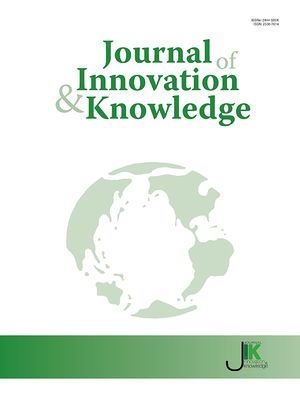
Editado por: Abbas Mardari
Más datosThis paper explores the impact of risk preference as well as interlinked credit and insurance contract on peasants’ new technology adoption. The results show that (1) risk preference has a significant impact on peasants’ adoption of innovative seeds, and the influence of exact probabilistic risk preference on peasants’ adoption of innovative seeds is greater than that of ambiguous probabilistic risk preference. (2) Interlinked credit and insurance contract has a significant positive impact on peasants’ adoption of innovative seeds, and interlinked credit and insurance contract plays a moderating role in promoting peasants’ adoption of innovative seeds. (3) Peasants’ education level, the degree of land fragmentation, the proportion of grain cultivation in the household-cultivated land, whether to provide meteorological disaster warning services, and the distance to financial services also have a significant impact on peasants’ adoption of innovative seeds. This interplay between collateral and the nature of credit-insurance interlinkage has direct and important implications for the design of programs to boost the adoption of innovative agricultural technologies simultaneously, and sheds light upon how the government can formulate relevant policies to promote innovative technological development.
Numerous agricultural technologies with demonstrated productivity gains, such as improved seed varieties, timely fertilizer application, agro-chemicals, and scientific use of pesticides, have not been widely adopted in developing countries (Jayne and Rashid, 2013; Genius et al., 2014; Ariel and Mushfiq, 2018; Takahashi et al., 2019). Wu et al. (2021) pointed out that in recent years, China approved about 8,000 agricultural scientific and technological achievements each year; however, only about 40% innovative technologies are successfully transformed into application, far lower than the 70%–85% transformation rate in developed countries. Many agricultural innovative achievements have been put aside, resulting in not only a huge waste of scientific research achievements but also a great obstacle to achieving China's food security strategy.
In recent studies, more and more researches have revealed that investing in new technologies is risky, which is a primary impediment to the adoption of improved agricultural technologies that could, to some extent, enhance agricultural production so as to boost the incomes of poor as well as small-scale households in developed and developing countries (Benyishay and Mobarak, 2014; Varshney et al., 2022). Meanwhile, agricultural production is strongly linked with the regional and seasonal environment, affected by natural conditions, therefore, extreme weather events, such as floods and droughts, can directly destroy productive assets that have accumulated at high opportunity costs through the years, which leading a great deal of rural households into the poverty traps (Barnett and Mahul, 2007; Dercon and Christiaensen, 2011; Ndegwa et al., 2020). An estimated two-thirds of population in developing countries, such as China, depend on agriculture and natural resources for their wellbeing (World Bank, 2007; Nicola, 2015; Tang and Luo, 2021), therefore, the risks related agriculture itself and natural environments hugely impact households’ choices.
While insurance mechanisms would seem to be a natural response to this problem of risk-inhibited technology adoption, an earlier generation of efforts to boost agricultural insurance in many countries collapsed under the influence of asymmetric information and transaction costs (Hazell, 1992; Barnett and Mahul, 2007; Bulte et al., 2020; Tessema, 2021; Park et al., 2022). In addition, uninsured borrowers may be deterred from taking on loans due to the risk of high default costs in states of the world where income is low, and the borrowers are unable to repay the loan. Binswanger and Sillers (1983) and Boucher et al. (2008) have emphasized so-called “risk rationing”. On the other hand, researchers believed that the credit support from financial mechanisms could largely mitigate the shortage of agricultural household funds and then promote their technology adoption (Giné and Yang, 2009). However, due to high natural risk and a lack of effective risk management tools, it is difficult for peasants to obtain credit support from formal financial institutions, which results in so-called “risk rationing” (Croppenstedt et al., 2003; Boucher et al., 2008; Amha and Peck, 2010). One obvious result is that high natural risks and credit constraints in agricultural production have severely inhibited agricultural technology adoption (Nicola, 2015; Nshakira-Rukundo, 2021).
In this context, some researches propose for a pilot application that involves the interlinkage of insurance and rural credit, which to some extent may expend on household technology adoption (Hill and Viceisza, 2012; Mobarak and Rosenzweig, 2014; Farrin and Miranda, 2015). However, different scholars put forward different views on the impact of interlinked credit and insurance contract (ICIC) on the adoption of new agricultural technologies. Some scholars find that due to the constraints of financial environment and imperfect insurance systems, small-scale peasants would reduce their investment in production activities with higher expected risks (Karlan et al., 2014; Ahmed et al., 2020). Compared with peasants who only buy agricultural insurance, ICIC can not only reduce the loan risk of financial institutions but also effectively help peasants to transform productive risk, which can be beneficial to enhance peasants’ adoption of new agricultural technologies (Tadesse, 2014). However, some scholars find that ICIC is not necessary to stimulate peasants’ agricultural investment and technological adoption. Compared with peasants who buy ICICs, peasants who only buy insurance contracts demonstrate higher new technology utilization rate, which may be related to the fears of defaulted loan. Meanwhile, current studies showed that the smaller the penalty of default is, the higher the level of peasants’ adoption of new agricultural technologies (Giné and Yang, 2009; Farrin and Miranda, 2015).
Regarding the ICIC in China, most researches mostly concentrate on its action mechanism, the impact of risk diversification on peasants as well as household income fluctuation. For instance, Liu et al. (2010) finds that the ICIC can effectively prevent information asymmetry caused by adverse selection; the risk-sharing between rural banks and insurance companies can solve moral hazards through interest binding and incentive compatibility, which could significantly alleviate the credit rationing of peasants. Carter et al. (2016) implies that the ICIC can effectively improve credit rationing for rural households, significantly increase rural households’ agricultural income, and effectively reduce the financial pressure on policy-based agricultural insurance premium subsidies. Carter et al. (2011) suggest that under the condition of ICIC, due to the improvement of the prices of the credit contract, the average net income of peasant households is higher than the average net income of peasant households under the conditions of uninsured and insurance-credit separation, therefore, the fluctuation of peasant households’ net income is reduced.
In the existing literature, the conclusion that ICIC can promote the adoption of innovative agricultural technology is controversial. Therefore, the goal of this paper is to examine the effect of ICIC on peasants’ innovative technological adoption in China. To do this, we put forward a model of a risk-averse household that is exposed to both idiosyncratic and covariant risk. The household chooses between a low-input, low-risk, and low-yielding technology versus a high-input, high-risk, and high-yielding technology. The technological choices of the households are examined in two perfectly competitive financial environments: In the control group, credit alone is available; In the treatment group, an ICIC is available. Within each contractual environment, we consider the impact of property rights regimes, ranging from those in which land is not mortgageable to loans that are fully collateralized. This paper adopts the field experiment method to empirically analyze the impact of ICIC on the adoption of peasants’ new agricultural technology, which not only provides a theoretical basis and decision-making reference for the popularization and utilization of new agricultural technology, but also enrich the existing research conclusions.
Compared with existing studies, this paper makes three academic contributions. First, because the implementation of innovative technology will be risky, and the benefits of new technology are uncertain, which result in the adoption of the new technology being the typical risky decision under the condition of uncertainty, which is known to contain both prior probabilities of risky decision-making and a mixture of unknown ambiguity probabilistic decisions (Halevy, 2007; Barham et al., 2014). uncertainty aversion tends to reduce the probability of technology adoption, while peasants tend to maintain the status quo (Lv et al., 2010; Barham et al., 2014; Keller et al., 2022). Based on this point of view, this paper further divides risk preference into definite probabilistic risk preference and ambiguous probabilistic risk preference and examines the influence of ambiguity aversion on peasants’ risk decision-making behavior, which is beneficial to enrich the researches on the mechanism of risk preference influencing peasants’ behavior.
Second, ICIC in China is still in the infancy, it is difficult to achieve enough statistical data to analysis, and the traditional questionnaire survey can only get the data at a certain time, not considering the dynamic change in weather and insurance claims, the “interaction” between banks and insurance companies as well as the relationship between the peasants’ technology adoption. Therefore, this paper tries to use the field experiment method to obtain data, and in this method, real peasants are chosen as experimental subjects. Meanwhile, the trading items, experimental tasks, as well as experimental information are all from real situations, which overcomes the problem of lack of external validity of laboratory economics. Therefore, we could believe that experimental results are closer to reality, and the conclusions are easier to be extended to the real world (Harrison and List, 2004).
Third, the impact of ICIC on peasants’ innovative technology adoption not only reflects in its direct effect but also reflects in its moderating effect by alleviating peasants’ risk aversion and credit rationing. However, there are few domestic studies on the impact of ICIC on the adoption of new agricultural technologies both considering direct and moderating effects, also no unified conclusion has been reached. Meanwhile, it is worthy to note that for some existing studies on ICIC in developing countries, most of which take African countries as samples, whose economic developments are quite different from the China's, resulting in weak guidance for China.
The rest of the paper is organized as follows. Section 2 explains the theoretical analysis framework. Section 3 introduces the experimental design, including risk preference and peasants’ technological choices. Section 4 is the data and methodology. Section 5 demonstrates the empirical results. Section 6 discusses the conclusions and policy implications of this paper.
Theoretical analysis frameworkThe impact of ICIC on peasants’ innovative technology adoptionPeasants are often faced with two choices when investing in production technologies: one is traditional low-risk technology that can be completed with their own funds. Another is high-yielding, high-risk innovative technology that requires additional investment besides household own funds. Supposed that peasants’ initial disposable wealth is W0, which just satisfies the requirements of adopting traditional technology. We assume the input cost of traditional technology isCT, and the innovative technology input cost isCI. At this circumstance, the capital that peasants who would like to choose innovative technology need to borrow from banks is L=CI−W0. If we assume the interest rate of the loan is rand if the peasants would like to get the loan, they should provide the collateralχ, andχ≥L(1+r).
It is assumed that all peasants possess fixed assets valuedχcan be mortgaged. And once the peasants default, the bank will confiscate their collaterals. The income from the agricultural technology investment income is affected by natural conditions, assuming the probability of disaster incidence isρ.
When peasants choose traditional technology, the expected utility function is:
When peasants choose innovative technology, the expected utility function is expressed as follows,
where, yT and yI are the benefits of selecting traditional technology and innovative technology under normal weather condition, respectively, and yTAccording to the hypothesis of economic rationality, only if UI>UT, will peasants choose innovative technology, namely:
For simplicity's sake, we reduce the weather condition in this paper to normal and disaster, and assume when a serious natural disaster occurs, the returns of investment concerning traditional and innovative technology are both 0, which cannot cover the cost, and peasants will lose collateral because they cannot repay the bank loans. Therefore, the expected utility of innovative technology is negative, which is smaller than the utility function of traditional technology. To avoid losing their collaterals and going bankrupt, peasants would be inclined to choose traditional technology rather than innovative technology.
According to equation (3), this paper assumes that the bank provides ICIC to peasants, that is peasants need to buy weather index insurance when borrowing money from banks, with the premium of m and the insurance compensation of T. In this condition, if the natural disaster occurs, insurance claims automatically repay bank loans. At this point, the insurance can play the role as collateral, and peasants do not need to provide additional collateral. Because peasants are required to pay the insurance premiums, the credit amount that the peasant needs is LI=CI+m−W0, with interest of the loan rI, and rI
Under the condition of ICIC, the expected utility difference between traditional and innovative technologies selected by peasants is expressed as follows:
where yI−LI×(1+rI)−m>yT. Therefore, when the weather index insurance compensation can repay the credit, peasants will not lose their collaterals. Then the expected utility of peasants choosing innovative technology is greater than that of traditional technology, that is, ΔU≥0. This implies that when peasants choose the ICIC, the insurance claim can be used to repay their credit in whole or in part if peasants suffer from natural disasters. The peasants’ plight is that they are unable to provide collateral and then they cannot enter the credit markets to invest in high-risk but high-premium innovative technology.Hypothesis 1 is proposed in this paper.
Hypothesis 1: The ICIC can effectively promote peasants’ adoption of innovative technology.
Agricultural production is significantly affected by great natural risks, and peasants’ risk preference has a significant impact on their agricultural production and investment behavior. Therefore, this paper learns from the research of Just and Pope (1978) and Hou et al. (2014), and introduces random production function as follows:
where x is vectors for various production inputs, a and β are the parameter vectors, εis the error term and is subject to independent co-distribution N(0,1). f(x;a)is the output function, and g(x;β)is the risk function. According to equation (6), peasants’ input will affect the yield, and the yield fluctuation is influenced by risk of agricultural technology investment. Therefore, changes in inputs affect the mean and variance of output at the same time. That is, E(y)=f(x;a), Var(y)=[g(x;β)]2. According to the different influence degrees and the direction of input on output variance, the input can be divided into three types: risk loving, risk-neutral, and risk averse. Marginal production risk can be expressed as:In equation (7), if ∂g(x;β)/∂xis positive (negative), indicating that the input x is a risk-loving (averse) type, which will enhance (reduce) the risk of the peasant's income fluctuation.
If the production function in equation (6) illustrated in Just and Pope (1978) is substituted into the utility function of peasants mentioned above, the utility optimization of peasants can be expressed as follows:
According to the above equation, the first-order condition satisfying utility maximization can be deduced as follows:
where, θ = E[U′E(W;z) × ε] /E[U′E(W;z)] is a risk-preference equation, and U′ = ∂U/∂W.Binswanger (1980) divided peasants into three types: risk aversion, risk neutrality, and risk preference. In Equation (9), the value of θ can be divided into less than, equal to, or greater than 0. Peasants with neutral risk, where θ is equal to 1, will choose inputs whose marginal output is equal to the ratio of input to output price. Generally speaking, peasants are risk-averse, that is, θ<0, then ∂f(x;β)/∂x Hypothesis 2 is proposed as follows, Hypothesis 2: Peasants who are risk-averse tend to choose traditional technology with low risk and low premiums, while those who prefer risk will choose innovative technology with high risk and high yields.
If the input vector x contains the premium of the ICIC, then this contract can protect the peasant's collateral from being confiscated by the banks and lead to production interruption when the peasant cannot repay the bank loans due to bad weather that results in no income. Therefore, the ICIC can effectively alleviate peasants’ risk aversion and credit constraints and improve the possibility of their choice of innovative production technology.
Therefore, Hypothesis 3 can be concluded as follows,
Hypothesis 3: The ICIC plays a moderating role in the impact of peasants’ risk preference on agricultural innovative technology adoption.
In order to obtain more real micro data of peasants’ risk preference, this paper measures peasants’ risk preference through experimental economics. In this paper, Holt and Laury's (2002) experimental scheme is appropriately simplified to ensure that respondents can understand it and effectively participate in the experiment positively. The risk experiment of this study will pay real money to all respondents, which can encourage respondents to complete the risk preference experiment truthfully and reduce the measurement error of risk preference. The whole experiment is carried out in four stages.
Stage 1: Test procedure. The testers introduce the rules of the game to the peasants and lets them understand the reward results and risk options. The focus of this stage is to let the respondents understand that the draw is random and the amount of reward depends on the respondents’ choice. In order to test whether the respondents are familiar with the rules of the game, the test game is designed as shown in Table 1, and the respondents are required to choose reward plan B before they can continue the game. Otherwise, the tester needs to explain the rules of the game to the peasants again. This setting helps ensure that the testers conduct risk measurement experiments on the basis of peasants’ totally understanding the rules of the game, which improves the accuracy of the measurement and provides a basis for screening invalid samples in the process of empirical analysis.
Stage 2: Formal test. After the peasants are familiarized with the experimental rules, testers provided 10 sets of test games, and each set of game includes low risk and high risk reward schemes, respectively. The peasants make risk choices for all 10 sets. That is, peasants will choose either plan A (low risk) or Plan B (high risk) from each of the 10 test games. The focus of the second stage is to let the peasants understand that the risk options in the face of them are directly related to the final premiums, which ensures that the risk preferences information displayed by peasants are authentic and reliable. At this stage, the study sets up two premises of exact probability and ambiguity probability to measure the degree of risk preferences of peasants, respectively.
We first measure the peasants’ exact probability. In this condition, the peasants are explicitly told that there are three red cards and three black cards, and ten sets of formal tests are set up (see Table 2). Then the peasants ’ ambiguity probabilities are measured. Here, participants are told there are six red and black cards in total, but they only know that there are more cards of one color than another one. In this case, tester will repeat the 10 sets of tests in Table 2.
Stage 3: Draw lots for rewards. The following, we will measure how much rewards that peasant could get. Peasants will be asked to select one of set that they are interested in, then the game will be implemented again and assign the rewards according to peasants’ choices. Among them, plan A is the “stable reward plan”, that is, peasants will get a stable reward of 20 Yuan if they choose plan A in this stage.
Stage 4: Validation test. In order to reconfirm that peasants complete the above tests with a correct understanding of the rules, a validation test is set up (see Table 3). If the peasants choose plan A, it proves that the peasants has correctly understood the rules and the above tests are valid.
According to the peasants’ actual selections, the risk preference index are calculated as follows: the risk preference index under exact probability is defined as the number of plan B selected in Table 2 divided by 10 when the peasants are told the colored cards’ distribution, and the risk preference index under ambiguous probability is defined as the number of plan B selected in Table 2 divided by 10 when the distribution of colored cards are unknown. Therefore, the risk preference index, no matter the distribution of colored cards is known or not, ranges from 0 to 1. The peasant's risk preference index under exact probability is equal to 1, which indicates that peasant is extremely risk loving under exact probability; when the risk preference index under ambiguous probability is 0, we can know that the peasant is extremely risk-averse under ambiguous probability.
The choice of agricultural technologyTechnology optionsPreliminary researches show that agricultural innovative technologies in the sampling areas mainly include new seeds, new fertilizers and pesticides. Among them, seeds have the greatest impact on crop yield, and peasants always pay the most attention to them. Therefore, this paper chooses innovative seeds to represent new technology. The assumption is that peasants are faced with two seeds options at the beginning of the period: traditional seeds and innovative seeds. It is obviously demonstrated in Table 4 that there are significant differences between the two seeds. In terms of traditional seeds, due to its low risk and low yield, peasants can satisfy themselves by their own funds. The failure of peasants in agricultural production this year will not significantly impact on production in the next year. Given that innovative seeds can bring high risk and high yield, peasants need loans in addition to their own funds, if bad weather occurs, the direct result for peasants is forfeiture of the loan collaterals, then bankruptcy.
Comparison of traditional and innovative seeds
The scientific field experiment is firstly based on the allocation of a control group and a treatment group. The way to solve this problem in a field experiment is to divide the sampling peasants into experimental treatment group and control group randomly in order to ensure that there is no significant difference between the two groups of participants. Then, we can compare the performance of peasants in two groups, determining the causal effect of the intervention on the performance of peasants.
Therefore, we randomly divide the peasants into control group and treatment group, respectively. The peasants in the control group are faced with two choices: the traditional seeds, which is not restricted by own funds, and the innovative seeds, for which loans are necessary. However, the peasants in treatment group are faced with two choices: the traditional seeds, which is not restricted by own funds, and the innovative seeds, which is supported by an ICIC instead of agricultural insurance. Boucher et al (2008) point out that the natural risks of agricultural production as well as the liquidity constraints of peasants can not only impact supply-side credit rationing, but also demand side risk-rationing, therefore, it is indisputable that peasants are often excluded from the formal credit market due to their low credit rating and lack of collateral (Farrin and Miranda, 2015), which hinders the adoption and promotion of new technologies. This paper attempts to analyze the impact of the external intervention by observing the behavior differences of peasants through a field experiment to analyze whether providing ICIC to peasants can alleviate credit risk rationing and then promote the adoption of new technologies.
Production condition settingTo simplify the experiment, it is assumed that peasants possess the same resources endowment, that is, the initial capital of 4,200 Yuan and 10 Mu of lands. The cost of living is assumed to be the same for all peasants, assuming it can be ignored. Weather conditions are reduced to two categories: disaster and normal. According to the local meteorological data and the probability of disaster occurrence, the probability of disaster weather in the experiment is determined to be 1/3. To simulate the real production environment, before the end of each round, the peasants decide the weather condition they encounter in this round by drawing lots. The peasants need to choose the seeds variety at the beginning of the year: No. 1 is the seeds that has been planted for many years with low cost and low yield; No. 2 is innovative seeds with a relatively high cost and high yield. It is highly affected by weather, and the loss under the bad weather is 1.55 times that of traditional seeds. Peasants do not know what the weather is like when they make their decisions, so it is a risky decision.
The production input and output concerning these two kinds of seeds are different under different weather conditions (see Table 5): If peasants choose the No. 1 seeds, their own capital is enough; if they choose the No. 2 seeds, peasants need to apply for a credit of 1,800 Yuan from local banks. At the same time, peasants are required to submit a pledge of 1,800 Yuan (assuming the peasants can provide it). At the end of the year, if the year's temperature and rainfall conditions are good for crop growth (good weather), the peasants who planted the No. 2 seeds will get the collaterals back after paying the credits. However, if the weather is bad, the peasants who planted No. 2 seeds could not repay the loan due to lack of yield from farming, and the bank would confiscate the mortgage; that is to say, it would lose 1800 Yuan in the end. In this case, the tester recorded a reward of −1800 Yuan.
Peasants’ technological choices of in the treatment group and control group Unit: number of peasants
Note: CG means Control group, TG represents treatment group.
The background of the treatment experiment and the input and output of crop seeds are the same as that of the benchmark experiment (see Table 5). The only difference is that peasants need to buy an additional weather index insurance with a premium of 200 Yuan. Therefore, the peasants who choose the No. 2 seeds also need to borrow 2000 Yuan and supply collaterals worthy of 2000 Yuan. If encounters bad weather, the bank can get an insurance payout of 2,000 Yuan instead of confiscation of peasants’ collaterals. At the end of the year, if this year is good for crop growth, peasants will get a satisfactory output, then peasants will have a great harvest.
Before the experiment, peasants in the treatment group first play a small game about the “ICIC” to help them understand the differences between “credit plus insurance” and traditional credit, then examine whether the peasants fully understand the product through several multiple-choice questions. If the peasants still do not understand it fully, the tester will continue to explain it in detail until peasants understands the product totally.
In order to maintain the accuracy of experimental results, each tester is responsible for four subjects and separates them with partitions, preventing peasants from influencing each other by communicating, which could to some extent ensure peasants’ independent decisions. Before the experiment, a unified experiment instruction manual is prepared to ensure that each subject received the same information. The tester is required to use neutral words when explaining the experimental instructions to avoid the demand effect caused by the tester's suggestion.
Collection of basic informationAfter the experiment, the peasants are invited to complete the questionnaire survey to obtain the personal characteristics of household heads, the household characteristics, their participation in technological trainings, and whether they had suffered from natural disasters and losses. The whole experiment lasted about 80 minutes. After all the experiments, the peasants get corresponding rewards (the sum of the rewards from the risk experiment and the rewards from technological choice).
Data and methodologyData specificationClimatic conditions and agricultural production conditions vary greatly in different regions of the China. The sampling areas selected in this paper are Yongshou County of Xianyang City and Heyang County of Weinan City in Shaanxi Province, as well as Anxiang County of Changde City in Hunan Province. The reasons for selecting these areas are as follows: First, Shaanxi province and Hunan Province are important wheat and rice producing provinces in China, respectively, among which Yongshou county and Heyang County are major wheat-producing counties in Shaanxi Province, and Anxiang county is a major rice producing county in Hunan Province. Second, the region has the international leading edge in original rice technology, especially Hunan, which is the center of rice breeding and cultivation in China, and has a good technological environment. Third, Small peasants are the main organizational form of grain production in China, and Shaanxi and Hunan are typical provinces where small households account for a large proportion in the rural areas. Households’ technology adoption behaviors in Shaanxi and Hunan provinces can better reflect the situation of peasants' land technology adoption in China. Fourthly, Yongshou, Heyang and Anxiang counties are short of water resources, water-saving crop varieties are the key agricultural production technology developed in these areas, so water-saving crop varieties have received widespread attention by peasants.
The research group collected a total of 720 questionnaires in the July and August, 2021, of which 688 are valid, with an effective rate of 95.56%. The contents of the questionnaire mainly included the basic characteristics of peasants’ households, cultivated land characteristics, the cultivated land planting situation, innovative technology adoption, financial assets and liabilities, risk experiments, etc..
Variables design and descriptive statistical analysisThe dependent variableThe dependent variable is the adoption of innovative seeds by peasants. If peasants choose traditional seeds, then the value of choice is 0, while that of innovative seeds is 1. The experiment is carried out in two rounds in the control group and the experimental group, respectively. There are also two rounds of results on the adoption of crop seeds by peasants. In order to preliminarily understand the distribution of technology choices of peasants in different groups and different experiment rounds, this paper first carries out a descriptive statistical analysis of the outcome of technological choice by peasants.
Table 5 shows that 688 sampling peasants participate in the first round of experiments, including 344 peasants in the control group and 344 peasants in the treatment group. In the control group, the proportion of peasants choosing traditional seeds is 42.02%, and that in the treatment group is 32.85%, with 9.17% lower than that in the control group, indicating that more peasants in the control group are unwilling to choose innovative seeds due to the dual pressure of natural risk and credit rationing. in order to simulate the real production situation, an exit option is set up after the first round of the experiment. Table 5 shows that after the first round of experiment, 18 peasants in control group withdraw agricultural production, and 15 peasants in control group exit agricultural production. This result demonstrates that even encountering bad weather and suffering loss, most peasants continue to their agricultural production, which further proves that peasants are highly dependent on agricultural production.
In the second round of experiments, 213 peasants in control group choose innovative seeds, accounting for 65.34% of the total sampling peasants. In treatment group, 76.06% of peasants choose innovative seeds, 10.72% higher than that in control group. Combining the experimental data of the two rounds, it can be preliminarily demonstrated that the ICIC is beneficial to improve peasants’ risk resistance ability and enhance peasants’ willingness of adopting innovative seeds.
The core explanatory variablesThe ICICThe intervention effect of ICIC can be defined as 1 for the treatment group and 0 for the control group.
Risk preferenceAnother core explanatory variable in this paper is risk preference, which includes both risk preference under exact probability and risk preference under ambiguous probability, according to the distribution of cards of different colors is known or not. Meanwhile, risk preference is a number within the range of [0,1], and the peasants’ risk preference is shown in Figure 1.
We can see that regardless of exact or ambiguous probability, most frequencies of peasants’ risk preference are concentrated at 0, indicating that these peasants are extremely risk-averse. In order to facilitate peasants’ understanding of the experiment, the experimental scheme is appropriately simplified in this paper. Basically, peasants can steadily get 20 Yuan, and this setting has incentive compatibility for each peasant. That is to say, peasants participating in the experiment will get a different-sized reward with a 100% possibility, which further stimulates peasants to choose the stable reward program. This conclusion is similar with the findings of Liu (2013) and Camerer et al. (2010), which reveal a high proportion of peasants continue to choose plans that can obtain stable rewards. In figure 1, the number of peasants with a risk preference index lower than 0.5 is significantly higher than that with a risk preference index higher than 0.5, indicating that most peasants have a low degree of risk preference either in the case of exact probability or ambiguious probability, that is, most peasants are risk-averse. The average risk preference index for peasants is 0.35 under exact probability and 0.28 under ambiguous probability, which implies that in the face of high uncertainty, peasants show stronger risk aversion. This is consistent with the “ambiguous aversion” proposed by Ellsberg (1961) and Qu and Cai (2018), who proposed that in the case of uncertain probability, people tend to demonstrate an aversion to ambiguous things.
Controlled variablesAccording to existing relevant literature (Li et al., 2017; Gao and Niu, 2019), this paper selects the other factors that affect peasants’ adoption of new technology as control variables, including the individual characteristics (peasant's age, gender, level of education, village cadres), household production and business operation characteristics (annual household income, household size, degree of land fragmentation, the ratio of crop planting area accounting for household lands), government support (technological training, technical information services for disaster prevention), and village characteristics (distance to nearest financial institution, distance to county).
Definition and descriptive statistics of variablesTable 6 shows the definition and descriptive statistical results of each variable. Among the 688 peasants in the first round of the experiment, 432 peasants adopt innovative seeds, accounting for 62.79% of the sampling peasants. Among the 655 peasants in the second round of the experiment, 464 peasants adopt innovative seeds, accounting for 70.84% of the sampling peasants. After the first round of experiments, peasants’ enthusiasm to choose innovative seeds is improved significantly. About 67.44% of the sampling peasants are male, aged about 58 years old, with an average of 7.28 years of education. Only 13.08% of the households have village cadres. The annual household income of samplings is about 47,400 Yuan, and the family population is about 4 people. The average number of crop plots are 2.75, which are relatively scattered. The crop planting area accounts for about 72.88% of the total household land area. Peasants’ enthusiasm to participate in crop planting technology training is generally not high, the data in Table 6 implies that peasants in common attend the trainings less than once a year. About half the sample villages provide meteorological service support for peasants. The distance from the village to the nearest financial service institution is about 2.6 KM, and the average distance from the county center is 16.18 KM.
Definition and descriptive statistics of variables
Due to the small scale of ICIC in China, if the questionnaire survey is adopted, the samples who adopted the ICIC will be not enough for analyzing, and only some data can be obtained at a certain point. It is difficult to dynamically investigate the relationship between ICIC and the adoption of agricultural technology. Therefore, this paper employs field experiment to obtain data concerning ICIC and innovative seeds adoption. From the perspective of research methods, the core concept of field experiment is to use experimental operation skills (such as randomizing subjects or controlling related variables) to evaluate the treatment effect of intervention events or the causal effect between variables. In other words, the field experiment uses scientific experimental methods to test the causal effect that happened in the real world, rather than the disturbance in the laboratory caused by people's decision-making. This can provide a strong empirical basis for economic theory research.
The basic operation method of a field experiment is to randomly select the samples from a population and then randomly divide the subjects into the control group and treatment group. According to the data comparison between the two groups, the final causal effect is obtained. Because the subjects are randomly divided into two groups, the treatment group is completely independent on the individual characteristics and other factors that may affect the experimental results.
According to the experimental design, the dependent variable in the first round is a binary selection between innovative seeds and traditional seeds. To examine the impact of ICIC and risk preference on peasants’ technological adoption, this paper first builds a binary Probit model to analyze it. The basic model is as follows.
Choice1 represents the peasant's choice of seeds in the first round of the experiment. If the peasant chooses innovative seeds, then the value is 1; otherwise, the value is 0. Interlink represents the treatment effect, that is, the influence of ICIC, which means the influence of ICIC on peasants’ technology adoption. Here, the value of the control group is 0, and that of the treatment group is 1. Risk represents the risk preference of peasants and X is the vector of control variables. The coefficientsα, β, and γi are the estimated values and vector of the regression coefficient, respectively, and λ(•) is the probability function of normal distribution.
In the first round of experiments, peasants who choose the innovative seeds may encounter bad weather resulting in bankruptcy, and be unable to carry out agricultural production continuously. Given this situation, before the second round of the experiment, we provide an exit option for sampling peasants, that is, peasants could choose to get out of agricultural production after the first round of production. Based on this, there are still two technology choices among peasants who continue to carry on agricultural production in the second round. Therefore, the Probit model is still used to further test whether the influence of ICIC on peasants’ technology adoption is strengthened and whether the influence of risk preference is reduced under the effect of ICIC. The basic model is as follows:
where Choice2represents the peasant's choice in the second round of the experiment and Weathermeans the weather condition encountered by peasants in the first round, which includes good weather and bad weather.ResultsIn this part, two rounds of experimental data are used to analyze the impact of risk preference and ICIC on peasants’ technology adoption, respectively.
Probit regression of first round dataBaseline regressionIn this paper, the Probit model is first used to analyze the impact of risk preference, ICIC, and related variables on peasants’ technology choice in the first round of the experiment, the results are shown in Table 7. The regression coefficient of exact probabilistic risk preference and ambiguous probabilistic risk preference on peasants’ technology adoption are highly significant at the 5% level, and the coefficients are both significantly positive, indicating that the higher the exact probabilistic risk preference and ambiguous probabilistic risk preference degrees of peasants, the higher the possibility of adopting new technology. As can be seen from the marginal effect regression results, the probability of adopting new technology will increase by 0.125% and 0.118% for every 1% increase in the risk preference of exact probability and ambiguous probability. Peasants with an adventurous spirit are more willing to choose high-risk, high-premium technology. This is consistent with the studies of Giné and Yang (2009) and Farrin and Miranda (2015). Their researches find that risk-averse peasants tend to choose informal risk management methods, such as loans from friends, household deposits, and other risk management methods, and they have weak risk tolerance and are unwilling to afford the risk impact brought by production technology investment. Here, hypothesis 1 in this paper is verified.
Parameter estimates of the Probit model in the first round of the experiment
***, **, and * indicate significance at 1%, 5%, and 10%, respectively; figures in parentheses are standard errors; ME is the marginal effect.
By comparing peasants’ regression results for new technology adoption under the condition of exact probability and ambiguous probability in Table 7, in the marginal effect regression results, it can be found that the absolute value of the coefficient of exact probability risk preference is greater than that of ambiguity probability risk preference, which is mainly caused by the characteristic of the “ambiguity aversion” of peasants. The difference between exact probabilistic risk preference and ambiguous probabilistic risk preference is that the latter includes peasants’ attitudes toward uncertain probability (i.e., ambiguous situations). The probability distribution of adopting new technology to improve yields is ambiguous, so peasants tend to choose “maintaining the status quo”. This adds realistic evidence to the peasants’ ambiguity aversion.
Meanwhile, the regression coefficient of ICIC is significant and positive at the 1% level, indicating that ICIC has a significant positive impact on peasants’ technology adoption. If the probability of the sampling peasants buying an ICIC increases by 1%, the probability of adopting new technology will increase by 0.123%. This is because, on the one hand, the ICIC is beneficial for reducing credit rationing, to help peasants enter the credit market, and to alleviate the capital constraints faced by peasants when they invest in new technology. On the other hand, it provides peasants with a means to disperse risks, and it reduces the risks of the investment in new technologies, thus promoting the adoption of new technologies. The study of peasants in Africa by Farrin and Miranda (2015) found that peasants’ willingness to adopt new technologies increased after they are provided with risk management means. The regression results of this study verify that when ICIC is provided, the insurance claim can repay the loan in whole or in part, reducing peasants’ risk of losing the mortgaged property. It can solve part of the plight of mortgaged property the peasants cannot provide and reduce the risk of peasants’ self-selected ration. This can encourage peasants to enter the credit market and get a loan, promoting peasants’ innovative technology adoption. Hypothesis 2 in this paper is supported.
Among the control variables, whether there are village cadres among household members has a significant positive impact on peasants’ technology adoption. This may be due to two reasons. On the one hand, village cadres generally have a relatively high level of education and have a stronger ability to accept new things, which also confirms that the education level of peasant has a significant positive impact. Second, village cadres in rural areas always possess stronger social capital, and their ability to obtain resources and information is more advantageous than that of ordinary peasants.
The larger the proportion of crop planting area in the total household's cultivated land area, the greater the importance of wheat/rice planting in the peasants’ agricultural production, and the stronger the willingness of adopting new technologies. At the same time, the enthusiasm of peasants to adopt new technology is also significantly higher in households if we provide positive meteorological information and technology services for peasants. This is because providing positive meteorological forecasts and prevention and response measures for peasants can significantly improve the risk response-ability of peasants, which enhances the probability of adopting new technology.
Robust checkIn order to further check the robustness of the above empirical analysis results, this paper conducts robustness tests on the benchmark regression results in Table 8 by changing the model form (replacing the Probit model with a Logit model) and by adding explanatory variables. Researchers indicates that risk perception significantly correlates with the decision to purchase agricultural insurance, positively affecting peasants’ technological adoption (Petrolia, et al., 2013; Qiu et al., 2020). Therefore, we introduce the question, “Do you think there has been a trend of global warming in the past 30 years?” We used a 5-point scale, with 1 being strongly disagreeing and 5 being strongly agreeing. From the Table 8, compared with the baseline regression results, the results are consistent in both the significance of the variables and the coefficient signs. The results of the robust tests all support the positive influence of exact probabilistic risk preference, ambiguity probabilistic risk preference, and ICIC on peasants’ technology adoption, and the positive influence of risk preference and ICIC on peasants’ innovative technology adoption, thus Hypothesis 1 and Hypothesis 2 are supported further.
Robust test of the impact of risk preference and ICIC on peasants’ technology adoption
***, **, and * indicate significance at 1%, 5%, and 10%, respectively; figures in parentheses are standard errors; ME is the marginal effect.
This paper uses the method of grouping regression to examine the moderating effect of ICIC on risk preference on peasants’ technology adoption behavior (Wen et al., 2005). The regression results in Table 9 reflect the influence of exact probabilistic risk preference and ambiguous probabilistic risk preference, respectively, on the adoption of innovative seeds by peasants whether or not they are provided ICICs. The results show that when ICIC is not provided, the influence of exact probabilistic risk preference on peasants’ adoption of innovative seeds cannot pass the test at the level of 10% significance. However, when ICIC is provided, the impact of exact probabilistic risk preference on the adoption of innovative seeds is significant at the 10% significance level, and the coefficient is positive. At the same time, the regression results also reflect the influence of risk preference under ambiguous probability on the adoption of innovative seeds by peasants in the conditions of providing ICIC or not. The results are similar to those under the condition of exact probability. That is, when an ICIC is provided, the ambiguous probabilistic risk preference of peasants passes the test at the significance level of 10%, and the coefficient is positive. Therefore, hypothesis 2 is supported again.
The effect of risk preference on technology adoption under different interlink effects
***, **, and * indicate significance at 1%, 5%, and 10%, respectively; figures in parentheses are standard errors; ME is the marginal effect.
This paper focuses on whether peasants are more willing to choose new technologies under the scenario of “ICIC” .The purpose of the second round of the experiment is to verify the mitigating effect of ICICs on peasants’ risk preferences. In addition, technology selection and weather condition in the first year are important influencing factors for peasants when choosing the technology in the second year (Tang et al., 2019). Therefore, these factors are introduced into the regression model as control variables, and the regression results are shown in Table 10.
Parameter estimate of the Probit model in the second round of the experiment
***, **, and * indicate significance at 1%, 5%, and 10%, respectively; figures in parentheses are standard errors; ME is the marginal effect.
Table 10 regression results show that under the condition of exact probability risk preference, ICIC have a significant impact on peasants’ selection of innovative seeds, and their regression coefficients have a positive impact on peasants’ innovation technology adoption behavior at the 5% level of significance. From the perspective of marginal effects, for every 1% increase in the probability of peasants buying ICIC, the probability of choosing innovative seeds will increase by 0.065%. However, compared with the first round of experiment, the influence degree and significance level of exact probabilistic risk preference on peasants’ innovation seeds selection decreases, indicating that the introduction of ICICs mitigated the negative impact of risk aversion on peasants’ innovation technology adoption. This conclusion is consistent with the research results of Carter (2011), Tadesse (2014), and Farrin and Miranda (2015), who find that ICIC can achieve a balance between the two goals of reducing natural risk and the credit default rate.
Meanwhile, the sustainability of the rural credit market can be ensured. Therefore, ICICs can not only effectively disperse risks but also help alleviate peasants’ credit constraints, which further verifies hypothesis 1. What's more, technology choice in the first round has a significant positive impact on a peasant's choice of innovative technology at the level of 1% significance, which indicates that peasants who choose innovative seeds in the first round are more inclined to choose innovative seeds in the second round of experiment. The reason is that peasants who choose innovative seeds achieve a higher income than peasants who choose traditional seeds under normal weather conditions, and they are still willing to choose innovative seeds in the second round of expriment. Peasants who choose traditional seeds in the first round and encounter bad weather may choose innovative seeds under an ICIC in the second round of expriment.
Another thing to note, under the ambiguous probability condition, the regression results of ICIC and risk preference on the adoption of innovative seeds by peasants show that the significance level and coefficient symbols of variables are basically the same except for the difference in coefficient size. This further verifies hypothesis 2.
The robust testIn order to further test the robustness of the above empirical analysis results, this paper conducts robustness tests on the benchmark regression results in table 11 by changing the model form (replacing the Probit model with the Logit model) and adding explanatory variables. Just as with the robustness test in the first round of experiment, we introduced the question, “Do you think there is a trend of global warming in the past 30 years?” We used a 5-point scale, with one being strongly disagreeing and five being strongly agreeing, and the results are shown in Table 11. Compared with the baseline regression results, the results are consistent with both the significance of the variables and the coefficient sign. The results of the robustness test all support the positive influence of exact probabilistic risk preference, ambiguous probabilistic risk preference, and ICIC on peasants’ technology adoption and the positive influence of risk perception on peasants’ technology adoption. Thus, hypothesis 1 and hypothesis 2 are once again established.
Robust test of the impact of risk preference and ICICs on peasants’ technology adoption
***, **, and * indicate significance at 1%, 5%, and 10%, respectively; figures in parentheses are standard errors; ME is the marginal effect.
In the second round of experiment, when examining the ICIC moderates the effect of risk preference on peasants’ technology adoption behavior, this paper still uses the method of grouping regression. That is, the impact of risk preference on peasants’ household technology adoption behavior in the presence and absence of ICIC, respectively. The results are shown in Table 12. If there is no ICIC provided, the influence of exact probabilistic risk preference on peasants’ adoption of innovative seeds cannot pass the test at the 5% significance level. When ICIC is provided, the impact of exact probability risk preference on the adoption of innovative seeds is significant at the significance level of 1%, and the coefficient is positive. However, the regression results also reflect that the ambiguity probability risk preference has no significant impact on the adoption of innovative seeds whether ICIC is provided or not.
The effect of risk preference on technology adoption under different interlink effects.
***, **, and * indicate significance at 1%, 5%, and 10%, respectively; figures in parentheses are standard errors; ME is the marginal effect.
Agricultural technology is an important driving force for the development of modern agriculture. The No. 1 document of the Communist Party of China Central Committee in 2017 called for “strengthening agricultural science and technology research and popularization, leading modern agriculture to accelerate development” . Peasants are the final carriers of agricultural technology transformation into productive forces; therefore, only when advanced agricultural scientific and technological achievements are adopted by peasants can they be transformed into productive forces and put into agricultural production. However, most peasants are risk-averse and tend to choose low-risk and low-premium technologies due to low incomes, limited resources, and inability to resist risks, which is a fundamental reason why peasants in developing countries easily fall into the poverty trap (Dercon et al., 2011). The application of new agricultural technologies in agricultural production is beneficial to improve agricultural productivity, promote agricultural economic growth, and enhance peasants’ income. However, China's agricultural technology conversion rate is only 35%–50%, far lower than 75%–80% in developed countries, and some advanced and applicable agricultural technologies have not been effectively promoted.
Insurance replaces collateral to some extent, and the insured peasants can enter the financial market and obtain the funds needed for technological investment, which promotes the adoption of advanced technologies by peasants. This can improve the income and welfare level of peasants and help eliminate poverty and income inequality (Ghosh et al., 2002). According to the theoretical analysis (Ghosh et al., 2002), agricultural insurance, as a vital risk management tool, improves the risk tolerance of peasants by dispersing risks in time and space. Peasants are more willing to allocate resources to risky technological investments, thus improving the allocation efficiency of resources and achieving Pareto optimality. The research results of this paper further confirm that providing ICIC for peasants can influence peasants’ production and investment behaviors to a certain extent and promote peasants’ adoption of new technologies.
Based on the field experiment with 688 wheat peasants in Shaanxi and rice peasants in Hunan provinces, this paper focuses on the impact of risk preference and ICIC on peasants’ adoption of innovative agricultural technologies. The conclusions are as follows:
Risk preference has a significant and positive impact on peasants’ adoption of innovative technologies. Most peasants are risk-averse, the higher the degree of risk preference, the higher the probability of adopting innovative agricultural technologies. The influence of exact probabilistic risk preference on peasants’ adoption of innovative seeds is greater than that of ambiguous probabilistic risk preference, which verifies that peasants are inclined to “ambiguity aversion.” Risk aversion and ambiguity aversion are significant barriers to technology adoption by peasants. It is necessary to take effective measures to reduce the risk aversion and ambiguity aversion of peasants, which is consistent with the research results of Carter (2011), Tadesse (2014), and Farrin and Miranda (2015).
ICIC has a significant positive impact on the adoption of innovative technologies. This can effectively promote the adoption and promotion of new agricultural technologies. Meanwhile, ICIC positively impacts risk preference on peasants’ adoption of innovative seeds. Specifically, risk preference has a significant positive impact on the adoption of innovative seeds when peasants are provided with ICIC. However, risk preference has no significant effect on the adoption of innovation seeds if the ICIC is not provided. Although ICIC is still in the early stage of development in China, it is necessary to promote it on a large scale in major grain producing areas, which coincides with the conclusion of Tang et al. (2019).
In addition, the education level of peasants, the degree of land fragmentation, the proportion of crop cultivation in the household-cultivated land, whether to provide meteorological disaster warning services and the distance to financial service institutions also have a significant impact on a peasant's adoption of innovative agricultural technology.
Policy implicationsAccordingly, this paper puts forward the following policy implications:
Considering that many peasants are risk averse, it is important to improve peasants’ understanding and identification of innovative technologies, which is helpful to reduce the ambiguity of peasants’ cognition of innovative technologies. Meanwhile, the government should focus on the development of technological demonstrations, technological assistance, and other services, and enhance peasants’ trust and confidence in agricultural innovative technologies.
Promoting the integration of agricultural insurance and rural credit market is an essential way to alleviate peasants’ credit constraint. ICIC can remit peasants’ risk aversion and credit rationing caused by high natural risks, so that peasants have relatively sufficient confidence and funds to invest in innovative technologies. At present, the promotion scope of this model in China is small, and the two markets are obviously divided. The government should adopt an open attitude, learn from the successful experience of other countries, promote pilot reform.
Agriculture is an industry greatly affected by natural risks, providing peasants with timely technology services, such as meteorological disaster consultations, early warning, prevention measurements, will play positive roles in promoting agricultural innovative technology.
Finally, the study has some limitations concerning the data collection. Firstly, the design chosen for measurement of peasants' risk preference imposes some limitations that are worth noting. For instance, we did not give enough consideration of experimental design in money variation, due to constraints of research funding, which is proved be important to change household uncertainty preference (Barham, 2014). On the other hand, ICIC is not widely promoted in the sample areas, and peasants have no sufficient understanding and perception concerning to ICIC only through testers’ explanation. As a result, peasants’ real selections may be different from the circumstances in experimental simulation, damaging the accuracy of experimental results.
This research was funded by “National Natural Science Foundation of China General Program “Economic Incentive, Peer Effects and Green Fertilization Behavior of Wheat Growers: A Randomized Controlled Trail”, grant number “71973087”; National Natural Science Foundation of China Youth Fund Project “Effects of Incentive Heterogeneity on Agricultural Technology Extension: A Randomized Controlled Experiment in Northern Wheat of China”, grant number “72003215”; Modern Technology System of Agricultural Industry “Economic Post Scientist Project in Rice Industry”, grant number “CARS-01-53”; Project of Ministry of Agriculture and Rural Affairs “The Analysis and Early Warning of Rice Market”, grant number “11220010”; Soft Science Project of Science and Technology Department of Shaanxi Province “The impact of Incentive Heterogeneity on the Extension of Green Agricultural Technology: A case study of Wheat Water and Fertilizer Integration Technology in Weihe Plain”, grant number “2022KRM131”; The Special Fund project of Basic Scientific Research Operation funds of Central Universities “The Influence of Peer Effect on Farmers’ Green Fertilization Behavior: Based on the Analysis of the Randomized Controlled Experiment of Winter Wheat in Fen-Wei Plain”, grant number “20SZYB21”.