, productivity, and employee engagement perspective.
To tackle the impact of AI from a clinical aspect, we conducted interviewed the director of the pharmacy, who thoroughly discussed how implementing AI tools will affect the medication management system in terms of medication errors, clinical practice, and the recruitment of pharmacists and talented technicians. Our post-interview coding activities are shown in the table above. Having one expert healthcare researcher and one healthcare worker made our access to data more accessible and simplified interviews with people in high positions in both HR and pharmacy.
In the second phase, we analyzed the interview transcripts following an iterative process with two main steps. Specifically, we chronologically discussed the status of the HR department in CMC before the introduction and development of AI tools at CMC DUBAI, focusing on understanding the situation of multiple HR and organizational functions before AI, what was the idea behind introducing it in the market, what HR and the company are anticipating from this implementation, and how it will create a positive impact on future hospital organizational performance. We attempted to explore how and in which area the implementation of AI tools will significantly help the overall growth of the CMC and HR practices specifically. We translated this analysis to create the case description in the following section. Using an abductive approach, we investigated the case by extrapolating from our data beyond the informants' answers and our theoretical findings. Our examinations' primary goal was to establish a connection between academic knowledge and empirical findings.
FindingsFollowing several in-depth interviews at the CMC, two important HRM parameters that AI-based technologies have impacted emerged. The relevant quotations from the interviews are included in Table 2. In particular, the findings allow us to highlight some relevant outcomes that can improve HR performance and the firm's competitive position in either: a) financial outcome, and/or b) organizational outcome (i.e., employee engagement and optimization of tasks). First, innovative digital technologies enable hospitals to achieve better HRM processes, thereby increasing productivity and the quality of results. Second, digital innovation also affords healthcare facilities better financial performance to guarantee the right balance between cost and quality of care. Despite the high investments needed, AI is a valuable tool to enable firms to gain a competitive position. Hiring more qualified HR staff and making all phases of the process faster enable healthcare facilities to perform better, which translates into greater competitiveness.
Representative quotes.
Respondent | Quote | Financial outcome | Organizational outcome | Key work |
---|---|---|---|---|
Director of Pharmacy | Hiring well experienced people in AI is difficult since its implementation is still new in the market, for instance to hire a pharmacist with experience in automation you need to be very lucky, those talents are very few and very difficult to be recruited due to their highest packages and their company's retention policies | Decrease in manpower | Increased productivity | Agarwal et al., 2019; Bombard et al., 2018; F. Schiavone et al., 2022 |
Director of Pharmacy | If perfectly practiced, AI tool s it would have a huge impact on manpower, overtime, staff rotations and reducing tasks | Decrease in headcount | Staff satisfaction | Bresciani et al., 2021a; Frey & Osborne 2017; Leone et al., 2021 |
Director of Human Resources | “One automated system working on AI algorithm would save at least 6 h of daily CV screening and 1 personnel dedicated to this job | Cost reduction | Time management | Kamble et al., 2020; Kraus et al., 2020; Hamilton & Sodeman, 2020; Kumar et al., 2022 |
Government relations officer | Before implementing of AI tools in government relations and digital portals, we used to stay in the queue for at least 3 h every day to get the employee residency card, job permit, family card and insurance card however we went digital and start linking our portals to government AI algorithms I am barely visiting the ministry of labor, everything can be sorted out online, delivery is automated, our job is much more easier and the employee is highly satisfied. | – | Employee engagement, increased efficiency | Sardi et al., 2020; Frey & Osborne, 2017; Gerards et al., 2021 |
Director of Human Resources | “AI will reflect on the operational aspects in HR and not on the strategic part that involves creativity and innovation. For example, Operational work such as attendance, screening, onboarding and any other administrative work will be almost eliminated | Cost reduction | Optimization of tasks, eliminate redundancy | Martins et al., 2020; Bouncken et al., 2021; Bresciani et al., 2021b; Kumar et al., 2022 |
Director of Human Resources | Before AI implementation we used to combine multiple efforts in order to hire for a specific position, for example we want a marketing director with 10 years of experience working in very decent hospitals and leading specific projects and campaigns related to health care, the amount of applicants we used to receive was beyond logic, now with the implementation of the talent acquisition management AI portal which will immediately eliminate all non-compliant resumes from the pool of applicants our job is becoming much more interesting whereby we are able to select the top notch candidate from a very few shortlisted ones | Cost containment | Proficiency in head hunting, attracting skilled staff | Paschen et al., 2021; Samson & Bhanugopan, 2022; Horváth & Szabó, 2019 |
Compensation and benefits lead | Payroll represents the most challenging, critical and hectic task for HR, from matching the attendance, to verifying justified and unjustified sick leaves, to checking physicians special remuneration formulas, back in 2018, preparation of payroll will start from 21st of the month called as the cut of date and it will finish by the 27th which means practically last week of each month is dedicated to payroll management, recently after the acquisition of the INFOR ERP system working on AI algorithms our life has changed upside down, payroll and all activities related form absence management to remunerations can be finished in the less than 1 working day and can be sent to finance for execution | Reducing manpower | Automation and optimization of tasks, increased productivity and efficiency | Kamble et al., 2020; Kraus et al., 2020; Hamilton & Sodeman, 2020 |
Onboarding specialist | We used to spend hours between hotels, travel agencies and transportation to pursue the onboarding of one employee, the risk of error was high since everything was done manually, now with AI tools since is automated with zero risk of error | Cost reduction, manpower reduction | Eliminating errors, optimization of tasks | Agarwal et al., 2019; Bombard et al., 2018; F. Schiavone et al., 2022; Kumar et al., 2022 |
Finance Manager | Investing in an automated ERP system run by AI algorithms would cost you around 5 M AED however while calculating the impact on productivity, manpower volume, work optimization and elimination of redundant tasks your Return on investment is desirable | Better financial performance, desirable return on investment | Increased productivity, efficiency, employee engagement | Kraus et al., 2020; Bombard et al., 2018; Samson & Bhanugopan, 2022 |
Director of Human resources | “Once the employee gets hired, orientation and induction sessions will be created by the usage of VR techniques for instance, the employee will get to know each department, and anything related to the company by just using the VR technique | Cost reduction | Staff education, time management | Köchling et al., 2022; Li et al., 2021; Martins et al., 2021 |
Director of human resources | “The artificial intelligence will help us as HR department to over towards strategic work instead of perofrming routine work” | Strategic planning | Mostaghel et al., 2022; Neufeind et al., 2018; Nijp et al., 2016 | |
Head of talent acquisition | “I can easily perform my recruitment activities while using AI tools which I feed with my requirement and they will do the job of my behalf, in a very reduced period my pool of candidates for any vanacy is filled, and the lead time of hiring is getting very short compared to the old practices” | Increased profits | Efficiency, short lead time of hiring | Mikalef & Gupta, 2021; Frederico et al., 2020; Martins et al., 2020 |
Our findings demonstrate how in the HR of the healthcare sector, digital innovation and technological advances would allow organizations to achieve better economic and financial results while increasing productivity, employee retention, and overall satisfaction (Frey & Osborne, 2017; Agarwal et al., 2019; Barbazza et al., 2021; Bouncken et al., 2021). As shown in Table 2, the analysis conducted allows for identifying two competitive aspects that AI implementation has impacted. Our study clarifies how the development of new technologies significantly affects employment (Frey & Osborne, 2017; Neufeind et al., 2018; Gerards et al., 2021) and HR management within the organization such as by creating more hybrid skill sets, decreasing repetitive activities, and reducing workplace risks (Tambe et al., 2019; Bresciani et al., 2021a; 2021b).
The implementation of digital HR systems operated by AI tools will drastically reduce the time spent preparing employee compensation and benefits plan, tasks which used to consume most of HR personnel's time and used to be conducted through very primitive techniques. Implementing AI tools revolutionized processes and afforded HR staff the time to focus on more strategic topics (Sardi et al., 2020; Gerards et al., 2021; Leone et al., 2021; F. Schiavone et al., 2022). Operations time is a crucial element of a firm's performance. Using AI tools reduces timing drastically (Chatterjee et al., 2022), profoundly impacting cost.
There is a significant impact of AI tools on the process of hiring and recruiting talented staff, whereby such a process takes a considerable amount of time and effort, starting from screening hundreds of relevant and irrelevant resumes to finding a small selection of candidates who match job requirements. However, thanks to AI tools, the entire process from job posting to screening according to required criteria until shortlisting relevant candidates is done automatically and very efficiently (Hamilton et al., 2020; Paschen et al., 2020). The advantages listed above enable healthcare facilities to improve the quality of internal and external services while also ensuring a safer patient experience, highlighting how increasingly healthcare ecosystems must be oriented toward patients and their needs, placing them at the center of the decision-making process (Håkansson Eklund et al., 2019; F. Schiavone et al., 2022). Healthcare facilities must adopt a patient-centered approach. Patients' needs have become a major concern within any organization (Ippolito et al., 2019; Marimuthu et al., 2022). The implementation of AI has facilitated access to a huge amount of information to develop personalized care paths (Schiavone & Ferretti, 2019; Kraus et al., 2021; Kumar et al., 2022).
Digitalization and AI enable better economic and financial outcomes (Ziyadin et al., 2018; Sahlin & Angelis, 2019; Vial, 2021; Prager et al., 2018; Kumar et al., 2022). Despite the high cost of acquiring and developing such technologies, the return on investment is high, facilitated by decreasing workforce and headcount volume. It improves overall organizational performance, increases productivity, employee retention, overall satisfaction, and efficiency, and the optimization of tasks (Håkansson Eklund et al., 2019; Hamilton et al., 2020; Paschen et al., 2020; Gerards et al., 2021; Leone et al., 2021; F. Schiavone et al., 2022). It is worth mentioning that replacing one talented employee with all activities involved (onboarding, orientation, training, etc.) is three times more costly than retaining him. It is evident how crucial the implementation of AI is in economic terms (Kumar et al., 2022). Optimization of resource allocation and cost containment is at the heart of the healthcare management debate. In this vein, new technologies play a key role in enabling companies to minimize costs.
Utilizing digital tools promotes improved coordination between the parties involved and improves communication and consequently overall performance (Sahlin & Angelis, 2019; Sardi et al., 2020; Samson & Bhanugopan, 2022; Cristofaro et al., 2022). Compared to traditional procedures, AI technologies allow for greater productivity, improved patient safety, and better use of resources and permits. Indeed, one of the main objectives of today's healthcare ecosystems is the optimal allocation of scarce resources to ensure the best possible performance (Tambe et al., 2019; Lindebaum et al., 2020; Trischler & Li-Ying, 2022). AI technologies also open opportunities for staff to improve their skills and knowledge to provide the company with a competitive edge and enhanced performance (Olan et al., 2022). People will become a valuable and a nonreplicable resource for a company when HRM practices align with its competitive strategy. Therefore, people are an essential resource for any organization, and increasing their level of preparedness and competencies increases an organization's competitive advantage (Amarakoon et al., 2018).
Our analysis highlights the added value of AI. New technologies represent product and process innovation (Haefner et al., 2021). They push stakeholders to rethink and reorganize the services provided (Leone et al., 2021). Particularly in the health sector, the human component is difficult to replace (Huang & Rust, 2018; Haefner et al., 2021). Based on resistance theory, employees could represent one of the main barriers to technological change (Kaur et al., 2020). They may perceive the superiority of AI benefits over the costs of acquiring them. Our results clarify that the benefits of AI outweigh their investment costs. This should motivate HR departments to adopt AI solutions and exploit their opportunities.
This study has several theoretical, managerial, and policy implications. From a theoretical standpoint, it offers new insights for management scholars. First, the study highlights the need to rethink HRM processes considering the relevant role of digital technologies. Therefore, this analysis represents a tool to unify different research strands, as it contributes not only to HRM studies but also to AI and performance measurement studies. It represents actionable work that will enable other scholars to continue in the research field to find solutions whereby AI tools will be fully integrated into all HRM activities.
Practitioners and policymakers always prioritize reducing costs and improving organizational performance. In this vein, our study shows the significant impact of AI implementation on many aspects such as by drastically reducing the number of workers required . With the internet of things, the population is moving toward digitalization and using technology to make life easier. This study underscores the idea of implementing AI Software in human resources activities to transform HR from a support service to strategic and operational assistance such as by developing employees, strengthening employee engagement, enhancing retention, and contributing to overall organizational performance.
HR has a role to play in the community. and is not solely restricted to traditional tasks such as recruiting, onboarding, employee relations, and compensation and benefits. HR can also be a part of thoughtfully orchestrating the strategic and operational activities of healthcare organizations to optimize tasks and achieve excellence in service. In this vein, automation and AI are essential to reshape HR and take it to another level. Additionally, this study makes a valuable contribution to the field by tackling one of the current hottest topics in management—HR transformation and digitalization.
Although the study focuses on a single case study in a well-known healthcare organization, the results can be used and generalized to other healthcare institutions and countries. Decreasing the headcount in human resources via optimization of tasks is an objective of all healthcare institutions that can be achieved by incorporating AI algorithms within human resources activities. This also applies to the headhunting process and the recruitment of highly skilled resources; the latter is a key performance indicator for all reputable healthcare organizations, which can be achieved only by adopting new AI software handles the recruitment process from screening to hiring. Additionally, the results would be beneficial to other competitors in overcoming severe problems in the HR field such as onboarding, recruitment, payroll, and government relations issues.
Conclusion and research limitationsWith the rapid expansion of global markets and the vast development of technology, the traditional perks that used to grant organizations a competitive advantage will no longer stand. Technology allows organizations to imitate products and features quickly. CHROs are keen on the importance of AI in introducing new tools to ignite organizations’ capabilities. The new role of HRs in the era of AI is to automate operational tasks. One would not be surprised to have machinery with position titles and set job descriptions soon. Robotics are currently used in distribution companies to automate order preparations. Tap buttons installed at homes shift consumer behavior toward receiving orders from their household rather than visiting supermarkets. Tap buttons have also influenced the use of drones to deliver goods, affording a highly automated ordering experience.
Even when considering traditional HR processes, AI is taking part in the human role. Talent acquisition, from sourcing future candidates to automatically selecting profiles based on a set of attributes evaluated by virtual testing centers, has been influenced by AI. Automated platforms can also facilitate increasing employee capabilities and competence without relying on direct HR interference.
HR's new role will be to build a competitive advantage by acquiring AI and utilizing it to increase companies' capabilities in aspects such as speed and responsiveness. The new strategic role of HR will move into reviewing companies' structure to best integrate AI. HR will be able to advise on the necessary changes to obtain a firm base model that entails innovation and implementation of change programs. To achieve these goals, HR teams will also need to develop their skills to adapt to this new role within the framework of the evolving organization.
Aside from the high investment, implementing AI tools will undoubtedly represent a significant added value for any organization over the competition. Some challenges are associated with the availability of competent and expert staff; however, these challenges can be overcome with good management and a qualified talent question team capable of proper headhunting. The management-focused analysis shows the many advantages of implementing cutting-edge digital solutions in terms of care and, most significantly, the success of the structures. This demonstrates the solid competitive potential of this revolutionary technology, which must be seen as assets to be used rather than challenges to be overcome. The results highlight the need for managers to evaluate their strategy and resource allocations and how using novel ideas improves the caliber of the outcomes. Finally, the study provides insights to policymakers who are entrusted with promoting the advantages of cutting-edge digital technology by formulating financial incentives for healthcare organizations to create and use digital tools.
This study is not without limitations. First, the results are context specific. Future research could focus on public hospitals, where leadership styles and HR processes could differ. The higher cost of AI acquisition could represent another barrier to the generalization of results because of the scarcity of resources in the healthcare sector. Additionally, the lack of skilled clinicians who can manage the automation process could impede the speed of adoption. Finally, future work could integrate a multivariate statistical method to reinforce the analysis and generalize results to a representative population sample.
The authors contribute to the article as follow: P.L., T.A.M., A.B. and F.S. conceived of the presented idea. A.B., P.L., T.A.M, and F.S. developed the theory and performed the empirical analysis. A.B., P.L., T.A.M, and F.S. verified the analytical methods. P.L. and F.S. encouraged A.B. and T.A.M. to investigate the topics and supervised the general findings of this work. All authors discussed the results and contributed to the final manuscript.
The authors want to thank the Guest Editors and the reviewers of this journal for their valuable and constructive comments and suggestions which helped to improve the manuscript. The authors also want to thank the cultural association “Knowmedtech” (Italy) for the support in the development and of the article.