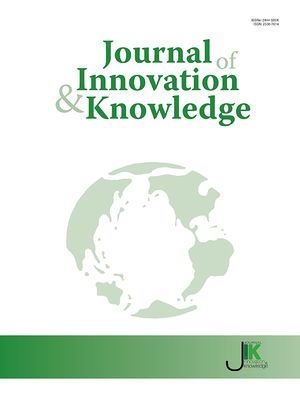
Editado por: Brij B. Gupta, Kwok Tai Chui
Más datosWith the widespread use of digital technologies such as big data, cloud computing and artificial intelligence in higher education, how to establish a scientific and systematic evaluation system to turn the traditional classroom with the one-way transmission of knowledge into an interactive space for exchanging ideas and inspiring wisdom has become an essential task for human resource management in universities, and a key to improving teaching quality. However, due to the debate between scientism and humanism in teaching evaluation, studies related to teaching performance have been isolated from human resource management, resulting in the lack of a systematic vision and framework for such studies. Relevant studies are still limited to the evaluation contents of different evaluation subjects. Evaluations also tend to focus only on the teaching process, ignoring the objectives of talent training, making it difficult for evaluations to play a goal-oriented role and hindering the further development of relevant studies. Therefore, this paper draws on human resource management methodologies and analyzes knowledge teaching evaluation system characteristics in colleges and universities in a big data context to construct a “multiple evaluations, trinity and four-step closed-loop” big data-based knowledge teaching evaluation system. “Trinity” represents evaluation from three performance dimensions: teaching effect, teaching behavior and teaching ability. “Multiple evaluations” represents the design of teaching performance indicators based on teaching data, breaking the barriers between different evaluation subjects. “Four-step closed-loop” draws on performance management theory to standardize the teaching performance management process from four aspects: planning, implementation, evaluation, and feedback. This evaluation system provides a systematic methodology for unifying the theory and practice of innovative knowledge teaching evaluation system in universities in a big data context.
Advanced information technology (IT), represented by artificial intelligence, big data, and mobile internet, is changing educational philosophies, teaching methods, and roles of teachers and students in higher education. Teachers are no longer transmitters of knowledge but activators of cognitive activities, managers of teaching activities, and supporters of learning activities. There is an urgent need to revolutionize teacher performance evaluation, drive the deep integration of modern IT and higher education, and continuously improve the quality of higher education talent training. Many scholars try using objective data recorded by various smart terminals to make predictive assessments of emerging teaching modes such as online education, flipped classrooms, and blended teaching through big data mining and machine learning algorithms (Cruz-Jesus et al., 2020; Golenhofen et al., 2020; Leal-Rodriguez and Albort-Morant, 2019). However, scholars also contend whether the excellence of higher education can be accurately measured, criticizing the tendency towards quantitative managerialism based on objective data, limiting the improvement of teaching quality and the achievement of teaching objectives. The pervasive scientism versus humanism debate in educational evaluation has been intensified, not dispelled by the widespread use of IT. At present, research on teaching evaluation has mainly focused on the evaluation content of different evaluation subjects or the development of evaluation research on an emerging teaching model, neglecting the objectives of talent training and making it difficult for evaluation to play a goal-oriented role. Furthermore, few studies have combined human resource management theory with teaching evaluation (Lohman, 2021), resulting in a lack of a systematic vision and framework for such research.
Therefore, this study conducts a bibliometric analysis of international research on teaching performance, analyzes the current research trends and hotspots in the field, reviews mainstream teaching evaluation models, summarises the shortcomings of relevant research, studies the factors that restrict the further development of teaching evaluation, and proposes specific directions for improving the innovation knowledge teaching evaluation system. This study offers a "multiple evaluations, three-in-one and four-step closed-loop" innovation leading evaluation system based on big data at three levels: the main body of the innovation knowledge leading evaluation system, the evaluation dimensions and the evaluation links. The evaluation system aims to make up for the systematic deficiencies of traditional knowledge teaching and establish a student-centred, output-oriented and continuous improvement evaluation system for innovative knowledge teaching. Its significance is mainly reflected in the following three aspects: firstly, it breaks the barriers between different evaluation subjects, proposes the establishment of multiple evaluation data sources based on big data, and innovates the teaching performance evaluation strategy. Secondly, it expands the connotation of teaching performance of university teachers in the era of big data based on the logic of "ability - behavior - result" and enriches the theory of teaching performance evaluation. Finally, it draws on the PDCA management concept to standardize the teaching evaluation process, providing a systematic methodology for university teaching evaluation in the context of big data.
Literature reviewThis literature review summarises the current popular research on knowledge teaching evaluation systems, analyzes the problems in the research on knowledge teaching evaluation systems and proposes directions for improving knowledge teaching evaluation systems.
Analysis of trends in knowledge teaching evaluation systemWe used the Science Citation Index Expanded (SCIE), a citation database in the Web of Science core collection, to understand the research hotspots of the knowledge teaching evaluation system and explore future developments. The search method was AK = “Teaching Evaluation/Teaching Assessment/Instructional Evaluation/Teaching Appraisal,” or AK = “Teaching Performance,” and Search until December 2021, resulting in 1125 documents. The basic information about the issuance is shown in Fig. 1. Fig. 1(a) shows the number of published articles about teaching evaluation until December 2021. From 1991 to 2002, the amounts of articles are small and had little change. However, from 2003 to 2021, the amounts have increased sharply, and the ratio keeps going up. Fig. 1(b) shows the top areas related to teaching evaluation. The chart shows that the top five areas are Education Educational Research, Computer Science, Engineering, the Health Care Sciences Services and Nursing. Computer Science is the most related area for teaching evaluation. Fig. 1(c) shows the productive scholars publishing in this area. Fig. 1(d) lists the top institutions in this area. The top three affiliations are the University of California System, University of London and Pennsylvania Commonwealth System of Higher Education Pcs. This figure shows that most of them are USA universities, corresponding to the following analysis about countries or regions. As shown in Fig. 1(e), countries or regions that are active in this field, of which the United States, China, and the United Kingdom are the top three.
In order to analyze the evolution of research trends on "teaching evaluation" and to understand the keyword themes corresponding to each period, this paper was used to import the literature data into Citespace (Chen, 2014), with the period set to 1991–2022 and the time slice set to 1 year, and to cluster the keywords by their occurrence in The clustering analysis was conducted on the timeline of their occurrence. The clusters were named by choosing the title terms and labelled by the LSI algorithm, and a timeline clustering map was chosen to display the clusters to analyze the historical span of the clustered topics and the links between clusters in a time series. The clustering results are shown in Fig. 2. Each circle in the figure represents a keyword that only appeared when first proposed (Gobbur et al., 2022). The lines in the graph represent links between keywords, and connecting lines indicate that two keywords appear together in one or more papers. The graph's vertical axis is the cluster number, and the horizontal axis is the year in which the keyword appeared and the network in which it co-occurred. The clustering module value Q is 0.3565, which is greater than 0.3, indicating a significant clustering structure, and the mean profile value S is 0.766, which is greater than 0.5, indicating a reasonable classification of the clustering results (Chen, 2014). From this Fig. 2, we can initially determine that international research on teaching performance has focused on five main themes, namely 'student assessment,' 'case studies,' 'medical education,' 'academic achievement', and 'artificial intelligence,' which are arranged vertically by size in the figure, demonstrating to some extent the hot spots and trends in research in the field of teaching performance. Each of these five themes will be analyzed in the following paper.
Keyword co-occurrence maximum clustering 0# student evaluation mainly concerns performance, competence, validity, instruction, and higher education. They span the period 1999 to 2021. It is suggested that SET (Student Evaluation Of Teaching) has long been an essential element of teaching performance evaluation research. Students, peers and teaching managers may all be evaluators of teachers' teaching performance, but student evaluation of teaching remains the main form of evaluation of teaching performance (Crisp, 2018; Dillon et al., 2020; Kayas et al., 2022; Koslow, 2018; Peterson et al., 2019). The keywords co-occur in the second-largest cluster 1# case studies mainly include teaching/learning strategy, quality, improving classroom teaching, evaluation methodology, and span 2001 to 2021. The studies mainly focus on the case validation of the effectiveness of a particular teaching strategy, especially with the widespread use of information technology in the teaching field, the number of related research hotspots has increased significantly. Wieling et al. (2009) used regression analysis to explore a hybrid teaching model combining face-to-face and online instruction, analyzing how online on-demand video recordings, online quizzes, etc., positively affect student performance. Leal-Rodriguez and Albort-Morant (2019) Study finds that experiential learning strategies facilitate students' understanding of theoretical concepts and superior performance. Keyword co-occurrence clusters 2# medical education mainly included skill, science, outcome, impact, and student performance, spanning 1996 to 2021. It is suggested that current international research on teaching performance is focused on the field of medical education. One of the earliest publications was a study on an evaluation scale based on a problem-based pedagogy in medical education (De Grave et al., 1996). 3# Clustering academic performance mainly includes the keywords experience, curriculum, technology, academic performance, and keywords spanning 2008 to 2021. It is suggested that current research on teacher performance evaluation is beginning to focus more on student academic achievement. For example, Golenhofen et al. (2020) explored the impact of mobile learning tools on students' academic achievement through instructional assessment. In their study Cruz-Jesus et al. (2020) attempted to assess students' academic achievement using artificial intelligence methods. 4# Artificial intelligence mainly includes teaching evaluation, teaching quality, artificial intelligence, improved algorithm, machine learning and other keywords, spanning 2007 to 2021. A literature review shows that one of the earliest articles on artificial intelligence in teaching was virtual microscopy in teaching (Sims et al., 2007). The fundamental studies on AI technology to improve teaching performance evaluation are all relevant articles published after 2018. For example, Lin et al. (2018) extracted opinions from students' qualitative evaluation of teachers through machine learning algorithms to improve the reliability and efficiency of students' teaching evaluation. Liu Meichen and Huang Jieru (2021) tried to build an online teaching quality evaluation model based on emotion recognition and data mining algorithms.
In summary, it can be inferred that, after entering the 21st century, international research on teaching performance has gradually become a hot spot for many scholars, with relevant research favouring teaching practice, its primary form of evaluation being student assessment. The research's content is mainly on information technology teaching strategies in teaching applications, mainly in medical education. The latest research frontier is the assessment of students' academic achievement and The application of artificial intelligence technology to evaluate teaching performance.
Overview of mainstream teaching evaluation modelsIn order to further summarise current research findings on teaching evaluation, we interpret three generic teaching evaluation frameworks, the Dynamic Model of Educational Effectiveness, the International System of Teacher Observation and Feedback (ISTOF) and the three basic dimensions of teaching quality (TBD), which are currently the most respected by scholars. One of these "dynamic models of educational effectiveness" "captures eight elements of the teaching role in teaching that relate to student achievement: knowledge delivery, structuring, questioning, instructional modeling, application, time management, how the teacher makes the classroom a learning situation, and classroom assessment. It is also proposed that the relevant factors be defined and measured in five dimensions: frequency, focus, stage, quality and differentiation (Reynolds & Neeleman 2021; Sarid, 2022). The International System for Teacher Observation and Feedback (ISTOF) originated from an international, transnational project (Charalambous et al., 2021; Guimarães & Lima 2021; Muijs et al., 2018). A team of experts from more than 20 countries conceptualized, defined and measured 11 elements of classroom practice relevant to teachers, including assessment and evaluation, clarity of teaching, classroom climate, classroom management, differentiation and inclusion, teaching skills, planning of individual lessons, long-term planning, professional knowledge, teacher professionalism and reflective skills, promotion of active learning and development of metacognitive skills. The Three Basic Dimensions of Teaching Quality (TBD) is the most prominent framework for teaching quality in German-speaking countries (Praetorius et al., 2018). An effective teaching model should contain three dimensions classroom management, student support and cognitive activation. From these three models of teaching evaluation, it can be seen that the current mainstream research in teaching evaluation still focuses on the teaching process of teachers and does not fully consider the needs of society for future talents and how to use an effective management process to ensure that the evaluation gets the desired effect, lacking systematic research.
Although IT has profoundly impacted teaching evaluation and scholars try using objective data recorded by various smart terminals to address the uncertainties of teaching evaluation, the critique of scientific measurement in teaching evaluation persists. Kreitzer and Sweet-Cushman (2021) debate overly objective evaluation criteria and managerialist tendencies. Research on "teaching evaluation" rarely refers to literature on human resource management, and even then, it only describes the research's meaning and does not apply the well-established concepts and techniques of human resource management to teaching evaluation (Crisp, 2018; Dillon et al., 2020; Koslow, 2018; Thomas et al., 2014). Current research hotspots and debates on teaching evaluation centre on the evaluation contents of different subjects (Heffernan, 2022); there is a lack of systematic research. Therefore, combined with literature combing, this paper summarizes three main problems of the knowledge teaching evaluation system.
First, although scholars have tried to use objective data to replace subjective evaluation, due to the complexity and uncertainty of teaching activities, many observations still rely on the subjective judgments of evaluation subjects. Second, previous research on evaluation content tends to focus on the teaching process, ignoring the causal relationship among evaluation indicators, making it difficult for evaluation to play a goal-oriented role, and ignoring the holistic nature of talent cultivation. Thus, there is no synergy between different courses in the evaluation process to develop key competencies for students. Finally, due to the controversy over the materialist tendency of teaching evaluation, well-established performance management concepts and human resources management theories are rarely applied to teaching evaluation. Therefore, teaching evaluation is often used as an administrative control tool, which is criticized, even more, forming a vicious circle.
Deming, a leading management scientist, says, “Universal causes can be attributed to system failures. These systemic problems are inherent within institutional processes. They can only be solved or reduced by changing systems, processes and procedures (Sallis, 2005).” Therefore, research related to performance management in mature human resource management can be drawn upon to address the dilemmas facing instructional performance evaluation.
Construction of a big data-based innovative knowledge teaching evaluation system in universitiesWith the widespread use of information technology in higher education, big data in education is exploding, bringing new opportunities for teaching performance evaluation. However, there is a gap between theory and practice in the teaching evaluation practice, from the evaluation concept and standard to the collection and analysis of evaluation evidence. There is an urgent need for big data in education to evaluate teaching performance and conduct systematic research based on human resource management concepts. Therefore, this paper constructs a “multiple evaluations, trinity, four-step closed-loop” innovative knowledge teaching system for university teachers from three levels: evaluation sources, dimensions, and processes. The system requires universities to establish an intelligent data environment to transfer all types of data information in real-time, followed by the use of an information technology platform to organize and optimize the storage of data information and then mine and utilize the data information to achieve intelligent decision-making and control of teaching evaluation. The process takes big data technology as the core, completes data collection and cleaning through machine learning and data mining, uses data modeling to carry out different types of teaching evaluation, and supports the performance management process based on data analysis (Fig. 3).
Establishing multiple sources of evaluation data based on big dataDue to the widespread use of new technologies such as various learning platforms, mobile APPs, digital terminals and wearable devices, the complex two-way interaction between teachers and students in teaching activities is recorded in the form of images, sounds and texts by various terminals, providing a more objective source of evaluation data for the innovative knowledge teaching evaluation system. However, teaching activities involve complex emotions, attitudes, abilities and other potential characteristics of teachers and students, which are still not fully characterized by these objective data and still require subjective judgment by different evaluation subjects. Therefore, to evaluate the performance of university teachers in the context of big data, it is necessary to design teaching performance evaluation indicators in an integrated manner on the one hand and make full use of objective teaching data recorded by various smart terminals to describe performance indicators. When objective data cannot characterize specific performance indicators, the evaluation content of different evaluation subjects is determined according to the principle of "who knows, who evaluates," and subjective and objective evaluation data are combined, complemented and verified to evaluate teachers performance. In addition, schools need to establish a multi-system shared database to collect the various types of data required for teaching performance evaluation, cleanse, convert and improve them, and process the raw data into an appropriate form. The most common methods of data cleaning include: interpolating missing values using weighting, machine learning, mean and EM algorithms, screening outliers using the Dixon test and Grubbs test, and converting objective data into graded data. In these ways, the data quality can be improved, and biases in the distribution between variables can be eliminated to facilitate further statistical analysis.alysis.
Conducting a trinity evaluation of teaching performanceThere is no coherent definition of performance in management circles. Some scholars focus on performance concerning outcomes or work effectiveness (Bernardin & Beatty, 1984; HwanSun, 2001; Jing, 2019; Weiqing & Kunjian, 2000); others look at behavior concerning the achievement of set work objectives (Campbell et al., 1998; Williams, 1999; Murphey & Cleveland, 1995). More research looks at performance as competencies concerning specific organizational systems and job positions (McClelland, 1973; Sandberg, 2000; Spencer & Spencer, 2008). These performance definitions are not isolated, and many studies suggest that they should be integrated. Brumbrach (1988) proposed that performance represents behavior and results, and Otley (1999) expounded that performance is the process of working and the results it achieves. Scholar Qin (2009) suggested that it is an all-around performance of an individual's ability and behavior and the results in the organization. Shoudong and Guohua (2014) advised that, when assessing performance, the theoretical basis and characteristics of performance should be considered comprehensively, looking at organizations' and individuals' abilities to perform certain behaviors and achieve specific results.
Teaching performance can be defined considering three dimensions: teaching effectiveness, teaching behavioral performance, and teaching competence. The evaluation of teaching effectiveness is based on a past perspective and provides a summative assessment of teachers' impact on students. The evaluation of teaching behavior is a process evaluation of teachers' teaching behavior from a present perspective. The evaluation of teaching competence is based on a future perspective and is a developmental evaluation of the teacher's potential competence and quality. At the same time, we should be clear that teaching effectiveness performance is the most important of the three dimensions, both in terms of what students have learned, far more critical than what teachers can teach and how they can teach it (Yang & Fan 2022). However, individually, these dimensions cannot measure teaching performance comprehensively. Teaching effectiveness performance is result-oriented, disregards teaching behaviors, and is not conducive to performance improvement and process control. Teaching behavior performance, although process-concerned, can quickly become incorrectly oriented, causing results to deviate from objectives. Although teaching competence performance helps improve teachers' professionalism and competence, teaching ability is often difficult to judge objectively, so it cannot lead to an innovative knowledge teaching evaluation system. Therefore, we draw on the Balanced Scorecard theory (Tuan, 2020); through the causal relationship between teaching performance indicators and based on the concept of balance, we design teaching evaluation dimensions from three aspects: teaching effectiveness, behavior performance, and ability performance.
Teaching effectiveness performance evaluationThe teaching effectiveness performance evaluation is a summary evaluation of the teaching effect of a particular course. The evaluation content is mainly based on the teacher's influence on students. However, with the continuous development of information technology, the classroom is no longer the only channel for acquiring knowledge. Human beings acquire knowledge more and more efficiently, and knowledge transfer is no longer the only goal of teaching and learning. The "core competencies" can be effectively delivered; that is the ultimate goal of higher education. In the 21st century, countries and international organizations worldwide have put forward theoretical frameworks on "Key Competencies." Although these frameworks differ in terms of research perspectives, values and targets, they share many similarities in indicator settings, such as greater emphasis on innovation and achievement motivation, information literacy, communication and exchange, teamwork, social participation and contribution, and self-planning. The essence of "Core Competencies" is not just knowledge and skills, interest, motivation and attitude, but also the thinking, judgment, expression and personality required to apply knowledge and skills and solve practical problems. Universities should make this the core objective of talent training and break it down into the teaching objectives of specific courses (Lee & Son 2021; Son et al., 2021). Objectives and key results (OKRs) are based on the performance management concepts of Management By Objectives (MBO) and Key Performance Indicator (KPI), incorporating milestones and critical results so that performance evaluation is consistent with objectives, measurable and timely(IRIKEFE, 2021), and has a good fit with the teaching effectiveness evaluation. Therefore, universities can learn from OKR performance evaluation, decompose talent cultivation objectives into key teaching outcomes of courses at different levels, and measure the achievement of crucial teaching outcomes according to students' learning. To achieve this, the teaching management department needs to break down the talent training objectives for each course according to the training program. The course syllabus should also be developed based on the course objectives, with a detailed definition of the expected outcomes of developing competencies and qualities for each type of student.
Additionally, we should note the differences in the teaching objectives of different disciplines. Therefore, when evaluating teaching effectiveness, we must be result-oriented and categorical and dynamically assess the teaching effectiveness of the course, "measure ourselves with our ruler," and provide timely feedback to teachers to facilitate the adjustment of teaching strategies and improve teaching quality. In this process, universities need to integrate data from student information systems and learning management systems, collect, collate, mine and predict data on students' daily performance, and analyze students' overall status by building models to assess the achievement of course objectives and make recommendations for a new round of teaching. The implementation path of teaching effectiveness evaluation is shown in Fig. 4.
Teaching behavior performance evaluationTeaching behavior performance is a process evaluation of teachers' teaching behavior based on a present-day perspective to correct deviations promptly. We can draw on the KBI (Key behavior Indicator) to conduct assessments of teaching behavior performance. KBI performance management tool is essential for evaluating the performance of an organization or employee on predetermined key behaviors in process management within a specific time and responsibility frame (Ge et al., 2007). KBI-based performance assessment of teaching behaviors is based on effective teaching methods and objectives, anchoring key teaching behaviors, breaking them down and refining them, and identifying evaluation criteria based on the characteristics of the indicators to assess teaching and learning activities. The process requires three components: first, identifying crucial teaching behaviors. With the deep integration of information technology and education teaching, the objectives and ways of teaching are undergoing profound changes. In order to build a scientific and reasonable teaching behavior performance, it is necessary to make an in-depth analysis of the current teaching mode reform and anchor the key teaching behavior according to the talent training objectives. Secondly, the key teaching behaviors should be broken down and refined. In other words, according to the "SMART" principle, the key teaching behaviors should be decomposed into quantifiable evaluation indicators, and their definition, scope and connotation should be clearly explained. Finally, the quantitative criteria are determined according to the meaning of the indicators. The quantitative criteria for key teaching behaviors can be divided into category criteria, frequency criteria, sequence criteria, gap criteria and ratio criteria, which need to be determined according to the specific characteristics of the indicators. The implementation often fails to achieve the expected results due to the heavy workload, time-consuming and subjective nature.
However, in big data, various documents and behavioral activities of teachers are recorded and stored by various teaching terminals, which significantly reduces the running costs of process evaluation and improves the objectivity and authenticity of evaluation. Universities should use the Internet to establish an online teacher evaluation system based on big data. Course teachers can conduct self-evaluation and other evaluations within a specified period. Teaching managers and supervisory experts can conduct random evaluations of courses. Through a real-time data feedback mechanism, the evaluation results are tallied, classified, summarised and analyzed through the web terminal and fed back to the teachers themselves to ensure that the problems reflected in the evaluation can be addressed promptly.
Teaching competency performance evaluationTeaching competency performance evaluation is a developmental evaluation of teachers' competencies, focusing on the professional knowledge skills, concepts, attitudes, and motivations teachers need to possess to deliver quality teaching. Schools can draw on the critical competency index, which represents quantitatively evaluating the potential traits of individual employees to align the development of individual organizational members' competencies with organizational development goals (Haixia & Zhisong, 2020). The teaching ability evaluation should not focus on the conclusive evaluation of teachers but should adhere to the principle of development; be based on the future; and focus on the teachers' professional development, the construction and training of the teaching team, the improvement of the quality of talent training, and the sustainable development of universities. We should evaluate teachers' knowledge and skills, values, ideology, and other potential traits in the evaluation process and avoid making qualitative judgments based on their teaching experience, academic qualifications, titles, teaching reform papers, and projects. To accurately characterize and evaluate teachers' abilities, it is crucial to fully use the comprehensiveness, completeness, dimensionality, fragmentation and authenticity of data and conduct high-quality analysis and processing to correlate relevant data with concepts. Accurate evaluation can help rationalize the allocation of teaching resources and improve teacher training.
Thus, the design of three-dimensional teaching performance fully reflects the balanced performance concept of "results + behavior + potential." Different dimensions of innovative knowledge teaching evaluation systems are carried out at different times for different evaluation contents, acknowledging the differential role of evaluation and considering the value needs of different participants. The model facilitates the complete stimulation of teachers' teaching potential. We can synthesize teachers' performance in various types of performance evaluation, scientifically establish evaluation models, and provide adequate support for their performance evaluation. The types of the big data-based innovative knowledge teaching evaluation system and related elements based on the balanced performance view are shown in Table 1.
Characteristics and elements of big data-based innovative knowledge teaching evaluation system based on the view of balanced performance.
Scholars agree that teaching evaluation should help teachers continuously improve the quality of their teaching (Fawns et al., 2021). However, current research on knowledge-based teaching evaluation systems still focuses on the content and form of evaluation of different evaluation subjects. Universities tend to treat the knowledge teaching evaluation system as a simple monitoring and assessment tool, lacking the awareness of performance management and neglecting the process of the knowledge teaching evaluation system. To address this, universities can draw on performance management theory and organize and implement knowledge teaching evaluation system according to the PDCA cycle of total quality management (Realyvásquez-Vargas et al., 2018):
- •
P: Teaching performance planning, the premise and foundation of performance management
- •
D: Teaching evaluation implementation, the guarantee of effective implementation of teaching performance management
- •
C: Teaching performance evaluation, the core joint of teaching performance management
- •
A: Teaching performance feedback, the key to the effectiveness of teaching performance management
These four links in an evaluation cycle are advanced gradually, and when the cycle ends, the next cycle is carried out according to the process and repeated to ensure the continuous improvement of college teachers’ performance. Simultaneously, schools should develop teaching performance management systems based on the cloud management platform of big data to realize data and information management support for teaching performance at all stages of planning, implementation, evaluation and feedback, in order to clarify performance targets, formulate plans, improve evaluation systems and sound feedback mechanisms for teaching performance. The process will help promote the overall improvement of teaching performance and build a virtuous cycle system of teaching performance management for university teachers based on the big data environment, as shown in Fig. 5.
Teaching performance planA teaching performance plan is the process of communication between the university and teachers about the teaching performance that teachers should be achieving. The result of the communication is formally written up as a contract about the teaching work goals and standards expected by both parties based on clear responsibilities, rights, and benefits. Specifically, we can divide this into the following three links.
- (1)
Clarify the strategic vision and design the implementation plan. Before the teaching performance plan, universities should use the SWOT analysis (Longhurst et al., 2020) to consider the external environmental factors such as social, cultural, economic and social responsibility, as well as internal factors such as student quality, faculty strength and financial situation, to identify the opportunities and challenges faced by the university, as well as its strengths and weaknesses, and to determine the strategic vision of the school for a certain period in the future. Based on this objective, the implementation plan is designed in three dimensions: teaching effectiveness, teaching behavior and teaching competence. In particular, the evaluation of teaching effectiveness performance is a summative evaluation of the effectiveness of the course based on the perspective of the past to improve teaching effectiveness. The content of the evaluation should be based on the impact of teachers on students in the teaching process, and the data source of the evaluation mainly relies on the learning behavior data accumulated by students in their daily course learning, supplemented by students' subjective feelings about the teaching effectiveness of the course. The performance of teaching behavior is a process evaluation of teachers' teaching behavior based on a present-day perspective to correct deviations promptly. The evaluation content is based on teachers' teaching behavior in the teaching process, and the data source of the evaluation mainly relies on the behavioral data accumulated by teachers in the teaching process. It can be supplemented by peers' or experts' evaluation results of daily teaching inspections. The Teaching Ability Performance is a developmental evaluation of teachers' teaching ability based on a future perspective to improve teachers' teaching ability. The evaluation is based on the potential attributes that teachers need to possess to deliver quality teaching. The primary data source for the evaluation is based on fundamental data from teacher management, training and assessment, supplemented by the results of teachers' self-assessment.
- (2)
Define teaching work responsibilities and design teaching performance indicators. The school should define teachers' job responsibilities by analyzing the critical business contents of their work, investigating the main results achieved through the goal analysis method, and writing descriptions concisely and accurately. Then, schools can design teaching performance indicators following the innovative principles: teaching performance indicators must be specific, measurable, attainable, relevant to other goals, and have clear time-bound deadlines (Pryvalova 2020). Further, the selection of key performance indicators must strive to be scientific and reasonable, allowing for the incentive and constraint effects of performance management and maximizing the level of teacher performance.
- (3)
Establishing performance index weights and performance evaluation model. Teaching performance indicators are characterized by diversity and structural complexity; using “data to speak” is key to the big data-based innovative knowledge teaching evaluation system. Here, first, we need to analyze the nature and characteristics of teaching work and related influencing factors, assigning corresponding weight to each performance indicator according to its importance in the performance indicator system. Simultaneously, since the big data-based innovative knowledge teaching evaluation system is a typical multi-objective decision analysis and requires the comprehensive analysis of evaluation results, we must also design scientific and reasonable evaluation models to reveal the relationship between relevant concepts and data to improve the simplicity, scientificity, and accuracy of knowledge teaching evaluation system.
Teaching performance implementation requires actioning the teaching performance plan and ensuring the performance management process's effectiveness through performance communication and coaching. In the specific implementation process, three aspects must be noted.
- (1)
Conducting performance coaching and formulating action plans. Since teaching is a two-way interaction between teachers and students, there are two levels of teaching performance communication and coaching. The first is performance communication and coaching between teaching managers and teachers. Teaching managers should ensure that teachers carefully study the school's talent training objectives, training programs, and syllabus to see that their programs match its vision. Teachers should communicate with students regarding teaching design, performance communication, and counselling objectives. Based on a complete understanding of students’ learning characteristics and needs, teachers should develop lesson plans and communicate course teaching objectives and plans to students to develop personalized learning plans and ensure the achievement of course teaching objectives.
- (2)
Strengthen process management and guarantee effective implementation. During the specific implementation of teaching activities, teaching managers should keep abreast of teachers’ work progress, understand their performance and the difficulties they encounter, discover and correct issues timeously to avoid irreparable losses, and identify high-performing behaviors to summarize and promote advanced working experiences to improve teachers’ overall teaching performance. Schools can adopt various teaching monitoring measures such as teaching supervision and inspection, multi-listening, and student survey to implement process management on the quality of all aspects of teaching.
- (3)
Retaining original information and providing an essential basis. Only when teachers’ work processes are clearly understood can the school correctly assess and evaluate their work. Therefore, only by mastering and accumulating the original performance information of teachers in specific teaching activities can the knowledge teaching evaluation system to be more realistic and credible and avoid bias. Managers should use information technology management tools while implementing process management to collect and collate information on the performance of teaching and learning activities to provide a reliable basis for the knowledge teaching evaluation system.
Knowledge teaching evaluation system refers to the school's objective, fair, and accurate evaluation of teachers’ performance, behaviors, and abilities through quantitative and qualitative comparative analysis and scientific evaluation methods following the predetermined evaluation procedures, unified standards, and specific index system under the established talent cultivation objectives key to the effectiveness of teaching performance management. With big data, the knowledge teaching evaluation system must consider two aspects.
- (1)
Selecting data sources and accurately characterizing indicators. When universities use big data to conduct knowledge teaching evaluation systems, they should fully use objective data recorded by various terminals. It is conducive to training teachers to use IT to teach and promote teaching performance improvement. The knowledge teaching evaluation system based on big data technology can form a virtuous cycle. When objective data cannot characterize performance indicators, universities need to select evaluation subjects, design evaluation questionnaires, and obtain evaluation data according to the “who knows, who evaluates” principle. For objective data, schools must use data mining technology to analyze and model unstructured data such as video, audio, and images to explore their deeper meanings. For subjective data, when designing and collecting questionnaires, they should try to obtain data by stating facts rather than judging the correctness and merit, avoiding evaluator bias.
- (2)
Clarify the evaluation form and carry out the evaluation scientifically. According to the evaluation content, the knowledge teaching evaluation system can be divided into teaching effect evaluation, teaching behavior evaluation, and teaching ability evaluation. The evaluation objective can be divided into summative, process, and developmental evaluation. The evaluation subject can be divided into student, peer, teacher, and leader evaluation. Time can be divided into phase evaluation and daily evaluation. Therefore, according to the purpose of the knowledge teaching evaluation system, choosing different evaluation contents, subjects, and time for evaluation are essential to ensure the scientific nature of big data-based knowledge teaching evaluation system.
Feedback on teaching performance is the communication between teaching managers and teachers about teaching performance in the evaluation cycle. The process involves recognizing achievements, identifying shortcomings, and suggesting improvement. Teaching performance feedback aims to let teachers know whether the teaching effectiveness has met the set teaching objectives, the teaching behavior is scientific and reasonable, and the teaching ability is sufficient to support the teaching objectives during the evaluation cycle. The following points are essential in this process.
- (1)
Encourage participation and effective feedback. Teaching performance feedback should encourage teachers to participate in the feedback process rather than simply informing teachers of the evaluation results. For positive performance, instructional managers should offer sincere praise to teachers for specific behaviors, show the school's appreciation, and motivate teachers to receive positive feedback. For negative performances, non-judgmental communication with teachers is essential. Specific evaluations should be proposed at the right time, so teachers understand the objectivity of performance evaluation, identify the problems themselves, and reach a consensus to solve these problems. Furthermore, instructional managers should record teachers’ key behaviors and categorize them to maintain and reinforce positive performance behaviors through incentives and find reasons for negative performance behaviors towards proposing improvement plans.
- (2)
Future-oriented and positive guidance. Although most teaching performance feedback content reviews and summarizes the past, performance feedback looks to the new evaluation cycle. Therefore, the feedback process should focus on the performance indicators’ content and implement performance improvement plans concerning teachers’ actual situations to support the next performance-planning round. Teaching managers also need to be aware that negative performance feedback can psychologically suggest teachers and affect their career development. Teachers should therefore be encouraged to feel that, despite the unsatisfactory results of their performance assessment, they have come to an objective understanding of themselves and have found a direction in which they should work, regardless of whether the results are good or bad. This approach will enable teachers to engage in future teaching with a positive attitude.
- (3)
Regular feedback and mechanism formation. Teaching administrators should use feedback on teaching performance as an effective measure to correct deficiencies found in the evaluation process and communicate with teachers timeously to avoid more significant mistakes. Further, teaching administrators should provide teachers with regular performance feedback to understand their performance evaluation results before the formal evaluation. This process will encourage teachers’ agreement with the results and guarantee feedback effectiveness. Therefore, whether through regular performance feedback or staged performance feedback, a corresponding system should be established, and only by institutionalizing it can we ensure performance feedback have a lasting effect.
With the development of the mobile Internet, big educational data, and artificial intelligence technologies, information technology has penetrated every aspect of education and teaching. It is driving a profound transformation of traditional education into intelligent education. Innovative applications such as intelligent recommendations, adaptive learning, and digital learner portraits have enriched the teaching experience of teachers and students and dissolved the temporal and spatial boundaries of traditional teaching practices. Learning resources are no longer static textbook content but generative knowledge co-constructed by learners. Students are no longer confined to physical space; they are extensively interconnected with the world through the Internet. Teachers are not only the transmitters of knowledge but also the managers of teaching activities and the facilitators of student learning in the IT environment. Although the accumulation of these changes has not yet resulted in a qualitative change, the future trend is clear. Scholars have suggested that traditional teaching evaluation theories and methods are controversial in explaining new educational phenomena and guiding intelligent educational practices. They, therefore, call for a reconstruction of the theory of knowledge teaching evaluation systems in the information technology era. Through the theoretical study of university teachers' knowledge teaching evaluation system, this paper proposes a knowledge teaching evaluation system of "multiple evaluations, three in one and four closed-loop steps." However, university teachers' knowledge teaching evaluation system in big data is complex. It requires continuous research and practice to promote modernization and reform in higher education as information technology is widely used in higher education. This paper has four shortcomings from the theoretical and practical perspectives that need further research.
- (1)
The "multiple evaluations, trinity and four-step closed-loop" big data-based evaluation system is still theoretical; it needs further tested and optimization. It is a long-term and complicated process to put it into practice and establish scientific performance management awareness in colleges and universities.
- (2)
Although this paper proposes to design the performance index system based on the Balanced Scorecard theory and the three dimensions, "teaching effectiveness," "teaching behavior," and "teaching ability," it should be further designed to evaluate the performance of universities. However, to effectively infer and evaluate the teaching performance of university teachers, we must first determine the content of the specific evaluation, building a scientific and reasonable teaching performance index system. However, the research on teaching performance indicators is currently mainly carried out for non-evaluating subjects. The index system is mixed and repeated, which is not conducive to practical performance evaluation. Moreover, with the reshaping of higher education by IT, the teaching objectives, contents, and methods have changed implicitly. Hence, the evaluation content of teaching performance in a big data context needs to be further studied.
- (3)
(3)Education data is a valuable educational asset, but it also involves the privacy of both educators and recipients of education. The public data openness movement has been an international trend, and as education is a public good, education data should also be opened up to the community appropriately. However, while promoting the education data openness movement, high importance should be attached to the privacy protection and security management of education data. Continuous efforts should be made to adopt more advanced and higher security measures to safeguard education data and protect education privacy data from leaks and malicious use. Develop management rules at multiple levels, including institutional, institutional, technical, and methodological levels, to effectively safeguard the security of education data of individuals, institutions, and the state. Establish a sound education data security management structure, including data production departments, data use departments, data management departments, and clarify the security management responsibilities of each department. Establish the confidentiality level of education data and take relevant handling measures according to the confidentiality level. Monitor the application status of education data in real-time and prevent illegal applications and infringement of user privacy.
- (4)
Wisdom evaluation based on extensive data mining and artificial intelligence is the main direction of future teaching evaluation. The wide application of these technologies will significantly improve the efficiency and quality of teaching evaluation. However, a big data-based knowledge teaching evaluation system is a human cognitive and decision-making activity. In its practice, it is necessary to uphold the concept of human-computer collaboration, focus on the data processing ability of intelligent analysis technology, let intelligent evaluation benefit stakeholders, avoid adverse effects from the quantitative analysis(Saura, 2021), and maintain educational thought as central in the application of intelligent technology. With problem-solving as the guide, dividing the roles between intelligent technology and humans in the knowledge teaching evaluation system must be further explored. Thus, the knowledge teaching evaluation system can move from "intelligence" to "wisdom."
This paper summarizes the debate on knowledge teaching evaluation system in colleges and universities in the past ten years, analyzes the factors restricting the further development of teaching evaluation, draws on performance management theory, and proposes a big data-supported knowledge teaching evaluation system with "multiple evaluations in one and four closed-loop steps" at three levels: the main body of the knowledge teaching evaluation system, evaluation dimensions, and evaluation links. The system proposes that, in a big data context, the knowledge teaching evaluation system needs to break the barriers between different data sources and different evaluation subjects and scientifically set evaluation indexes and their sources. The balanced performance concept needs to evaluate the teaching effectiveness, behavior, and teaching ability aspects based on past, present, and future perspectives. The knowledge teaching evaluation system needs to be standardized in performance planning, implementation, evaluation, and feedback. This evaluation system can fully use the objective data recorded by various intelligent terminals to enhance the reliability of the evaluation. It can also realize the unification of teachers' ability improvement and students' ability cultivation based on the causal relationship between teaching ability and behavior and effect. It can also regulate the knowledge teaching evaluation system at the management level to avoid unsatisfactory teaching performance. The evaluation system provides a systematic methodology for unifying the theory and practice of university knowledge teaching evaluation systems.
This study is funded by the Research Project on Higher Education Teaching Reform in Hebei Province, entitled "Research on Teaching Performance Evaluation System of College Teachers in the Context of Big Data", project no.2018GJJG207