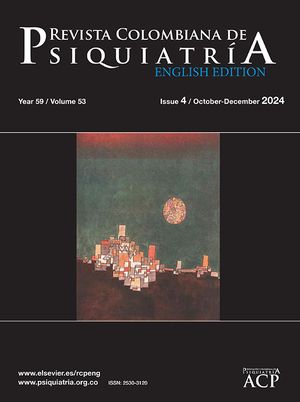
This systematic review summarises the existing evidence on the implementation of technology-based mental healthcare models in the primary care setting.
MethodsA systematic search was conducted (MEDLINE, Embase, CENTRAL) in August 2019 and studies were selected according to predefined eligibility criteria. The main outcomes were clinical effectiveness, adherence to primary treatment and cost of implementation.
Selection criteriaStudies with an experimental or quasi-experimental design that evaluated the implementation of technology-based mental healthcare models were included.
ResultsFive articles met the inclusion criteria. The models included technological devices such as tablets, cellphones and computers, with programs and mobile apps that supported decision-making in the care pathway. These decisions took place at different times, from the universal screening phase to the follow-up of patients with specific conditions. In general, the studies showed a decrease in the reported symptoms. However, there was great heterogeneity in both the health conditions and the outcomes, which hindered a quantitative synthesis. The assessment of risk of bias showed low quality of evidence.
ConclusionThere is not enough evidence to support the implementation of a technology-based mental healthcare model. High quality studies that focus on implementation and effectiveness outcomes are needed to evaluate the impact of technology-based mental healthcare models in the primary care setting.
Esta revisión sistemática resume la evidencia existente sobre los modelos de implementación basados en tecnologías para el abordaje de la salud mental en el contexto de la atención primaria.
MétodosSe realizó una búsqueda sistemática en las principales bases de datos electrónicas (MEDLINE, Embase, CENTRAL) en agosto del 2019 y se eligieron estudios según criterios de selección predefinidos. Los desenlaces principales fueron efectividad clínica, adherencia al tratamiento y costos de implementación.
Criterios de selecciónSe incluyeron estudios con diseños experimentales o cuasiexperimentales que evaluaran la implementación de modelos basados en tecnologías para el abordaje de la salud mental.
ResultadosCinco artículos cumplieron con los criterios de selección. Los modelos incluían dispositivos tecnológicos como tabletas, celulares y computadores con programas y aplicativos móviles que ayudaban con la toma de decisiones en la ruta de atención. Estas tenían lugar en diferentes momentos, desde el tamizaje a la población general, hasta el seguimiento de los pacientes con alguna condición específica. De forma general, en los estudios se evidencia una disminución de los síntomas reportados, sin embargo, tanto las condiciones de salud como los desenlaces muestran gran heterogeneidad, lo que impidió realizar una síntesis cuantitativa. La evaluación del riesgo de sesgo mostró baja calidad de la evidencia.
ConclusiónNo hay suficiente evidencia para determinar la superioridad de un modelo de atención optimizado por tecnologías para el abordaje de la salud mental. Es necesario realizar estudios primarios con una adecuada calidad metodológica que evalúen desenlaces relevantes de implementación y efectividad.
Mental health is essential to well-being; nevertheless, low-income and medium-income countries not only lack trained staff, management guidelines and infrastructure,1–3 but also face barriers such as inequities in resource distribution.4,5 Hence, international organisations such as the World Health Organization (WHO) and the Pan American Health Organization (PAHO) have made proposals such as the Mental Health Gap Action Programme (mhGAP)6 in order to raise awareness of mental health. Some countries have leveraged these strategies to launch national care plans specifically for mental health conditions. One example in Latin America is Chile, which has a national model for detection, diagnosis and treatment of depression based on technology use.7
The use of technological tools for health is more than a growing trend around the world. It has demonstrated its potential to improve the efficiency of the use of mental health resources.8,9 This is primarily due to its contributions to breaking down barriers to access to services, increasing coverage, making treatments more flexible, decreasing perceived stigma and increasing user commitment, among other things. Technology may be involved at different points in care (screening, diagnosis, treatment and follow-up) in both primary care and specialised medicine settings.10,11 Some examples of such integration are currently under development in Colombia.8,10
Although the use of technologies has proven quite effective in the management of mental health disorders, these tend to be insufficient interventions when made in isolation from other elements of care.12 Therefore, the proposal of a "care model" incorporating components of technology, mental health and primary care has gained traction as a strategy for addressing shortcomings in and barriers to managing these health conditions.
The concept of a model refers to a systematic process that includes actions from the admission of the patient to the healthcare service-providing institution and also includes phases of screening, diagnosis, treatment, follow-up and referral, always in accordance with the needs of the community.13
Taking all of this into account, the main objective of this review was to describe and synthesise the existing evidence on implementing technology-based care models in addressing mental health disorders in the primary care context in order to specifically evaluate the clinical effectiveness of those models, as well as other pertinent outcomes.
MethodsInclusion criteria for studiesTypes of studyRandomised controlled trials (RCTs), before-and-after studies (with or without a control group), interrupted time series (ITS) studies and studies with any other type of prospective design that met the eligibility criteria were included. The studies selected could measure outcomes by comparing institutions or examining a single institution before and after implementation. Mixed-methods studies were included if they evaluated any of the outcomes identified. Strictly qualitative studies were excluded. No studies were excluded on the basis of language or year of publication.
PopulationResearch units corresponded to health centres where technology-based models were implemented. No other restrictions in relation to the sociodemographic characteristics of the population that received care at those centres were applied.
InterventionsImplementation of models focused on the use of technological or digital tools such as smartphones, tablets, Internet use or apps on other devices, developed in primary care contexts for screening, diagnosis, treatment or follow-up of mental health conditions.
Studies that reported interventions implemented in contexts other than primary care, such as psychiatric hospitals; studies with the main objective of examining outcomes in healthcare professionals; and studies that used technologies solely as a communication medium (e.g. telephone calls) were excluded.
Outcomes- -
Clinical effectiveness (measurement of symptoms using validated instruments).
- -
Treatment adherence (continuity in the use of technological tools provided by the model).
- -
Costs (change in care before and after implementation or between intervention and control group).
- -
Reach of the model (number of participants enrolled compared to total number of eligible individuals) and response rate (patients who continued with the recommendations or management proposed by the model).
The systematic search was conducted in six electronic databases (CENTRAL, MEDLINE, EMBASE, PsycINFO, Web of Science and Virtual Health Library [VHL]) and updated until August 2019. The search strategy appears in Appendix 1. Studies were also found by reviewing cross-references included in the articles identified.
Two review groups, Arturo Marroquín-Rivera and Juan Camilo Rosas (AMR and JCR) and María Gómez-Ayala and Felipe Botero-Rodríguez (MGA and FBR), independently screened the articles found based on their titles and abstracts and selected the studies that met the eligibility criteria based on their full texts. Where they disagreed, they consulted a third-party researcher (MC). To screen and select the articles, the Rayyan QCRI14 web app was used to facilitate the process of classification of references and discussion. Two authors, AMR and JCR, independently extracted the data from the studies selected using a data extraction form specifically for this review and resolved discrepancies by referring to the original text.
Data analysisEvaluation of risk of biasDue to the differences in design among the studies included, domains of the Cochrane tool to evaluate risk of bias (RoB 2) and the "Risk Of Bias In Non-randomized Studies - of Interventions" (ROBINS-I) tool to assess non-randomised studies were adapted. The domains considered were: bias due to confounding/randomisation, bias in selection, bias in classification, lost in follow-up, bias in measurement and bias in selection of the reported result.
For the heterogeneity analysis, differences among the studies were sought and explained in narrative form.
Data synthesisThe studies included varied greatly in terms of study design, target population, interventions and comparators used, instruments of measurement, and points for follow-up and evaluation of outcomes. Taking this into account, the performance of a meta-analysis of the results obtained was not a viable option. As a result, data synthesis was performed based on narrative summaries. In addition, the models identified were summarised in terms of four stages: 1) screening, 2) diagnostic confirmation, 3) treatment and 4) follow-up (Fig. 1).
Models identified and technology use by implementation stage.
a) Yu, 2018. GAD-7: generalised anxiety disorder-7. SMS: short message service.
b) SMART, 2017. GAD-7: generalised anxiety disorder-7. PHQ-9: patient health questionnaire-9. EDSS: electronic decision support system. IVRS: interactive voice response system. ASHAs: accredited social health activist.
c) The Allillanchu Project, 2018. SRQ: self-reporting questionnaire.
d) Quanbeck, 2018.
e) Sorkin, 2019. HSC: Hopkins System Checklist. HQT: Harvard Trauma Questionnaire.
The literature search yielded a total of 6753 references; five of them met the eligibility criteria and therefore were included for analysis in the review.15–19
Fig. 2 summarises the search results. The most common reasons why studies were excluded in the screening process were that they did not meet the definition for a care model sought by the researchers or that they did not report outcomes of interest.
Evaluation of risk of biasSeveral of the studies included had components in addition to implementation outcomes; hence, in accordance with our objectives, only the evaluation of treatment effectiveness and adherence were taken into account in evaluating bias.
Among the five studies included, the sole RCT17 showed various biases due to confounding (participant screening and randomisation), with intervention blinding and bias in measurement of outcomes. In addition, baseline differences were detected among patient groups, including variations in level of education, age and prevalence of depression and post-traumatic stress disorder (PTSD). Taking this into account and adding it to the possible influence of each provider on the outcomes of interest, the study was considered to carry a high risk of bias. The four remaining articles corresponded to non-randomised studies seen to be compromised in their entirety by biases due to confounding and, to a lesser extent, bias in measurement of outcomes.
Data extractionGeneral characteristics of the studiesThe five articles selected were published between 2017 and 2019. All of them enrolled elderly individuals only; four screened the general population who visited the health centre15–18 and one screened patients with a history of psychoactive substance use.19
The studies included screened different aspects of mental health, except the Allillanchu study,15 which also evaluated seizure disorder. The most commonly examined diseases were anxiety and depression; these figured in four of the studies.15–18 Other matters evaluated were psychoactive substance use and alcohol use,15,19 quality of life,19 PTSD,17 risk of suicide15 and related problems such as stigma, which influences the implementation of, adherence to and impact of interventions.18 Among the symptom evaluation tools used, the most common were the PHQ-9 scale18 and the GAD-7 scale16,18 (Table 1).
Characteristics of the studies included.
Name of study — Year | Type of study | Implementation site | Target population | Participant care site | Number of participants (n) | Mental health conditions evaluated | Phases included in the model | Technological tools | Primary outcomes | Follow-up time |
---|---|---|---|---|---|---|---|---|---|---|
SMART, 2017 | Before-and-after study with no control | Andhra Pradesh, India | Adults over 18 years of age | Two primary care centres, homes of participants (30 villages of registered tribes) | 238 | Depression and anxiety | Screening, diagnosis, treatment and follow-up | Tablet, EDSS*, IVRS** | Change on clinical scale | 3 months |
The Allillanchu Project, 2018 | Mixed-methods study | Lima, Peru | Adults over 18 years of age | Five public general primary care centres | 143 | Depression, anxiety, psychosis, seizure disorder, AUD*** | Screening, intervention, follow-up | Tablet, digital platform, text messages | Seeking of mental healthcare | 3 weeks |
Implementing a Mobile Health System to Integrate the Treatment of Addiction Into Primary Care, 2018 | Type 2 hybrid effectiveness/implementation study | United States | Adults over 18 years of age with a diagnosis of | A primary care centre for patients with psychoactive substance use disorder | 207 | Psychoactive substance use disorder, AUD | Diagnosis, treatment, follow-up | Mobile app, website | Substance use, quality of life, use of medical services, model adoption, costs | 6 months |
Implementation of a Guided, Digital Cognitive Behavioral Program for Anxiety in Primary Care: Preliminary Findings of Engagement and Effectiveness, 2018 | Before-and-after study with no control | United States | Adults 20−65 years of age | Four general primary care centres | 63 | Anxiety | Screening, diagnosis, treatment and follow-up | Electronic medical record, mobile app, text messages | Change on clinical scale, commitment to app use | 2 months |
[0,1–11] | ||||||||||
Novel Health Information Technology to Aid Provider Recognition and Treatment of Major Depressive Disorder and Posttraumatic Stress Disorder in Primary Care, 2019 | Randomised two-group (two-arm) trial | United States | Adults over 18 years of age Refugees from Cambodia in the United States | Two community clinics with a federal primary care qualification | 390 patients (272 in the intervention group and 118 in the control group) | Depression, post-traumatic stress disorder | Screening, diagnosis, treatment and follow-up | Tablet, electronic app with evidence-based information | Change on clinical scale, concordance between positive screening and medical diagnosis, following of evidence-based recommendations | 12 months |
Of the five studies included, four conducted screening through electronic tablets.15–18 Two of these specified that they used special apps to administer the questionnaires;15,18 the other two did not.16,17 Regarding context, three of the studies conducted screening in a clinical/urban environment17–19 and the fourth conducted screening directly in the homes of potential participants and in a rural setting.18
In the Sorkin et al. study,17 the intervention had three main components: 1) an online tutorial for mental health management focused on trauma; 2) tablet-enhanced screening of all patients prior to medical consultation; and 3) provision of algorithms and guidelines to medical staff through mobile platforms. In the experimental group, healthcare providers were given the score for all patients who completed electronic screening; in the control group, physicians only received scores for cases at high risk of self-aggressiveness or hetero-aggressiveness. The proportion of diagnoses made by medical staff was significantly higher in the experimental group than in the control group, both for depression (OR: 6.5; 95% confidence interval [CI]: 1.48–28,79) and for PTSD (OR: 23.3; 95% CI: 2.99–151.62).17 The three remaining studies corresponded to uncontrolled research lacking comparative measurements in this stage.
The Strengthening Mental Health and Research Training in Africa (SMART) project,18 implemented in India, developed a task distribution system in which accredited social health activists (ASHAs) conducted screening for depression and anxiety in the target population and advised patients with positive results to get counselling with physicians in health camps.
DiagnosisTwo studies implemented a diagnosis stage in previously screened patients. Both carried out that stage using clinical practice algorithms and guidelines for patient diagnosis, which were available in mobile apps installed on tablets.17,18 With regard to Sorkin et al.,17 clinical decision-making was based on an 11-point algorithm designed to diagnose major depression and PTSD. At the end of the intervention, the proportion of patients who received a diagnosis of either of the two diseases in the intervention group was compared to the same proportion in the control group. The participants in the intervention group were 2.6 times more likely to have a diagnosis consistent with their screening compared to the control group.
TreatmentAll five studies included a stage related to patient treatment linked to a technological/digital strategy. Two used mobile apps with modules and strategies as treatment aids16,19; another two used management algorithms through digital platforms1,18 and one designed an automated system to deliver motivational text messages (SMS) to incentivise consultation with mental health specialists.15
Regarding the two studies that used apps in the treatment stage,16,19 the study by Quanbeck et al.19 evaluated how mobile health technologies facilitated integration of treatment for addiction into primary care. The Seva app offered patients interactive modules for learning how to solve problems and self-regulate, tools for coping with high-risk situations, and cognitive behavioural therapy strategies, among other things. The Lantern intervention consisted of a mobile app that provided the patient with a cognitive behavioural programme based on protocols for people with generalised anxiety disorder (GAD). Both studies collected data on app use, including logins and use of modules.
With regard to the two studies that used management algorithms on digital devices, both were developed based on evidence-based and culturally validated scales and guidelines for management. In the SMART study,18 patients diagnosed with mental illness received psychotherapy from healthcare professionals affiliated with the study or were referred to hospitals able to provide more complex care. Regarding the Sorkin et al. study,17 patients were managed using the digital algorithms designed in the intervention group or were managed as usual in the control group. Participation by physicians could encompass aspects such as pharmacotherapy, active surveillance with psychosocial support and referral to a mental health specialist. Whereas in the SMART study the treatment provided was not evaluated in detail in relation to the management algorithms, in the Sorkin study these were primary outcomes that were evaluated by means of review of medical records after the care model had been implemented for 12 weeks.
Finally, the Allillanchu study15 did not include a direct treatment, but did implement a text message system with the aim of increasing specialised medical consultations among patients identified in primary care.
Follow-upThe five studies included reported data on patient follow-up during the implementation of the models. Concerning the strategies used, one study conducted interviews with participants to enquire about specialised medical consultations,15 one study optimised the follow-up stage using digital algorithms and automated voice messages,18 another followed up patients by means of messages through a mobile app,16 another study performed follow-up after 12 weeks of intervention using validated scales,17 and one of the studies granted medical staff access to clinical reports on app use with patient-generated data regarding their substance use and general well-being. All follow-up processes were extremely heterogeneous in terms of both methods and objectives of follow-up, which ranged from optimising management continuity to serving as a medium for collecting information.
In the SMART study, treatment data were shared among medical providers and ASHAs. ASHAs could use algorithms to better plan follow-up and prioritise patients who required timelier visits to ensure treatment adherence. In parallel, physicians, ASHAs and patients received automated voice messages to facilitate follow-up and treatment continuity.
Effect of interventionsSymptom severityAll studies demonstrated effectiveness on the part of the interventions evaluated. Regarding depression, the SMART study18 showed a nine-point reduction in the median in its population, while Sorkin et al.,17 who compared two groups, found a decrease in the score in both. The Allillanchu project,15 for its part, pointed to a benefit of this intervention through a qualitative evaluation. Among interventions for the management of anxiety, there was also a nine-point reduction on the GAD-7 scale subsequent to the intervention.18 This trend was also represented in the study by Yu et al.,16 with a 4.1-point decrease in the average score (®= –2.06; SD=0.77).
The impact on behaviour of the Seva strategy19 was demonstrated in the increase in abstinence from alcohol among those who used the app on more days (p=0.02), while those who advanced more in the app also showed a higher rate of abstinence from alcohol and psychoactive substances (p=0.01), and furthermore exhibited fewer risky behaviours for spreading human immunodeficiency virus (HIV) (p=0.02).
In addition, positive outcomes were found with regard to seeking professional mental healthcare and stigma, measured using two instruments (the Knowledge, Attitude and Behaviour about mental health instrument and the Barriers to Access to Care Evaluation — treatment stigma subscale). The SMART project18 showed that knowledge, attitudes and behaviours related to mental health improved towards acceptance of the topic subsequent to evaluation using these instruments.
Treatment adherenceTwo studies reported data related to treatment adherence,16,19 although the definition of management adherence differed between the two. The Yu et al. study16 evaluated this outcome taking into account the number of modules completed by each participant, the number of times that each technique was practised and the number of days that each person logged on to the application. A "committed" participant was defined as a participant who completed three or more techniques. On average, during the two months of implementation, the subjects used the Lantern app for 18.4 days (SD=15.8), completed 11.2 units (SD=14.3) out of 40 and practised 16.1 techniques (SD=20.3) out of a total of 26. Although there were significant differences in favour of "committed" patients, longitudinal analyses indicated that the number of units or techniques completed and the days of app use were not related to significant differences in terms of changes in scores on the GAD-7 at the end of the two months. Quanbeck et al.19 evaluated management adherence by means of continuity of use of the Seva mobile app. At the start of implementation, app use at the three sites ranged from 94% (90/96) to 99% (69/70); after 12 months of implementation, app use adherence was found to be between 53% (41/78) and 60% (39/65) across the three sites.
While one of the studies evaluated adherence through commitment to app use,16 the second assessed this outcome through continuity in the use of the tool at the end of the implementation period.19 Therein lay the first major difference that precluded joint analysis of results obtained. Moreover, although the decrease in the use of the mobile app in the study by Quanbeck et al. could be compared to findings in similar studies, the lack of a control group rendered suitable appreciation of the results impossible.
CostsNone of the studies included evaluation of variation in costs due to the implementation of the model; however, Quanbeck et al.15 reported data related to implementation costs. In that study, the costs reported were subdivided into the following categories: operational costs, costs of implementation, costs per patient and total costs for each of the three primary care centres in the study. Operating costs per patient were estimated at 1185 United States dollars (USD), total costs per clinic were on average 124,000 USD among the three clinics and costs per patients were an average of 1400 USD.
Reach and response rateAll studies included collected data with respect to the reach or response rate of the intervention, but only in the study by Quanbeck et al. was this explicitly defined as an outcome of interest.19 In the study by Sorkin et al., the patients seen by physicians trained in the digital algorithms were significantly more likely to start on a prescription (44% versus 14%; p<0.001) or begin active surveillance with psychological support (2.6% versus 0%; p=0.008) compared to the patients in the control group. In the Allillanchu study,15 of the 159 subjects eligible due to positive screening, 127 attended the follow-up interview and 92 of them reported having sought help from a specialised professional after being advised to do so by their primary care physician. In the SMART study,18 of the 238 individuals identified as positive in the screening conducted by ASHAs, just 30 (13%) visited a physician, 19 at health camps and 11 at primary care centres. Following implementation of the model, the use of mental health services was seen to increase from 0.8% at the start of the intervention to 12.6% at the end thereof.
Regarding the Quanbeck et al. study, out of 3,226 patients eligible to use the Seva app, 238 were enrolled in the study, thus achieving a reach of 8.31% of people with substance use at the three sites of implementation of the study. In this case, the number of patients enrolled was correlated with the total number of mobile phones available at each care site; this was mentioned as a limitation on achieving a greater reach.
The response rate achieved by the different studies varied greatly; this variation might have been due to multiple factors. One of them was related to the differences between the implementation sites: models applied in urban areas, as in the Allillanchu study in Lima, Peru, had a higher response rate compared to studies conducted in remote rural areas with limited opportunities for access to healthcare on the part of the target population, as in the SMART project, implemented in Andhra Pradesh, India. The differences in the mental health conditions addressed at each site may also have influenced the scope of the intervention; patients with conditions associated with greater social stigma were less likely to enrol in the proposed care model.
DiscussionTo our knowledge, this review is the first to systematically evaluate existing evidence on technology-based care models for addressing mental health. At present, there are studies evaluating similar interventions, but these studies have only evaluated one point in the patient's primary care5 or adherence to a certain technology3; they have not assessed the structured implementation of an optimised model with digital technologies as a whole from patient identification to patient treatment and follow-up over time. Although RCTs that evaluated technological interventions for mental health disorders were identified,20–22 they were excluded from the review as they did not take into account the different stages of patient care. Of the five studies included, just one was an RCT; the other four lacked a control or randomisation process. As a result, the quality of the evidence was seriously compromised by a high risk of bias, and this was compounded by small sample sizes and short follow-up periods. The RCT included, for its part, was classified as having a high risk of bias due to compromise in its processes of participant screening, blinding and analysis of outcomes.
The studies selected enabled identification of screening of medical disorders, with apps on tablets being the most prevalent technology-enhanced process across the care models, followed by patient treatment through mobile apps. This was consistent with a recent systematic review that identified apps as the most commonly used digital technologies in the treatment of patients with severe mental illness.23
Moreover, these studies showed an improvement in endpoints; it was unclear, however, whether this was due solely to the implementation of a new model, or to the implementation of the model added to the use of technological tools. Psychotherapy and rehabilitation approaches start from the assumption that the individual is willing and able to dedicate time to this treatment, allocate around one hour per session and engage in a longer-lasting type of treatment than other types of clinical intervention. The use of technologies to facilitate application and adaptation of interventions has arisen as a result, since it does not depend on office hours or hospital settings.24 On the other hand, it does involve more active participation on the part of the patient; it also entails subsequent legal and professional responsibility in services and treatments provided or indicated through these platforms, which might alter the communication between the two players.25
With this in mind, this review did not find sufficient evidence to support the use of a technology-based mental healthcare model over other existing models.
Strengths and limitationsOne of the greatest strengths of this review was the spectrum of models sought: no mental health disorder, time of care or technology used was excluded. The search was also not limited by year, location, or language, which ensured that it would encompass a larger quantity of pertinent articles. In focusing on studies that assessed interventions linked to the development of a care model, we arrived at a better evaluation of the use of technologies as tools intended to optimise care processes for patients with mental disorders and the effectiveness of interventions in a care context closer to reality.
However, this review did have some limitations, which could not be separated from its greatest strengths. Given that we did not limit our searches according to the above-mentioned criteria, we found a great deal of heterogeneity among studies. This made it impossible to conduct a quantitative synthesis of the results for disorders across studies such as depression and anxiety. Although individual findings with respect to these interventions were favourable across all studies, their low methodological quality undermined confidence in the results obtained. First and foremost, the absence of randomisation and a control group due to confounding factors, added to other previously mentioned factors, seriously affected the quality of the evidence.
In addition, the small number of eligible studies restricted the robustness of the conclusions of this study. This limitation can be attributed in part to the process of determining the definition of a "care model". Given that the existing literature on this topic is scant, the review team adapted a set of characteristics considered essential when determining whether the studies retrieved featured mental healthcare models. This contributed in large part to the limited number of eligible studies identified and the lack of homogeneity among those studies.
Moreover, there was likely some publication bias, bearing in mind that the search was primarily focused on the most important electronic databases and did not delve into grey literature. Added to this was the fact that the low number of studies included did not allow for an analysis using funnel diagrams or statistical methods to evaluation publication bias.
Implications for practice and future researchFor implementation research on healthcare models to result in learning processes on a national or global level, studies of good methodological quality that evaluate both the implementation of models and the effectiveness of the interventions derived from them must be conducted. Regarding evaluation of effectiveness, the way in which confounding factors are controlled for must always be assessed, either through randomisation processes or through analysis of baseline characteristics in groups and possible factors that could end up influencing outcomes. The conduct of hybrid implementation/effectiveness studies could help to address this divide, in particular hybrid type 2 studies since they are focused on simultaneous evaluation of clinical interventions and implementation outcomes.26 In addition, as we did not find studies done in a context similar to Colombia, we believe it is important to conduct feasibility studies adapted to the needs and capacities of the Colombian population and health system.
Among the studies identified, those that evaluated the use of digital apps during the treatment phase did so by creating and testing their own apps. However, none of the studies developed these apps according to pre-established recommendations or consensuses. Although scant literature exists to date, a recent review set out multiple considerations in creating mental health apps in order to improve the functionality of these digital tools.27 Future research should focus on standardising app development according to evidence-based recommendations, then validating these tools by means of experimental studies. In addition, longer follow-up times are desirable to evaluate patient adherence to such digital tools, the use of which is essentially self-regulated.
ConclusionThis review provides clinicians and decision-makers with a synthesis of the evidence existing to date on technology-based care models for the broad spectrum of disorders in mental health. Implementation of technological models that address the different stages of patient care, as seen in the five studies included, is a viable strategy that could optimise processes of screening, diagnosis, treatment and follow-up of patients with this type of disorder. However, the evidence existing to date and the quality thereof are insufficient for determining whether a technology-enhanced care model is superior to other similar models in addressing mental health. Studies with good methodological quality that evaluate the implementation and effectiveness of these models must be conducted before the best technology-based mental healthcare model can be identified.
FundingThe research reported in this publication was funded by the National Institute of Mental Health (NIMH) of the National Institutes of Health (NIH) of the United States with Grant Number 1U19MH109988 (multiple principal investigators: Lisa A. Marsch, PhD, and Carlos Gómez-Restrepo, MD, PhD). Juan Camilo Rosas and Arturo Marroquín Rivera participated as Young Researchers, funded by the Jóvenes Investigadores e Innovadores program by the Colombian Ministry of Science, Technology and Innovation (Contract 829, 2018).
Conflicts of interestThe authors declare that they have no conflicts of interest.
We would like to thank the entire DIADA project working team, based at Pontificia Universidad Javeriana [Pontifical Xavierian University] in Bogotá, Colombia, and at Dartmouth College, New Hampshire, United States, for their contributions to the article manuscript review process.
PubMed: (implementation OR model of care OR "Health Plan Implementation" [Mesh] OR "Implementation Science" [Mesh] OR "Regional Health Planning" [Mesh] OR "Social Planning" [Mesh] OR "Risk Evaluation and Mitigation" [Mesh] OR "Health Services" [Mesh] OR "United States Public Health Service" [Mesh] OR "Women's Health Services" [Mesh] OR "Student Health Services" [Mesh] OR "Child Health Services" [Mesh] OR "School Health Services" [Mesh] OR "United States Indian Health Service" [Mesh] OR "Preventive Health Services" [Mesh] OR "Adolescent Health Services" [Mesh] OR "Personal Health Services" [Mesh] OR "Rural Health Services" [Mesh] OR "Suburban Health Services" [Mesh] OR "Urban Health Services" [Mesh] OR "Delivery of Health Care" [Mesh] OR "Quality Indicators, Health Care" [Mesh] OR "Health Care Sector" [Mesh] OR "Health Care Surveys" [Mesh] OR "Delivery of Health Care, Integrated" [Mesh] OR "Health Care Reform" [Mesh] OR "Peer Review, Health Care" [Mesh] OR "Health Care Evaluation Mechanisms" [Mesh] OR "Health Care Quality, Access, and Evaluation" [Mesh] OR "Process Assessment (Health Care)" [Mesh] OR "Outcome Assessment (Health Care)" [Mesh] OR "Health Care Costs" [Mesh] OR "Health Care Rationing" [Mesh] OR "Quality of Health Care" [Mesh] OR "Quality Assurance, Health Care" [Mesh] AND ("Primary Health Care" [Mesh]) AND (mental health OR mental diseases OR mental disorders OR "Mental Health" [Mesh] OR "Mental Health Services" [Mesh] OR "Mental Health Associations" [Mesh] OR "Community Mental Health Services" [Mesh] OR "Community Mental Health Centers" [Mesh] OR "Mental Health Recovery" [Mesh] OR "Health Plan Implementation" [Mesh] AND (Mobile Applications" [Mesh] OR Electronics" [Mesh] OR Electronic Health Records" [Mesh] OR Electronic Data Processing" [Mesh] OR Technology" [Mesh] OR "Wireless Technology" [Mesh] OR "Remote Sensing Technology" [Mesh] OR "Biomedical Technology" [Mesh] OR "Educational Technology" [Mesh] OR "Technology, High-Cost" [Mesh] OR "Technology Assessment, Biomedical" [Mesh] OR "Information Technology" [Mesh] OR "Cell Phone" [Mesh] OR "Cell Phone Use" [Mesh] OR "Smartphone" [Mesh] OR "Text Messaging" [Mesh] OR "Computers, Handheld" [Mesh] OR e-medicine OR mhealth OR emedicine OR telemedicine OR ipad* OR "Personal Digital Assistant*" OR pda* OR palmtop* OR "videotape" [All Fields] OR "recording" [All Fields] OR "videotape recording" [All Fields] OR "video"[All Fields] OR "telecoaching" [All Fields] OR "teleconference" [All Fields] OR "educational technology" [All Fields] OR "internet" [MeSH Terms]).
Web of science: ("implementation" OR "care model" OR "Health plan implementation" OR "implementation science" OR "regional health planning" OR "social planning") AND ("mental health" OR "mental disease" OR "mental disorders therapy" OR "mental health service" OR "community mental health" OR "mental health recovery") AND ("mobile application" OR "electronics" OR "electronic medical record" OR "information processing" OR "technology" OR "wireless communication" OR "medical technology" OR "educational technology" OR "biomedical technology assessment" OR "information technology device" OR "mobile phone" OR "wireless communication" OR "medical technology" OR "wireless technology" OR "cell phone" OR "smartphone" OR "text messaging" OR "handheld computers" OR "mhealth" OR "palmtop" OR "video" OR "telecoaching" OR "internet").
EMBASE: ('implementation science'/exp OR 'health care planning'/exp OR 'social aspect'/exp OR 'risk evaluation and mitigation strategy'/exp OR 'health service'/exp OR 'public health service'/exp OR 'preventive health service'/exp OR 'health care delivery'/exp) AND ('primary health care'/exp) AND ('mental health'/exp OR 'mental disease'/exp OR 'mental disorders therapy'/exp OR 'mental health service'/exp OR 'community mental health'/exp OR 'mental health recovery'/exp OR 'health care planning'/exp) AND ('mobile application'/exp OR 'electronics'/exp OR 'electronic medical record'/exp OR 'information processing'/exp OR 'technology'/exp OR 'wireless communication'/exp OR 'medical technology'/exp OR 'educational technology'/exp OR 'biomedical technology assessment'/exp OR 'information technology device'/exp OR 'mobile phone'/exp OR 'smartphone'/exp OR 'text messaging'/exp OR 'personal digital assistant'/exp OR 'mhealth'/exp OR 'telemedicine'/exp OR 'tablet computer'/exp OR 'educational technology'/exp OR 'Internet'/exp).
The Cochrane Library: (mh "implementation science" OR mh "health planning" OR mh "health plan implementation" OR mh "regional health planning") AND (mh "Primary Health Care) AND (mh "mental health" OR mh "mentally ill persons" OR mh "mental disorders" OR mh "mental health services" OR mh "community mental health centers" OR mh "community mental health services" OR mh "mental health recovery") AND (mh technology OR mh "mobile applications OR mh electronics OR mh "electronic health records" OR mh "electronic data processing" OR mh "wireless technology" OR mh "Biomedical technology" OR mh "educational technology" OR mh "information technology" OR mh "cell phone" OR mh smartphone OR mh "text messaging" OR mh "computers, handheld" OR mh telemedicine OR mhealth OR emedicine OR telemedicine OR ipad OR palmtop OR video OR internet)
PSYCHinfo: implementation OR model of care OR "Health Plan Implementation" OR "Implementation Science" OR "Regional Health Planning" OR "Social Planning" OR "Risk Evaluation and Mitigation" OR "Health Services" OR "United States Public Health Service" OR "Women's Health Services" OR "Student Health Services" OR "Child Health Services" OR "School Health Services" OR "United States Indian Health Service" OR "Preventive Health Services" OR "Adolescent Health Services" OR Personal Health Services" OR Rural Health Services" OR "Suburban Health Services" OR "Urban Health Services" OR "Delivery of Health Care" OR "Quality Indicators, Health Care" OR "Health Care Sector" OR "Health Care Surveys" OR "Delivery of Health Care, Integrated" OR "Health Care Reform" OR "Peer Review, Health Care" OR "Health Care Evaluation Mechanisms" OR "Health Care Quality, Access, and Evaluation" OR "Process Assessment" OR "Outcome Assessment" OR "Health Care Costs" OR "Health Care Rationing" OR "Quality of Health Care" OR "Quality Assurance, Health Care") AND ("Primary Health Care") AND (mental health OR mental diseases OR mental disorders OR "Mental Health" OR "Mental Health Services" OR "Mental Health Associations" OR "Community Mental Health Services" OR "Community Mental Health Centers" OR "Mental Health Recovery" OR "Health Plan Implementation") AND ("Mobile Applications" OR "Electronics" OR "Electronic Health Records" OR "Electronic Data Processing" OR "Technology" OR "Wireless Technology" OR "Remote Sensing Technology" OR "Biomedical Technology" OR "Educational Technology" OR "Technology, High-Cost" OR "Technology Assessment, Biomedical" OR "Information Technology" OR "Cell Phone" OR "Cell Phone Use" OR "Smartphone" OR "Text Messaging" OR "Computers, Handheld" OR e-mdicine OR e-medicine OR mhealth OR emedicine OR telemedicine OR ipad* OR "Personal Digital Assistant*" OR pda* OR pad OR palmtop* OR "videotape" OR "recording" OR "videotape recording" OR "video" OR "telecoaching" OR "teleconference" OR "educational technology" OR "internet".
LILACS: (salud mental OR mental health) AND (prevención OR atención primaria) AND (tw:telemedicina OR e-medicine OR tecnologia OR technology) AND (tw:modelo).
Please cite this article as: Rosas JC, Gómez-Ayala MC, Rivera AM, Botero-Rodríguez F, Cepeda M, Suárez-Obando F, et al. Modelos de atención en salud mental basados en tecnologías: revisión sistemática de la literatura. Rev Colomb Psiquiat. 2021;50:30–41.