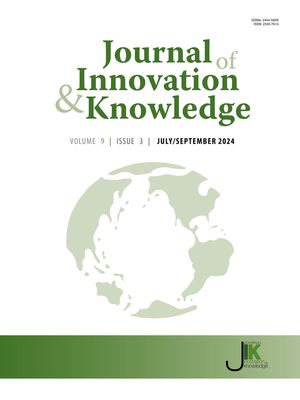
Editado por: Marcin W. Staniewski
Más datosThe sharing economy is a relatively recent phenomenon that aims to promote environmentally friendly practices. The sharing economy is characterized by the convergence of information, marketing and technology to create a new paradigm in which customers value access above ownership, allowing them to make better use of resources. It provides a modern business strategy that can pave the way for energy efficiency and sustainable economic development. However, the economic and environmental effects of the sharing economy are debatable and require more research. Indeed, the sharing economy has upended the current economic standard, raising concerns about the benefits and disadvantages of the new behaviour. Therefore, the present study fills a gap in the literature by quantitatively assessing the possible impacts of the sharing economy on sustainable economic development and energy efficiency in China over the period 2000 to 2019. Recently introduced QARDL estimation methodology is applied to achieve the objective, controlling for population growth, unemployment, energy prices, and inflation. The sharing economy is shown to make a positive contribution to promoting sustainable development across all quantile ranges, while it contributes to enhanced energy efficiency at higher quantiles only. According to the findings, the sharing economy turns out to be a socioeconomic phenomenon with the potential to promote energy efficiency and sustainable development. Therefore, we emphasize the potential of the sharing economy and recommend the Chinese promote sharing economy models to reach their full potential for sustainable development in energy efficiency and economic terms by enacting suitable taxation and legislation policies.
Energy efficiency and sustainable development are major issues for various governments particularly following a considerable increase in natural resource exploitation, which has resulted in serious environmental issues (Dabbous & Tarhini, 2021). In 1987, the term sustainable development was popularized as “development that meets current demands without jeopardizing future generations' ability to meet their own needs”. The majority of standards deal with environmental, social and economic aspects of sustainability (Boar et al., 2020). The so-called triple bottom line, which includes environmental, economic and social responsibilities, is the foundation of sustainable development (Elkington, 1994; Longoni & Cagliano, 2018). The so called 3Ps—planet, profit and people—are the traditional pillars or fields of social responsibility (Amaladoss & Manohar, 2013). There are microeconomic and macroeconomic aspects to sustainable development (Buchholz et al., 2009; Szczuka, 2015). From a macroeconomic standpoint, the focus is on the long-term development of economies (Szczuka, 2015). From a microeconomic standpoint, significant emphasis is put on the viability of individual businesses. However, the microeconomic aspect, from our viewpoint, encompasses sustainability from the perspective of individual people, non-profit organizations and public institutions (Jelinkova et al., 2021). Using less energy to generate the same amount of products and services is referred to as energy efficiency, and has numerous benefits. Energy efficiency techniques, for example, are helpful to increase ecosystem stability, reduce CO2 emissions, lessen fossil fuel reliance and encourage industrial competition by cutting costs of operation (Akram et al., 2020). Energy efficiency can help fight global climate change in two ways. “The less energy used, the fewer emissions released”, says the first principle. This means that energy efficiency makes a substantial contribution to potential energy strategies to fight climate change and global warming. Secondly, energy efficiency that is cost effective achieves environmental benefits by minimizing the economic costs of achieving climate policy objectives (Javid & Khan, 2020). Moreover, energy efficiency is an important part of any green development programme which aims to reduce emissions (Akram et al., 2020; Harun et al., 2022; Maheswaranathan & Bhavan, 2022; Shair et al., 2021; Treptow, 2022). Achieving sustainable development goals requires better energy efficiency. Energy efficiency has various societal benefits, regardless of its influence on air pollution. It decreases business energy expenses, increases productive capacities, enhances energy security, lowers energy prices, and reduces environmental emissions (Aliu & Hajdini, 2021; Alsoud et al., 2021; Hernawati et al., 2021; Hieu et al., 2021; Hussain et al., 2021; Mattayaphutron & Mahamat, 2021; Nawaz et al., 2021; Nazir et al., 2022; Yilmaz, 2022).
How can sustainable development and energy efficiency be promoted? The answers are numerous, but the rising trend for sharing economies has significant implications for the promotion of both. The sharing economy, which is built on sharing idle assets, is a contentious phenomenon (Leung et al., 2019). The sharing economy is characterized as “a community-based internet platform that facilitates peer-to-peer sharing of accessibility to products and services” (Mi & Coffman, 2019). It is a “growing tendency in consumer behaviour” that is “exploding rapidly” (Fagerstrøm et al., 2017). It is predicted to provide a number of societal benefits, including the ability to save and/or earn money, help change consumer behaviour, reduce resource consumption, and promote sustainable consumption approaches, paving the way for sustainable economic growth. The sharing economy relates to beneficial socioeconomic and environmental outcomes. It is regarded as a step forward in society in terms of cost-effective methods and resource efficiency. It is expected to have several benefits, including resource savings, increased living standards, new revenue streams and increased availability of low cost products and services (Alshahrani, 2021; Diep & Hieu, 2021; Hasker, 2021; Schor, 2016).
From the perspective of economic, social, and environmental responsibility, sharing has the ability to contribute to sustainable development (Tetrevova & Kolmasova, 2021). Sharing can be viewed as a strategy for creating innovation and guaranteeing product sustainability from the standpoint of economic responsibility. Sharing also helps strengthen producer and consumer relationships, for example, by sharing transportation modes such as heavy duty vehicles and their cargo facility, delivery/order information, storage capacity, business deals or references, or data about cooperative advertising or consumer opinions, all of which have a beneficial interpersonal multiplier impact. It also helps develop customer relationships, for instance, by sharing expertise (Gaba et al., 2021; Hamsal et al., 2021; Jelinkova et al., 2021; Lee & Brahmasrene, 2020; Lima et al., 2021).
The sharing economy has sparked some academic debate about economic sustainability and energy efficiency. Only a few empirical studies focus on the impact of the sharing economy on economic sustainability and there is still a research gap in this area (Demailly & Novel, 2014; Geissinger et al., 2019; Parente et al., 2018). Furthermore, some research claims that the sharing economy has disrupted conventional production and consumption patterns, which might lead to more advantages and a better economy, with energy savings and a low environmental impact (Pouri & Hilty, 2018).
Following this discussion, the principal objective of our study is to estimate the role of the sharing economy in achieving energy efficiency and sustainable development in China over the period 2000–2019. In particular, we make a quantitative assessment of the potential implications of the sharing economy for energy efficiency and sustainable development. To this end, we propose two models. The first aims to predict the role of the sharing economy on sustainable economic development, controlling for population growth, inflation, and unemployment rate. The second model estimates whether a rising sharing economy aims to reduce energy intensity, thereby increasing energy efficiency, while controlling for population growth and energy prices.
Why we select China? Because the sharing economy has reached a new development level in China, thanks to the application and advancement of information technology. The market share of the sharing economy in China increased continuously from 2015 to 2019. In 2016, the annual growth rate reached 95.74%, but has declined since then. Once-popular enterprises such as peer-to-peer (P2P) financing and bike-sharing have seen losses since 2018. Between 2016 and 2019, China's sharing economy revenue grew at a much faster rate than the conventional economy. In 2019, internet vehicle passenger traffic made up 37.1% of overall taxi passenger traffic, with a penetration level of 47.4% amongst internet users. Shared housing revenue accounted for 7.3% of overall lodging revenue, with a penetration rate of 9.7%. Currently, such businesses focus on lodging, transportation, skills and knowledge, and services, amongst other things. In 2019, 800 million people were anticipated to have participated in business activities, with 78 million network operators employing 6.23 million people. This means that more than half of China's population has participated in the sharing economy in some capacity. In recent times, China has seen both tremendous growth and failures in the sharing economy. In the last two years, more government regulation and simplification have been presented (Greening & Azapagic, 2012; Liu et al., 2020). Fig. 1 indicates the development of the market size of the sharing economy in China between 2015 and 2019.
China has maintained rapid economic growth for nearly 40 years since liberalization in 1978, with phenomenal GDP growth. A comprehensive economic growth model with high energy input and investment lies behind this rapid growth. Because of their fossil fuel dependence, the major chemical and energy industries have grown. According to the National Bureau of Statistics of China, China's investment in environmental pollution management in 2015 alone accounted for 1.29% of the country's total GDP. China's total energy output and consumption were 3.62 billion tonnes of standard coal equivalent (SCE) in 2015, compared to 4.30 billion tonnes of SCE in 2003. In terms of energy structure, coal was China's main energy source until 2015, accounting for over 64% of the country's primary energy use, which is 40% greater than the global average. To measure China's energy efficiency, the above data on GDP and energy consumption are crucial (Yang & Li, 2017). However, experts often overlook one essential factor of energy efficiency and sustainable development, the sharing economy, which, as previously stated, has a significant impact on these factors and needs serious consideration. Therefore the present study is a quantitative estimation of the effect of the sharing economy on sustainable economic development in China (Echaust & Małgorzata, 2020; Marchiori & Franco, 2020; Shen et al., 2020; Tsai et al., 2020; Vranešević & Perić, 2020).
Following the introduction, section 2 provides a brief review of the existing literature. The data and methodology are given in section 3. The results and discussion are provided in section 4, and section 5 gives the conclusion of the study and policy implications.
Review of existing literatureTheoretical framework for the sharing economy and sustainable developmentThe term “sharing economy” is not quite new. The idea is a part of the history of human civilization. Since the Industrial Revolution, the sharing economy has referred to peer-to-peer commercial trade, but has been dominated by giant enterprises. In the past century, the state was controlled by major corporations, and the industrial age changed the kinds of exchange, commerce, and employment connected with economic sharing. In the new millennium, digital technology has revitalized sharing behaviours, self-employment, and other forms of community-based exchange (Schor, 2016). Sustainable development, according to Muñoz and Cohen (2017) is a crucial idea of our time, which is both a means of understanding the world and a method for overcoming global challenges. The global society, physical environment and world economy are three complex systems for sustainable development. These ideas are similar to the new digital economy, sharing economy, and even economic democratization (Darity Jr et al., 2021; George, 1999; Lydeka & Karaliute, 2021; Paraschiv et al., 2021; Parshakov et al., 2021; Slatten et al., 2021).
Consideration of the conditions that led to the formation of the sharing economy and how they align with the tenets of sustainable development is necessary in order to comprehend the relationship between the sharing economy and sustainable development. The sharing economy is characterized as a complex of economic, technological, environmental and social changes.
The following list, based on the work of Gold (2003); Lyaskovskaya (2021), and Zhu and Liu (2021) indicates the necessary prerequisites for the emergence of a sharing economy.
The present idea of the sharing economy, which represents a significant shift in the technological and technical aspects of consumption and production was, in the opinion of the majority of researchers, brought about by the digitalization of many spheres of life. The connections between digitalization and the new phase of industrialization are widely acknowledged as the most important aspects of the shift to the digital world, including big data, augmented production, robotization, cloud computing, 3D printing, storage of data etc. Digitalization has had a great influence on consumption techniques, which affect millions of families worldwide, rather than how goods and services are created. New sharing economy business models have emerged as a result of these shifts, demonstrating a new level of socially and ecologically conscious behaviour engrained in sustainable purchasing. The conclusion that new models of business are contained in a sharing economy of responsible and sustainable consumption, coincides with the socially conscious economic and ecological objectives of sustainable purchasing and consumption, made possible by analysis of the conditions that led to the birth of sharing economy (Lyaskovskaya & Khudyakova, 2021). Additionally, the sharing economy is presented in various studies as an economic benefit that facilitates environmentally friendly consumption of shared goods and acts as a stepping stone to a strong and sustainable society (Boar et al., 2020; Curtis & Lehner, 2019). The connections between the sharing economy and the long-term health and growth of national economies, as well as its implications for the attainment of sustainable development goals, are highlighted by these arguments (Curtis & Mont, 2020; Mi & Coffman, 2019).
There have been numerous pieces of research on the sharing economy and sustainable development. Recently Dabbous and Tarhini (2021) scrutinize data from 18 OECD countries over the period 2014 to 2018 in order to estimate the role of the sharing economy on energy transition and sustainable development. The authors measure the sharing economy in terms of internet search data on Google trends. The authors observe that the sharing economy affects energy efficiency and sustainable development positively, through fixed effects estimation with Driscoll-Kraay standard errors. Lyaskovskaya & Khudyakova, 2021 quantitatively assess the effect of the sharing economy on three aspects of sustainable development, but find that it has no significant impact on sustainable development achievement. George (1999) discusses the sharing economy in Indonesian cities, particularly in the online transportation industry, specifically Grab, Uber and Go-Jek. The findings reveal that, in Indonesia, the sharing economy enhances inclusiveness, social value and economic worth. In the Czech Republic, Jelinkova et al. (2021) appraise customers' perspectives on the advantages of the sharing economy in terms of environmental, economic and social responsibilities. The customers, who belonged to various age groups and achieved various educational levels, perceived advantages of the sharing economy in terms of environmental, economic and social responsibilities. Rehman and Deyuan, (2018) explore the effect of population growth on economic growth in Pakistan and conclude that population growth affects economic growth positively. Likewise, Garza-Rodriguez et al. (2016) explore the same effect, considering Mexico, and find that population growth has a negative impact on sustainable development. Mohseni and Jouzaryan (2016) study the effect of unemployment and inflation on sustainable development in Iran and find negative impacts of both. However, Meidani and Zabihi (2011), also in Iran, find a positive contribution of unemployment on GDP per capita growth.
Ngai et al. (2008) investigate the factors behind the success of the sharing economy for sustainable development, and conclude that the sharing economy supports sustainable development. Liu and Chen (2020) conclude that the sharing economy is helpful in promoting sustainable development by improving market impetus, efficiency in resource utilization, consumption concepts and transformation concepts. Pouri and Hilty (2018) conceptualize the sharing economy in terms of sustainable development and find different positive and negative aspects of the sharing economy for different sectors of the economy. Cheng and Edwards (2019) conclude that sharing economies lead to monopolization of sharing firms and disturbance of government regulations that cause sustainable development to decline. Cheng (2016) reviews the existing literature on the sharing economy and sustainable development and concludes that the sharing economy has a long intellectual history that influences the fields of consumption, social movement and lifestyle, and the sharing paradigm (Nikolaou, 2022; Șerban et al., 2022; Velín-Fárez, 2021; Ye et al., 2022; Yu et al., 2022; Zirra et al., 2022).
Thus we form the hypothesis:
H1 The sharing economy has a significant and positive impact on the sustainable development of China.
This section examines the link between sharing economy use and energy efficiency. Previous research indicates a discussion about the effect of the sharing economy on long-term economic development and environmental advantages (Zmyślony, Leszczyński, Waligóra, & Alejziak, 2020). Energy efficiency is thought to improve productivity and competitiveness (Rajbhandari & Zhang, 2018; Williams & Horodnic, 2017). However, the nexus between the sharing economy and energy efficiency is not explored in many previous studies. Only a few can be found in the present literature studying this association. For instance, Abubakari (2021) and Belk (2014) assert that the sharing economy helps energy conservation, trash reduction, carbon footprint reduction and emissions reduction (Muñoz & Cohen, 2017). Fitriyanto and Iskandar (2019) study the impact of energy prices and GDP per capita on energy intensity in ASEAN countries and conclude that both variables affect energy intensity negatively. Likewise, Mahmood and Ahmad (2018) find that GDP and energy prices influence energy intensity negatively in European countries.
Ala-Mantila et al. (2016) explore the relationship between household size, urban structures, lifestyles, and greenhouse gas emissions. The development of collaborative consumption is observed to have the potential to reduce lone dwellers' greenhouse gas emissions by compensating for lower intra-household sharing with inter-household sharing (Surya et al., 2020). Some researchers explore the impact of information and communications technology (ICT) sustainability on energy consumption and the environment. For instance, Coroama and Hilty (2014) observe there is consumption of energy and resources by ICT at various levels of the supply chain. Bashroush et al. (2016) asserts that, because of the increased demand for ICT applications, consumption of energy keeps rising, despite all efforts at energy efficiency. A similar claim is made by Das (2019), and this has a lot to do with the sharing economy, because its software applications necessitate a lot of hardware and data centres, which in turn use a lot of natural resources and energy. Ganapati and Reddick (2018) assert that sharing saves energy in the long term, since it provides a sustainable and resource-conserving strategy by selling services rather than products. Hence, the question arises, whether a sharing economy has a positive or negative effect on energy efficiency. Therefore we develop the hypothesis:
H2 The sharing economy has a negative and significant effect on energy efficiency.
The comprehensive review of studies to date clearly demonstrates the significant contribution of the sharing economy to achieving energy transition and sustainable development. However, the quantitative assessment of this nexus is insufficiently covered in the literature. Particularly, China has not received enough attention in terms of this nexus. Knowing these gaps in the literature, there are several worthy contributions of our study. Firstly, to the best of our knowledge, this study accompanies very few existing studies that make quantitative assessments of the objectives. Secondly, as a proxy for the sharing economy, the study applies Uber China's social embeddedness, assessed through online reports and surveys collecting daily observations. Thirdly, this study is novel in terms of the estimation methodology employed, i.e., the quantile autoregressive distributed lag (QARDL) model, which assesses the quantitative relationship at various quantiles to provide a comprehensive picture of the relationship. Thus the study makes a significant contribution to the literature by providing the practical implications of a rising sharing economy for energy efficiency and sustainable economic development, which are useful for individuals and policy makers.
Data and methodologyConceptual framework of the studyTo assess the impact of the sharing economy on sustainable development and energy transition, we propose two models. Model 1 assesses the relationship between sustainable development and the sharing economy, controlling for population growth, unemployment, and inflation. Model 2 estimates the relationship between energy efficiency and the sharing economy, controlling for energy prices and population growth. The conceptual framework of the study is given in Figs. 2 and 3, below.
Following Dritsakis and Stamatiou (2016), Mohseni and Jouzaryan (2016), Sahnoun and Abdennadher (2019), and Adaramola and Dada (2020), Model 1 is specified as:
where GDPP stands for gross domestic product per capita, SE denotes sharing economy, POPG represents population growth and INF stands for inflation.Similarly, taking support from the studies of Adom (2015), Mahmood and Ahmad (2018) and Dabbous and Tarhini (2021), Model 2 is specified as:
where EEF stands for energy efficiency and EP stands for energy prices.Variable measurements and data sourcesSustainable development is measured by gross domestic product per capita (constant 2015 $). Unemployment rate is measured as unemployment (% of total labour force). Energy efficiency is calculated as total final energy consumption divided by GDP. Consumer price index (CPI) is taken as the measure of inflation. Population growth (%) is taken as a proxy for population growth. All data is taken from the World Development Indicators (WDI). Lastly, we measure sharing economy as Uber China's social embeddedness. The data concerning Uber China and its stakeholders has been widely publicized in national and international news outlets. The rapid expansion of the internet has enhanced our access to these reports, and Uber, Didi, and other car-sharing platforms' development and competing activities are frequently highlighted in newspapers, magazines, and other new media. We review industry reports and internal papers to obtain daily observations. The description of all variables are formulated in Table 1.
Description of the variables.
Variable | Proxy | Data source | References |
---|---|---|---|
Sustainable development | GDP per capita (constant 2015 $) | World Development Indicator (WDI) | Dabbous and Tarhini, (2021) |
Sharing economy | Data on development of Uber, Didi and car sharing industry in China | National and international mainstream media reports | Fan et al. (2019), Nguyen (2021) |
Energy efficiency | Total final energy consumption (% of GDP) | World Development Indicator (WDI) | Dabbous and Tarhini, (2021) |
Inflation | Consumer Price Index (CPI) | World Development Indicator (WDI) | Murad et al. (2019) |
Unemployment | Unemployment (% of total labour force) | World Development Indicator (WDI) | Dabbous and Tarhini (2021) |
Population growth | Population growth (%) | World Development Indicator (WDI) | Boldeanu and Constantinescu (2015), Dabbous and Tarhini (2021) |
The augmented Dickey-Fuller (ADF) tests are amongst the most often employed techniques for determining the presence of a unit root in the majority of empirical studies. By including the lagged values of the outcome variable, the ADF test augments the Dickey Fuller test. Lagged and k-lagged first differences are regressed on the 1st difference of a variable on constant and linear deterministic trends, expressed as:
where Δdenotesfirst difference, Y is the macroeconomic series and εtisthe error term. In this test, optimal lag length is chosen by the t-sig approach. The null hypothesis of the test states that the series contains a unit root while the alternative hypothesis states the reverse.However, the ADF test can give a biased conclusion of a unit root in the presence of structural breaks in the time series. The issue arises when the series is interrupted, and such a shock could have a long-lasting impact on the series. The situation worsens if the series remains stationary. Initially, the shock only has a temporary impact, but if there is a structural break, the shock has a lasting impact. This can inadvertently result in the unit root not being rejected. Consequently, it has been demonstrated in numerous investigations that an unexplained break in the series lessens the bias of the unit root tests. Zivot and Andrews (2002) develop the concept of Perron (1989) and add an endogenous break to the model, creating the sequential trend break model. Unlike Perron (1989), Zivot and Andrews’ (2002) model is characterized by the absence of the crash dummy variable, D(TB). These models are designated as Models A, B, and C respectively. Changes to the intercept and slope are permitted in models A and B, respectively, whereas changes to both the intercept and slope are permitted in model C.
These equations test the alternative hypothesis of the stationarity of the series against the null hypothesis of a unit root. According to Zivot and Andrews (2002), every point is taken into account as a potential break point, and a regression is done for each potential break point in turn. In contrast to Perron (1989), Zivot and Andrews (2002) show less strong evidence against unit roots by taking an endogenous break point.QARDL modelIn line with the work of Ali et al. (2021), Godil et al. (2021), and Shu, Li, Ma, & Qureshi, 2022, we employ a novel QARDL technique to examine the nonlinear relationship between the sharing economy and the other study variables (Cho et al., 2015). In a nutshell, the QARDL estimation enables the assessment of the long-term quantile impact of the variables on China's sustainable development. Furthermore, for both short-term and long-term symmetry, the Wald test is employed to examine the dependence of the parameters in each quantile. The traditional linear ARDL model is:
Model 1:
Model 2:
The Schwarz information criterion is used to choose the lag orders p and q, and t represents the white noise error term. GDPP, SE, POPG, UE, INF, and EP represent sustainable development, sharing economy, population growth, unemployment rate, inflation and energy price, respectively. Equations (i) and (ii) are transformed into quantile form, as shown in equations (iii) and (iv):
where the terms ε (τ) = EFt – QEFt (τεt−1) and 0 <τ<1 indicate the quantiles. In order to apply data analysis approaches, several quantile pairs, ranging from 0.05 to 0.95, are analysed. The white noise disturbance term in equations (v) and (vi) accounts for potential sequential association:.We can represent the above equations with an error correction model (ECM), where equations (vii) and (viii) take into account the quantile ARDL approach:The total short-run effect of earlier GDPP on current GDPP and earlier EEF on current EEF is determined by β*=∑i=1p−1β1taking the delta approach. But the combined short-run effect of current and earlier values of SE on current EEP and GDPP is computed as β*=∑i=1q−1β2. The residual aggregate short-run influence of the prior and present values of the control variables is calculated using a similar procedure. Finally, the speed of adjustment parameter in equation (iv) must be negative and significant (Cho et al., 2015).
Wald test and granger causality testWe apply the Wald test to identify the long-run and short-run asymmetric effects of all independent variables, (speed of adjustment of parameters), H0 ρ* (0.05) = ρ* (0.10)…ρ* (0.95). A similar hypothesis is tested for βSE, βUE, βPOPG and βINF (the long-run parameters) and σSE,σUE,σPOPGandσINF (the short-run parameters).
Troster (2018) introduces the quantile Granger causality test, which is applied to analyse the quantile causal associations amongst the dependant and independent variables. There is no Granger causality between a variable (Xi) and another variable (Yi) in the case of no contribution of Xi in the estimation of Yi. An explanatory vector is supposed (Ni= Niy, Nix)’∈Re, s= o+q, where Nix is the earlier indication group of Xi Nix = (Xi-1, ….., Xi-q)’ ∈ Rq.. The H0 of no Granger causality between Xi and Yi is:
where Yi is Fy (.| NiY, NiX), the provisional distribution purpose, given that (NiY, NiX). The DT check is applied by the classification of the QAR method m (•) for the whole π ∈ ⊂ Γ [0,1], based on the null hypothesis of no Granger causal association as:The coefficients [∂(π)= (λ1(π) λ2 (π)] and μt are estimated through maximum likelihood in equal quantile points, and − Ω−1Y (.) is the reverse of a fundamental distribution function. Lastly, the QAR (1) equation based on equation (xi) is:
Empirical findings and discussionsTable I shows the descriptive statistics such as the mean, standard deviation, data range and Jarque–Bera (J-B) test for the variables under examination. In comparison to the other variables, GDPP has the highest mean, while EEF has the lowest mean. The standard deviation shows that EP has the highest variation, compared to other variables, and GDPP has the lowest. Finally, the J-B statistics for all variables have significant values that reject the null hypothesis of normal distribution at the 1% significance level. This implies that the use of the QARDL technique to forecast the short-run and long-run connection at a range of quantiles is essential for the current investigation.
Descriptive analysis.
Note: *=P > 0.05, **=P = 0.05, and ***=P < 0.05.
Table II presents the findings of the unit root test for all the study variables. The Zivot & Andrews (ZA) and ADF unit root tests are used to check the unit root process. ADF is a traditional method for examining the unit root of series, whereas ZA adjusts for structural breaks in the data. At the 5% level of significance, both the ZA and ADF tests show that the data are nonstationary (Table II). This signifies that all of the variables have the same order of integration I(1).
Unit Root/Stationarity tests.
Note: ADF and ZA test statistics are specified by the values in the table.
*=P < 0.05, **=P = 0.05, and ***=P > 0.05.
Table III shows the findings of QARDL Model 1. It indicates that the coefficient of adjustment speed or ρ∗ (τ) is significant and negative at all quantiles. The findings suggest that GDPP and other variables such as SE, UE, POPG and INF revert to long-term equilibrium. Furthermore, the coefficient of speed of adjustment is shown to be substantially higher in the initial and middle quantiles, and lowest in the higher quantiles. The coefficient of sharing economy is positive and significant at all quantiles, indicating that the sharing economy significantly contributes to sustainable development in China. This proves that the sharing economy has the potential to strengthen long-term economic growth through its favourable impact on the GDPP. This finding is in line with Curtis and Lehner (2019), Jelinkova et al. (2021) and Karobliene and Pilinkiene (2021) who support the importance of the sharing economy in terms of decreasing resource and energy consumption, and potentially assisting in the achievement of sustainable development. The sharing economy has the potential to significantly improve sustainability by lowering the overall amount of resources needed and reducing pollutants, toxins, and ecological footprints. Additionally, it may encourage sustainable production and consumption methods, use sustainable energy, restructure infrastructure and cities, and minimize educational, gender, and income disparities, while simultaneously boosting life standards and life quality (Pérez-Pérez et al., 2021). The coefficient of unemployment is significant and negative only across the low to medium range of quantiles (0.10–0.60). This shows that a rise in unemployment is associated with a decline in sustainable development. The findings of Akinnifesi (1986), Rowley and Feather (1987) and Anghel et al. (2017) support our findings, arguing that a rise in unemployment is associated with a decline in sustainable growth, because unemployment is not only associated with economic issues but also with social issues such as increasing poverty and crime rates and mental health issues, which suggest negative consequences for sustainable development (Šileika & Bekerytė, 2013). The findings reveal that the impact of population growth is insignificant across all quantiles (0.10–0.95), while inflation, as expected, exerts a negative influence on sustainable growth, but only at medium to high quantiles (0.60- 0.95) in line with the findings of Adaramola and Dada (2020) and Wollie (2018), Madurapperuma (2016), who argue that inflation is a hurdle in the way of economies growing in a sustainable manner.
QARDL results (model 1).
Note: The table provides the quantile estimation results. The t-statistics are in brackets. ***, ** and * show 1%, 5% and 10% levels of significance, respectively. Source: Author's estimations.
Table IV provides the results of the Wald test for parameter constancy. In China's case, the null hypothesis of ECM linearity is clearly rejected. Similarly, integrating the coefficient of GDPP and SE is indicated to be significant, which suggests that the long-run association between EF and EC differs across quantiles. The estimated coefficients of UE, POPG, and INF likewise yield the same results, with significant Wald statistics observed at various quantiles.
Wald test results for Model 1.
Note: The p-values are in square brackets. ***, ** and * indicate significance at the 1%, 5% and 10% levels, respectively.
Source: Author's estimations.
The Granger causality findings are given in Table V, below. We observe a two way causality amongst all the variables at all quantiles, i.e. from 0.05 to 0.95. The results show that present and previous realizations of EI, EC, ENER, GDP, and GDPP are useful indicators of EF, and vice versa.
Granger causality in quantile test results for Model 1.
Source: Author's estimation.
We now proceed to the QARDL findings for Model 2, and the corresponding results are given in Table VI below.
QARDL results (Model 2).
As in Model 1, all the variables in Model 2 are statistically significant. The coefficient of adjustment speed ρ∗ (τ) is significant and negative at all quantiles, and EEF, EP, POPG, and SE revert to long-term equilibrium. Specifically, the findings indicate that the sharing economy exerts a positive influence on energy efficiency measured in terms of energy intensity, as it has a negative impact on energy intensity at higher quantiles only (0.80–0.95). The sharing economy is intended to promote energy efficiency by promoting the use of underutilized resources. This supports earlier research which shows that the sharing economy helps conserve energy, reduce waste, and cut carbon footprints and emissions (Dabbous & Tarhini, 2021; Leismann et al., 2013). Thus, the empirical findings indicate that the sharing economy is an economic engine with the potential to have long-term effects on consumption by allowing consumers to access resources without owning them, and on the environment by increasing energy efficiency. The energy price is found to exert a negative influence on energy intensity at all quantiles. This implies that, with an increase in energy prices, there comes a reduction in energy use which leads to more efficient use of energy (0.10–0.90), in line with Filipović et al. (2015), Fitriyanto and Iskandar (2019), and Rajbhandari and Zhang (2018). Lastly, population growth is observed to influence energy intensity positively only at lower and middle quantiles, indicating that with an increase in population, the consumption of energy increases, raising energy intensity and reducing energy efficiency. The studies of Avtar et al. (2019), Kaushal (2018), and De Vita et al. (2006) support these results.
The Wald test results for parameter constancy for Model 2 are provided in Table VII, below. Again we reject the null hypothesis of ECM linearity. The integrating coefficients of EEF and SE are observed to be significant, which suggests that the long-run association between SE and EEF differs across quantiles. Significant Wald statistics are observed at several quantiles, and the calculated coefficients of EI and POPG produce the same results.
Wald test results for Model 2.
Variable | Wald statistics (p-value) |
---|---|
Ρ | 11.111*** |
[0.000] | |
ΒSE | 11.072*** |
[0.002] | |
ΒEI | 3.551*** |
[0.010] | |
ΒPOPG | 2.331*** |
[0.005] | |
φ1 | 14.459*** |
[0.004] | |
ω0 | 3.545** |
[0.010] | |
λ0 | 2.664 |
[0.444] | |
θ0 | 5.551*** |
[0.000] |
Notes: The p-values are in square brackets. ***, ** and * indicate significance at the 1%, 5% and 10% levels, respectively.
Source: Author's estimations.
Table VIII gives the Granger causality results for Model 2. A two way causal association is observed between EEF and all other variables. Current and previous values of EP, POPG, and SE are found to be clear indicators of the values of EEF.
Granger causality in quantile test results for Model 2.
Source: Author's estimation.
Although China has made significant achievements in terms of the sharing economy, its role in achieving sustainable economic development and energy efficiency has not yet been explored. China has made tremendous improvements in terms of economic growth, but its energy structure is fossil fuel dominated, which raises serious concerns about sustainability in terms of economic development and energy consumption. Therefore, estimating the role that the sharing economy plays in achieving sustainable economic growth and efficiency in energy consumption is of immense importance in the context of China. This study estimates the impact of the sharing economy on energy efficiency and sustainable economic development by applying the QARDL estimation technique. We propose two models for empirical estimation: Model 1 for sustainable development measurement in terms of the sharing economy, controlling for population growth, inflation, and unemployment rate; and Model 2 measuring the impact of the sharing economy on energy efficiency, controlling for energy prices and population growth. According to the estimations, the sharing economy makes a significant contribution to both achieving energy efficiency and sustainable development. Specifically, the sharing economy has a positive impact on sustainable development at all quantiles, but its effect on energy efficiency is significant only at high quantiles (0.80–0.95). All the control variables have significant impacts on sustainable development and energy efficiency, either positive or negative.
The empirical findings of the study have various practical consequences. They show that the sharing economy is effective and necessary in the business world for supporting sustainable production and consumption processes, and motivates individuals to engage in sharing behaviours. Secondly, the sharing economy is a response to economies' expanding issues, accommodating an increasing number of people with limited resources. As a result, policymakers are motivated to stimulate the expansion of this new economic activity. Governments also have a part to play in enabling models of sharing to reach their full potential for sustainable growth and energy efficiency by enacting suitable taxation and legislation policies. Thirdly, the findings show that the shared economy helps reduce carbon emissions and pollution, which benefits the environment. Consumers should be more aware of this vital problem, and policymakers and educational institutions should urge them to take on more sharing behaviours and move towards consumer choices that lessen environmental damage. Fourthly, due to the decline in oil prices and rise in renewable energy costs, the association between energy consumption, growth and energy price is becoming increasingly important, particularly for developing nations. As a result, the findings of Model 2, examining the influence of the sharing economy on efficient use of energy, controlling for POPG and prices of energy, provide policymakers with useful information that more relevant policies should be adopted to promote the use of underutilized resources. Energy prices should be maintained by the government up to the level that fulfils the energy requirements of all sectors, and they should curb the intensive utilization of energy. More limits should be imposed on rapid population growth.
Like other studies, the current study is not without limitations. This study explores the sharing economy's role in energy efficiency and sustainable development in China. Future studies could further explore this association by considering different countries or groups of countries. Methodologically, several advanced estimation techniques such as MMQR, CS-ARDL, and non-linear ARDL estimations could also be applied by future studies.
FundingThis paper was supported by Project of New Liberal Arts Project of Ministry of Education (Grant No.: 2021140078).